โ arXiv โ Hugging Face โ Papers with Code
TL;DR#
Current multilingual embedding models often underperform on Arabic NLP tasks due to the language’s unique morphology, diverse dialects, and cultural nuances. Existing benchmarks also lack sufficient coverage of these aspects. This necessitates the development of Arabic-specific embedding models and a comprehensive evaluation framework.
This paper introduces Swan, a family of Arabic-centric embedding models, focusing on both small and large scale applications. It also proposes ArabicMTEB, a benchmark that evaluates cross-lingual, multi-dialectal, and multi-cultural performance on eight diverse tasks. Swan-Large achieves state-of-the-art results, while Swan-Small surpasses Multilingual-E5-base. The research demonstrates that Swan models are dialectally and culturally aware and provide valuable resources for future NLP research.
Key Takeaways#
Why does it matter?#
This paper is crucial for researchers in Arabic NLP because it introduces Swan, a family of dialect-aware Arabic embedding models, and ArabicMTEB, a comprehensive benchmark for evaluating Arabic text embeddings across diverse tasks. This work addresses the scarcity of high-quality Arabic resources and provides valuable tools and datasets for advancing research in this important area. Its findings on the effectiveness of dialect-aware models and the establishment of a robust benchmark will significantly impact future research. The public availability of the models and benchmark further enhances its significance for the research community.
Visual Insights#
๐ผ This figure provides a detailed breakdown of the ArabicMTEB benchmark, illustrating the eight distinct task categories it encompasses: Retrieval, Crosslingual Retrieval, Bitext Mining, Re-ranking, Semantic Textual Similarity, Pair Classification, Classification, and Clustering. Each category is further categorized to indicate its relevance to the broader field of Arabic natural language processing.
read the caption
Figure 1: Details of ArabicMTEB
Benchmark | Language | Tasks | Datasets | #Tasks | CRTR | Arabic Culture/Domains |
---|---|---|---|---|---|---|
MTEB Muennighoff et al. (2022) | English | RTR, STS, PairCLF, CLF, RRK, CLR, SUM | 56 | 7 | ร | ร |
C-MTEB Xiao et al. (2023) | Chinese | RTR, STS, PairCLF, CLF, RRK, CLR | 35 | 6 | ร | ร |
De-MTEB Sturua et al. (2024) | German | RTR, STS, PairCLF, CLF, RRK, CLR | 17 | 6 | ร | ร |
F-MTEB Ciancone et al. (2024) | French | RTR, STS, PairCLF, CLF, RRK, CLR, BTM | 17 | 7 | ร | ร |
Es-MTEB Mohr et al. (2024) | Spanish | RTR, STS, PairCLF, CLF, RRK, CLR | 17 | 6 | ร | ร |
Polish-MTEB Poลwiata et al. (2024) | Polish | RTR, STS, PairCLF, CLF, CLR | 26 | 5 | ร | ร |
Ru-MTEB Poลwiata et al. (2024) | Russian | RTR, STS, PairCLF, CLF, RRK, CLR | 23 | 6 | ร | ร |
Scand. MTEB Enevoldsen et al. (2024) | Danish | RTR, CLF, BTM, CLR | 26 | 4 | ร | ร |
Norwegian | ร | ร | ||||
Swedish | ร | ร | ||||
ArabicMTEB (Ours) | Arabic | RTR, STS, PairCLF, CLF, RRK, CLR, BTM, CRTR | 94 | 8 | โ | โ |
๐ผ This table compares various text embedding benchmarks from the literature. It shows the tasks covered by each benchmark (Retrieval, Semantic Textual Similarity, Pair Classification, Classification, Clustering, Re-ranking, and Bitext Mining), the number of datasets used, and whether each benchmark includes cross-lingual and/or culturally specific tasks. This allows for a comparison of the scope and focus of different benchmarks, highlighting the unique contributions of ArabicMTEB.
read the caption
Table 1: Comparison of Various Text Embedding benchmarks proposed in the literature across the different covered task clusters. RTR: Retrieval, STS: Semantic Textual Similarity, PairCLF: Pair Classification, CLF: Classification, CLR: Clustering, RRK: Reranking, BTM: BitextMining, CRTR: Crosslingual Retrieval.
In-depth insights#
Arabic Embeddings#
The research paper explores the development of Swan, a family of Arabic embedding models designed to address limitations of existing multilingual models in capturing Arabic linguistic and cultural nuances. Swan offers two variants: a smaller model based on ARBERTv2 and a larger one built on ArMistral, a pretrained Arabic large language model. ArabicMTEB, a comprehensive benchmark suite, is introduced to evaluate these models across diverse tasks and datasets, showcasing Swan-Large’s state-of-the-art performance. The study highlights Swan’s dialectal and cultural awareness, demonstrating its superior performance in various Arabic domains while offering monetary efficiency. The focus on Arabic-specific models and benchmarks represents a significant advancement in Arabic NLP, providing valuable resources for future research and applications.
Swan Model#
The Swan model, introduced in this research paper, is a family of Arabic-centric embedding models designed to address both small-scale and large-scale applications. It encompasses two main variants: Swan-Small, based on ARBERTv2, and Swan-Large, built on the ArMistral pretrained large language model. A key strength of Swan is its dialect-aware and culturally aware nature, excelling in various Arabic domains while maintaining efficiency. The models’ performance is rigorously evaluated using a comprehensive benchmark, ArabicMTEB, demonstrating state-of-the-art results on several Arabic NLP tasks. The availability of both a small and large variant ensures applicability across diverse computational resource constraints, making Swan a significant contribution to Arabic NLP.
ArabicMTEB#
ArabicMTEB is a comprehensive benchmark designed to evaluate Arabic text embedding models. Unlike existing benchmarks that often lack sufficient Arabic coverage or neglect dialectal and cultural nuances, ArabicMTEB offers a holistic assessment using 94 datasets across eight diverse tasks. These tasks include Arabic text retrieval, bitext mining, cross-lingual retrieval, re-ranking, semantic textual similarity, classification, pair classification, and clustering. The benchmark’s strength lies in its ability to evaluate models across various linguistic aspects, including MSA and multiple dialects, and cultural domains, providing a more realistic and applicable assessment of embedding model capabilities for real-world Arabic NLP applications. Its inclusion of domain-specific and culturally aware datasets further enhances its value for researchers seeking to develop robust and nuanced Arabic language technologies.
Benchmarking#
The benchmarking section of the research paper introduces ArabicMTEB, a novel and comprehensive benchmark designed to evaluate Arabic text embedding models. Unlike existing benchmarks that lack sufficient Arabic language coverage or neglect dialectal and cultural nuances, ArabicMTEB assesses performance across eight diverse tasks and 94 datasets, encompassing various Arabic varieties and domains. This robust evaluation framework offers a more realistic and applicable assessment of embedding models’ capabilities in real-world scenarios. The key tasks within ArabicMTEB include retrieval, classification, semantic similarity, and cross-lingual capabilities, reflecting a holistic approach to model evaluation. The benchmark also considers dialectal and cultural aspects of the Arabic language, showcasing its commitment to thorough and nuanced evaluation in Arabic NLP. By addressing the limitations of existing benchmarks, ArabicMTEB provides a valuable resource for future research and development in Arabic language technologies.
Future Work#
The provided text does not contain a section or heading specifically titled ‘Future Work’. Therefore, it’s impossible to generate a summary for such a section. To provide a meaningful summary, please provide the text from the ‘Future Work’ section of your research paper.
More visual insights#
More on figures
๐ผ This figure illustrates the methodology used to generate synthetic data for training the Arabic embedding models. Specifically, it demonstrates how positive and hard negative examples are created using a large language model (LLM), in this case Command-R+. The process involves generating tasks related to real-world usage and using the LLM to generate a positive example (a relevant document) and a hard negative example (a document that is closely related to the query but less useful).
read the caption
(a) Positive and hard negative generation
๐ผ This figure illustrates the process of generating synthetic data for Arabic text embedding models. It starts with real-world text, using a model to create tasks. Then, it uses the model to generate synthetic data, which is further divided into Modern Standard Arabic (MSA) and dialectal Arabic data.
read the caption
Figure 2: Methodology to generate our synthetic data.
More on tables
Family | Language | Type | Dataset | Level | Size |
---|---|---|---|---|---|
Monolingual | Arabic | Human | ORCA-MSA | Sentence | 378K |
ORCA-DIA | Sentence | 122K | |||
MMARCO-ar | Sentence | 8.1M | |||
Synthetic | Synth-MSA | Paragraph | 100K | ||
Synth-DIA | Paragraph | 15K | |||
Synth-DOM | Paragraph | 20K | |||
Crosslingual | Arabic to 15 Langs | Human | MMARCO | Sentence | 3M |
Arabic to 6 Langs | Human | XOR-TyDi | Sentence | 20.5K | |
Multilingual | 11 Langs | Human | Mr-Tydi | Sentence | 49K |
16 Langs | Human | Miracl | Sentence | 343K | |
Total | 12.5M |
๐ผ Table 2 details the diverse datasets used to train the Swan Arabic embedding models. The table shows a breakdown of the data sources, including human-generated datasets (ORCA and mMARCO), and synthetic datasets. The synthetic data is further categorized into three types: (1) Modern Standard Arabic (MSA), (2) Dialectal Arabic (Egyptian and Moroccan dialects), and (3) Domain-specific datasets (Medical, Financial, Legal, and News domains). This table provides a comprehensive overview of the training data’s composition and the different linguistic variations covered in the training process.
read the caption
Table 2: The diverse datasets employed for training our Arabic embedding models. In the synthetic dataset, we have three datasets: the MSA dataset, the Dialectal dataset (Egyptian and Moroccan), and domain-based focusing on Medical, Financial, Legal and News domains.
Task | Datasets | Languages | Dialects | Metric |
---|---|---|---|---|
RTR | 36 | 1 | 4 | nDCG@10 |
CRTR | 12 | 7 | 0 | nDCG@10 |
CLF | 18 | 1 | 6 | AP |
BTM | 11 | 5 | 8 | F1 |
RRK | 5 | 2 | 0 | MAP |
STS | 5 | 1 | 3 | Spearman Corr |
CLR | 4 | 1 | 0 | v-measure |
PairCLF | 3 | 1 | 0 | AP |
Total | 94 | 9 | 11 |
๐ผ This table provides a detailed breakdown of the tasks included in the ArabicMTEB benchmark. It shows the number of datasets, languages, and dialects used for each task, along with the specific evaluation metric employed. The tasks cover a range of natural language processing capabilities, including retrieval, semantic textual similarity, classification, reranking, and more, offering a comprehensive assessment of Arabic text embedding models’ performance. The ‘Total’ column indicates the unique number of languages represented across all tasks.
read the caption
Table 3: Overview of our Tasks in ArabicMTEB. โTotal represents the unique languages.
Model | Size | Dim. | RTR | STS | PairCLF | CLF | RRK | CLR | BTM | Avg. |
---|---|---|---|---|---|---|---|---|---|---|
arabertv02-base | 160M | 768 | 8.62 | 39.77 | 66.30 | 55.77 | 60.03 | 41.74 | 0.70 | 38.99 |
CamelBERT | 163M | 768 | 9.21 | 47.69 | 67.43 | 55.66 | 60.20 | 39.89 | 1.85 | 40.28 |
ARBERTv2 | 164M | 768 | 15.12 | 47.88 | 68.87 | 56.85 | 62.21 | 39.25 | 1.99 | 41.74 |
ATM-V2 | 135M | 768 | 37.45 | 55.90 | 70.12 | 46.42 | 61.45 | 32.35 | 12.98 | 45.24 |
text2vec | 118M | 384 | 27.69 | 59.37 | 71.41 | 47.94 | 57.76 | 37.26 | 38.32 | 48.54 |
LaBSE | 471M | 768 | 34.98 | 54.15 | 70.60 | 49.57 | 62.17 | 41.42 | 33.28 | 49.45 |
Me5-small | 118M | 384 | 55.14 | 56.73 | 73.97 | 50.85 | 67.92 | 42.37 | 38.47 | 55.06 |
Me5-base | 278M | 768 | 56.91 | 57.99 | 74.30 | 52.30 | 69.07 | 42.56 | 33.90 | 55.29 |
Swan-Small | 164M | 768 | 58.42 | 59.34 | 74.93 | 57.34 | 68.43 | 40.43 | 42.45 | 57.33 |
e5-mistral-7b | 7110M | 4096 | 56.34 | 57.02 | 70.24 | 53.21 | 66.24 | 39.44 | 70.5 | 59.00 |
Me5-large | 560M | 1024 | 64.01 | 59.45 | 75.06 | 53.43 | 70.79 | 42.49 | 66.33 | 61.65 |
Swan-Large | 7230M | 4096 | 65.63 | 59.10 | 75.62 | 54.89 | 69.42 | 41.24 | 71.24 | 62.45 |
๐ผ This table presents a comprehensive evaluation of various Arabic text embedding models on the ArabicMTEB benchmark. It compares the performance of Swan-Small and Swan-Large to other state-of-the-art multilingual and Arabic-specific models across eight different tasks, including retrieval, semantic textual similarity, classification, and clustering. The results are shown as average scores across 94 datasets, providing a detailed comparison of model performance across different aspects of Arabic text embedding.
read the caption
Table 4: Overall ArabicMTEB results
Model | RTR | STS | CLF | BTM | Avg. |
---|---|---|---|---|---|
arabertv02-base | 8.67 | 41.64 | 47.97 | 0.99 | 24.82 |
MARBERT | 5.45 | 50.06 | 53.46 | 2.34 | 27.83 |
ARBERTv2 | 7.52 | 49.36 | 54.31 | 2.51 | 28.43 |
CamelBERT | 6.92 | 59.48 | 50.69 | 2.65 | 29.93 |
AlcLaM | 8.56 | 50.90 | 54.74 | 7.54 | 30.44 |
ATM-V2 | 36.23 | 74.13 | 34.39 | 11.67 | 39.10 |
Me5-base | 61.60 | 74.84 | 34.87 | 3.30 | 43.65 |
Me5-small | 57.61 | 76.35 | 34.78 | 12.35 | 45.27 |
Me5-large | 66.88 | 77.02 | 35.47 | 51.08 | 57.61 |
e5-mistral-7b | 72.35 | 77.37 | 35.91 | 57.62 | 60.81 |
Swan-Small | 63.16 | 76.57 | 54.52 | 59.38 | 63.41 |
Swan-Large | 77.03 | 79.22 | 53.46 | 72.10 | 70.45 |
๐ผ This table presents a detailed comparison of various Arabic text embedding models’ performance on the Dialectal ArabicMTEB benchmark. The benchmark specifically focuses on evaluating how well models handle the diverse variations within the Arabic language’s dialects. The table displays the results for several models across a range of tasks, including retrieval, semantic textual similarity, classification, and others, enabling a comprehensive assessment of their capabilities in understanding dialectal Arabic text.
read the caption
Table 5: Dialectal ArabicMTEB results.
