TL;DR#
Current large multimodal models (LMMs) struggle with long and high-resolution videos due to a lack of suitable datasets. This paper highlights the significant challenge of limited high-quality video instruction data, hindering advancements in video understanding. Existing datasets either have low resolution or short durations, insufficient to train robust models.
To overcome this, the researchers introduce VISTA, a video augmentation framework. VISTA synthesizes long-duration and high-resolution video data by combining existing videos and captions. It generates a new video instruction-following dataset, VISTA-400K, and a high-resolution video benchmark, HRVideoBench. Experiments demonstrate that fine-tuning models on VISTA-400K significantly improves their performance on various benchmarks, achieving an average of 3.3% improvement on long-video tasks and 6.5% on high-resolution tasks.
Key Takeaways#
Why does it matter?#
This paper is important because it addresses the critical need for high-quality datasets in long-duration and high-resolution video understanding, a currently under-explored area. VISTA-400K, a novel dataset generated by the proposed method, significantly improves the performance of existing models. This opens new avenues for research in video understanding and establishes a new benchmark, HRVideoBench, for high-resolution video analysis, pushing the field forward.
Visual Insights#
🔼 The figure illustrates the VISTA framework, which leverages existing video-caption datasets to produce high-quality video instruction-following data. VISTA combines videos both spatially and temporally to create synthetic videos with longer durations and higher resolutions. These videos are then paired with newly generated question-answer pairs, effectively augmenting the original dataset. The figure also shows a bar graph comparing the average accuracy of baseline models versus models fine-tuned with the VISTA-400K dataset, highlighting the performance improvements achieved on various video understanding benchmarks (Long Video Bench, LVBench, HRVideoBench, Short Video Bench). The improvement demonstrates VISTA’s effectiveness in enhancing video understanding models’ ability to handle long-duration and high-resolution video content.
read the caption
Figure 1: VISTA is a simple but effective framework that generates high-quality video instruction data from existing video-caption pairs. Our VISTA-400K dataset enhances model performances on various long and high-resolution video benchmarks.
Subset | Instruction Type | Video Source | #Videos | Avg. Duration | Avg. Resolution |
---|---|---|---|---|---|
Long Video Captioning | Video Captioning | Panda-70M [5] | 58,617 | 33.2s | 1277x720 |
Event Relationship QA | Freeform QA/MCQ | Panda-70M [5] | 56,854 | 33.4s | 1278x720 |
Temporal NIAH | Freeform QA/MCQ | Panda-70M [5] (N), MiraData [14] (H) | 59,751 | 67.6s | 640x358 |
Two Needle NIAH | Freeform QA | Panda-70M [5] (N), FineVideo [8] (H) | 52,349 | 112.4s | 591x382 |
Spatial NIAH | Freeform QA/MCQ | InternVid [50] (N), OpenVid-1M [33] (H) | 59,978 | 9.9s | 1726x971 |
Spatiotemporal NIAH | Freeform QA/MCQ | OpenVid-1M [33] (N), FineVideo [8] (H) | 56,494 | 89.9s | 591x383 |
HR Video Grid QA | Freeform QA/MCQ | InternVid [50] | 59,901 | 3s | 1920x1080 |
VISTA-400K | - | - | 403,944 | 48.6s | 1160x666 |
🔼 This table presents a statistical summary of the VISTA-400K dataset, a synthetic video instruction-following dataset created using the VISTA framework. It details the number of videos, average duration, and average resolution for each of the seven subsets of the dataset. Each subset employs a different video augmentation technique to create synthetic videos of varying lengths and resolutions. The ‘Needle-in-a-Haystack’ (NIAH) subsets combine short, low-resolution videos (‘N’) with longer, high-resolution videos (‘H’) to create more challenging training examples for video understanding models. The table provides crucial information for understanding the characteristics and composition of the VISTA-400K dataset.
read the caption
Table 1: Statistics of our synthetic video instruction-following dataset. “(N)” and “(H)” corresponds to the “needle” (short or low-res videos) and the “haystack” (long or high-res videos) in NIAH subsets.
In-depth insights#
Long-Video Augmentation#
The concept of ‘Long-Video Augmentation’ presents a crucial advancement in video understanding, particularly concerning the limitations of current models with short-duration video data. The core idea revolves around artificially extending the length of existing video clips to create a larger, more diverse training dataset. This addresses the scarcity of long-duration, high-quality video data, a significant bottleneck for training robust and effective video understanding models. The augmentation process likely involves techniques like concatenation of multiple short clips, possibly with careful selection to maintain narrative coherence and contextual relevance. Synthesizing long videos offers a cost-effective alternative to the expensive process of acquiring and annotating extensive, real-world long video datasets. However, careful consideration is needed to avoid introducing artificial artifacts or inconsistencies that could negatively impact model performance or lead to overfitting. The effectiveness of this method hinges on several factors, including the quality of the original short videos, the sophistication of the concatenation algorithms, and the potential need for additional data augmentation strategies to further improve the diversity of the augmented data. Ultimately, the success of long-video augmentation rests on its ability to create a synthetic dataset that sufficiently resembles real-world long videos, enabling models to generalize well to unseen long-duration video inputs.
HR-Video Benchmark#
A high-resolution video benchmark is crucial for evaluating the capabilities of video language models (VLMs) to understand fine details and subtle actions within high-resolution videos. Existing benchmarks often focus on low-resolution videos, limiting our understanding of VLM performance on the increasingly common high-resolution video data. A comprehensive HR-video benchmark would need to include diverse video types, with varied object details, subtle actions, and complex scenes. This would require careful consideration of video resolution, frame rate, and overall quality, as these factors significantly impact the performance of VLMs. The benchmark should also incorporate diverse question types, testing not just object recognition but also higher-order reasoning and temporal understanding, reflecting the nuanced complexity of high-resolution videos. A robust HR-video benchmark would greatly advance the field by facilitating the development of more sophisticated VLMs, capable of handling the richness of high-resolution video information and contributing to numerous real-world applications. Furthermore, it could highlight the limitations of current VLMs and guide future research on model architecture and training data towards improving their comprehension and reasoning abilities with high-resolution videos.
VISTA Dataset#
The VISTA dataset represents a novel approach to augmenting video data for improved long-duration and high-resolution video understanding. Instead of relying solely on collecting new videos, VISTA cleverly synthesizes new video-instruction pairs from existing datasets. This is achieved by spatially and temporally combining existing videos and generating corresponding question-answer pairs, thereby expanding the scope and resolution of the training data. The resulting VISTA-400K dataset is substantial, comprising a diverse array of synthesized videos, significantly increasing the quantity of high-quality long and high-resolution video-instruction data. This data augmentation strategy addresses a critical bottleneck in video LMM training, proving its effectiveness through improved performance on various benchmarks, highlighting the power of data-centric solutions to enhance video comprehension capabilities. A particularly valuable contribution is the introduction of HRVideoBench, a benchmark specifically designed for evaluating high-resolution video understanding, further underscoring the impact of VISTA’s contribution.
