↗ arXiv ↗ Hugging Face ↗ Papers with Code
TL;DR#
Prior research on task-agnostic image generation using diffusion transformers yielded suboptimal results due to high computational costs and limitations in generating high-fidelity images. This paper challenges this notion by proposing that text-to-image models already possess inherent in-context generation abilities, requiring only minimal tuning to effectively activate them. The study demonstrates this through several experiments showing effective in-context generation without additional tuning. This finding counters the idea of complex model reformulations for task-agnostic generation.
The proposed solution, In-Context LoRA (IC-LORA), involves a simple pipeline. First, images are concatenated instead of tokens, enabling joint captioning. Then, task-specific LoRA tuning uses minimal data (20-100 samples), thus significantly reducing computational cost. IC-LORA requires no modifications to the original diffusion transformer model; it only changes the training data. Remarkably, the pipeline generates high-fidelity images. While task-specific in terms of tuning data, the architecture and pipeline remain task-agnostic, offering a powerful, efficient tool for the research community.
Key Takeaways#
Why does it matter?#
This paper is important because it offers a novel and efficient approach to adapt existing text-to-image models for diverse generative tasks. It challenges existing assumptions by demonstrating the inherent in-context learning capabilities of these models, requiring only minimal tuning. This significantly reduces the computational resources and data requirements, making it highly relevant to researchers working with limited resources. The framework’s task-agnostic nature opens