Model | News | Legal | Medical | Finance | Wikipedia | Avg | Cost |
---|---|---|---|---|---|---|---|
Swan-Large | 90.42 | 89.96 | 81.64 | 57.34 | 93.10 | 82.49 | 0.75$ |
Openai-3-large | 88.1 | 89.68 | 80.24 | 61.46 | 91.52 | 82.20 | 9.88$ |
Cohere-v3.0 | 85.23 | 86.52 | 63.27 | 42.80 | 90.96 | 73.76 | 7.54$ |
Swan-Small | 81.55 | 78.86 | 70.97 | 42.48 | 80.46 | 70.86 | 0.44$ |
Openai-3-small | 71.42 | 85.23 | 71.50 | 32.90 | 82.20 | 68.65 | 3.75$ |
Cohere-light-v3.0 | 70.32 | 86.83 | 67.68 | 22.68 | 90.34 | 67.57 | 2.55$ |
Openai-ada-002 | 65.34 | 81.83 | 71.76 | 39.62 | 76.79 | 67.07 | 1.66$ |
๐ผ This table presents the performance of different models on the Domain-Specific ArabicMTEB benchmark. The benchmark focuses on evaluating Arabic text embeddings across various domains including News, Legal, Medical, Finance, and General knowledge. The table shows the scores achieved by each model on each domain. This allows comparison of the models’ performance across various specialized domains within the Arabic language.
read the caption
Table 6: Domain-Specific ArabicMTEB results.
Model | MSA-Culture | Egyptian-DIA | Morocco-DIA | Avg. |
---|---|---|---|---|
Swan-Large | 82.19 | 83.55 | 65.35 | 77.03 |
Cohere-v3.0 | 81.86 | 82.90 | 65.23 | 76.66 |
OpenAI-3-large | 81.49 | 78.45 | 64.90 | 74.95 |
Cohere-light-v3.0 | 80.75 | 64.82 | 56.84 | 67.47 |
Me5-large | 78.65 | 61.34 | 60.66 | 66.88 |
OpenAI-3-Small | 74.55 | 65.89 | 54.13 | 64.86 |
Swan-Small | 75.56 | 60.35 | 53.56 | 63.16 |
Me5-base | 74.56 | 56.34 | 53.91 | 61.60 |
Me5-small | 73.81 | 53.56 | 45.45 | 57.61 |
ATM-V2 | 63.78 | 23.45 | 21.45 | 36.23 |
ARBERTv2 | 9.34 | 8.55 | 4.67 | 7.52 |
MARBERT | 2.73 | 0.44 | 0.19 | 1.12 |
๐ผ This table presents a detailed breakdown of the performance of various models on the Cultural ArabicMTEB benchmark. It shows the scores achieved by each model across different cultural datasets, specifically focusing on unique cultural aspects from various Arab countries, revealing the models’ ability to capture culturally sensitive nuances in the Arabic language.
read the caption
Table 7: Cultural ArabicMTEB results.
Model | ArRTR | DOM-RTR | DIA-RTR | STS | PairCLF | CLF | RRK | CLK | BTM | Avg. |
---|---|---|---|---|---|---|---|---|---|---|
Swan-Small | 15.12 | 8.46 | 7.52 | 37.88 | 62.87 | 56.85 | 62.21 | 39.25 | 1.99 | 32.46 |
+ Arabic | 28.39 | 39.34 | 15.23 | 41.49 | 70.25 | 51.89 | 68.57 | 39.12 | 18.74 | 41.45 |
+ Synthetic-MSA | 31.07 | 40.45 | 53.45 | 55.78 | 74.23 | 54.27 | 68.88 | 39.43 | 18.19 | 48.42 |
+ Synthetic-DOM | 32.01 | 49.02 | 49.34 | 52.90 | 75.45 | 54.43 | 67.45 | 40.56 | 17.35 | 48.72 |
+ Synthetic-DIA | 31.20 | 38.66 | 59.43 | 51.23 | 72.86 | 57.56 | 66.67 | 37.34 | 19.90 | 48.32 |
Swan-Large | 44.46 | 64.52 | 66.23 | 48.63 | 72.34 | 50.43 | 69.39 | 38.28 | 44.20 | 55.39 |
+ Arabic | 54.53 | 66.43 | 70.34 | 52.93 | 75.24 | 52.54 | 70.49 | 40.21 | 48.35 | 59.01 |
+ Synthetic-MSA | 56.34 | 67.90 | 72.89 | 57.89 | 76.90 | 50.21 | 70.92 | 41.76 | 62.34 | 61.91 |
+ Synthetic-DOM | 58.42 | 76.54 | 71.65 | 55.92 | 75.19 | 50.19 | 70.21 | 39.33 | 51.23 | 60.96 |
+ Synthetic-DIA | 57.09 | 65.06 | 77.03 | 56.90 | 76.42 | 54.89 | 69.32 | 39.41 | 65.56 | 62.41 |
๐ผ This table presents the results of an experiment designed to analyze how the use of synthetic data impacts the performance of the Swan model. The model is evaluated across several key retrieval tasks: Arabic retrieval (ArRTR), domain-specific retrieval (DOM-RTR), and dialectal retrieval (DIA-RTR). The table allows for a comparison of the Swan model’s performance using different combinations of real and synthetic datasets, thereby quantifying the influence of the synthetic data on the model’s performance across various dimensions of Arabic language.
read the caption
Table 8: The impact of Synthetic Data on Swan performance. ArRTR: Arabic retrieval, DOM-RTR: Domain-specific retrieval, and DIA-RTR: Dialectal Retrieval
Model | ARC | Hellaswag | Exams | MMLU | Truthfulqa | ACVA | AlGhafa | Average |
---|---|---|---|---|---|---|---|---|
ArMistral-7B-Chat | 43.20 | 55.53 | 45.54 | 43.50 | 52.44 | 77.06 | 35.57 | 50.41 |
Jais-13b-chat | 41.10 | 57.70 | 46.74 | 42.80 | 47.48 | 72.56 | 34.42 | 48.97 |
AceGPT-13B-chat | 43.80 | 52.70 | 42.09 | 41.10 | 49.96 | 78.42 | 31.95 | 48.57 |
AceGPT-13B-base | 39.90 | 51.30 | 39.48 | 40.50 | 46.73 | 75.29 | 30.37 | 46.22 |
AraLLama-7B-Chat | 39.45 | 50.23 | 38.24 | 41.03 | 50.44 | 70.45 | 32.54 | 46.05 |
ArMistral-7B-Base | 41.50 | 52.50 | 38.92 | 37.50 | 51.27 | 69.64 | 30.24 | 45.94 |
Jais-13b-base | 39.60 | 50.30 | 39.29 | 36.90 | 50.59 | 68.09 | 30.07 | 44.98 |
AceGPT-7B-chat | 38.50 | 49.80 | 37.62 | 34.30 | 49.85 | 71.81 | 31.83 | 44.81 |
AraLLama-7B-Base | 38.40 | 50.12 | 38.43 | 40.23 | 45.32 | 69.42 | 31.52 | 44.78 |
AceGPT-7B-base | 37.50 | 48.90 | 35.75 | 29.70 | 43.04 | 68.96 | 33.11 | 42.42 |
๐ผ This table compares the performance of ArMistral, a new Arabic language model, against other state-of-the-art Arabic LLMs across various benchmarks. The benchmarks assess capabilities in different areas including commonsense reasoning (ARC), natural language inference (Hellaswag), multiple-choice questions (Exams), general knowledge (MMLU), truthfulness (TruthfulQA), commonsense reasoning (ACVA), and Arabic-specific knowledge (AlGhafa). The average score across all benchmarks provides a comprehensive comparison of the models’ overall performance.
read the caption
Table 9: Comparison of ArMistral with other Arabic LLMs
Task | Dataset | Type | Language | Citation | Size |
---|---|---|---|---|---|
BitextMining | Darija | S2S | Moroccan Arabic Dialect to English | Nagoudi et al. (2023b) | 2000 |
BitextMining | Narabizi | S2S | Arabizi to French | Nagoudi et al. (2023b) | 144 |
BitextMining | Mt_en2ar | S2S | English to MSA | Nagoudi et al. (2023b) | 4000 |
BitextMining | Mt_fr2ar | S2S | French to MSA | Nagoudi et al. (2023b) | 4000 |
BitextMining | Mt_es2ar | S2S | Spanish to MSA | Nagoudi et al. (2023b) | 4000 |
BitextMining | Mt_ru2ar | S2S | Russian to MSA | Nagoudi et al. (2023b) | 4000 |
BitextMining | Cs_dz_fr | S2S | Algerian Arabic Dialect to French | Nagoudi et al. (2023b) | 200 |
BitextMining | Cs_eg_en | S2S | Egyptian Arabic Dialect to English | Nagoudi et al. (2023b) | 200 |
BitextMining | Cs_jo_en | S2S | Jordanian Arabic to English | Nagoudi et al. (2023b) | 200 |
BitextMining | Cs_ma_fr | S2S | Moroccan Arabic to French | Nagoudi et al. (2023b) | 200 |
BitextMining | Cs_ps_en | S2S | Palestinian Arabic to English | Nagoudi et al. (2023b) | 200 |
BitextMining | Cs_ye_en | S2S | Yemeni Arabic to English | Nagoudi et al. (2023b) | 200 |
Classification | MassiveIntent | S2S | Multilingual (Arabic subset) | FitzGerald et al. (2022) | 100 |
Classification | MassiveScenario | S2S | Multilingual (Arabic subset) | FitzGerald et al. (2022) | 100 |
Classification | OrcaSentiment | S2S | Arabic | Elmadany et al. (2022) | 5000 |
Classification | OrcaDialect_region | S2S | Arabic | Elmadany et al. (2022) | 5000 |
Classification | OrcaDialect_binary | S2S | Arabic | Elmadany et al. (2022) | 5000 |
Classification | OrcaDialect_country | S2S | Arabic | Elmadany et al. (2022) | 5000 |
Classification | OrcaAns_claim | S2S | Arabic | Elmadany et al. (2022) | 5000 |
Classification | OrcaMachine_generation | S2S | Arabic | Elmadany et al. (2022) | 5000 |
Classification | OrcaAge | S2S | Arabic | Elmadany et al. (2022) | 5000 |
Classification | OrcaGender | S2S | Arabic | Elmadany et al. (2022) | 5000 |
Classification | OrcaAdult | S2S | Arabic | Elmadany et al. (2022) | 5000 |
Classification | OrcaDangerous | S2S | Arabic | Elmadany et al. (2022) | 5000 |
Classification | OrcaEmotion | S2S | Arabic | Elmadany et al. (2022) | 5000 |
Classification | OrcaHate_speech | S2S | Arabic | Elmadany et al. (2022) | 5000 |
Classification | OrcaOffensive | S2S | Arabic | Elmadany et al. (2022) | 5000 |
Classification | OrcaIrony | S2S | Arabic | Elmadany et al. (2022) | 5000 |
Classification | OrcaSarcasm | S2S | Arabic | Elmadany et al. (2022) | 5000 |
Classification | OrcaAbusive | S2S | Arabic | Elmadany et al. (2022) | 5000 |
Clustering | Arabic_news | P2P | Arabic | Our Paper | 2500 |
Clustering | Arabic_topic | S2S | Arabic | Our Paper | 30 |
Clustering | Arabic_baly_stance | P2P | Arabic | Elmadany et al. (2022) | 1000 |
Clustering | Arabic_baly_stance | S2S | Arabic | Elmadany et al. (2022) | 100 |
PairClassification | Arabic_xnli | S2S | Arabic | Our Paper | 538 |
PairClassification | Arabic_sts | S2S | Arabic | Our Paper | 1256 |
PairClassification | Arabic_mq2q | S2S | Arabic | Our Paper | 244 |
Reranking | Miracl_ar | S2P | Multilingual (Arabic subset) | Zhang et al. (2023) | 750 |