Model Finetuning#
Model finetuning in the context of large multimodal models (LMMs) for video understanding involves adapting pre-trained models to excel at specific video-related tasks. This process is crucial because LMMs, while powerful, often require further specialization to handle the nuances of long-duration and high-resolution videos. The effectiveness of finetuning hinges on the quality and diversity of the training dataset. A well-curated dataset, such as the VISTA-400K dataset described in the paper, allows the model to learn essential spatiotemporal relationships and high-resolution details. Augmentation techniques further enhance the dataset, creating synthetic data to address the scarcity of naturally occurring high-quality, long videos. The results demonstrate that finetuning on this augmented data leads to substantial improvements across various video understanding benchmarks, showcasing the significance of a data-centric approach to improving LMMs for video. The improvements highlight the importance of high-quality data in finetuning. Careful consideration of the benchmark selection is also essential; as demonstrated in the paper, the creation of HRVideoBench enables a proper assessment of high-resolution video understanding, an area previously overlooked.** Finally, the choice of base model significantly influences the results; different models will have varying levels of adaptability and benefit differently from finetuning. Therefore, a comprehensive model finetuning strategy must consider the dataset, augmentation techniques, benchmark choice, and the suitability of the base model.
Future Work#
The paper’s ‘Future Work’ section could explore several promising avenues. Improving the video augmentation techniques is crucial. Currently, the methods are primarily based on simple spatial and temporal combinations; more sophisticated techniques like generative models or advanced video editing algorithms could create more realistic and diverse synthetic data. Expanding the dataset is vital. While VISTA-400K is significant, a larger and more varied dataset with a broader range of video types and qualities would further improve model performance. In addition to quantity, improving the quality of captions and QA pairs through more advanced language models or human annotation will result in more accurate and informative training data. Finally, investigating the transferability of models trained on VISTA-400K to other video understanding tasks is key to validating the framework’s generality. This would involve comprehensive testing on various benchmarks for diverse downstream tasks. Addressing these aspects will enhance the robustness and applicability of the proposed approach.
More visual insights#
More on figures
🔼 This figure illustrates the VISTA framework’s seven video augmentation methods for generating synthetic video instruction-following data. Starting with input short videos and their captions, VISTA spatially and temporally combines them to create longer, higher-resolution videos (e.g., by concatenating clips, inserting short clips into longer ones at different timepoints or locations, or arranging low-resolution videos in a grid). Then, using a large language model, VISTA synthesizes question-answer pairs about these new, augmented videos. Each of the seven subsets demonstrates a different augmentation technique, including methods for creating long videos, long video captions, questions about event relationships, and various needle-in-a-haystack (NIAH) QA pairs for testing temporal and spatial video understanding.
read the caption
Figure 2: Our proposed video augmentation and instruction-following data synthesis schemes for VISTA-400K. Given input videos, We perform spatiotemporal video combinations to produce augmented video samples with longer duration and higher resolution.
🔼 Figure 3 presents a qualitative analysis comparing the performance of baseline video language models (VLMs) against VLMs fine-tuned using the VISTA dataset. Two example scenarios are shown: ‘helicopter’ and ’table tennis’. Each scenario displays the question, followed by the responses generated by several models (baseline LongVA, baseline VideoLLaVA, VISTA-enhanced LongVA, and VISTA-enhanced VideoLLaVA for the ‘helicopter’ example; baseline Mantis-Idefics2, and VISTA-enhanced Mantis-Idefics2 for the ’table tennis’ example). Incorrect or hallucinated responses are highlighted in red, while accurate responses are shown in green. This visual comparison highlights how VISTA improves the accuracy and reduces hallucinatory outputs of VLMs.
read the caption
Figure 3: Qualitative comparisons between the baseline models and our VISTA-finetuned models. Red text indicates hallucinations or incorrect responses, while green text highlights the correct responses that correspond accurately to the video content.
🔼 This figure showcases two example questions from the HRVideoBench dataset, a high-resolution video understanding benchmark. The examples highlight the dataset’s focus on evaluating fine-grained object details and subtle actions within high-resolution videos. The first example requires identifying a car’s color in a rearview mirror, demonstrating the need for precise object recognition at high resolution. The second example tasks the model with determining the directional movement of a person from a specific angle and at a specific location within the video, showcasing the benchmark’s assessment of localized action recognition. The image emphasizes the high resolution and detailed nature of the videos used in the HRVideoBench.
read the caption
Figure 4: Example questions from our HRVideoBench. Zoom in for better visualizations.
More on tables
Long Video Understanding | Short Video Understanding | |
---|---|---|
Video-MME w/o subtitles | MLVU | LVBench |
LongVideoBench | MVBench | |
NExT-QA | ||
— | — | — |
Models | Size | avg |
— | — | — |
Proprietary Models | ||
GPT-4V [1] | - | 59.9 |
GPT-4o [35] | - | 71.9 |
Gemini-1.5-Pro [44] | - | 75.0 |
Open-source Models | ||
VideoChat2 [23] | 7B | 39.5 |
LLaMA-VID [25] | 7B | - |
ST-LLM [29] | 7B | 37.9 |
ShareGPT4Video [4] | 7B | 39.9 |
LongVILA [55] | 7B | 50.5 |
LongLLaVA [49] | 7B | 52.9 |
Video-XL [41] | 7B | 55.5 |
VideoLLaVA [26] | 7B | 39.9 |
VISTA-VideoLLaVA | 7B | 43.7 |
Δ - VideoLLaVA | +3.8 | |
Mantis-Idefics2 [13] | 8B | 45.4 |
VISTA-Mantis | 8B | 48.2 |
Δ - Mantis-Idefics2 | +2.8 | |
LongVA [63] | 7B | 52.4 |
VISTA-LongVA | 7B | 55.5 |
Δ - LongVA | +3.1 |
🔼 This table compares the performance of several baseline video language models (LLMs) against versions of those same models fine-tuned on the VISTA-400K dataset. The comparison is made across multiple benchmarks designed to test both long and short video understanding capabilities. The table shows the average performance across multiple categories, including ‘short,’ ‘medium,’ and ’long’ video lengths, as well as overall performance. The best results achieved by open-source models are highlighted in bold. The final column indicates the performance improvement (Δ) after fine-tuning with VISTA-400K.
read the caption
Table 2: Comparisons between baseline models and VISTA-finetuned models on long/short video understanding benchmarks. The best results among open-source models are bolded. ΔΔ\Deltaroman_Δ denotes the performance differences before and after finetuning on VISTA-400K.