exciting avenues for further research in efficient and versatile image generation systems.
Visual Insights#
🔼 Figure 1 presents example outputs from the In-Context LoRA (IC-LoRA) method. It showcases three distinct tasks: portrait photography, font design, and home decor. For each task, four images were generated simultaneously using a single diffusion process. Importantly, separate IC-LoRA models were trained for each task using a small dataset (20-100 samples) of task-specific examples. The figure highlights the capability of IC-LoRA to generate high-fidelity images while requiring only minimal tuning for each task.
read the caption
Figure 1: In-Context LoRA Generation Examples. Three tasks from top to bottom: portrait photography, font design, and home decoration. For each task, four images are generated simultaneously within a single diffusion process using In-Context LoRA models that are tuned specifically for each task.
Full paper#
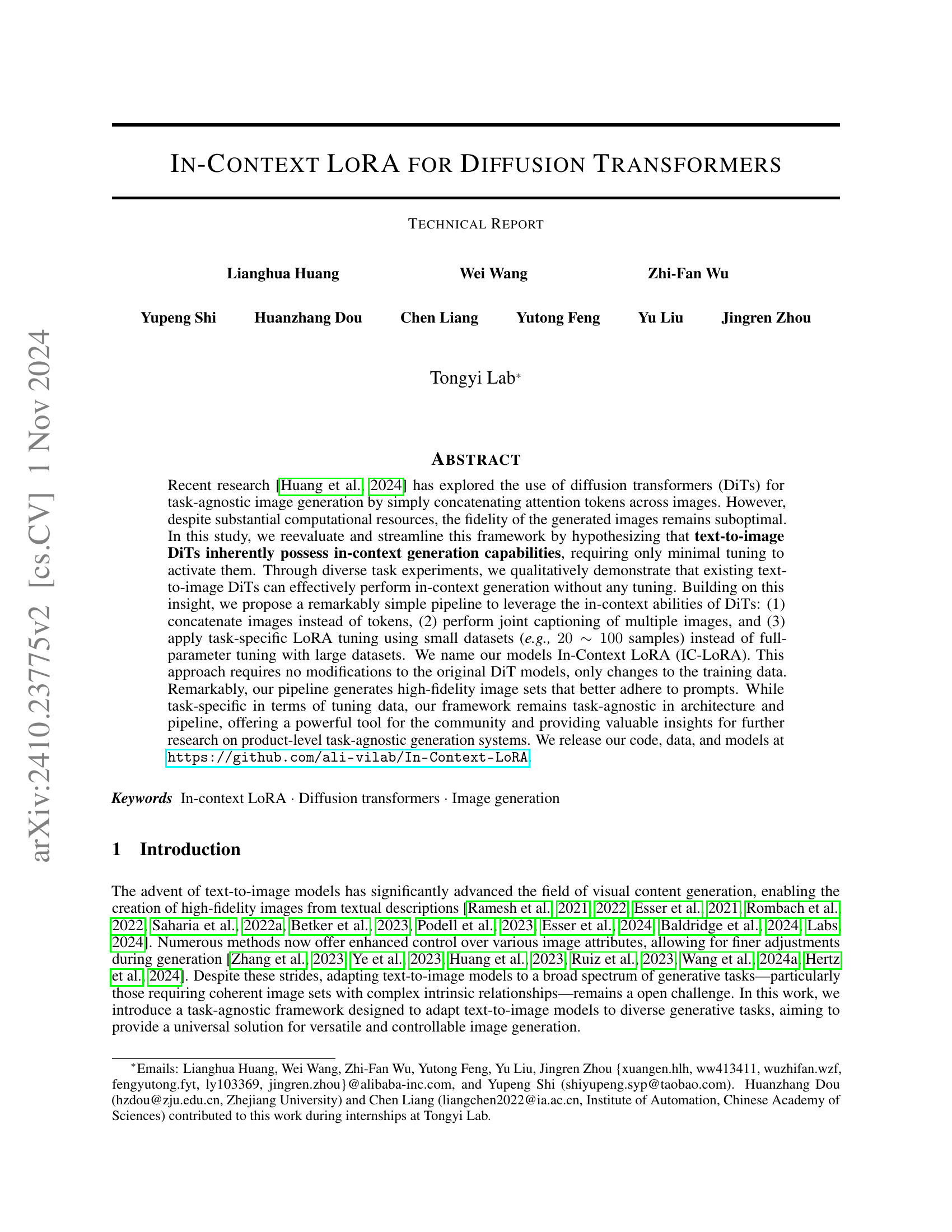
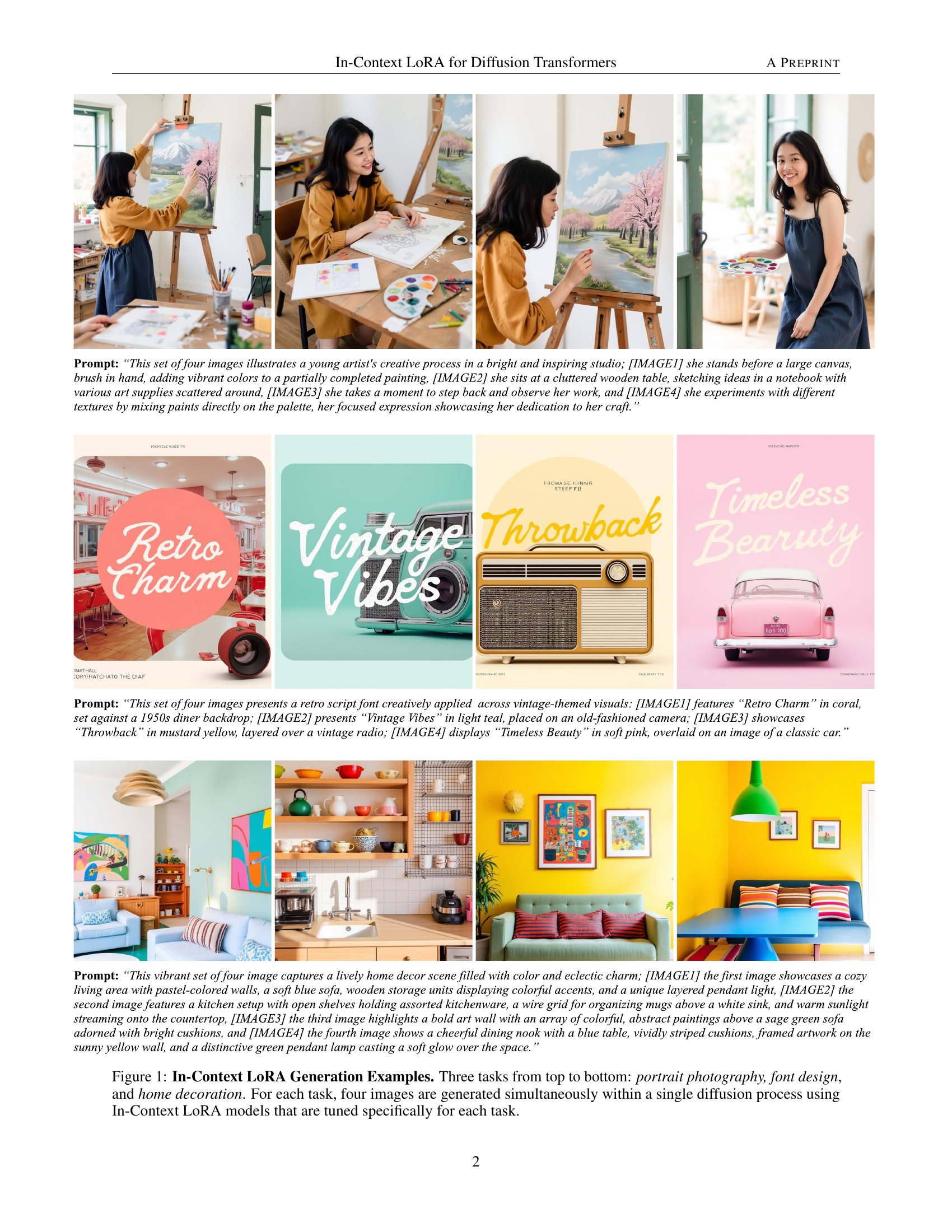
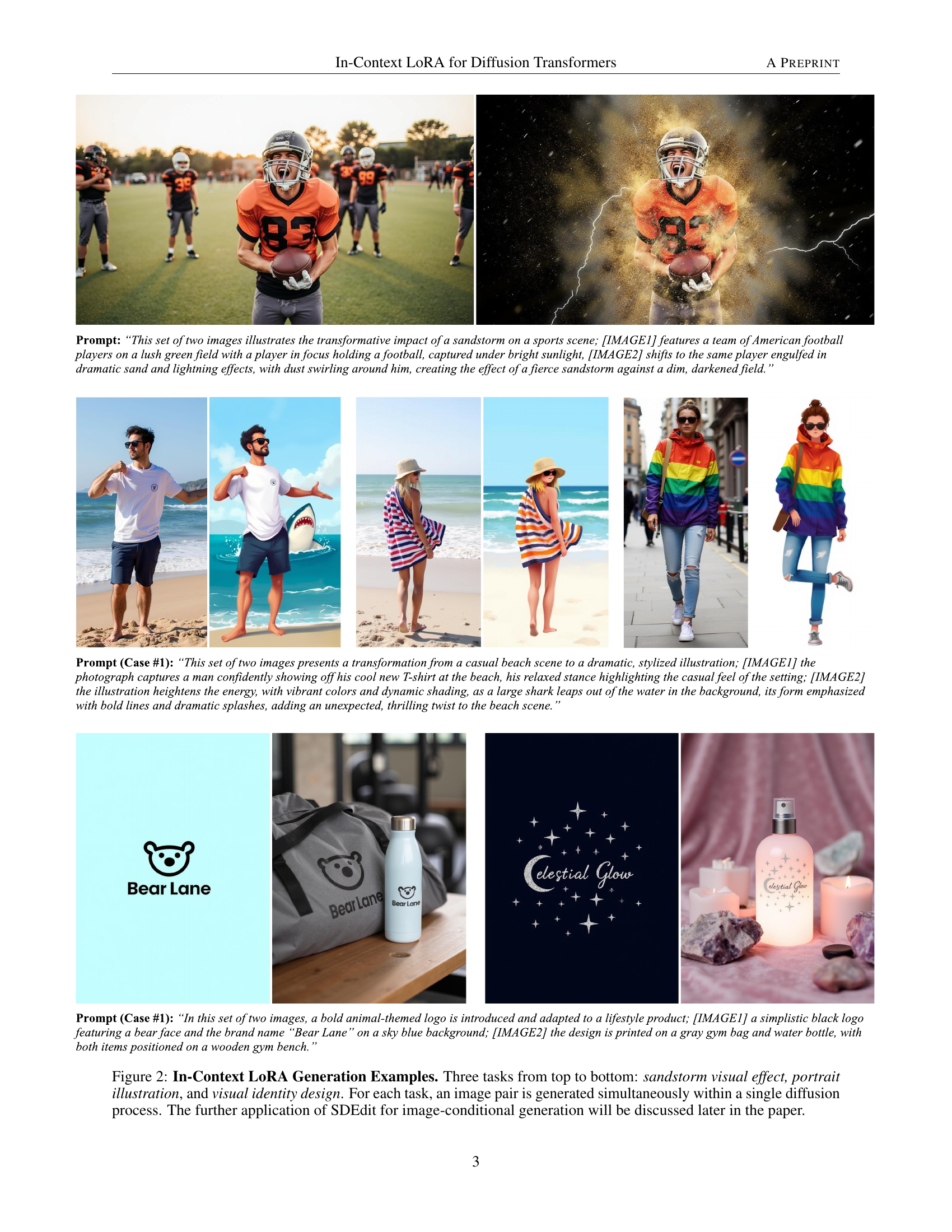
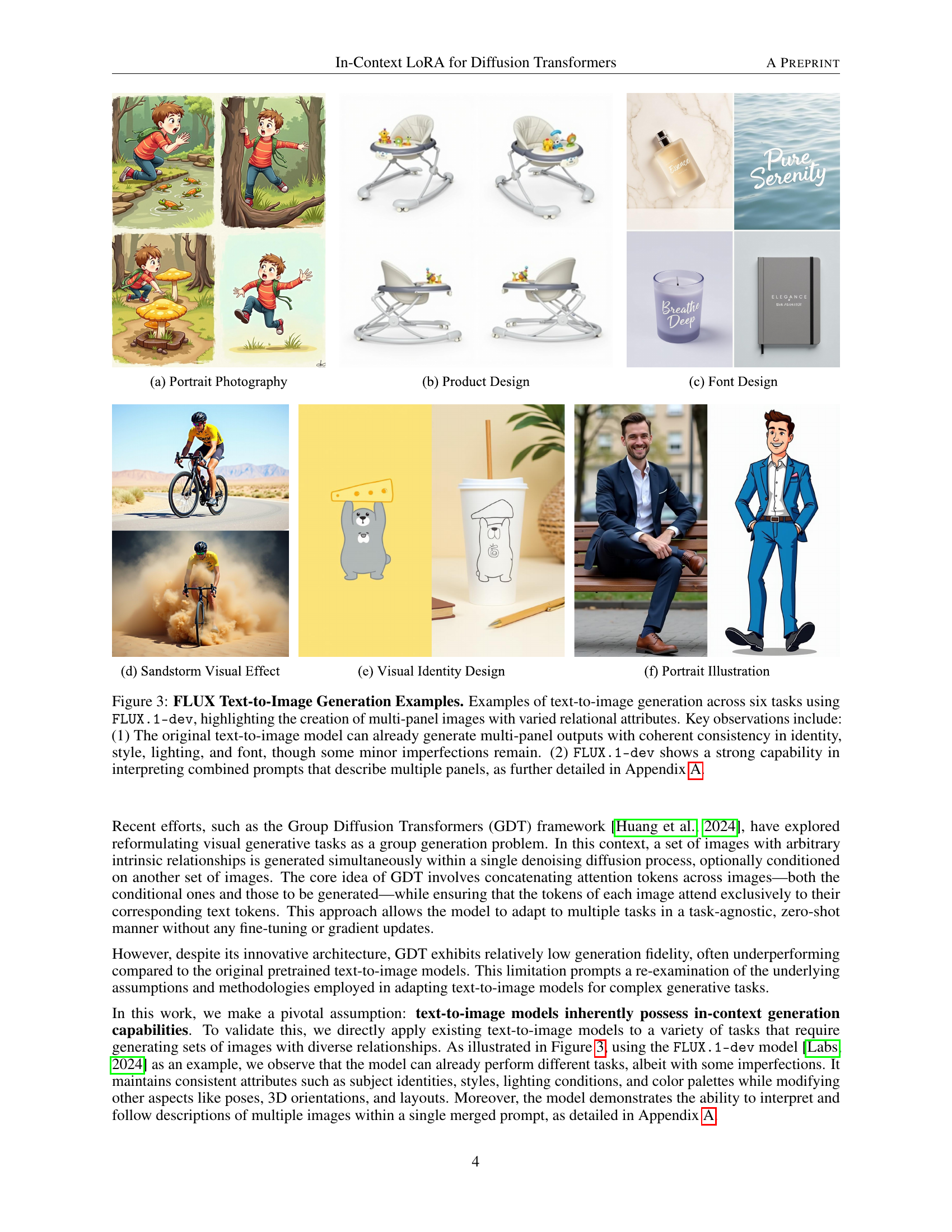
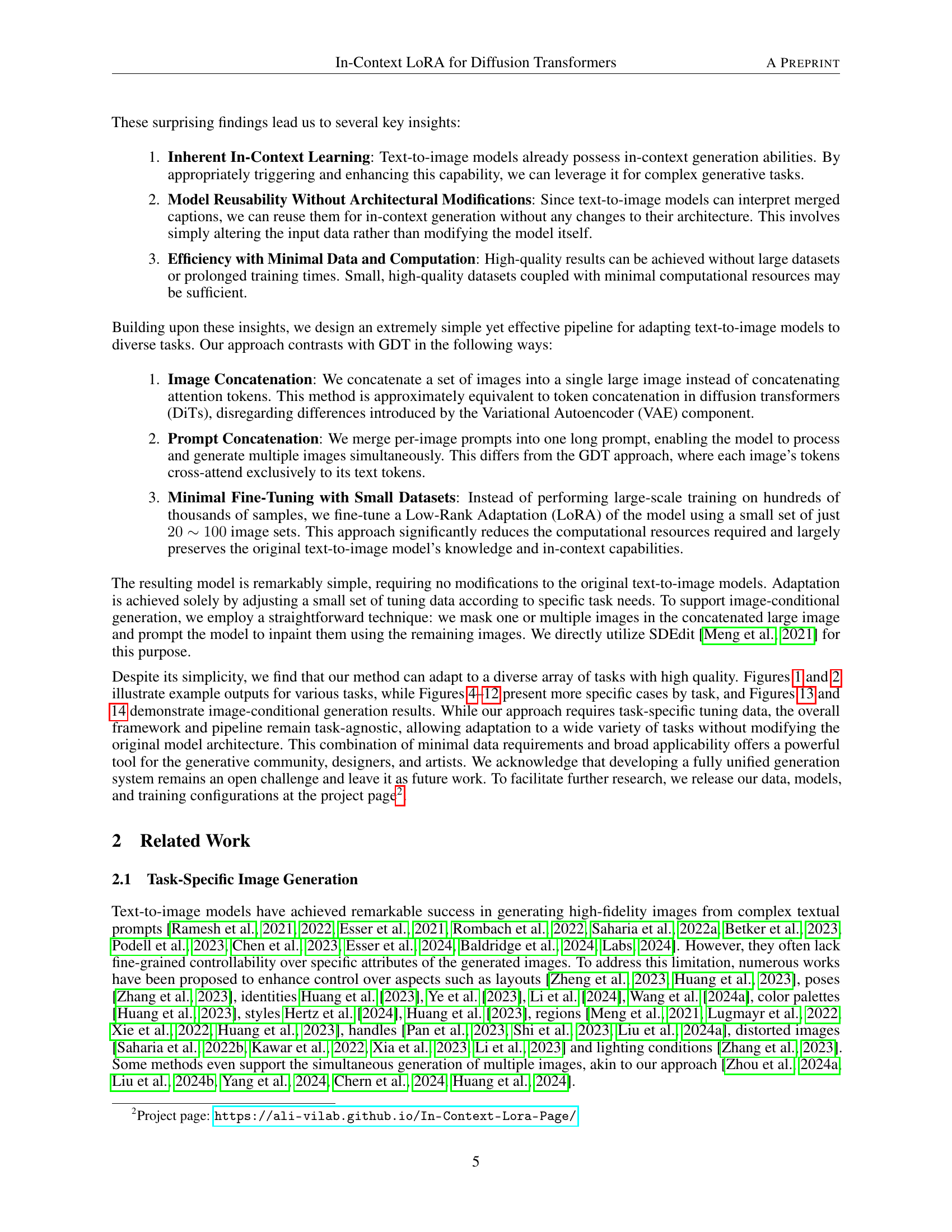
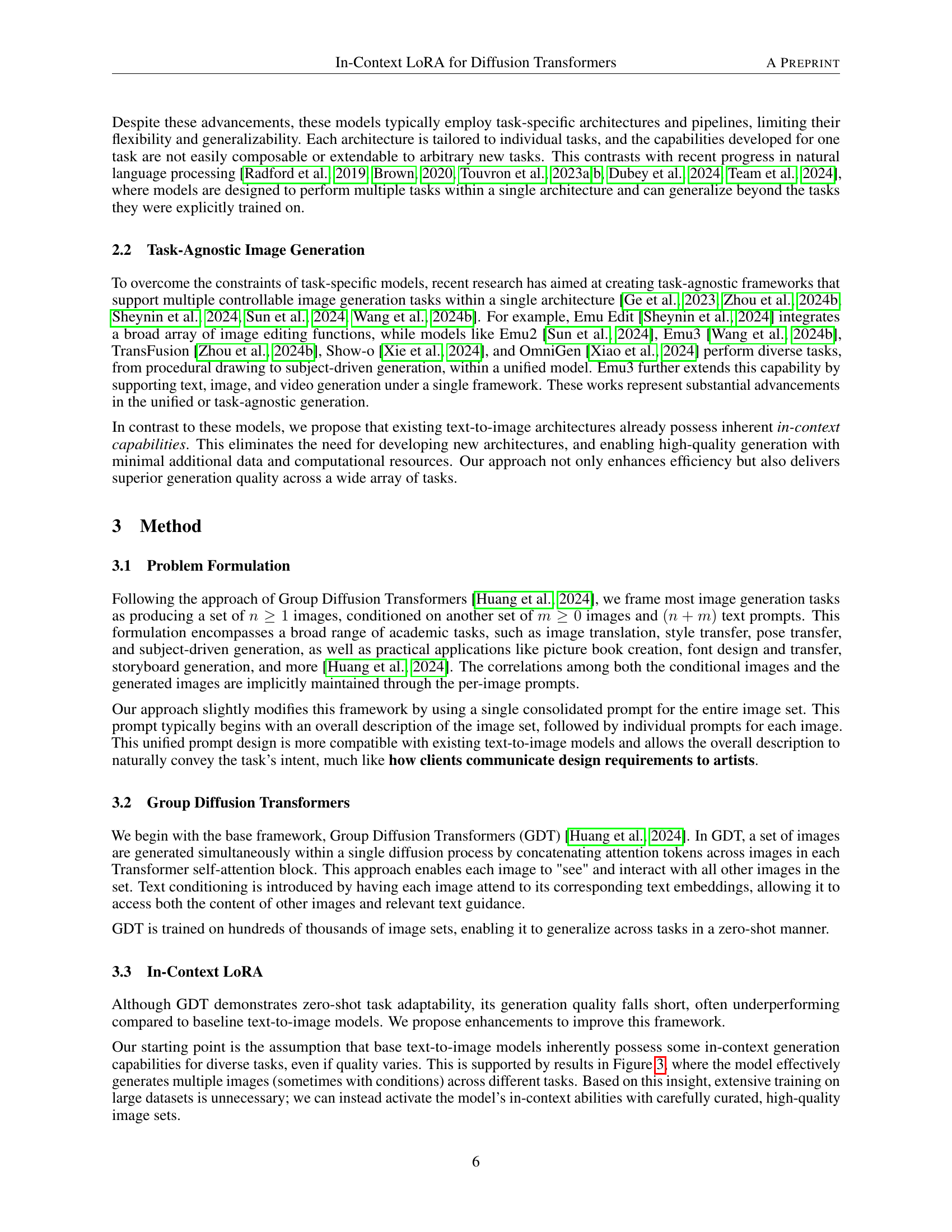
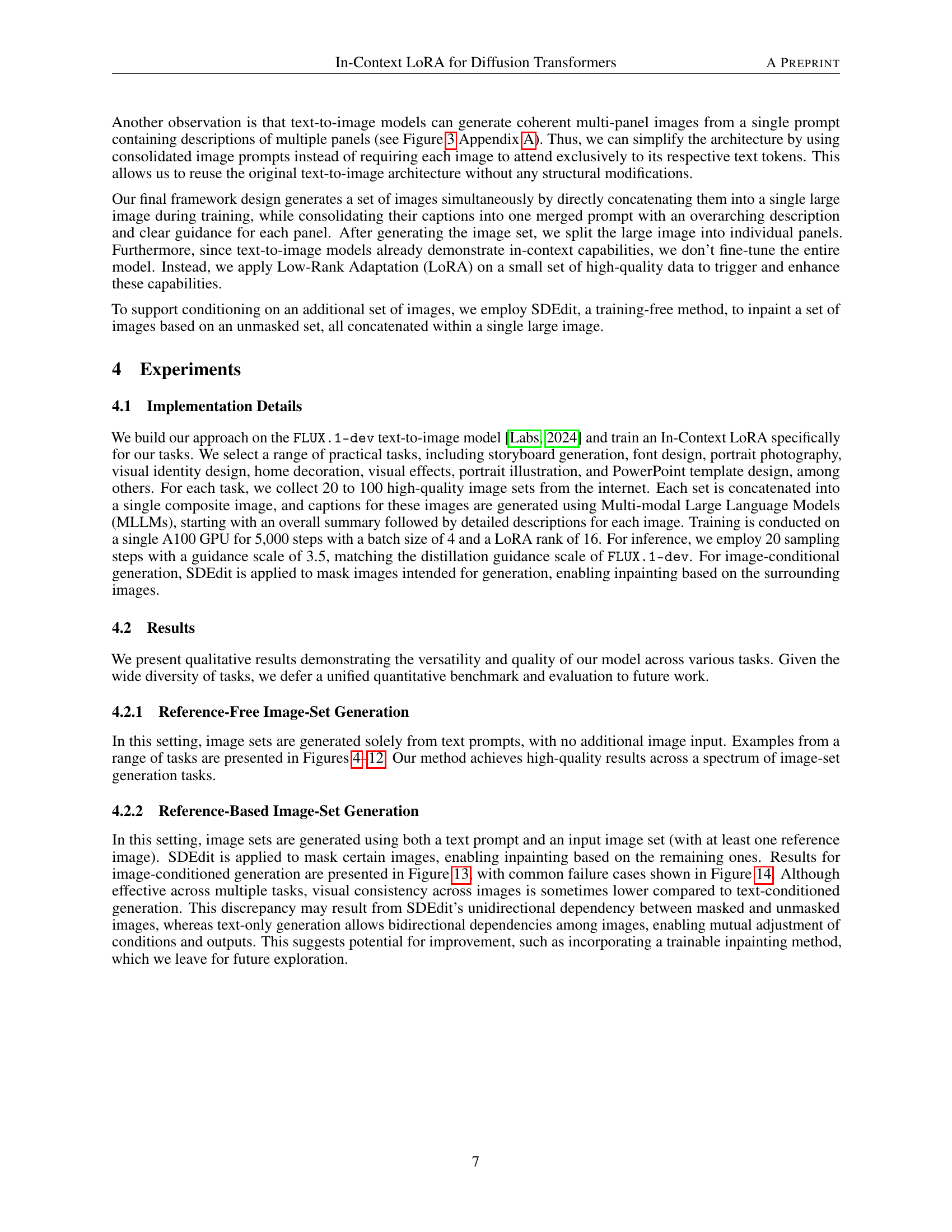
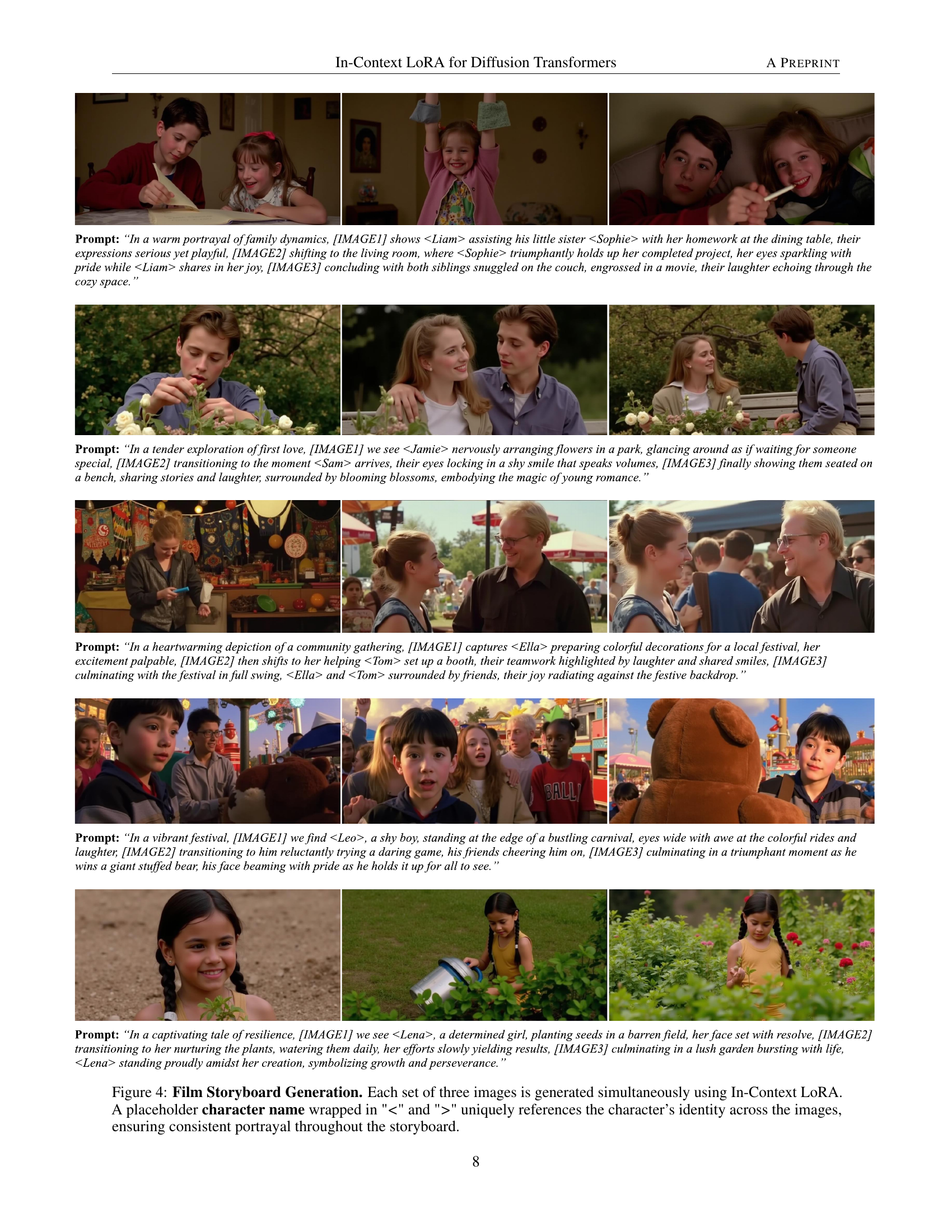
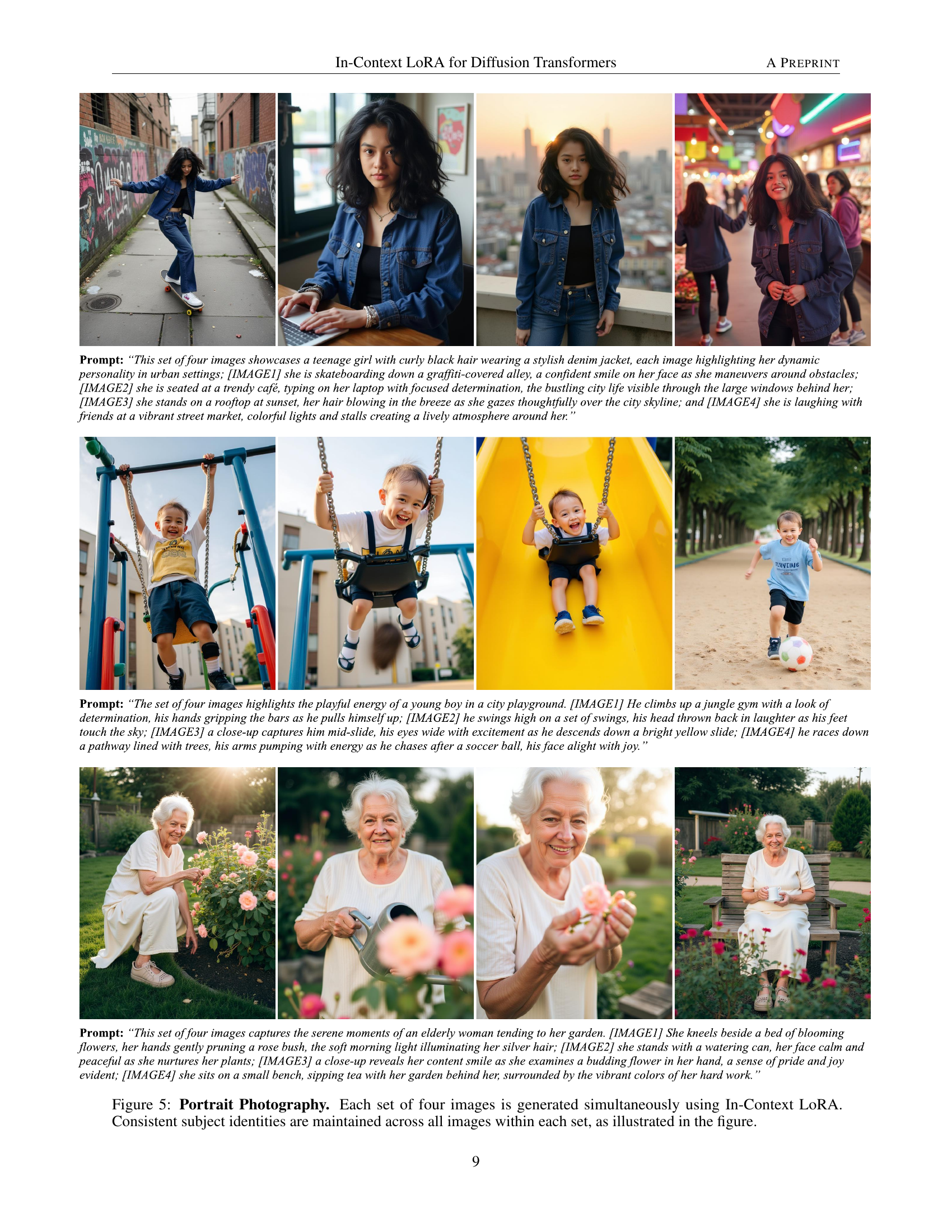