Reranking | Mmarco_arabic | S2P | Arabic | Our Paper | 3000 |
Reranking | MedicalQA_arabic | S2P | Arabic | Our Paper | 4350 |
Reranking | Mmarco_en2ar | S2P | English to MSA | Our Paper | 500 |
Reranking | Mmarco_ar2en | S2P | MSA to English | Our Paper | 500 |
Retrieval | MultiLongDoc | S2P | Multilingual (Arabic subset) | MDQA | |
Retrieval | XPQA | S2S | Multilingual (Arabic subset) | XPQA | |
Retrieval | Mintaka | S2S | Multilingual (Arabic subset) | Mintaka | |
Retrieval | Lareqa | S2P | Arabic | Nagoudi et al. (2023b) | 220 |
Retrieval | Dawqs | S2S | Arabic | Nagoudi et al. (2023b) | 318 |
Retrieval | Exams | S2S | Arabic | Nagoudi et al. (2023b) | 2600 |
Retrieval | Mkqa | S2S | Arabic | Nagoudi et al. (2023b) | 340 |
Retrieval | Mlqa | S2S | Arabic | Nagoudi et al. (2023b) | 517 |
Retrieval | Arcd | S2S | Arabic | Nagoudi et al. (2023b) | 693 |
Retrieval | Tydiqa | S2S | Arabic | Nagoudi et al. (2023b) | 5700 |
Retrieval | Xsquad | S2S | Arabic | Nagoudi et al. (2023b) | 5700 |
Retrieval | Crosslingual_ar2de | S2P | MSA to German | Our Paper | 1831 |
Retrieval | Crosslingual_ar2en | S2P | MSA to English | Our Paper | 1831 |
Retrieval | Crosslingual_ar2es | S2P | MSA to Spanish | Our Paper | 1831 |
Retrieval | Crosslingual_ar2hi | S2P | MSA to Hindi | Our Paper | 1831 |
Retrieval | Crosslingual_ar2vi | S2P | MSA to Vietnamese | Our Paper | 1831 |
Retrieval | Crosslingual_ar2zh | S2P | MSA to Chinese | Our Paper | 1831 |
Retrieval | Crosslingual_de2ar | S2P | German to MSA | Our Paper | 1831 |
Retrieval | Crosslingual_en2ar | S2P | English to MSA | Our Paper | 1831 |
Retrieval | Crosslingual_es2ar | S2P | Spanish to MSA | Our Paper | 1831 |
Retrieval | Crosslingual_hi2ar | S2P | Hindi to MSA | Our Paper | 1831 |
Retrieval | Crosslingual_vi2ar | S2P | Vietnamese to MSA | Our Paper | 1831 |
Retrieval | Crosslingual_zh2ar | S2P | Chinese to MSA | Our Paper | 1912 |
Retrieval | MoroccoCultural | S2P | Arabic | Our Paper | 100 |
Retrieval | SyriaCultural | S2P | Arabic | Our Paper | 100 |
Retrieval | LibyaCultural | S2P | Arabic | Our Paper | 100 |
Retrieval | LebanonCultural | S2P | Arabic | Our Paper | 100 |
Retrieval | QatarCultural | S2P | Arabic | Our Paper | 100 |
Retrieval | SudanCultural | S2P | Arabic | Our Paper | 100 |
Retrieval | AlgeriaCultural | S2P | Arabic | Our Paper | 100 |
Retrieval | MauritaniaCultural | S2P | Arabic | Our Paper | 100 |
Retrieval | TunisiaCultural | S2P | Arabic | Our Paper | 100 |
Retrieval | IraqCultural | S2P | Arabic | Our Paper | 100 |
Retrieval | EgyptCultural | S2P | Arabic | Our Paper | 100 |
Retrieval | SomaliaCultural | S2P | Arabic | Our Paper | 100 |
Retrieval | UAE_Cultural | S2P | Arabic | Our Paper | 100 |
Retrieval | OmanCultural | S2P | Arabic | Our Paper | 100 |
Retrieval | KuwaitCultural | S2P | Arabic | Our Paper | 100 |
Retrieval | BahrainCultural | S2P | Arabic | Our Paper | 100 |
Retrieval | Saudi_ArabiaCultural | S2P | Arabic | Our Paper | 100 |
Retrieval | JordanCultural | S2P | Arabic | Our Paper | 100 |
Retrieval | PalestineCultural | S2P | Arabic | Our Paper | 100 |
Retrieval | YemenCultural | S2P | Arabic | Our Paper | 100 |
Retrieval | MoroccoDIA | S2P | Moroccan Arabic Dialect | Our Paper | 100 |
Retrieval | EgyptDIA | S2P | Egyptian Arabic Dialect | Our Paper | 100 |
Retrieval | NewsDomainSpecific | S2P | Arabic | Our Paper | 1000 |
Retrieval | LegalDomainSpecific | S2P | Arabic | Our Paper | 1000 |
Retrieval | MedicalDomainSpecific | S2P | Arabic | Our Paper | 1000 |
Retrieval | FinanceDomainSpecific | S2P | Arabic | Our Paper | 1000 |
Retrieval | WikipediaDomainSpecific | S2P | Arabic | Our Paper | 1000 |
STS | STS17 | S2S | Arabic | Cer et al. (2017) | 8060 |
STS | STS22 | P2P | Arabic | Semenov et al. (2023) | 500 |
STS | Arabic_sts | S2S | Arabic | Our Paper | 750 |
STS | Arabic_stsb_multi_dialect | S2S | Arabic Dialectal | Our Paper | 1500 |
STS | Arabic_sts | P2P | Arabic | Our Paper | 500 |
๐ผ This table provides a comprehensive overview of the datasets used in the ArabicMTEB benchmark. It lists each dataset’s name, type (Sentence-to-Sentence, Sentence-to-Paragraph, Paragraph-to-Paragraph), language(s) included, citation, and size. The table is categorized by task (Bitext Mining, Classification, Clustering, Pair Classification, Reranking, Retrieval, Semantic Textual Similarity), providing a clear view of the diverse data sources used to evaluate Arabic text embedding models.
read the caption
Table 10: Benchmark Datasets Overview. Abbreviations: S2S = Sentence to Sentence, S2P = Sentence to Paragraph, P2P = Paragraph to Paragraph.
Task | Instructions |
---|---|
Reranking | Given an Arabic search query, retrieve web passages that answer the question in {Lang}. Query:{query}. |
BitextMining | Retrieve parallel sentences in {Lang}. |
Retrieval | Given an Arabic search query, retrieve web passages that answer the question. Query:{query}. |
Crosslingual Retrieval | Given an Arabic search query, retrieve web passages that answer the question in {Lang}. Query:{query}. |
STS | Retrieve semantically similar text. Text: {text}. |
Pair Classification | Retrieve texts that are semantically similar to the given text. Text: {text}. |
Clustering | Identify the topic or theme of the given news article. Article:{article}. |
Classification | Classify the text into the given categories {options}. |
๐ผ This table lists the instructions used for evaluating different tasks in the ArabicMTEB benchmark. Each task (such as reranking, bitext mining, retrieval, etc.) has a corresponding instruction showing how the model should perform the task, including the format of the query and any specific guidelines.
read the caption
Table 11: Prompts used for evaluation.
Model | Dim. | Retrieval | STS | PairCLF | CLF | Re-rank | Cluster | BTM | Avg |
---|---|---|---|---|---|---|---|---|---|
Number of datasets | 23 | 5 | 3 | 18 | 5 | 4 | 12 | 70 | |
Swan-Large | 4096 | 65.63 | 59.10 | 75.62 | 52.55 | 69.42 | 41.24 | 71.24 | 62.11 |
multilingual-e5-large | 1024 | 64.01 | 59.45 | 75.06 | 53.43 | 70.79 | 42.49 | 66.33 | 61.65 |
e5-mistral-7b-instruct | 4096 | 56.34 | 57.02 | 70.24 | 53.21 | 66.24 | 39.44 | 70.50 | 59.00 |
Swan-Base | 768 | 58.42 | 58.44 | 74.93 | 57.34 | 68.43 | 40.43 | 42.45 | 57.21 |
multilingual-e5-base | 768 | 56.91 | 57.99 | 74.30 | 52.30 | 69.07 | 42.56 | 33.90 | 55.29 |
multilingual-e5-small | 384 | 55.14 | 56.73 | 73.97 | 50.85 | 67.92 | 42.37 | 38.47 | 55.06 |
LaBSE | 768 | 34.98 | 54.15 | 70.60 | 49.57 | 62.17 | 41.42 | 33.28 | 49.45 |
text2vec-base | 384 | 27.69 | 59.37 | 71.41 | 47.94 | 57.76 | 37.26 | 38.32 | 48.54 |
ARBERTv2 | 768 | 15.12 | 37.88 | 62.87 | 56.85 | 62.21 | 39.25 | 1.99 | 39.45 |
CamelBERT-msa | 768 | 9.21 | 47.69 | 67.43 | 55.77 | 60.20 | 39.89 | 1.85 | 40.29 |
arabertv02-large | 1024 | 7.34 | 34.26 | 63.63 | 54.32 | 56.71 | 37.26 | 10.97 | 37.78 |
arabertv02-base | 768 | 8.62 | 39.77 | 66.30 | 55.77 | 60.03 | 41.74 | 0.70 | 38.99 |
CamelBERT-mix | 768 | 7.19 | 46.47 | 67.23 | 56.68 | 57.50 | 38.72 | 0.41 | 39.17 |
MARBERTv2 | 768 | 5.88 | 45.21 | 70.89 | 54.89 | 58.64 | 40.81 | 0.45 | 39.54 |
ARBERT | 768 | 8.07 | 29.89 | 61.86 | 56.92 | 61.09 | 37.10 | 2.28 | 36.74 |
CamelBERT-da | 768 | 4.07 | 41.05 | 65.82 | 53.75 | 54.44 | 37.63 | 0.31 | 36.72 |
MARBERT | 768 | 2.22 | 40.62 | 66.46 | 54.35 | 53.09 | 36.33 | 0.40 | 36.21 |
CamelBERT-ca | 768 | 2.74 | 36.49 | 62.26 | 46.26 | 51.34 | 35.77 | 0.09 | 33.56 |
๐ผ This table presents a comprehensive evaluation of various Arabic text embedding models on the ArabicMTEB benchmark. It compares the performance of Swan-Large and Swan-Small against several state-of-the-art multilingual and Arabic-specific models across eight diverse tasks, including retrieval, semantic textual similarity, pair classification, classification, reranking, clustering, and bitext mining. The results are shown in terms of average scores across multiple datasets for each task, providing a detailed comparison of the models’ strengths and weaknesses.
read the caption
Table 12: ArMTEB Results.
Model (HN) | 1 | 3 | 7 | 15 | 31 |
---|---|---|---|---|---|
Swan-Small | 48.84 | 52.19 | 54.13 | 56.25 | 51.93 |
Swan-Large | 59.48 | 59.35 | 60.42 | 59.44 | 59.83 |
๐ผ This table presents the results of an experiment evaluating the impact of the number of hard negative samples used during the training of two embedding models: Swan-Small and Swan-Large. It shows the average performance scores obtained by varying the number of hard negatives (HN) in the training data (1, 3, 7, 15, 31) and provides insight into how this hyperparameter affects model performance.
read the caption
Table 13: Impact of number of Hard Negatives (HN).