HRVideoBench | MSVD-QA | MSRVTT-QA | TGIF-QA | ActivityNet-QA | |||||||
---|---|---|---|---|---|---|---|---|---|---|---|
High-Res Video Understanding | Open-Ended Video QA | ||||||||||
Models | avg | object | action | acc. | score | acc. | score | acc. | score | acc. | score |
VideoLLaVA [26] | 32.5 | 36.0 | 27.9 | 60.3 | 3.7 | 42.1 | 3.0 | 63.5 | 3.8 | 48.6 | 3.3 |
VISTA-VideoLLaVA | 47.5 | 50.0 | 44.2 | 71.5 | 4.0 | 58.5 | 3.5 | 78.0 | 4.3 | 49.1 | 3.4 |
Δ - VideoLLaVA | +15.0 | +14.0 | +16.3 | +11.2 | +0.3 | +16.4 | +0.5 | +14.5 | +0.5 | +0.5 | +0.1 |
Mantis-Idefics2 [13] | 48.5 | 50.9 | 45.4 | 57.4 | 3.5 | 34.9 | 2.7 | 65.7 | 3.8 | 46.5 | 3.1 |
VISTA-Mantis | 51.0 | 53.5 | 47.7 | 65.2 | 3.8 | 46.4 | 3.1 | 71.4 | 4.0 | 48.8 | 3.3 |
Δ - Mantis | +2.5 | +2.6 | +2.3 | +7.8 | +0.3 | +11.5 | +0.4 | +5.7 | +0.2 | +2.3 | +0.2 |
LongVA [63] | 48.0 | 52.6 | 41.9 | 56.3 | 3.5 | 37.7 | 2.8 | 55.4 | 3.4 | 48.0 | 3.2 |
VISTA-LongVA | 50.0 | 56.1 | 41.9 | 61.0 | 3.7 | 42.5 | 3.0 | 67.5 | 3.9 | 51.8 | 3.4 |
Δ - LongVA | +2.0 | +3.5 | +0.0 | +4.7 | +0.2 | +4.8 | +0.2 | +12.1 | +0.5 | +3.8 | +0.2 |
🔼 This table presents a quantitative comparison of different video language models’ performance on high-resolution video understanding and open-ended video question answering tasks. The HRVideoBench benchmark assesses the models’ ability to understand high-resolution video details, while the open-ended benchmarks (MSVD-QA, MSRVTT-QA, TGIF-QA, and ActivityNet-QA) evaluate their performance on general video question-answering tasks. The table shows the average accuracy (acc.) and scores achieved by each model on each benchmark. Specifically for HRVideoBench, both object and action understanding accuracies are shown.
read the caption
Table 3: Quantitative results on HRVideoBench and open-ended video QA benchmarks. “acc.” represents accuracy.
Models | Video-MME | HRVideoBench |
---|---|---|
Video-MME | w/o sub. avg | avg |
VISTA-Mantis | 48.2 | 51.0 |
w/o Long Video Captioning | 47.9 | 48.0 |
w/o Event Relationship QA | 47.7 | 49.5 |
w/o Temporal NIAH | 47.5 | 48.0 |
w/o Two Needle NIAH | 48.1 | 50.5 |
w/o Spatial NIAH | 47.2 | 47.5 |
w/o Spatiotemporal NIAH | 47.7 | 50.0 |
w/o HR Video Grid QA | 47.8 | 48.0 |
w/o Video Augmentation | 45.7 | 44.5 |
🔼 This ablation study investigates the impact of each video augmentation subset within VISTA-400K on the performance of the Mantis-Idefics2 model. For each row, a modified version of VISTA-400K is created by replacing one of the seven subsets with an equal number of training examples from the VideoChat2-IT dataset. The table shows the average performance scores on the Video-MME and HRVideoBench benchmarks for the modified models, highlighting the contribution of each subset to the overall performance gains.
read the caption
Table 4: Ablation study results for VISTA-Mantis. Each “w/o [Subset]” denotes a Mantis-Idefics2 model finetuned on a modified VISTA-400K by replacing the corresponding subset with the same amount of training examples from VideoChat2-IT [23].
avg | short | medium | long | m-avg | test | val | |
---|---|---|---|---|---|---|---|
Long Video Understanding | |||||||
Video-MME w/o subtitles | |||||||
Models | |||||||
VideoLLaVA | 39.9 | 45.3 | 38.0 | 36.2 | 45.0 | 29.3 | 39.1 |
VideoLLaVA (SFT on VISTA-400K) | 43.6 | 47.3 | 43.8 | 39.8 | 48.7 | 32.6 | 41.0 |
Δ - VideoLLaVA | +3.7 | +2.0 | +5.8 | +3.6 | +3.7 | +3.3 | +1.9 |
VideoLLaVA (SFT on VISTA-400K + 300K VideoChat2-IT) | 43.7 | 48.2 | 43.9 | 38.9 | 49.5 | 33.8 | 42.3 |
Δ - VideoLLaVA (SFT on VISTA-400K) | +0.1 | +0.9 | +0.1 | -0.9 | +0.8 | +1.2 | +1.3 |
🔼 This table presents a comparison of the performance of three different models on long video understanding benchmarks. The first model is the baseline VideoLLaVA model. The second model is VideoLLaVA finetuned on the VISTA-400K dataset. The third model is VideoLLaVA finetuned on both the VISTA-400K dataset and an additional 300K videos from the VideoChat2-IT dataset. The benchmarks used are Video-MME, MLVU, LVBench, and LongVideoBench. The table shows the average performance across short, medium, and long video clips for each benchmark and model, as well as the improvement achieved by fine-tuning. ‘SFT’ denotes supervised finetuning.
read the caption
Table 5: Comparison between the baseline VideoLLaVA model, VideoLLaVA finetuned on VISTA-400K and VideoLLaVA finetuned on VISTA-400K + 300K VideoChat2-IT data (VISTA-VideoLLaVA in the main paper) on long video understanding benchmarks. “SFT” indicates supervised finetuning.
Models | avg | object | action |
---|---|---|---|
High-Resolution Video Understanding | |||
HRVideoBench | |||
VideoLLaVA | 32.5 | 36.0 | 27.9 |
VideoLLaVA (SFT on VISTA-400K) | 44.0 | 42.1 | 46.5 |
Δ - VideoLLaVA | +11.5 | +6.1 | +18.6 |
VideoLLaVA (SFT on VISTA-400K + 300K VideoChat2-IT) | 47.5 | 50 | 44.2 |
Δ - VideoLLaVA (SFT on VISTA-400K) | +3.5 | +7.9 | -2.3 |
🔼 This table compares the performance of three different models on the HRVideoBench benchmark: the baseline VideoLLaVA model, VideoLLaVA fine-tuned on the VISTA-400K dataset, and VideoLLaVA fine-tuned on both VISTA-400K and an additional 300K samples from the VideoChat2-IT dataset. The results show the average performance, object recognition accuracy, and action recognition accuracy for each model on the benchmark. The ‘A’ values represent the performance differences between the fine-tuned models and the baseline model. ‘SFT’ denotes that supervised fine-tuning was used for the models.
read the caption
Table 6: Comparison between the baseline VideoLLaVA model, VideoLLaVA finetuned on VISTA-400K and VideoLLaVA finetuned on VISTA-400K + 300K VideoChat2-IT data (VISTA-VideoLLaVA in the main paper) on HRVideoBench. “SFT” indicates supervised finetuning.