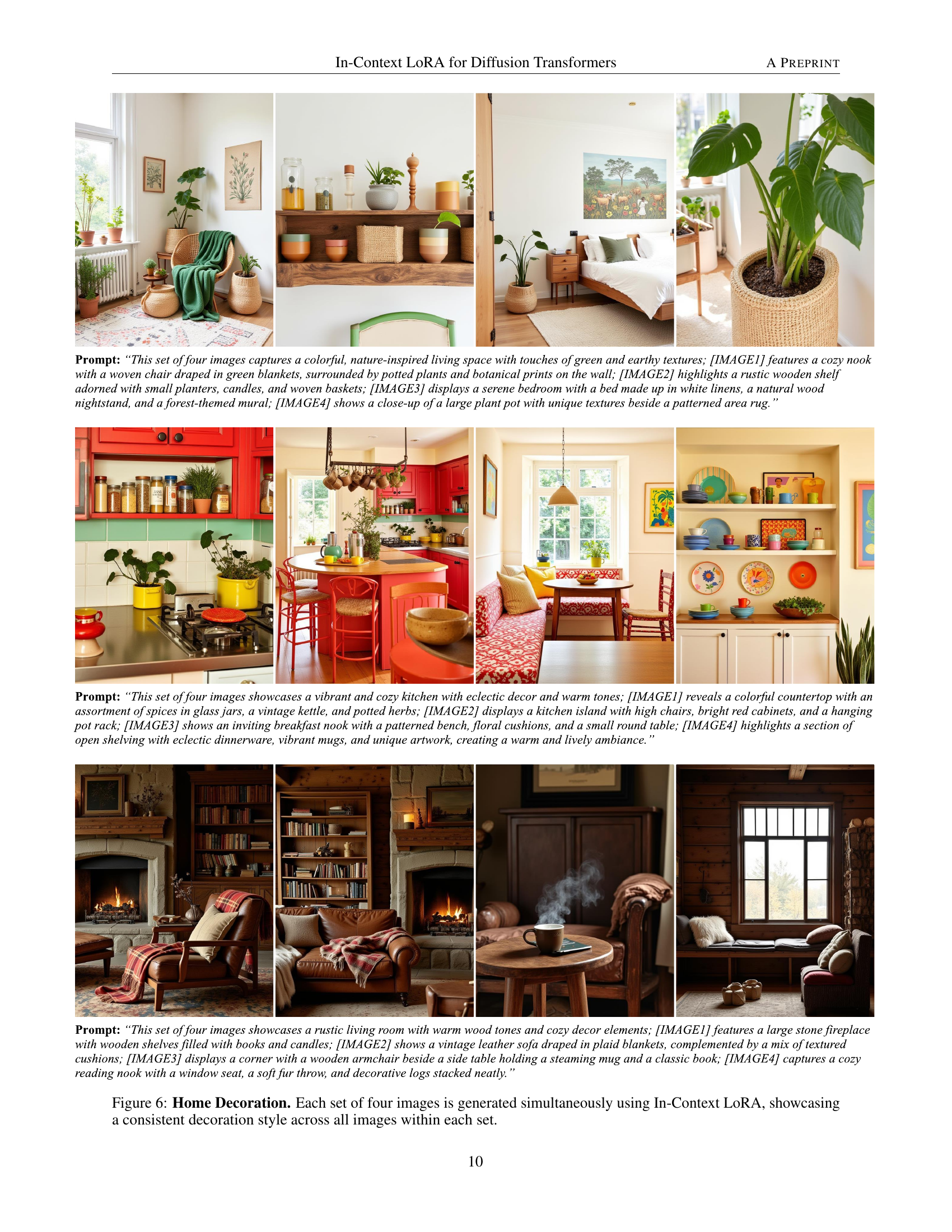
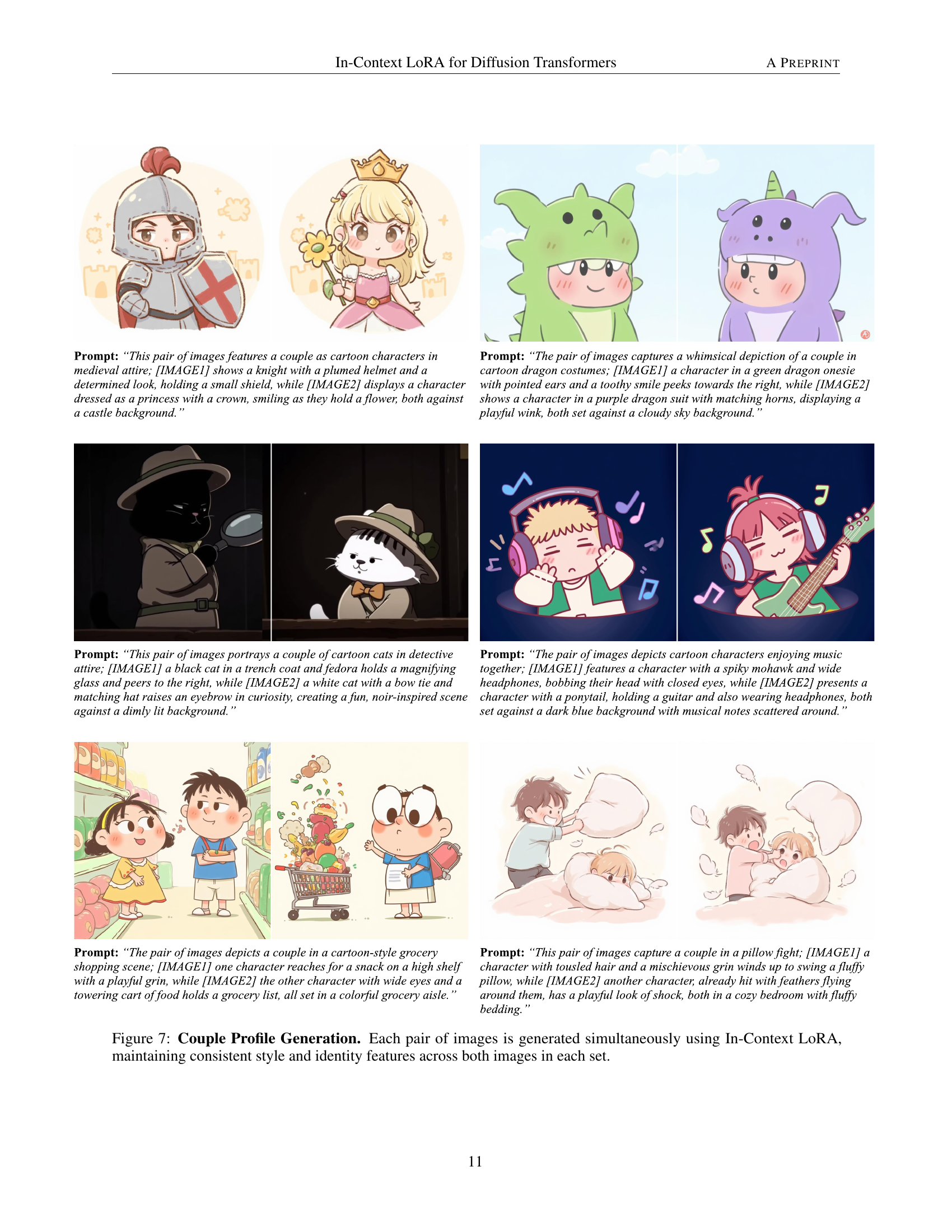
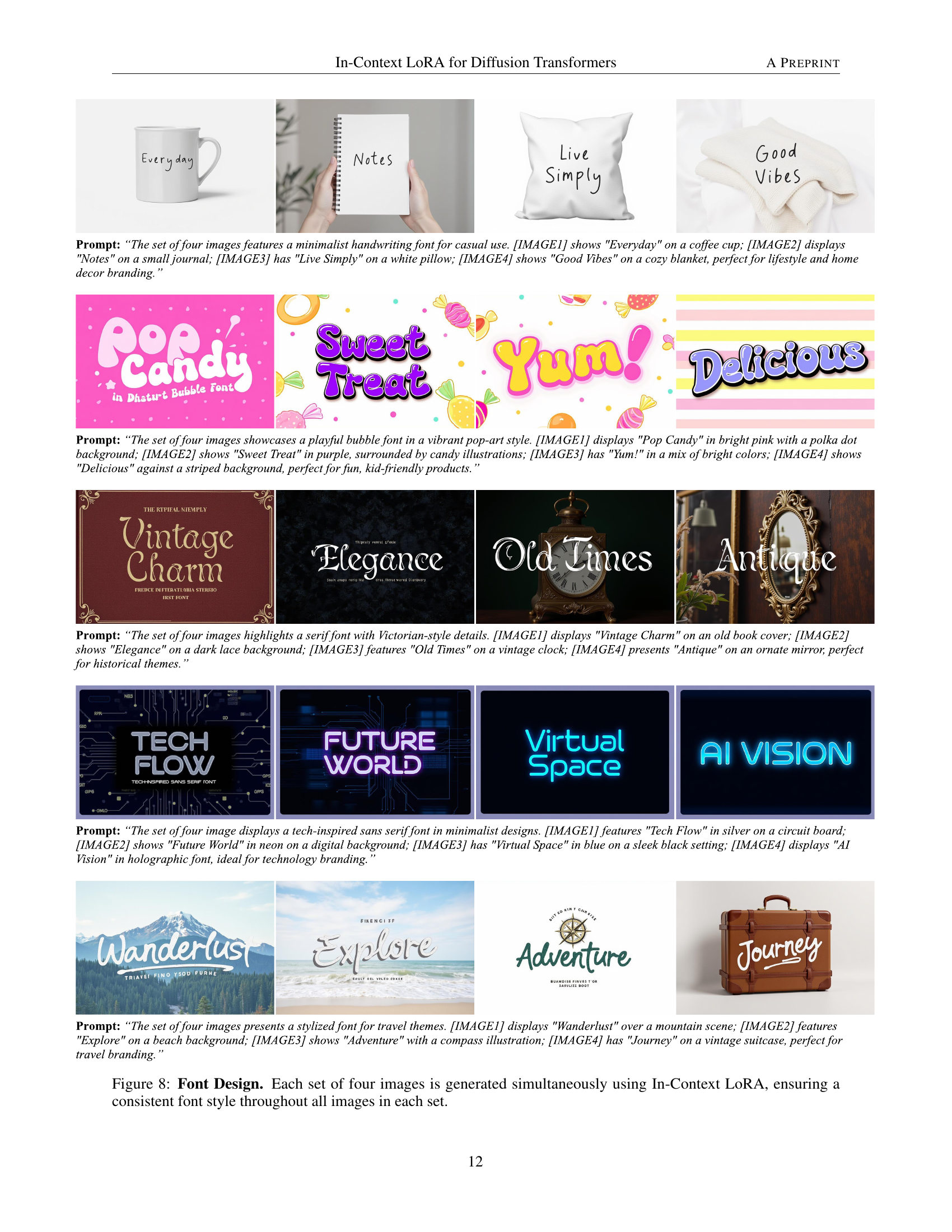
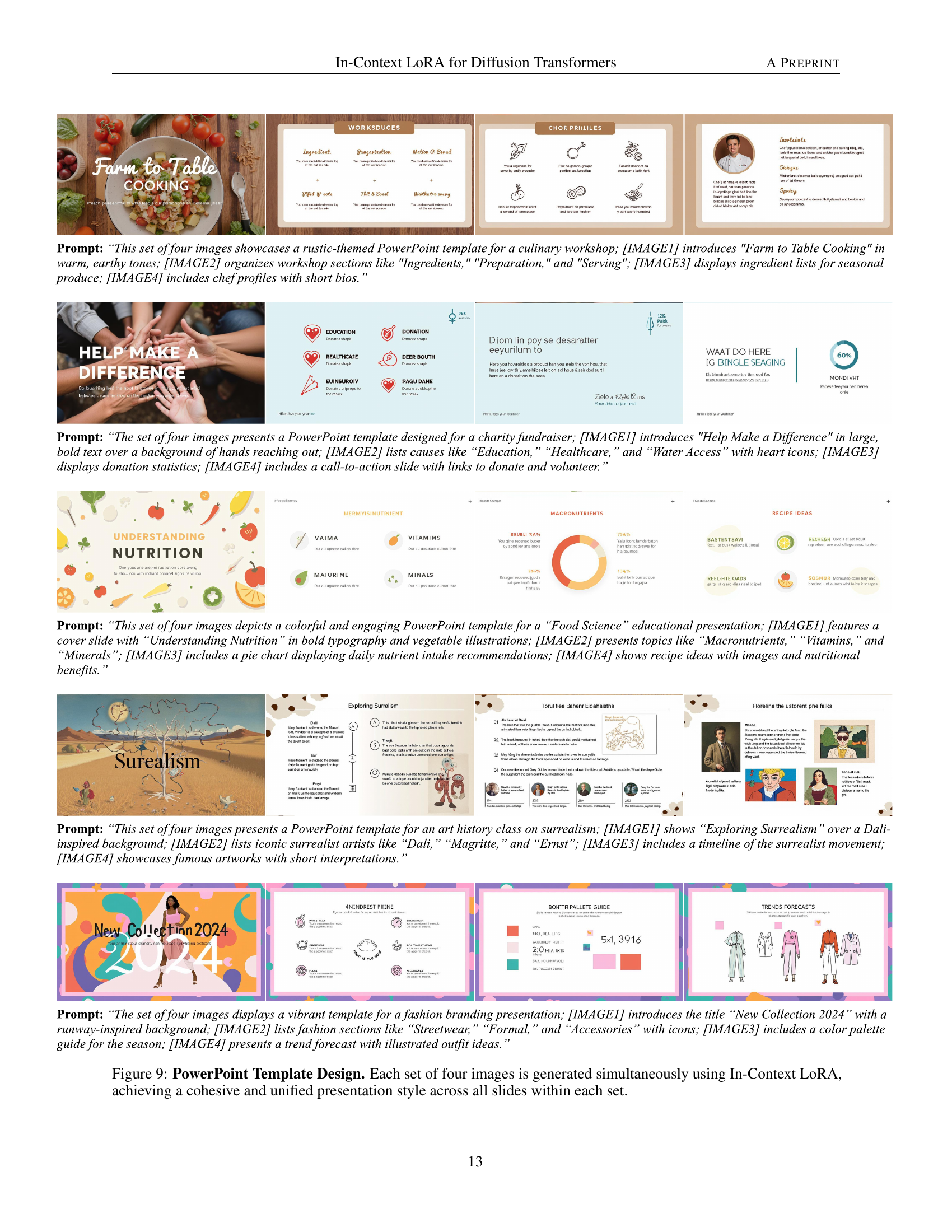
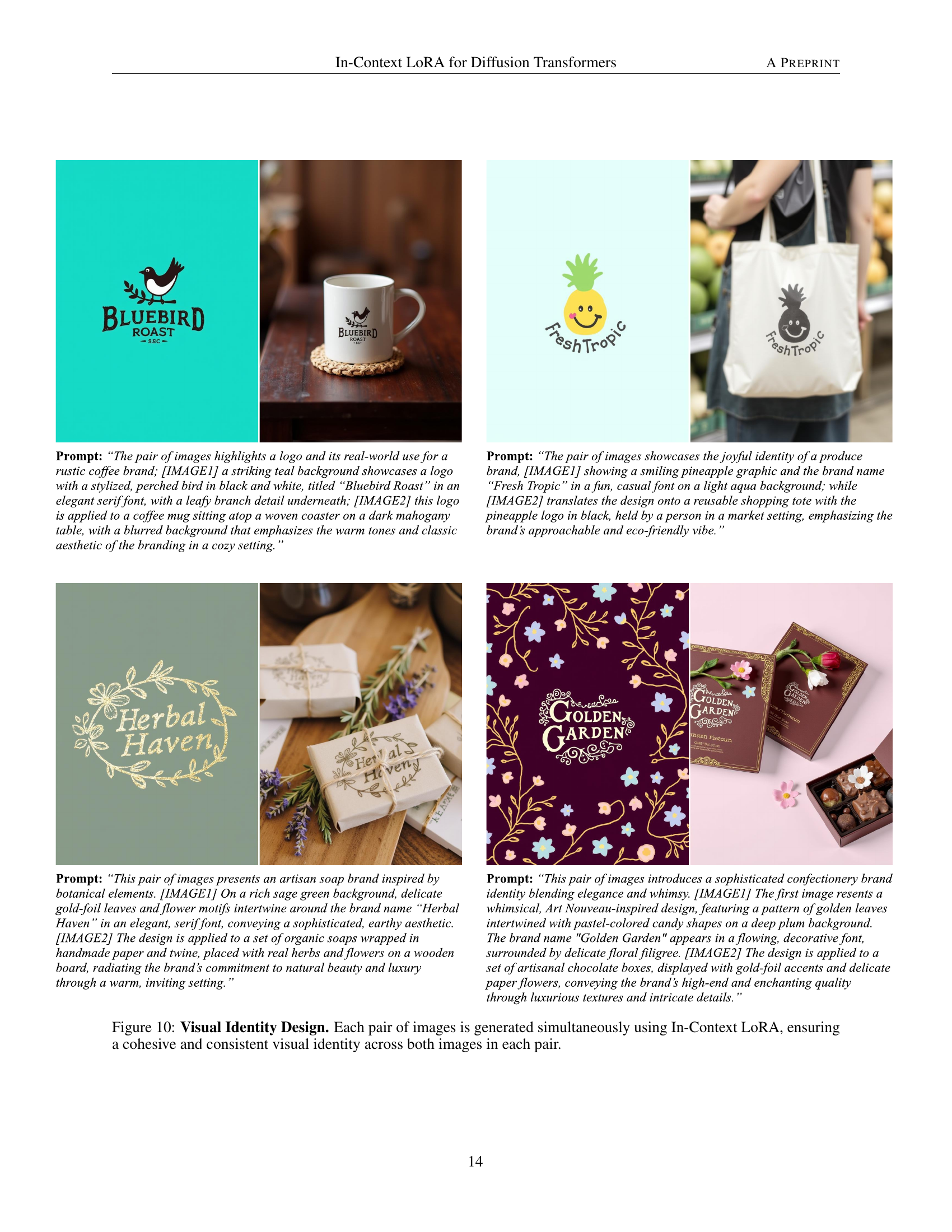
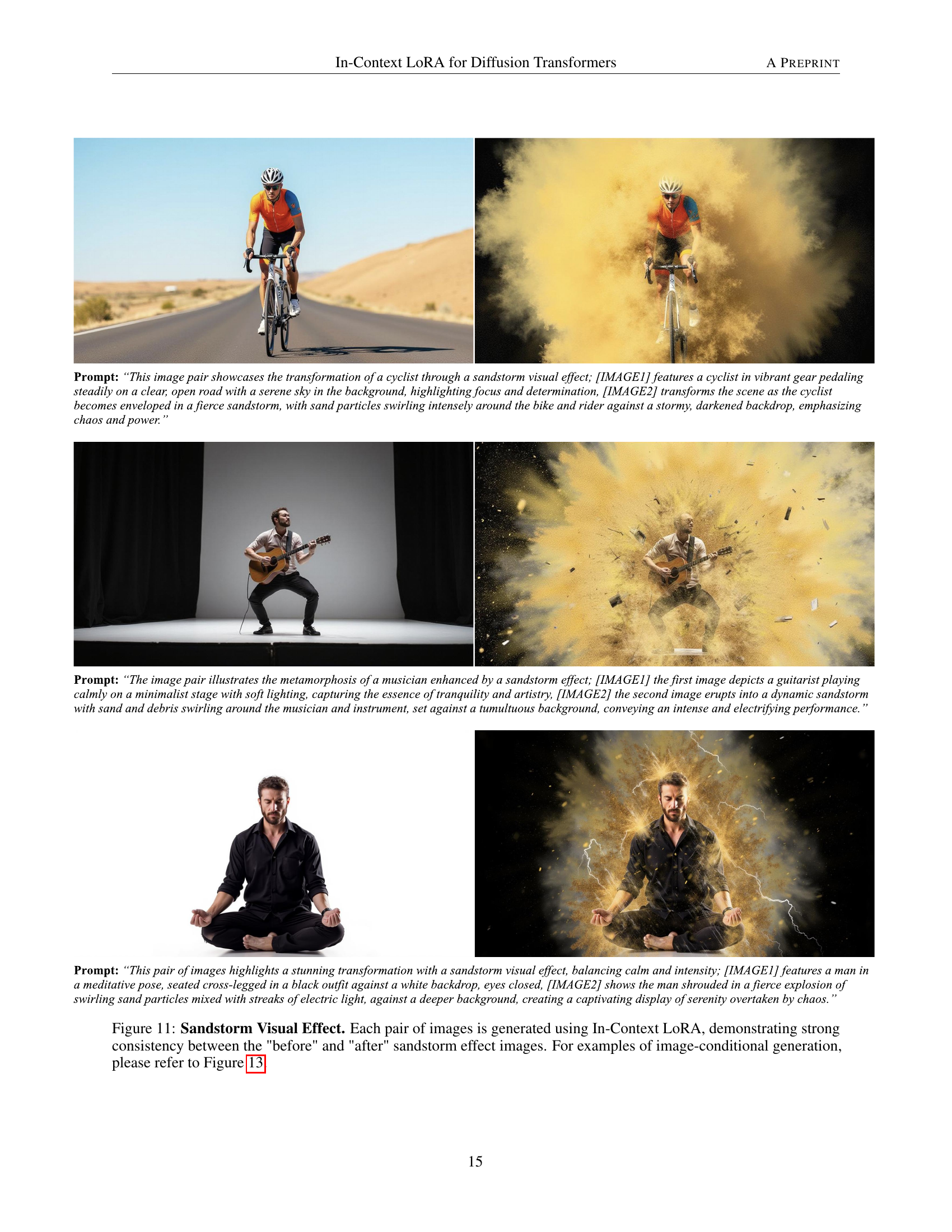
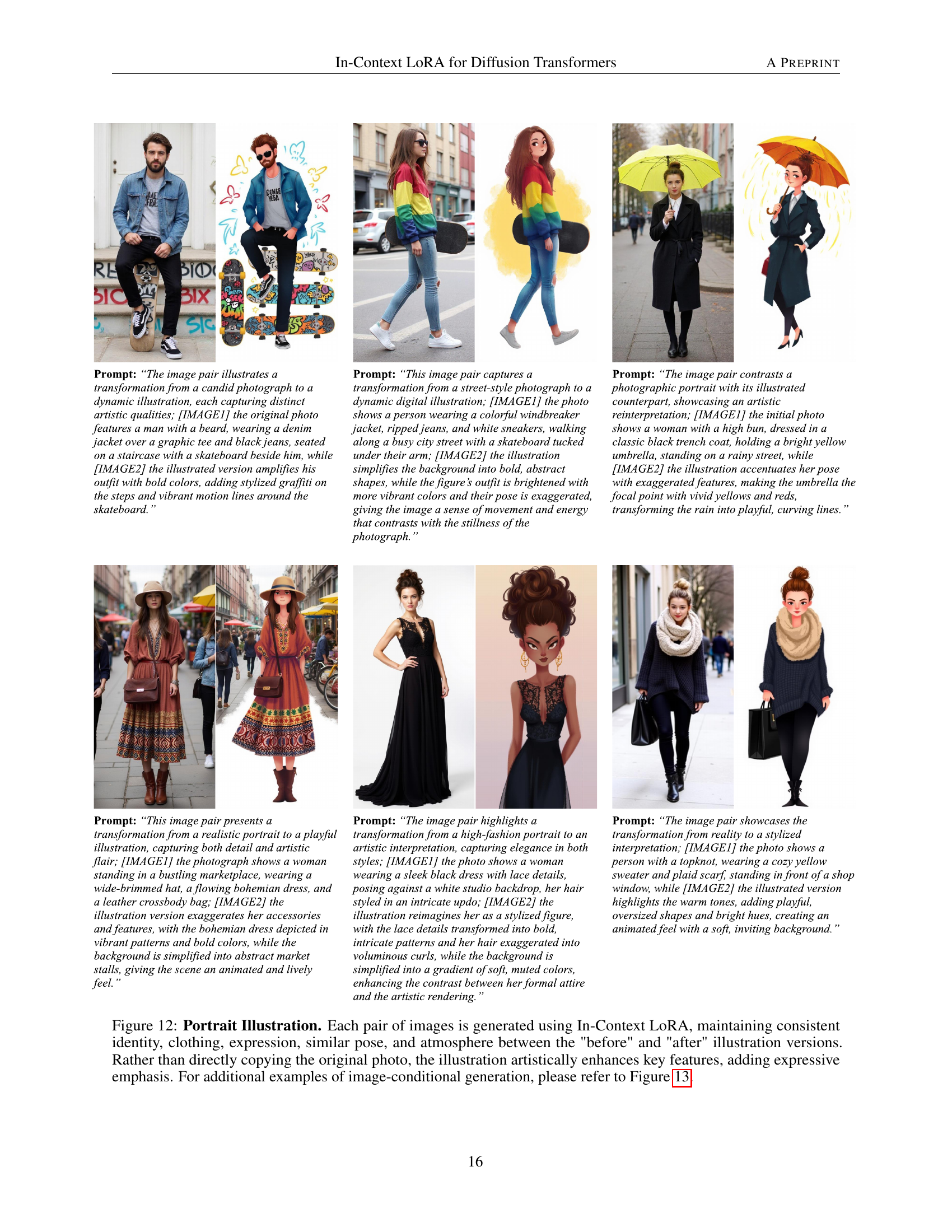
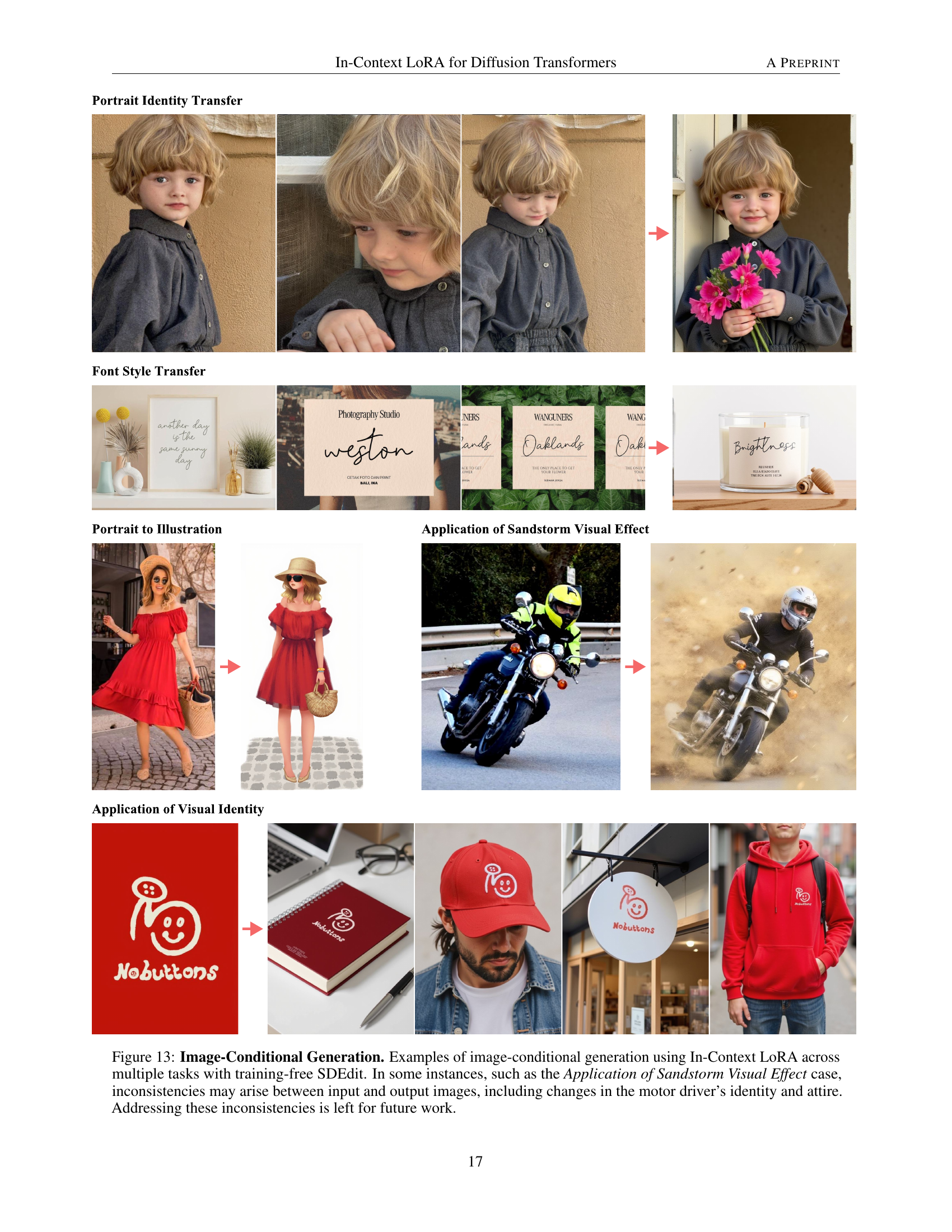
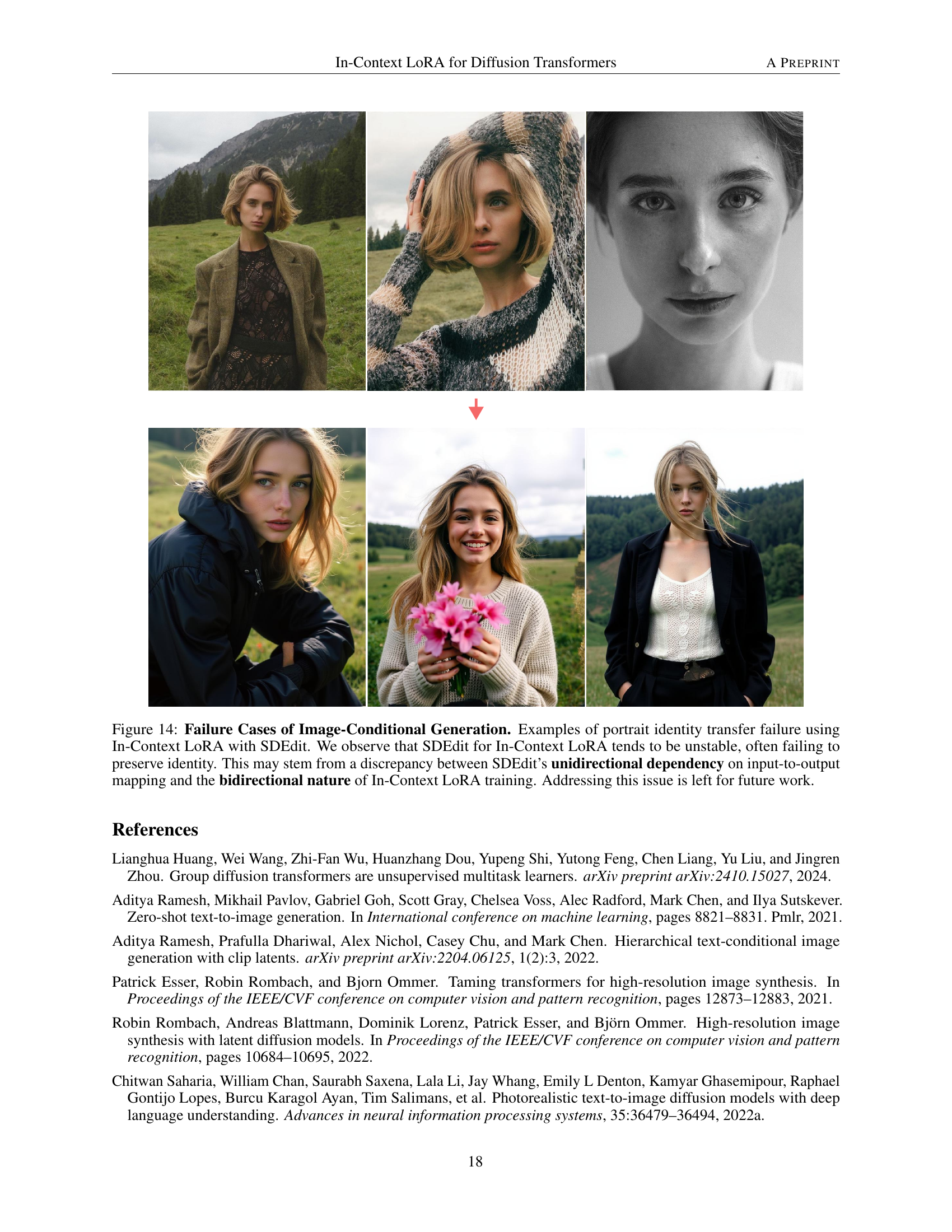
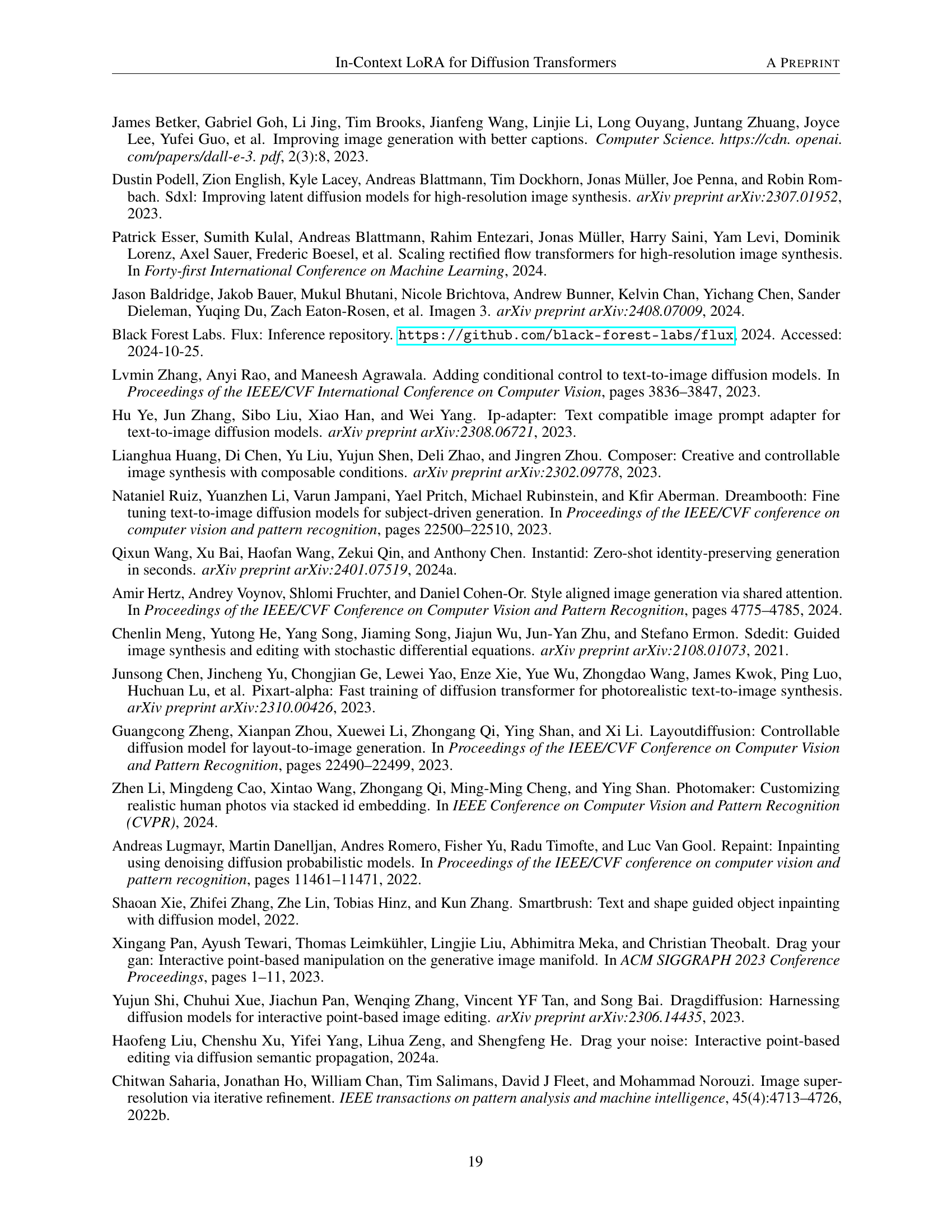
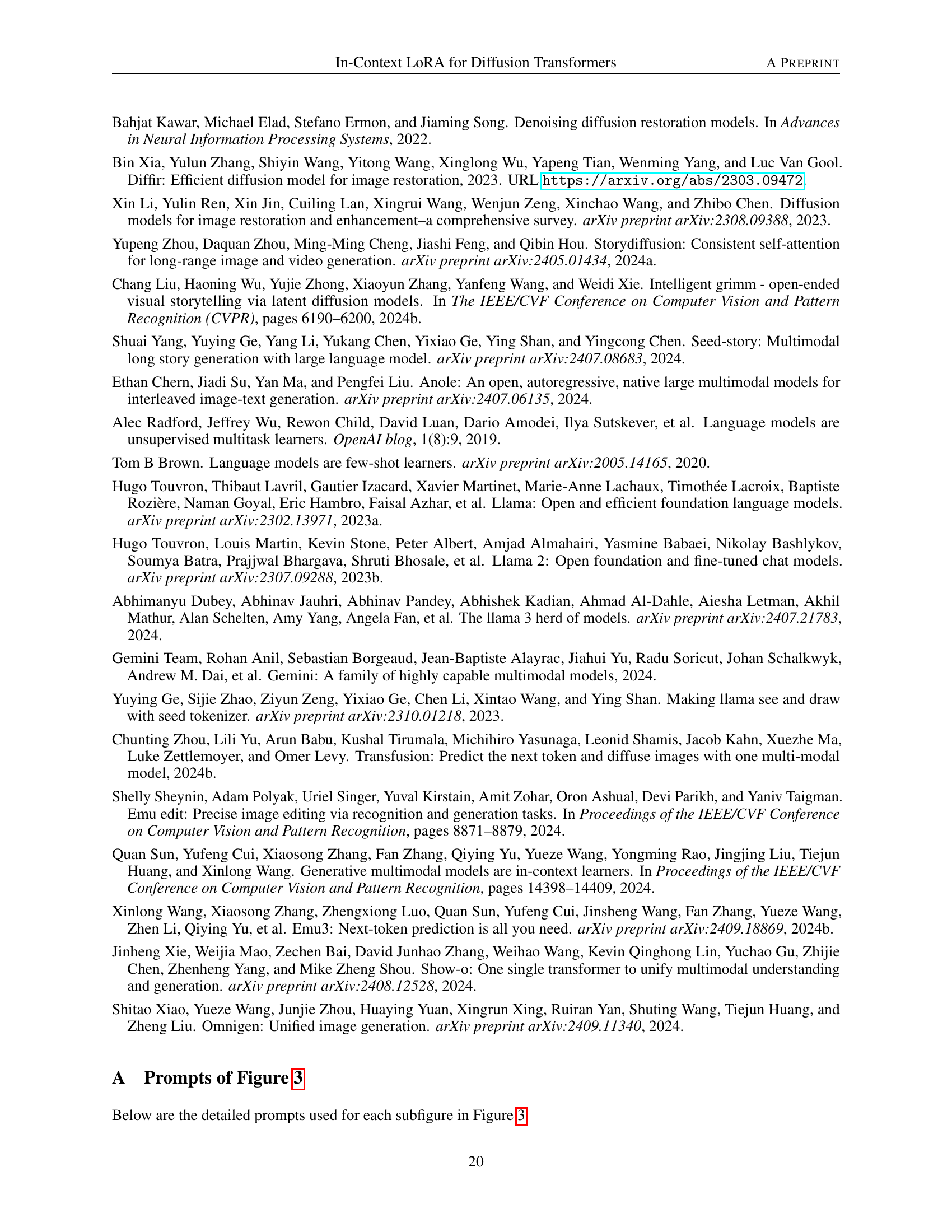