Model | Swan-Large | Me5-large | Cohere-light-v3.0 | Swan-Base | OpenAI-3-large | Cohere-v3.0 | Me5-small | Me5-base | ATM-V2 | ARBERTv2 | MARBERT |
---|---|---|---|---|---|---|---|---|---|---|---|
Algeria | 89.34 | 93.34 | 89.44 | 90.45 | 86.95 | 88.99 | 91.23 | 90.66 | 84.99 | 18.27 | 1.50 |
Bahrain | 93.71 | 93.77 | 93.52 | 86.48 | 91.98 | 92.40 | 93.08 | 89.04 | 90.49 | 27.48 | 5.74 |
Egypt | 98.34 | 94.58 | 91.37 | 95.66 | 91.45 | 87.81 | 93.02 | 91.65 | 88.45 | 11.54 | 1.63 |
Iraq | 92.45 | 90.90 | 86.98 | 88.34 | 92.43 | 87.83 | 89.02 | 90.78 | 81.22 | 17.34 | 1.92 |
Jordan | 92.34 | 92.79 | 90.07 | 89.70 | 94.56 | 91.18 | 93.67 | 92.25 | 87.95 | 27.46 | 4.50 |
Kuwait | 93.45 | 96.34 | 96.10 | 90.44 | 88.53 | 92.51 | 96.17 | 94.94 | 89.97 | 36.67 | 4.92 |
Lebanon | 95.66 | 93.05 | 92.38 | 90.45 | 90.23 | 91.04 | 91.92 | 92.85 | 87.14 | 22.55 | 1.82 |
Libya | 89.56 | 88.43 | 87.27 | 85.45 | 89.66 | 85.75 | 87.21 | 85.32 | 79.95 | 28.88 | 2.46 |
Mauritania | 92.44 | 92.92 | 92.61 | 89.45 | 90.31 | 92.05 | 20.99 | 3.32 | 0.63 | 0.50 | 0.00 |
Morocco | 90.34 | 85.49 | 83.19 | 86.34 | 83.56 | 85.47 | 81.73 | 86.59 | 4.75 | 0.32 | 0.00 |
Oman | 94.45 | 94.26 | 92.37 | 91.98 | 92.45 | 92.61 | 93.00 | 93.04 | 84.21 | 11.24 | 3.43 |
Palestine | 90.45 | 90.67 | 87.50 | 91.18 | 87.45 | 83.33 | 85.22 | 86.49 | 77.83 | 27.25 | 3.63 |