Models | avg | short | medium | long |
---|---|---|---|---|
VideoLLaVA | 41.6 | 46.1 | 40.7 | 38.1 |
VISTA-VideoLLaVA | 45.1 | 50.2 | 45.7 | 39.3 |
Δ - VideoLLaVA | +3.5 | +4.1 | +5.0 | +1.2 |
Mantis-Idefics2 | 49.0 | 60.4 | 46.1 | 40.3 |
VISTA-Mantis | 50.9 | 61.8 | 48.6 | 42.3 |
Δ - Mantis-Idefics2 | +1.9 | +1.4 | +2.5 | +2.0 |
LongVA | 54.3 | 61.6 | 53.6 | 47.6 |
VISTA-LongVA | 59.3 | 70.0 | 57.6 | 50.3 |
Δ - LongVA | +5.0 | +8.4 | +4.0 | +2.7 |
🔼 This table presents a comparison of the performance of baseline video language models and their VISTA-finetuned counterparts on the Video-MME benchmark, specifically using the ‘with subtitles’ setting. It shows the average accuracy scores, as well as scores for short, medium, and long video questions, demonstrating the improvement achieved by fine-tuning on the VISTA dataset.
read the caption
Table 7: Comparison between VISTA-finetuned models and baseline models on Video-MME w/ subtitle benchmark.
Full paper#
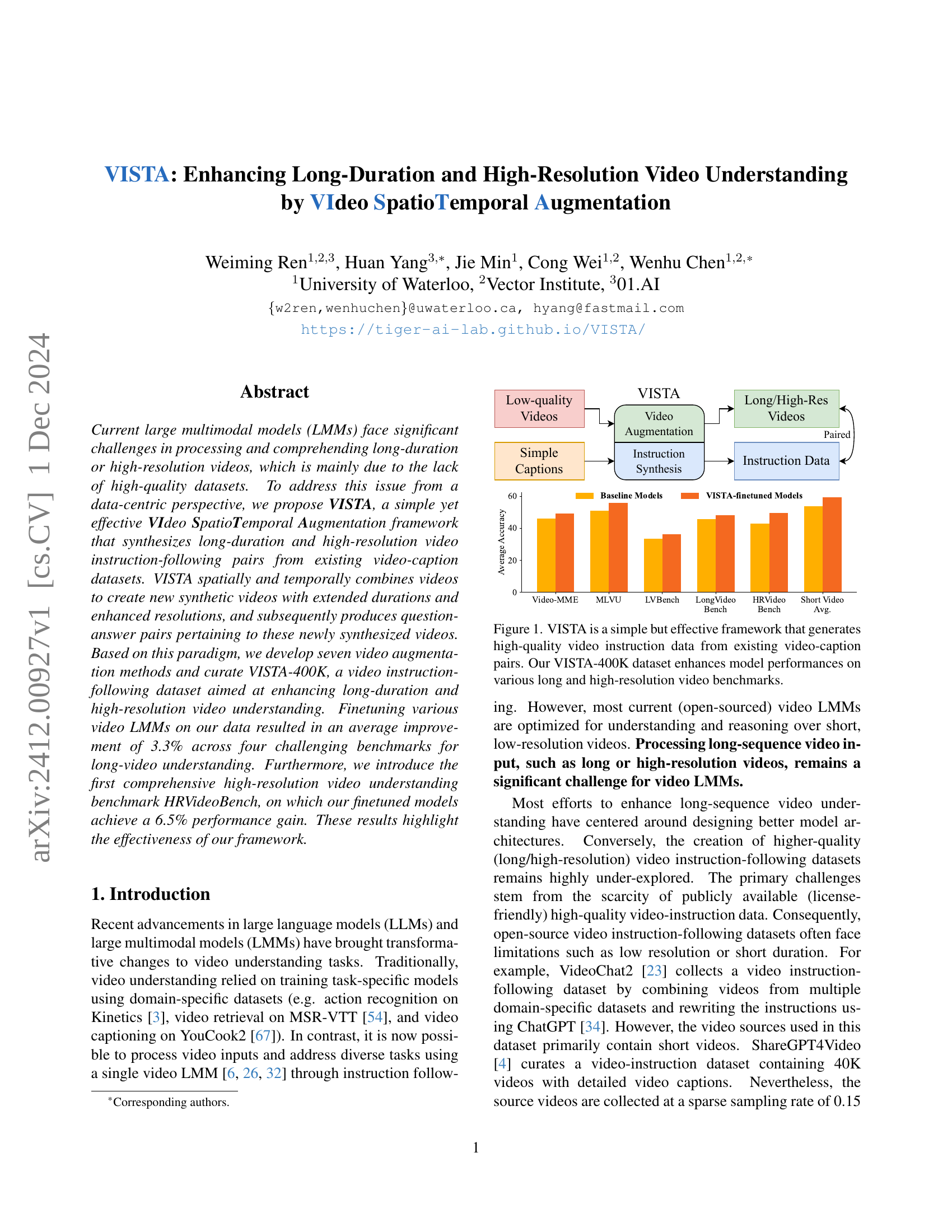
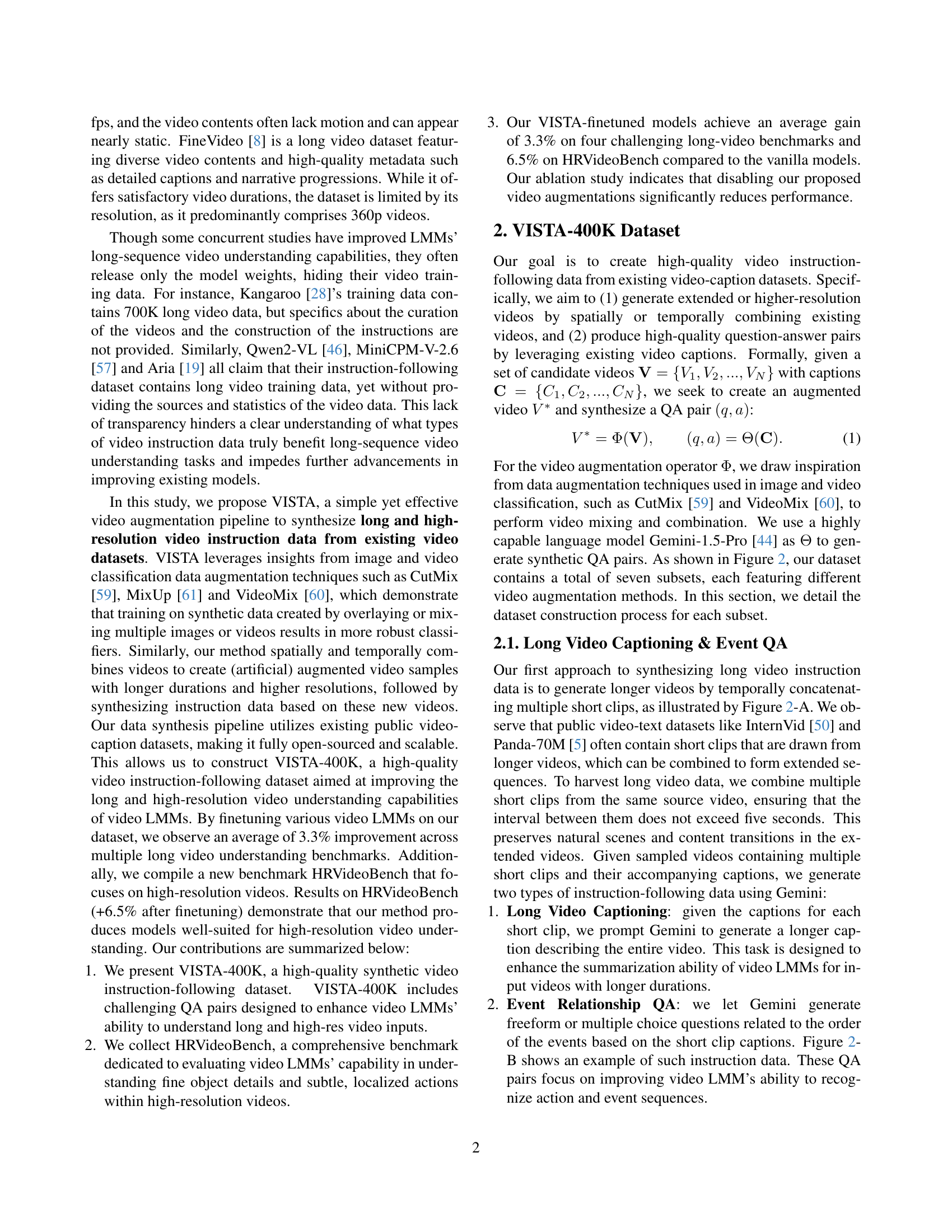