Qatar | 98.79 | 93.44 | 91.80 | 92.35 | 95.66 | 89.98 | 91.20 | 90.49 | 85.50 | 29.15 | 7.00 |
Saudi_Arabia | 95.34 | 93.49 | 92.98 | 91.47 | 90.45 | 92.12 | 92.72 | 91.47 | 86.48 | 25.06 | 2.50 |
Somalia | 90.23 | 94.78 | 93.67 | 88.34 | 89.55 | 92.30 | 21.25 | 2.50 | 20.81 | 2.62 | 0.00 |
Sudan | 92.36 | 91.99 | 86.90 | 90.89 | 91.45 | 90.72 | 89.49 | 87.60 | 82.47 | 24.51 | 2.50 |
Syria | 91.46 | 91.83 | 90.56 | 90.45 | 90.56 | 86.97 | 88.69 | 88.75 | 87.45 | 13.81 | 3.63 |
Tunisia | 94.57 | 94.64 | 93.46 | 95.54 | 85.34 | 90.92 | 93.79 | 92.04 | 84.40 | 25.04 | 4.15 |
UAE | 96.09 | 95.14 | 93.41 | 94.12 | 97.66 | 93.53 | 94.45 | 91.56 | 91.79 | 31.92 | 2.00 |
Yemen | 92.34 | 91.24 | 89.40 | 92.12 | 89.54 | 89.70 | 88.25 | 89.89 | 83.08 | 5.29 | 1.29 |
Avg. | 93.19 | 92.65 | 90.75 | 90.56 | 90.49 | 89.86 | 83.81 | 81.56 | 73.98 | 19.34 | 2.73 |
๐ผ This table presents the results of a country-level cultural evaluation, assessing the performance of various models on tasks related to cultural aspects of different Arab countries. It shows the average scores for each model across all 20 countries included in the study, providing insights into their ability to capture cultural nuances in Arabic language data.
read the caption
Table 14: Country level Cultural evaluation
Full paper#
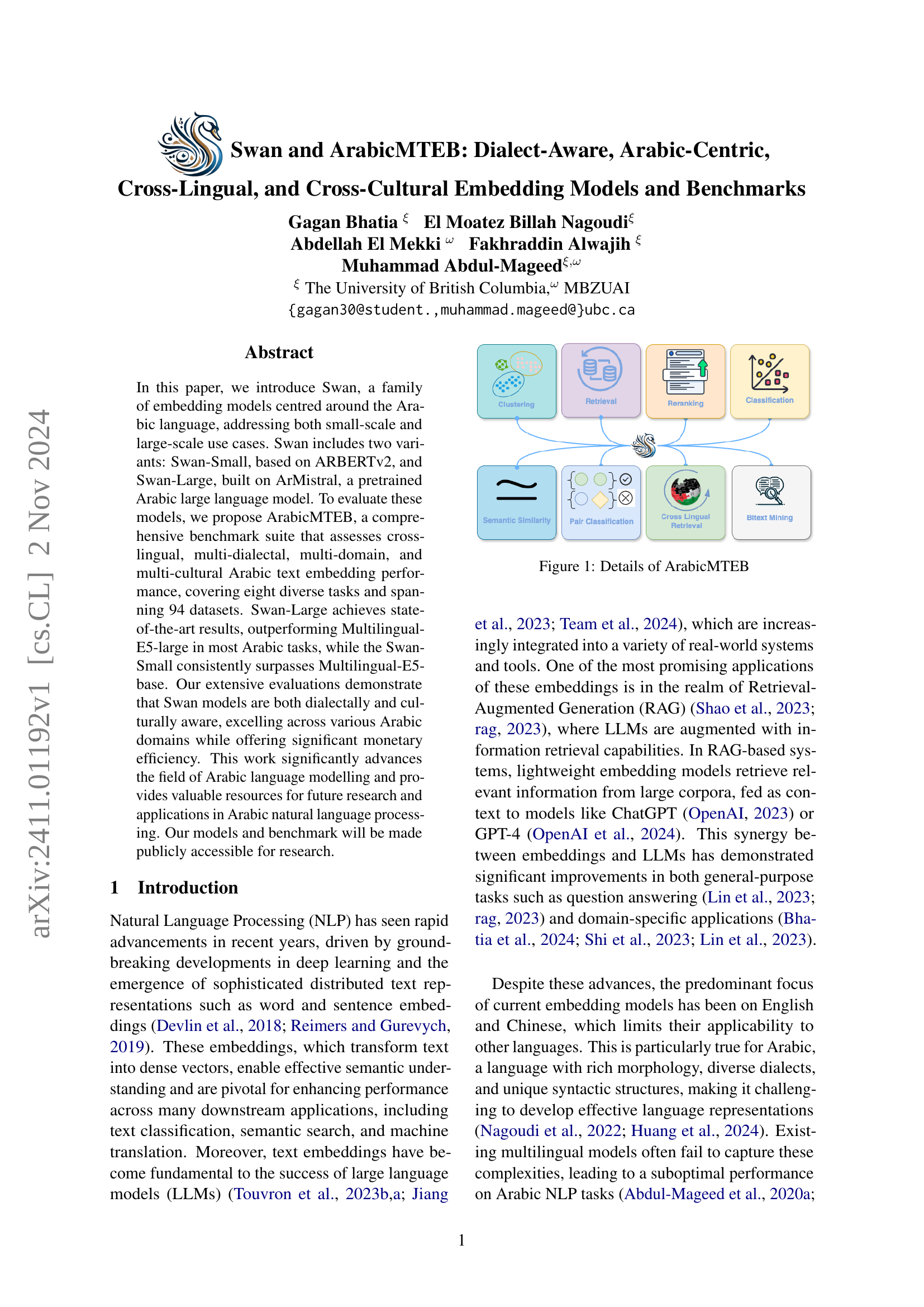
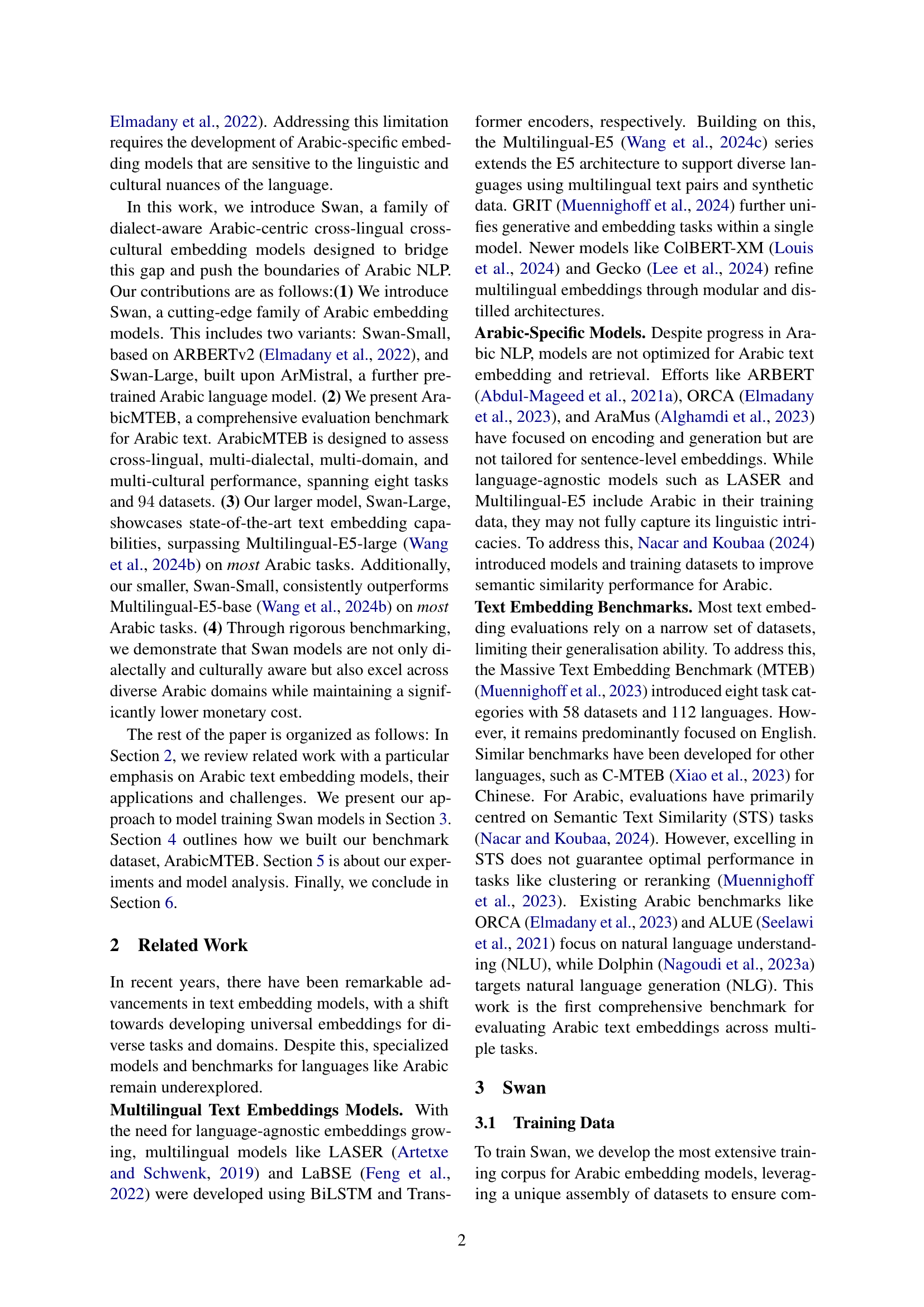
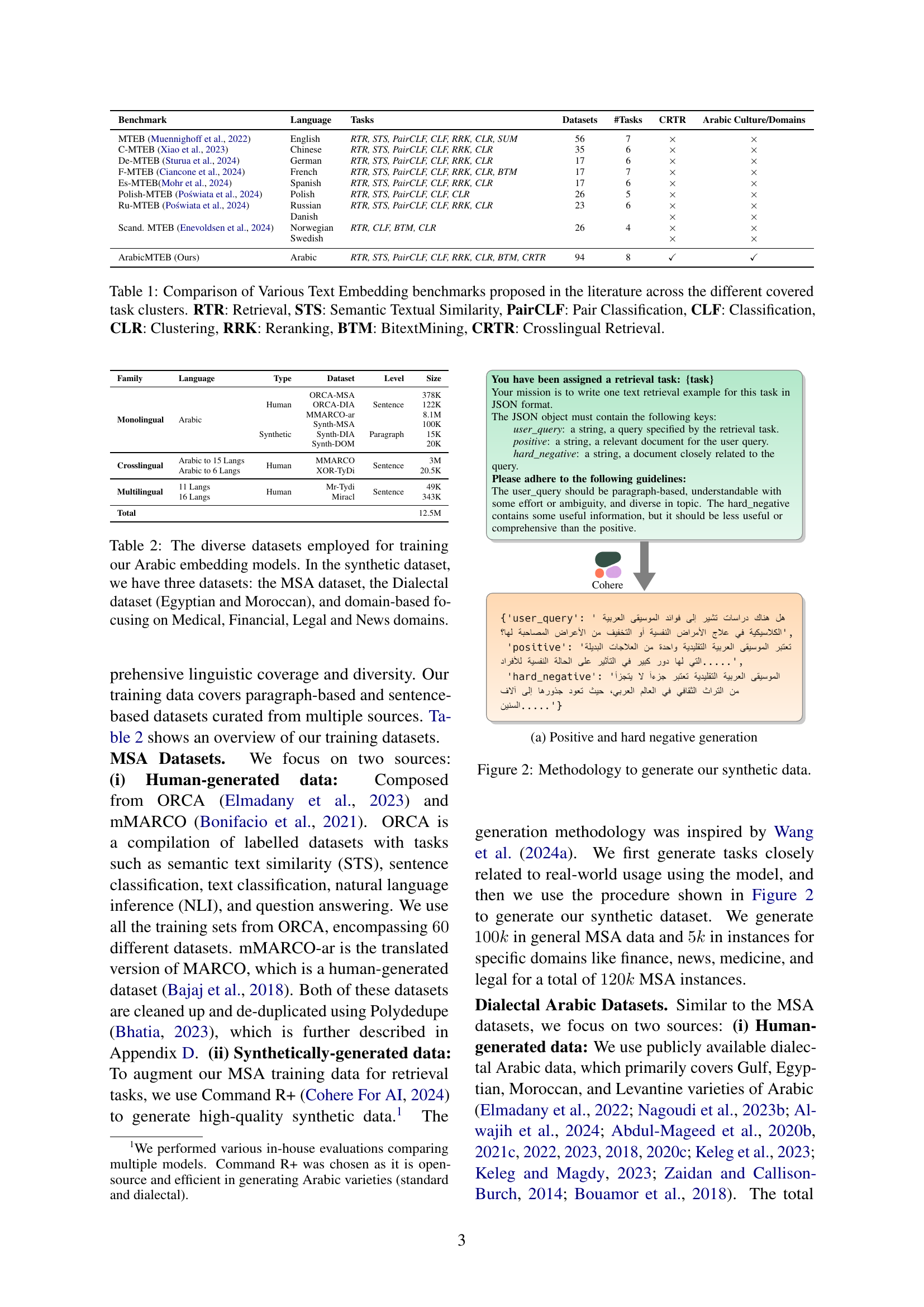
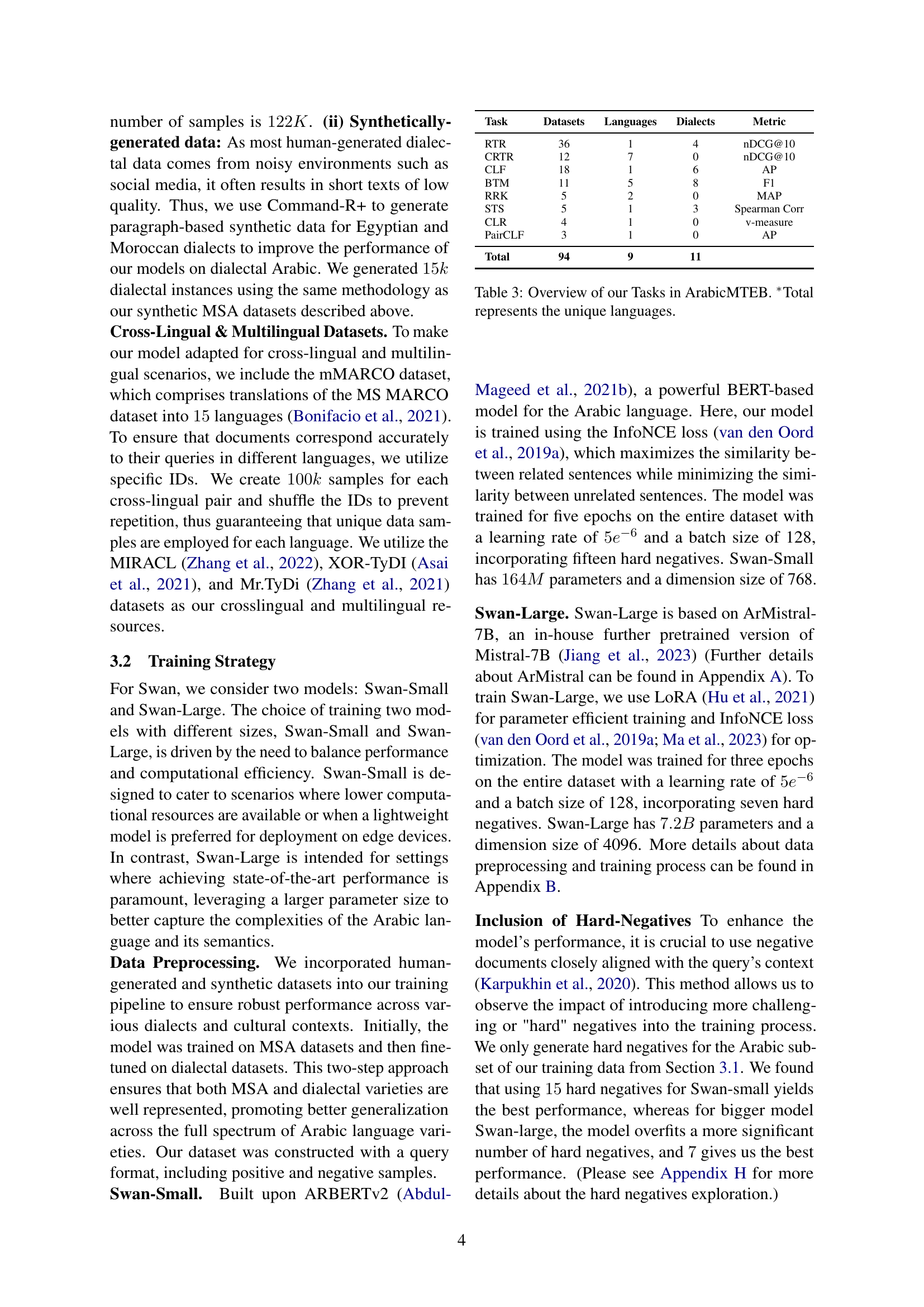
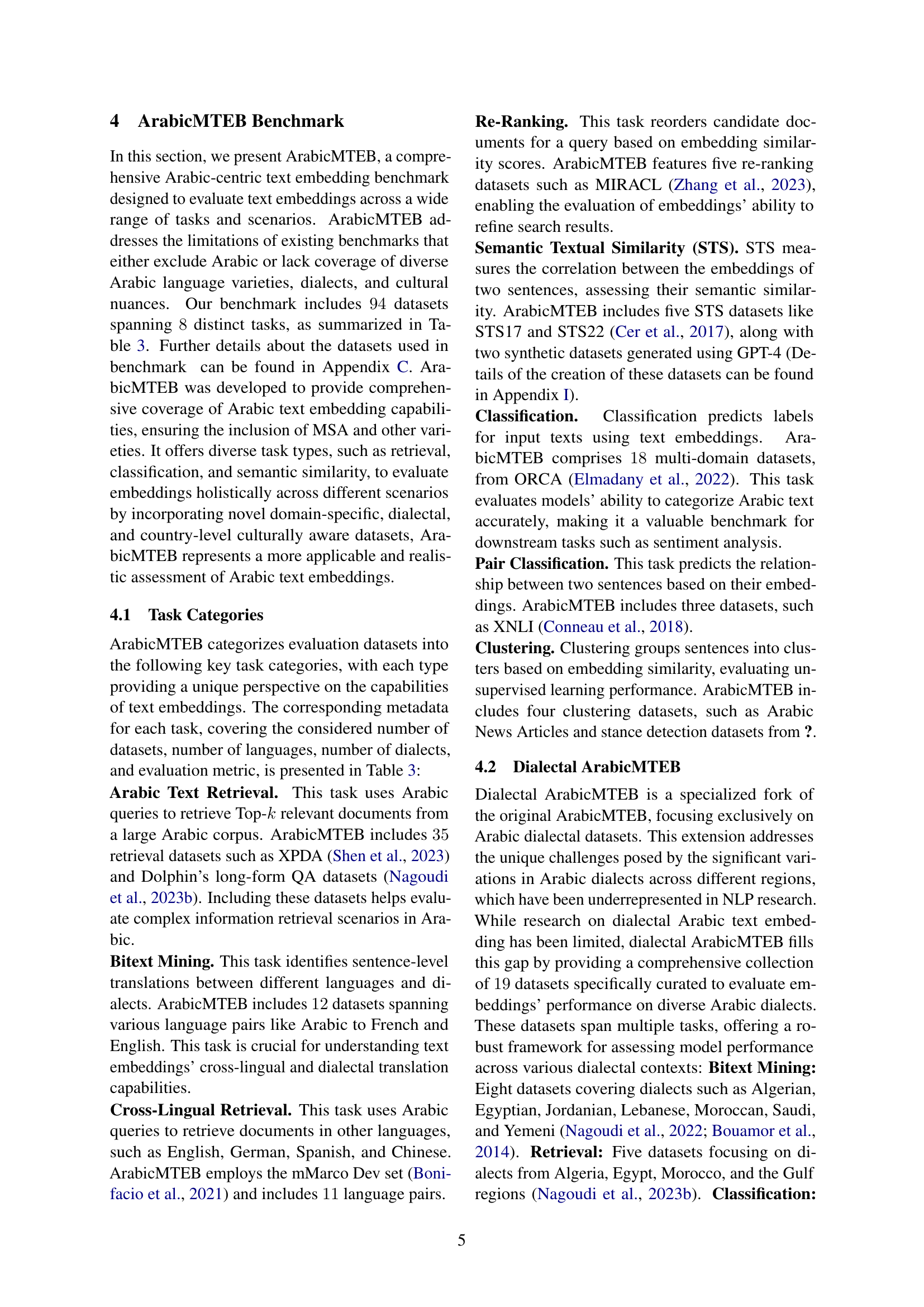
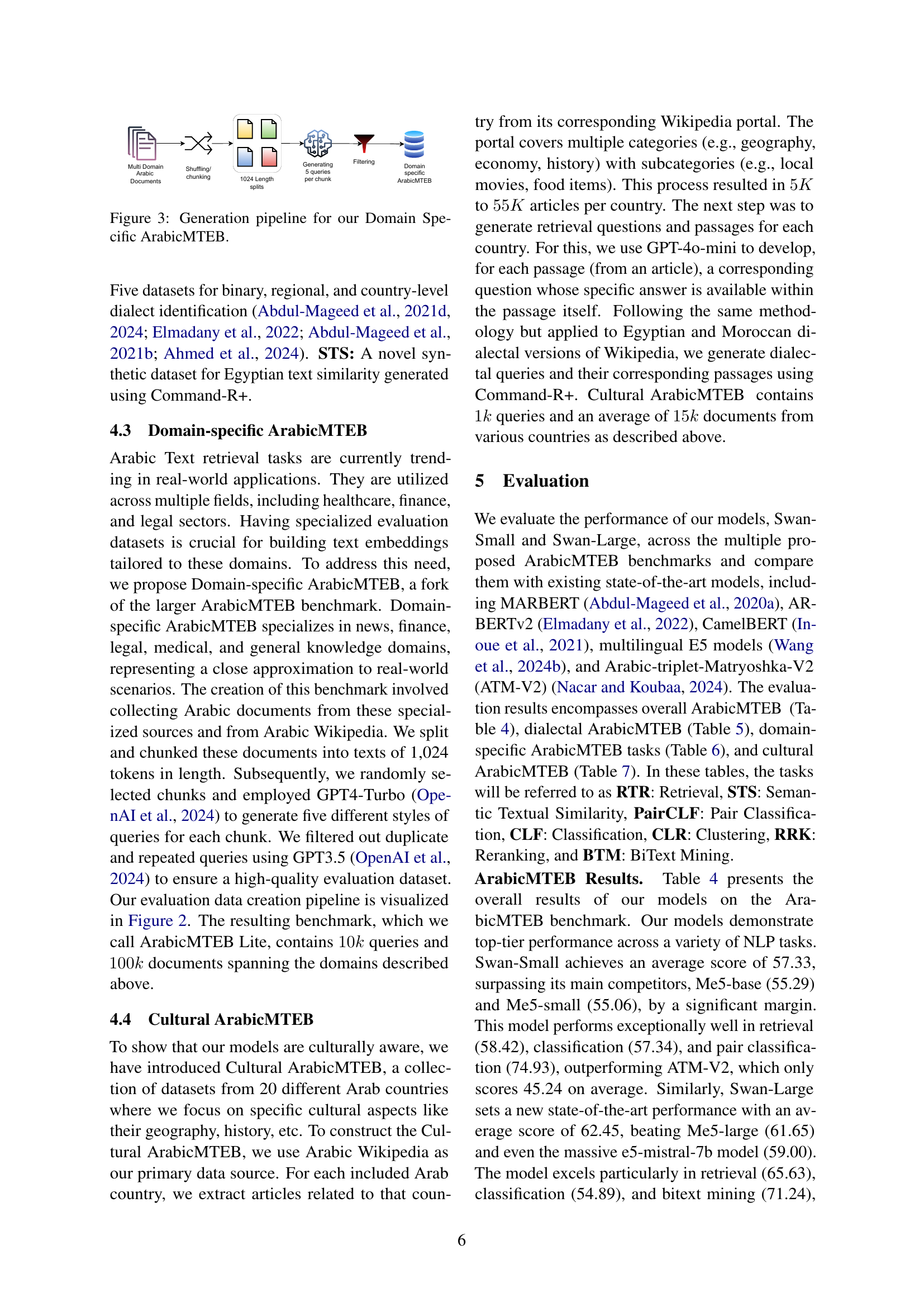
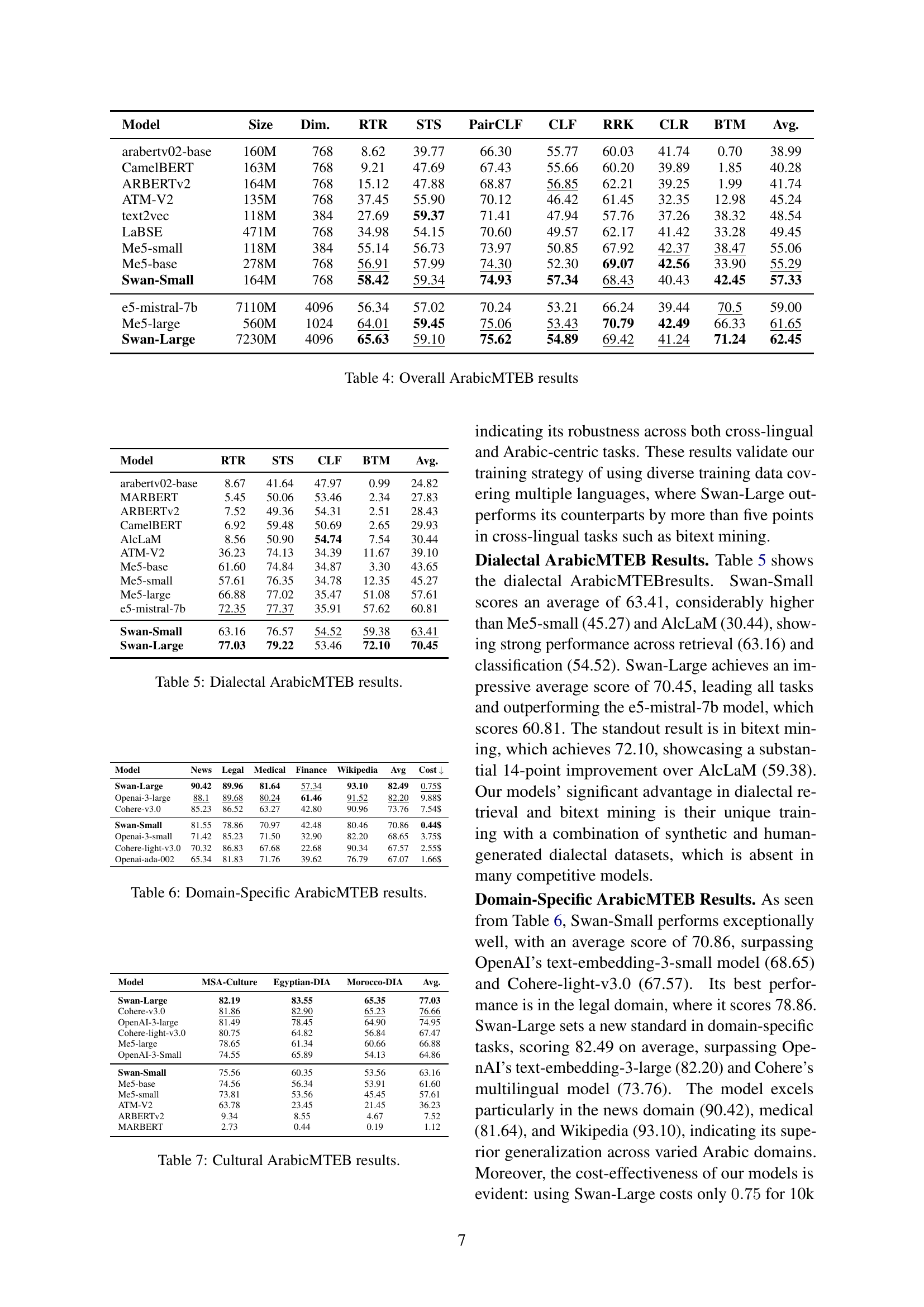
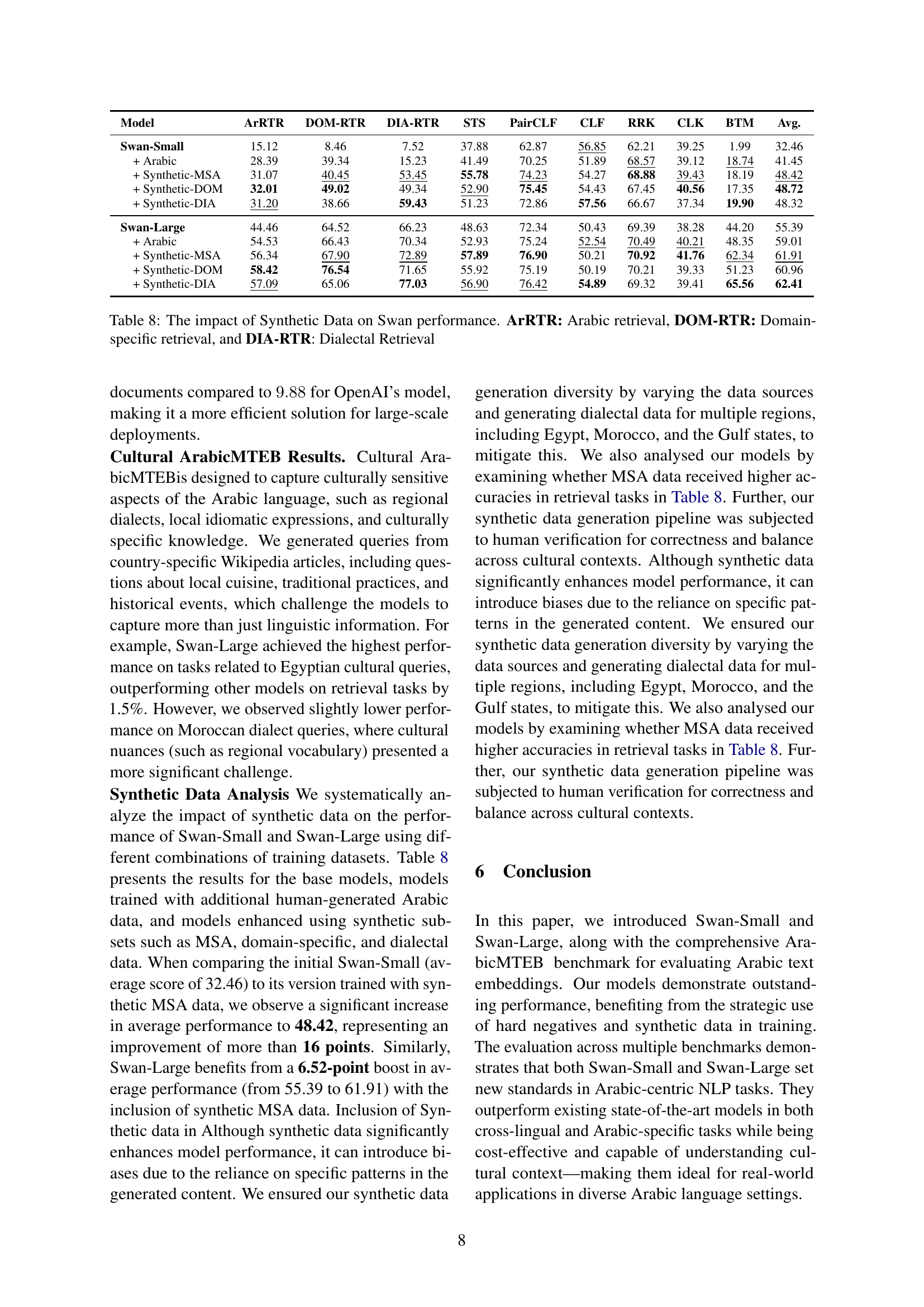
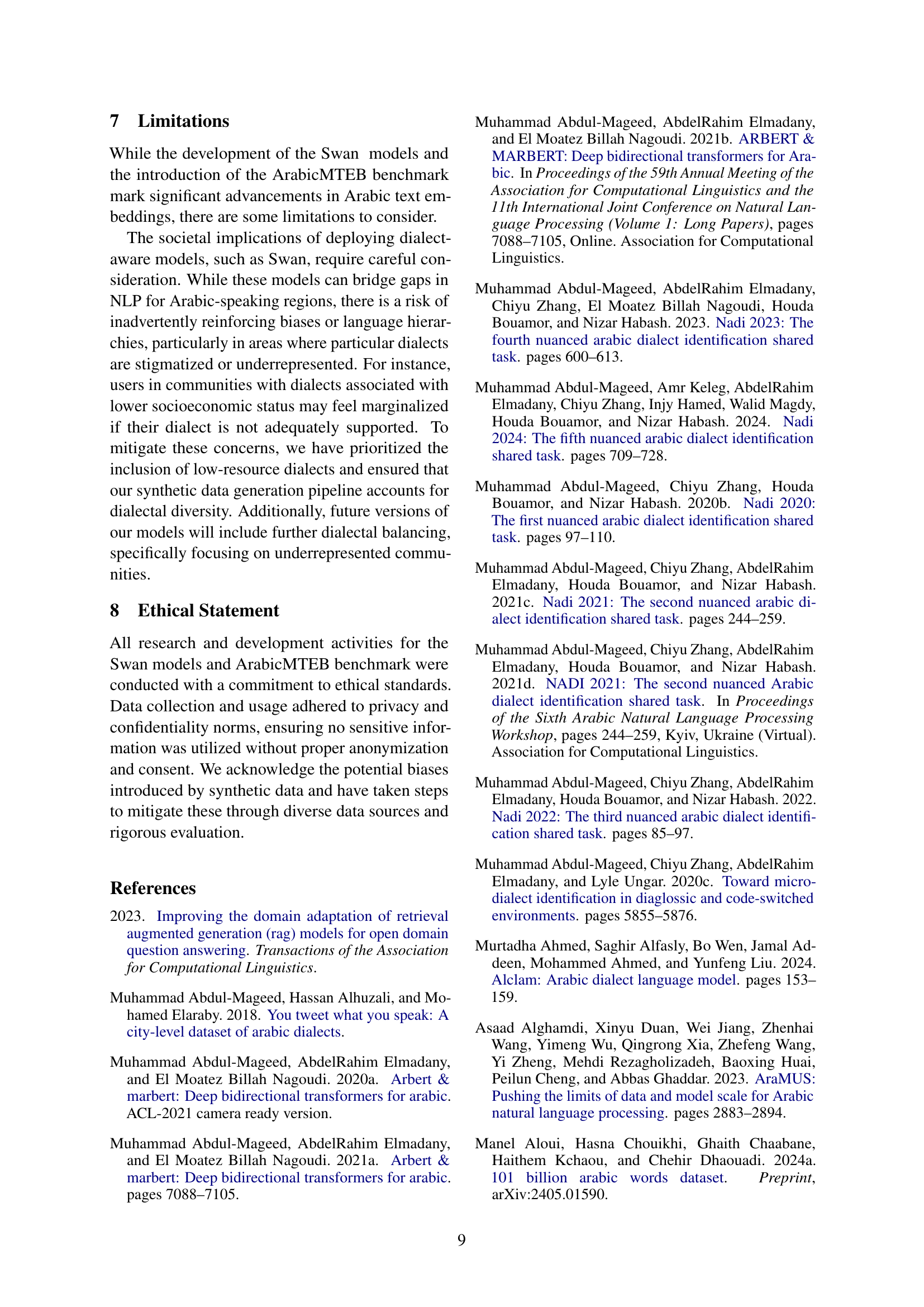
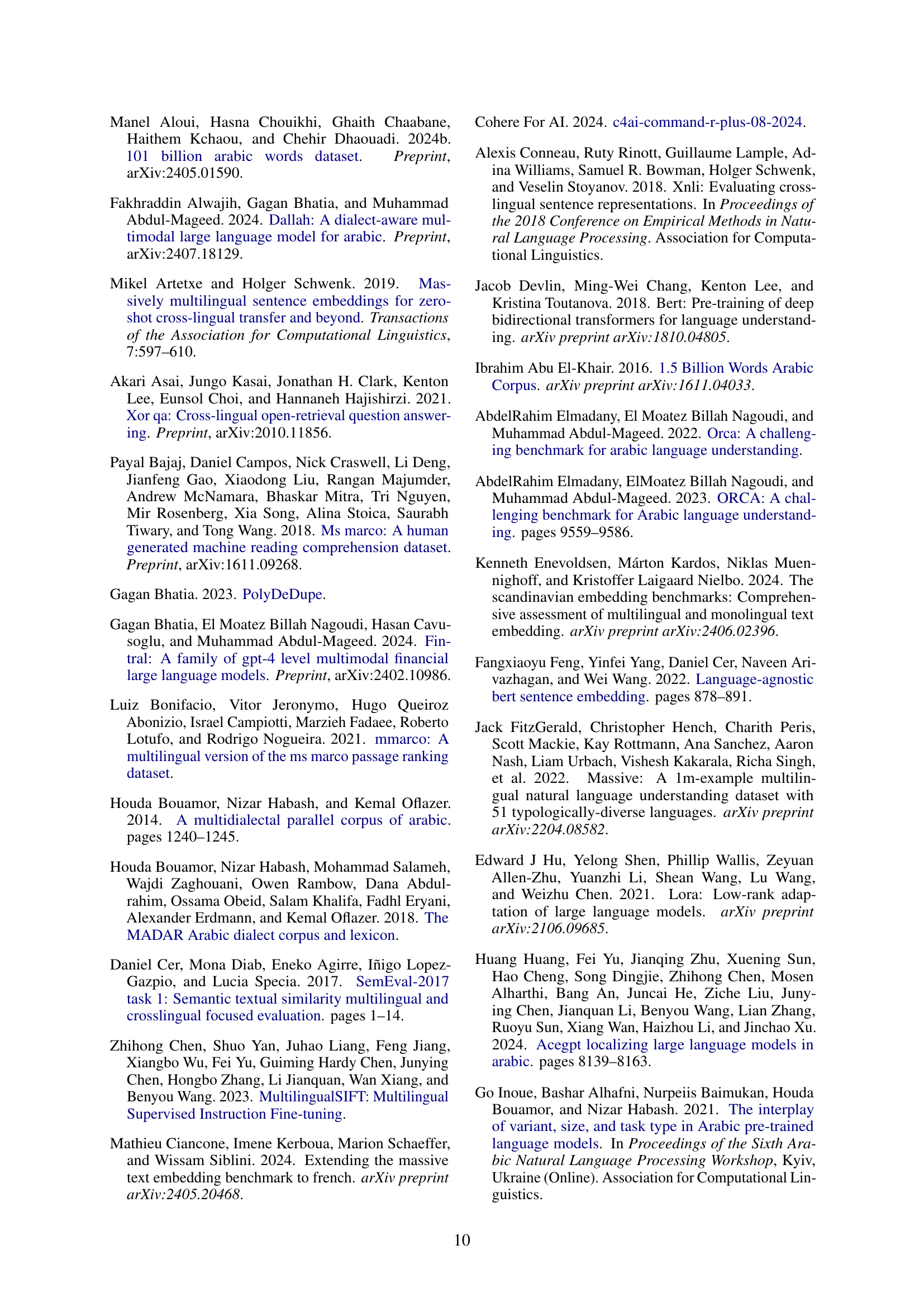
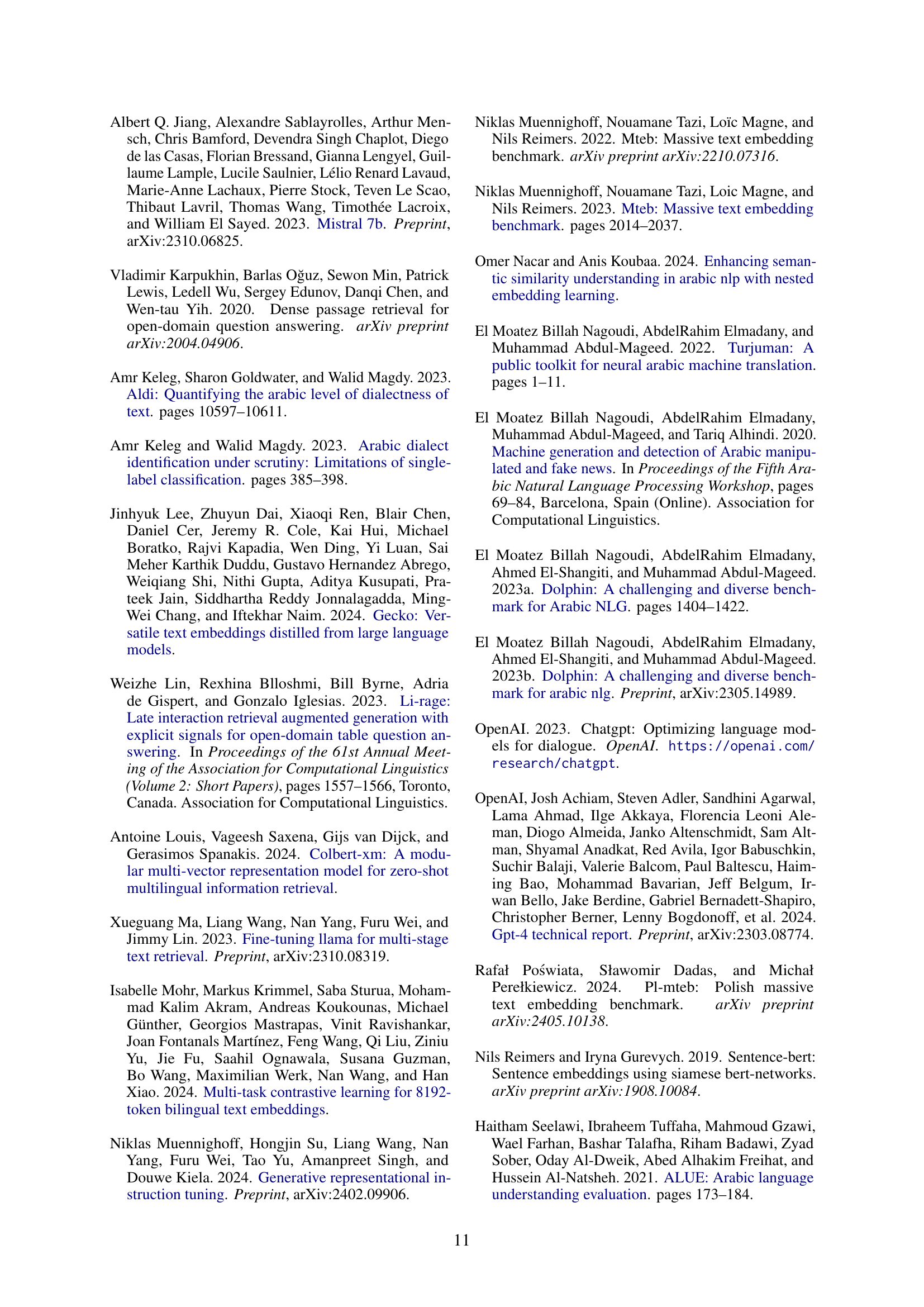
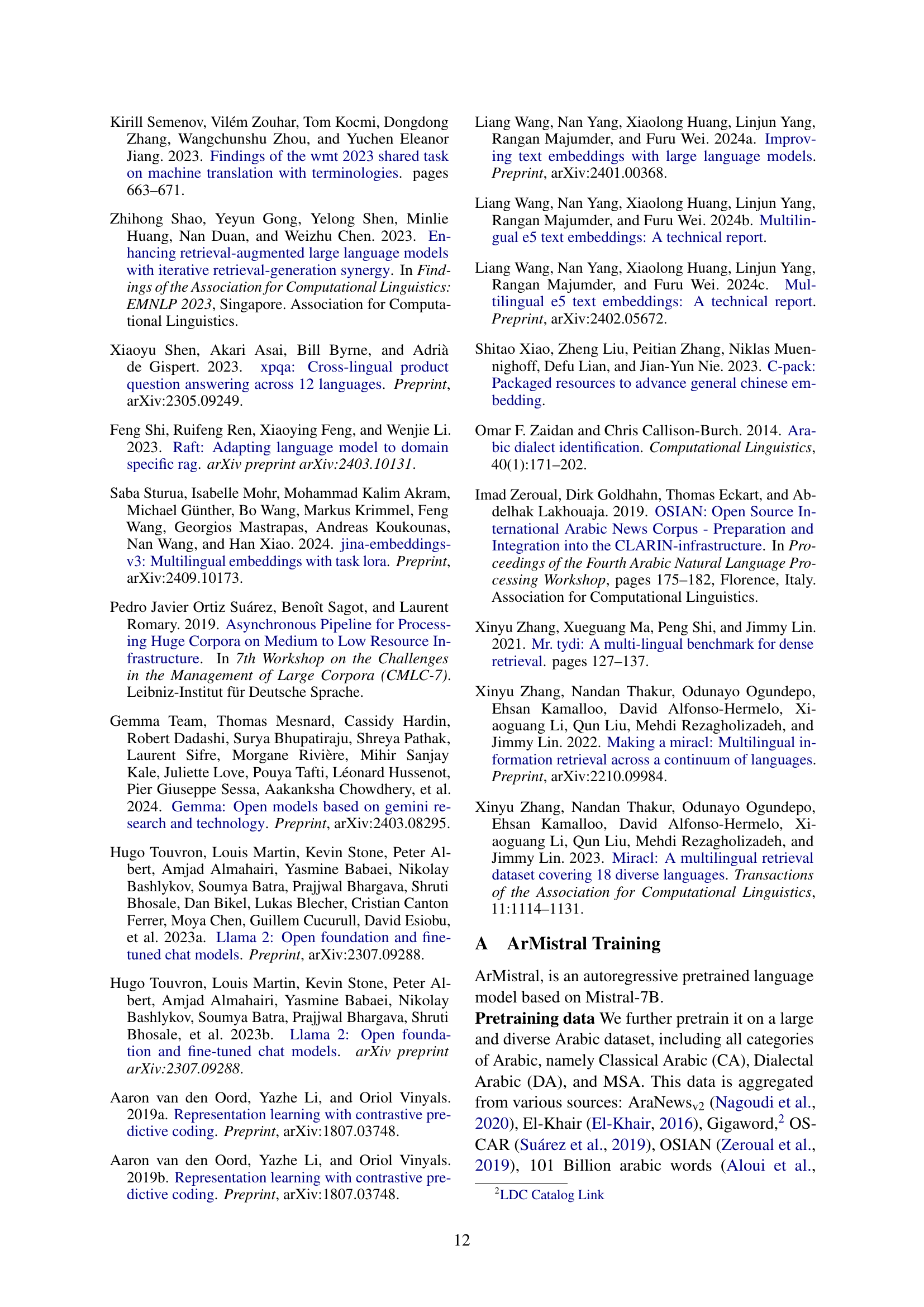
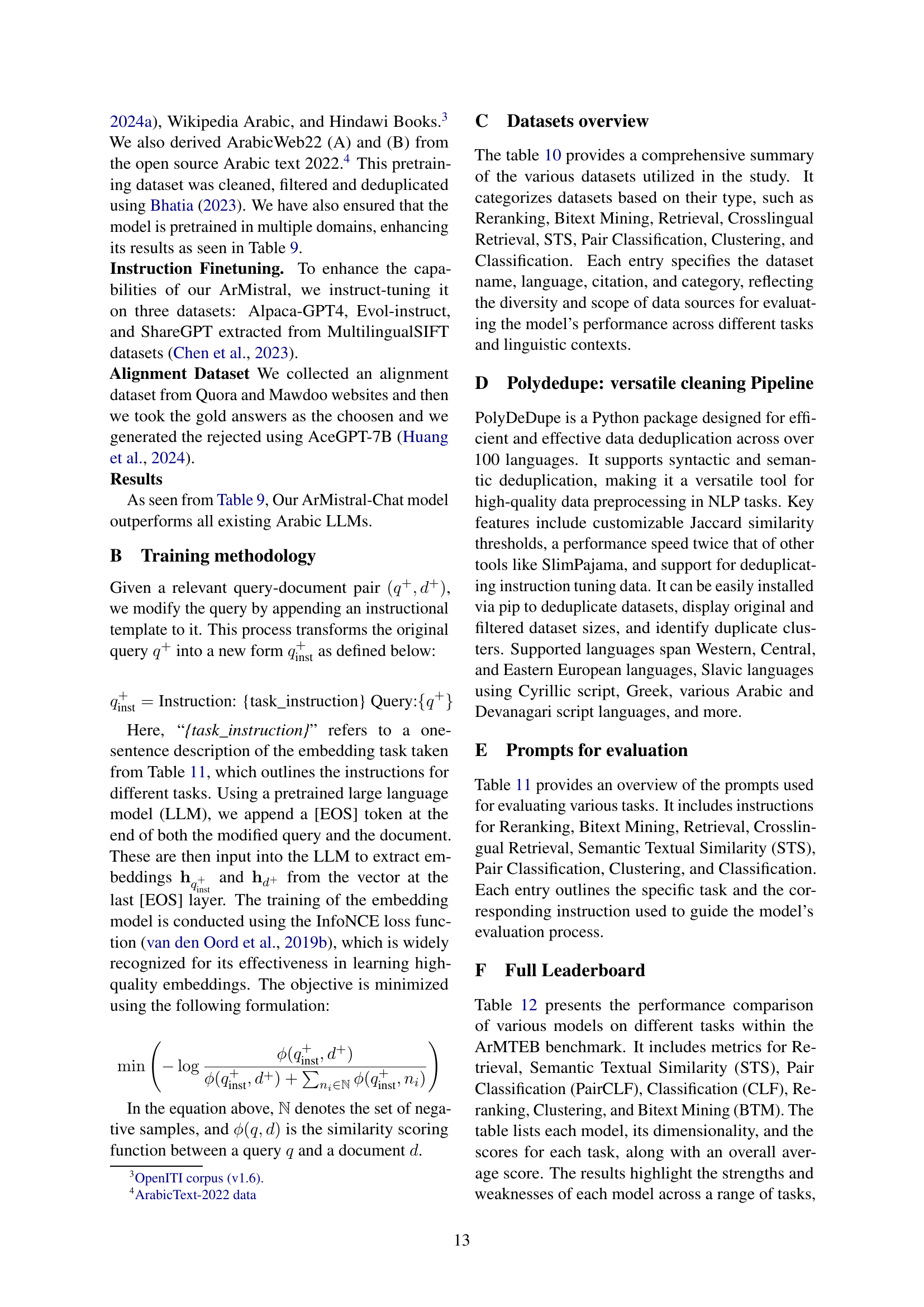
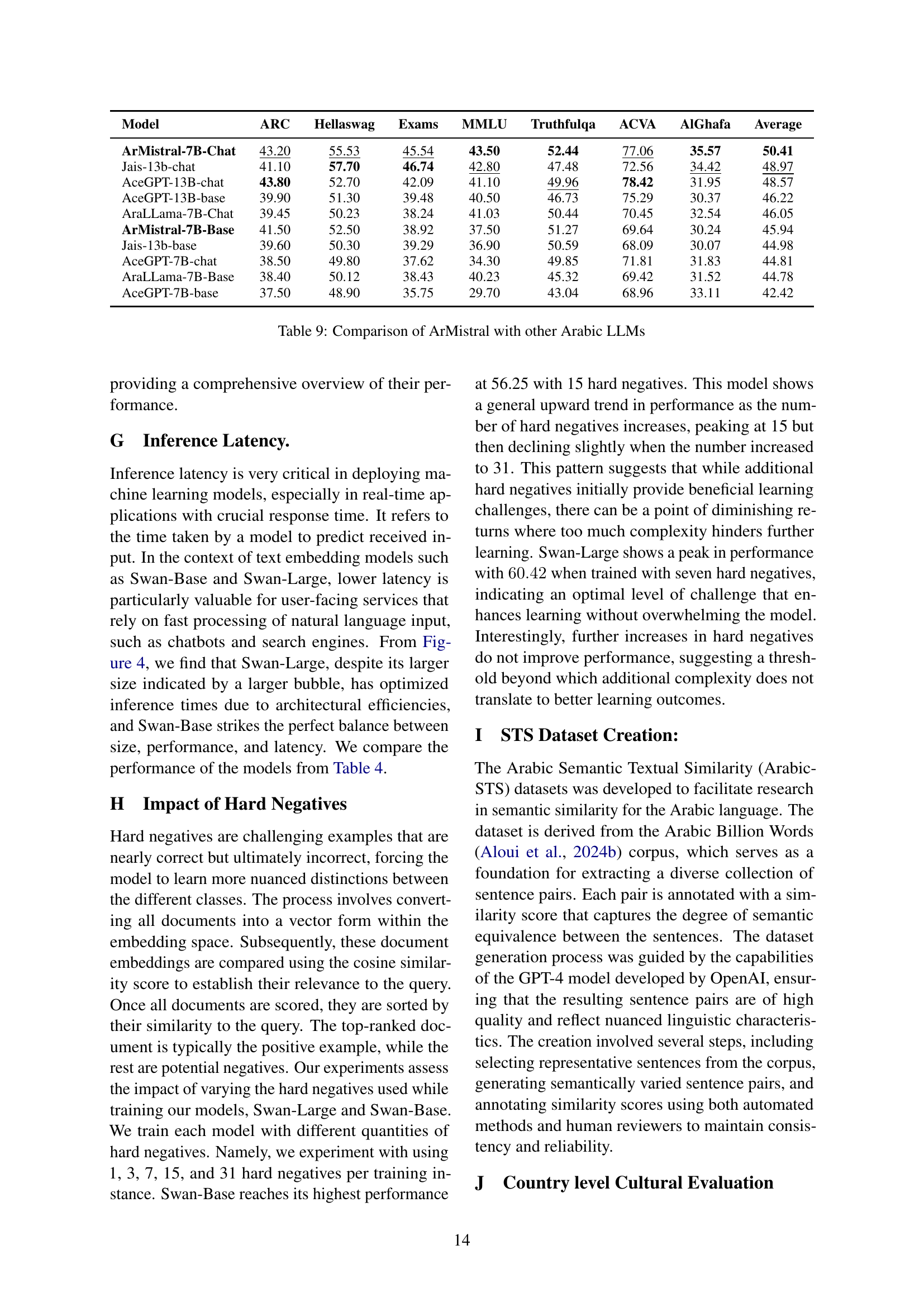
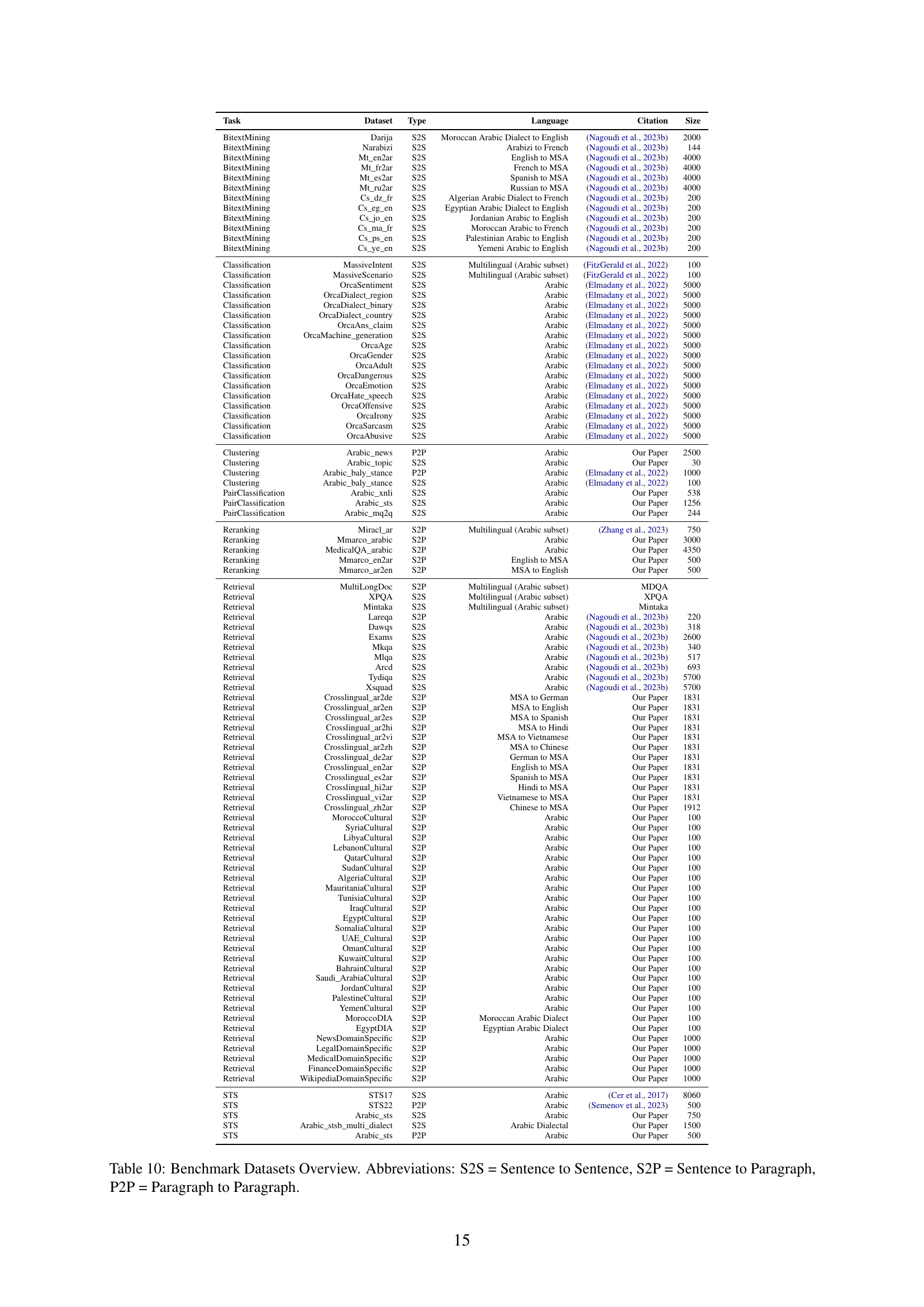
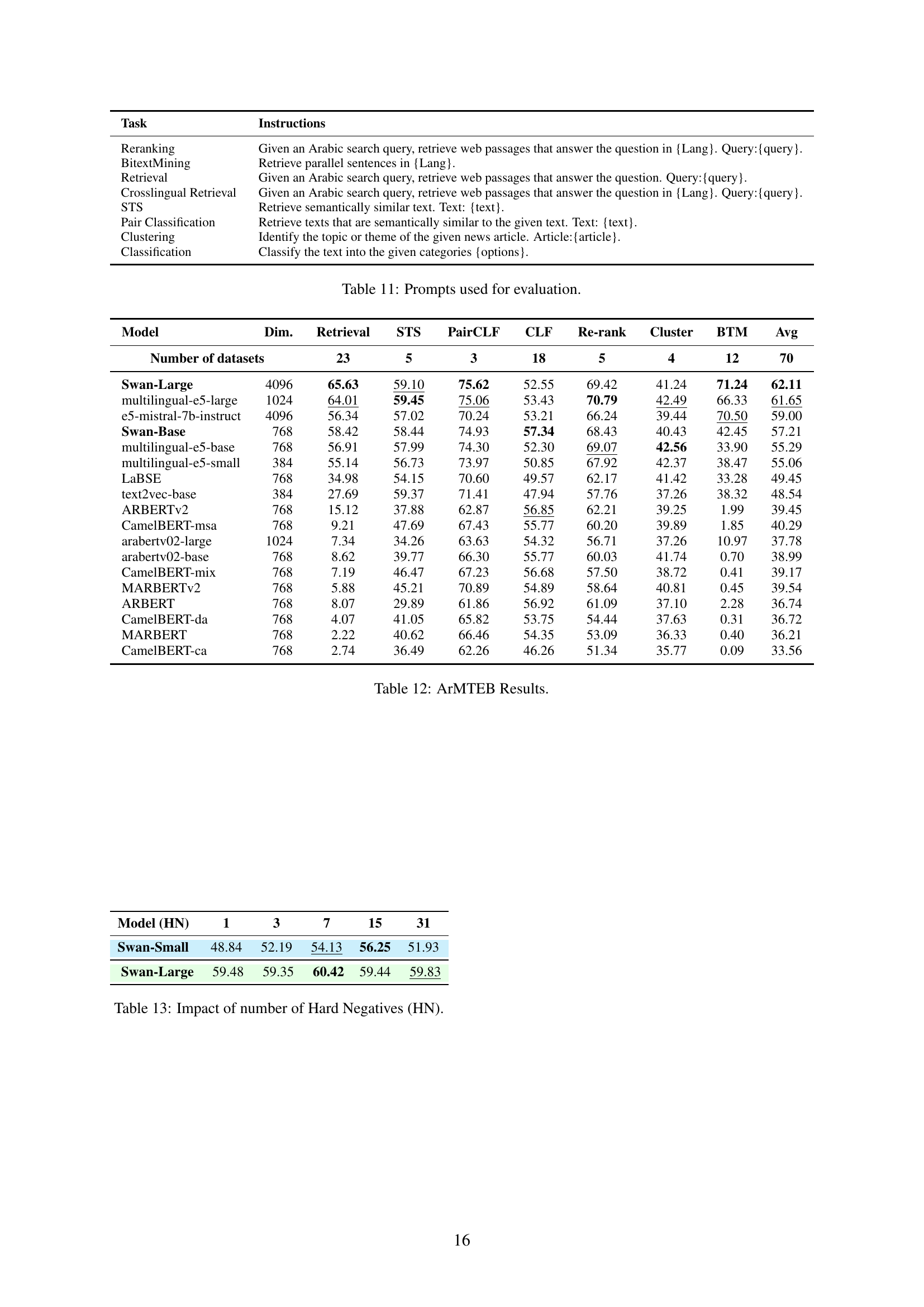
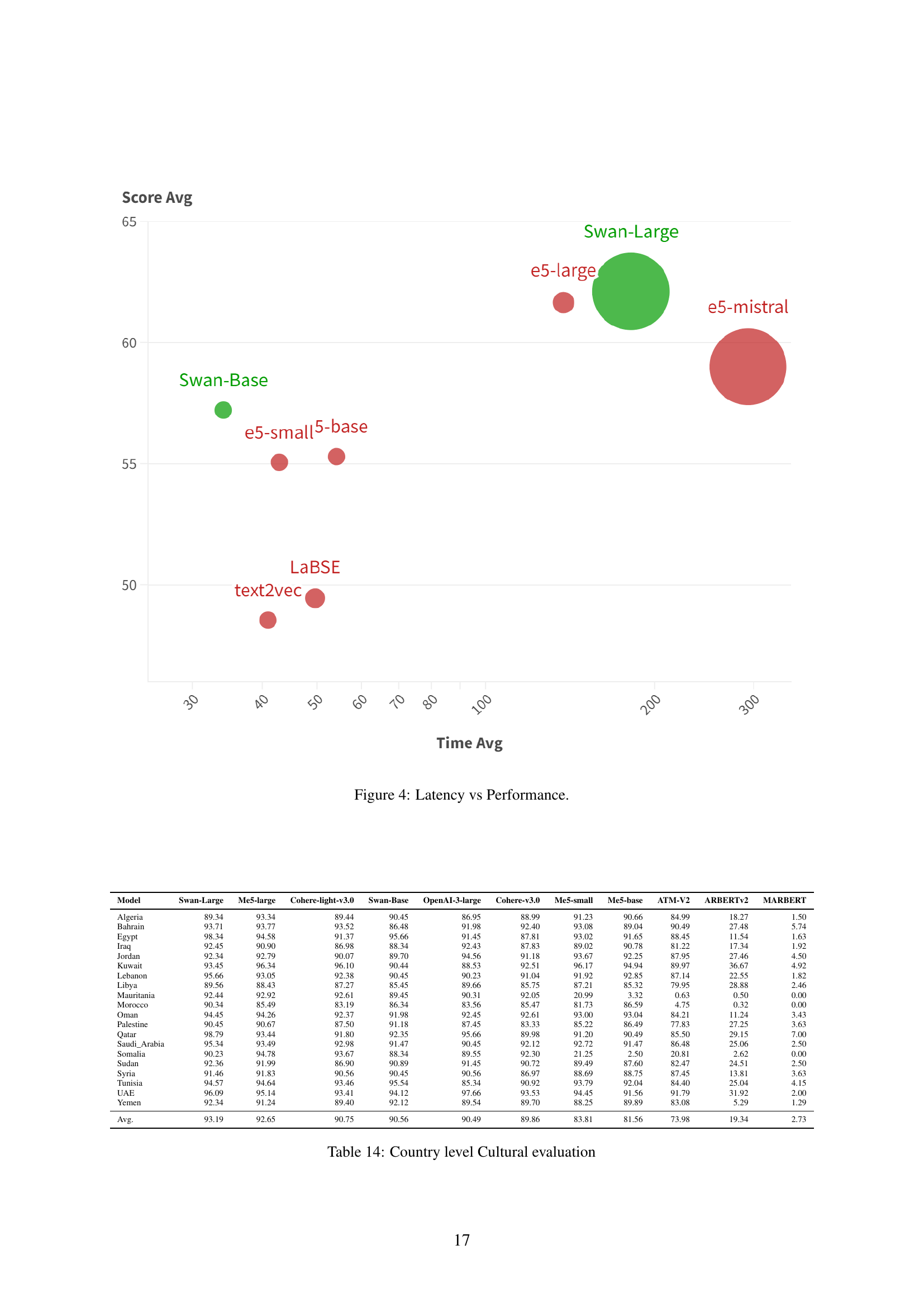