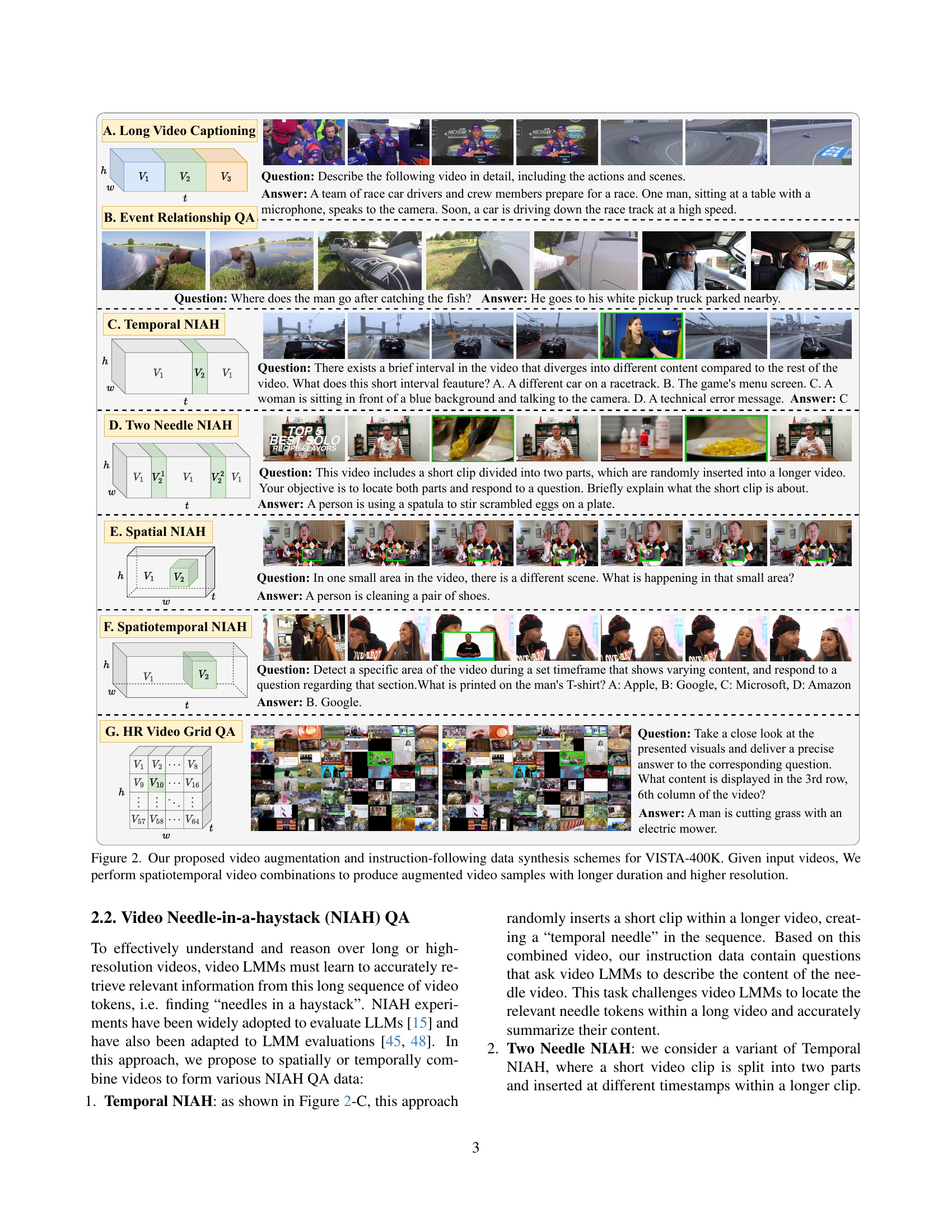
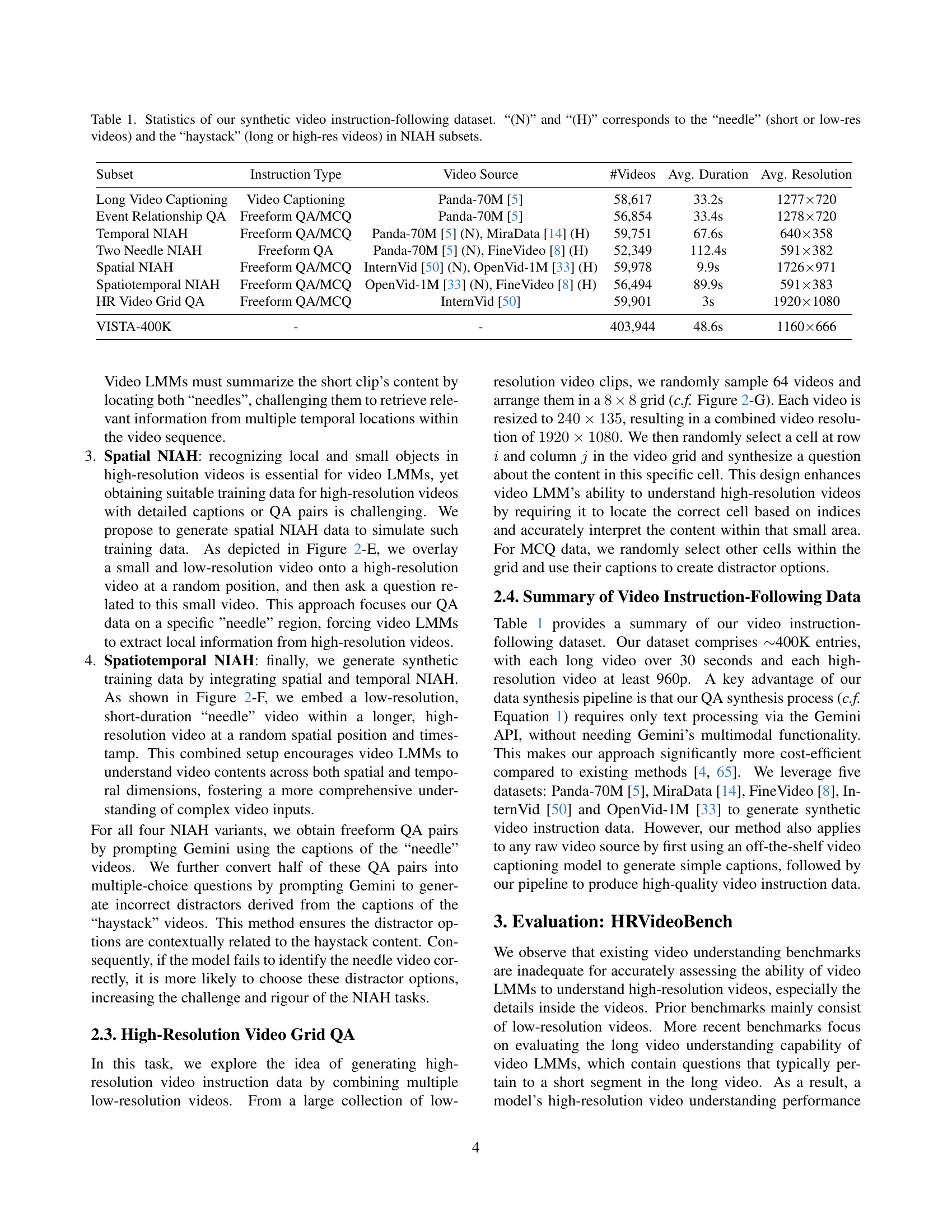
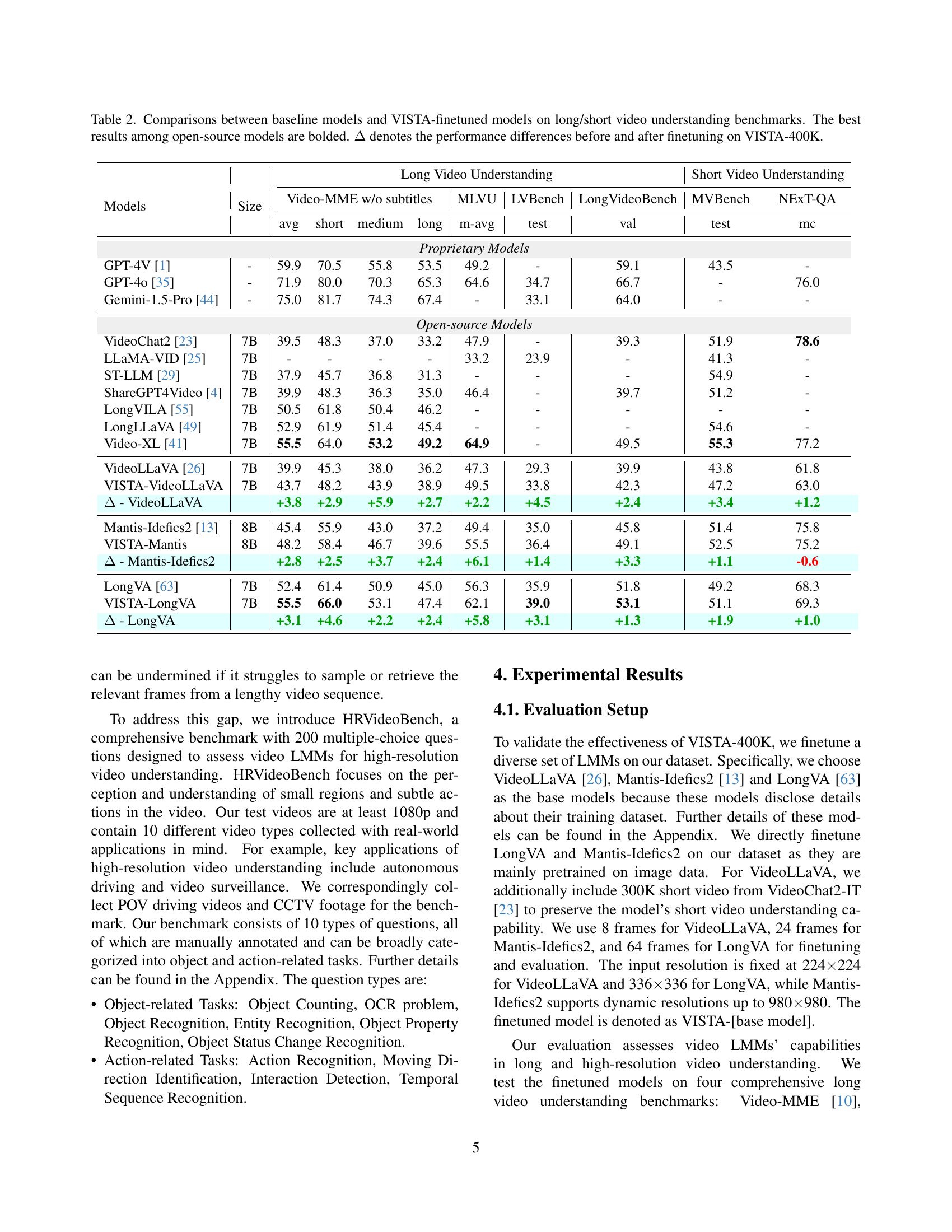
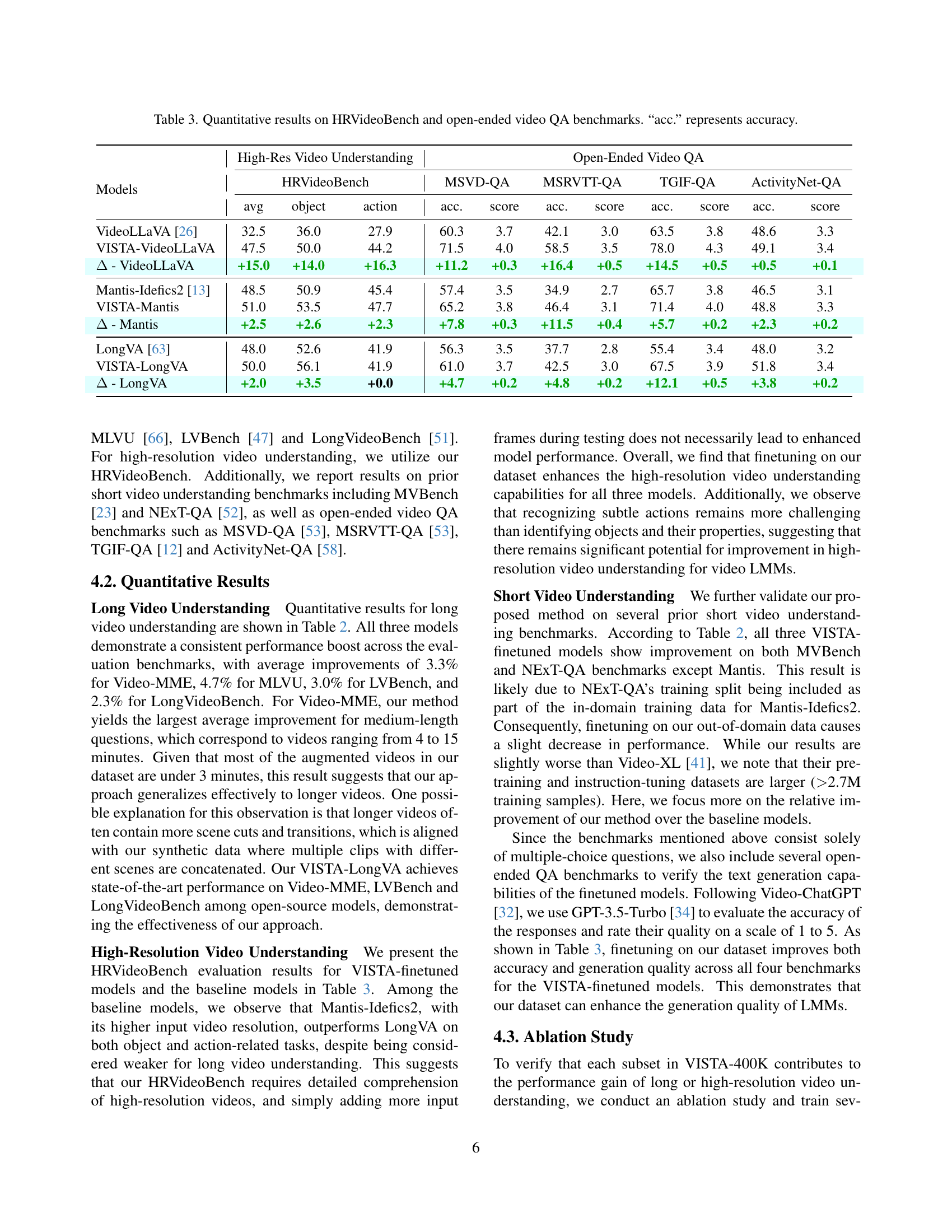
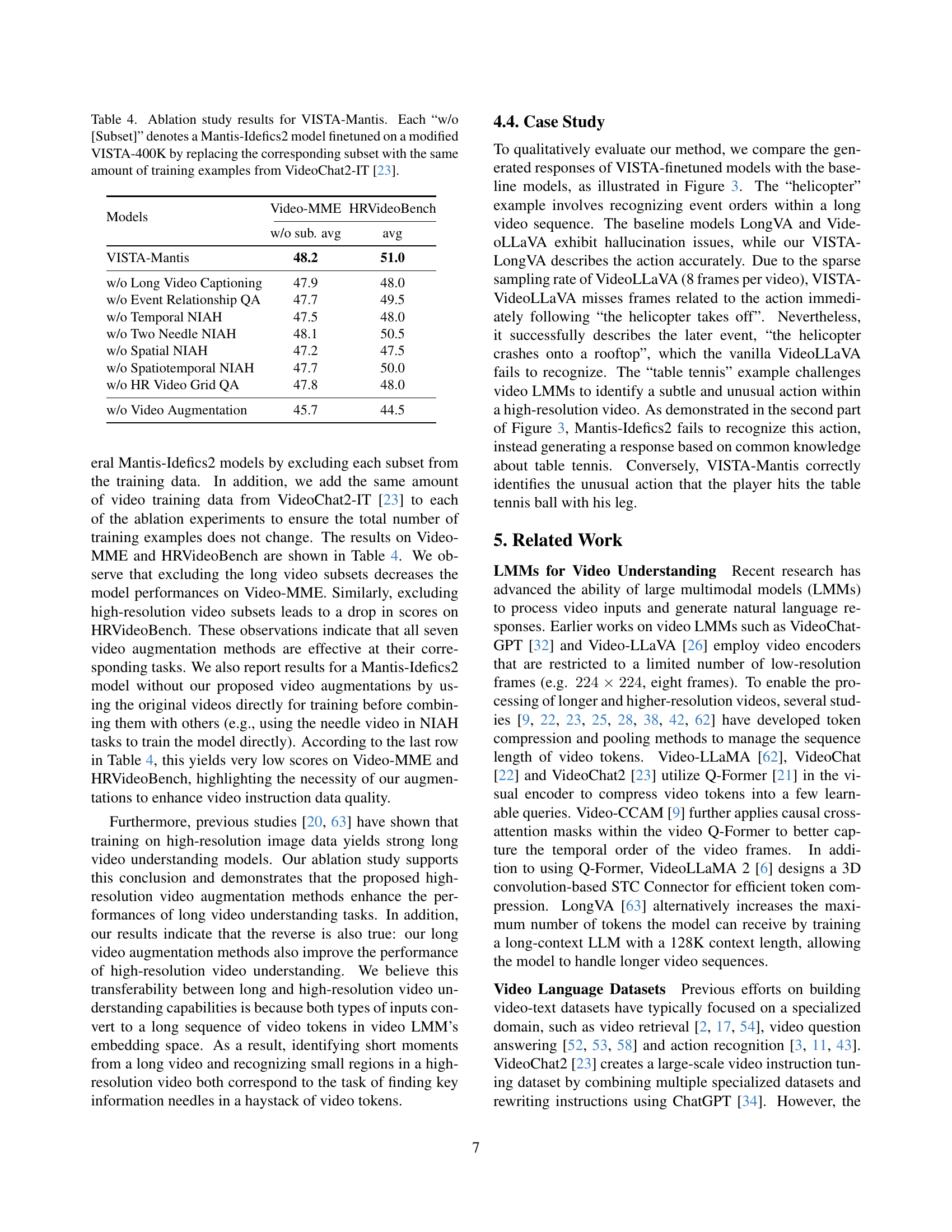
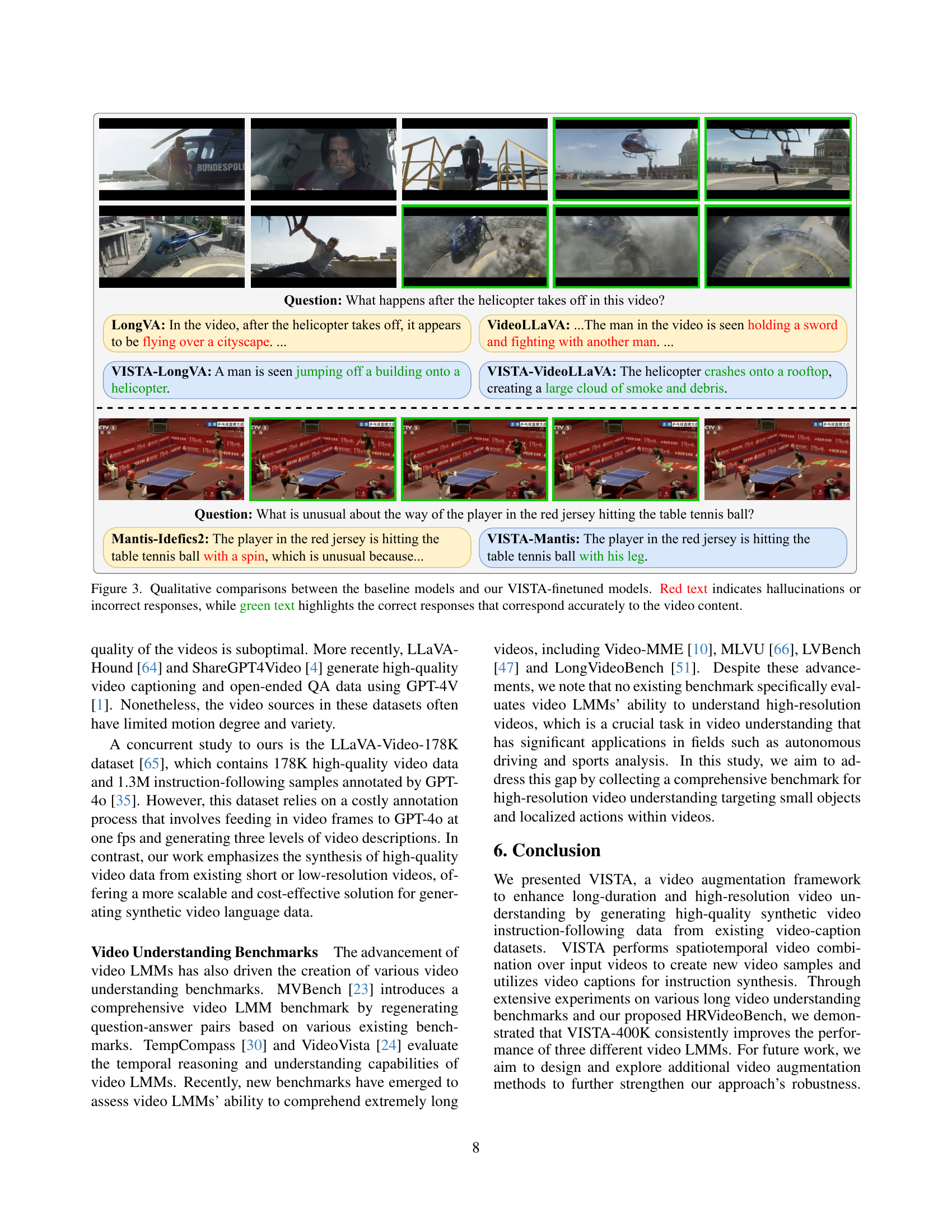
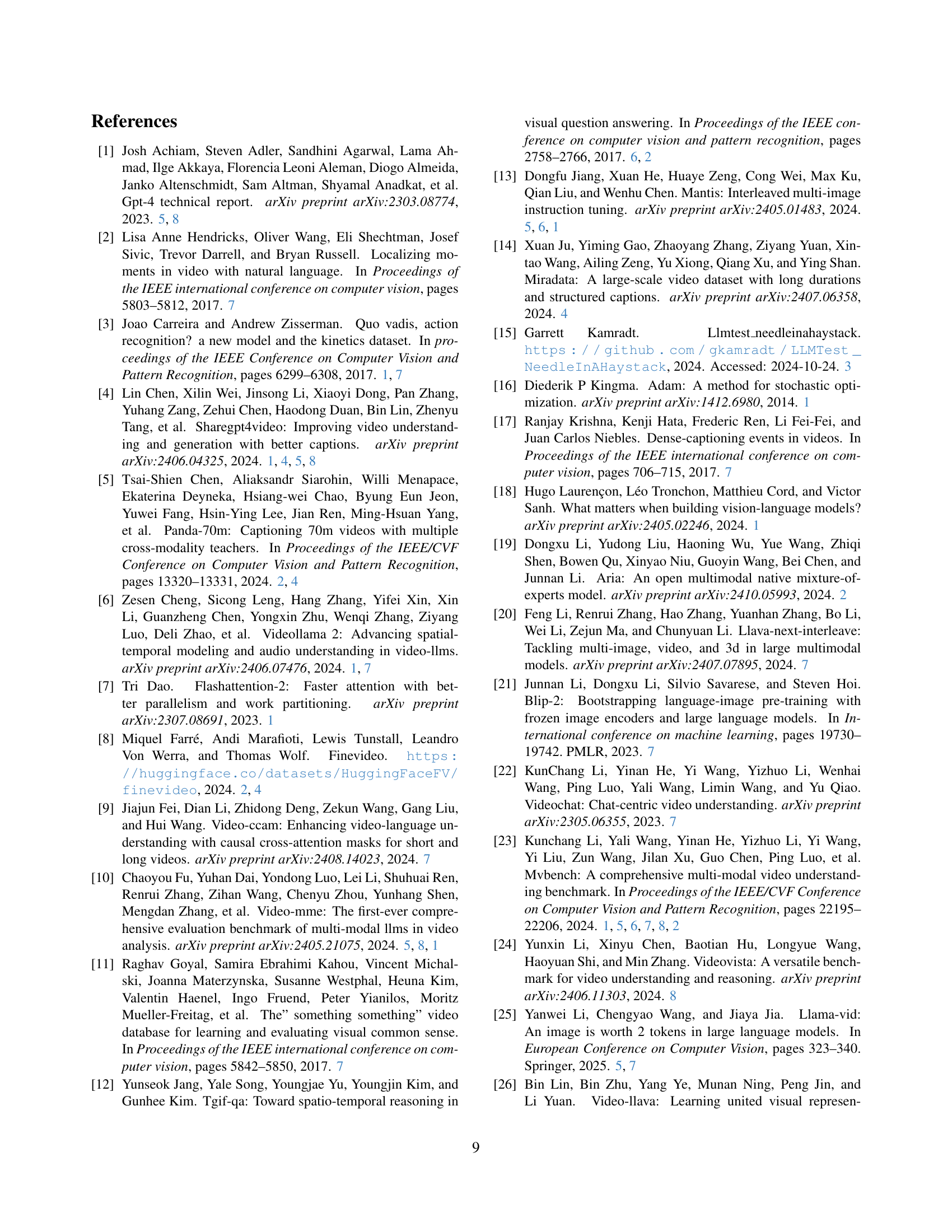
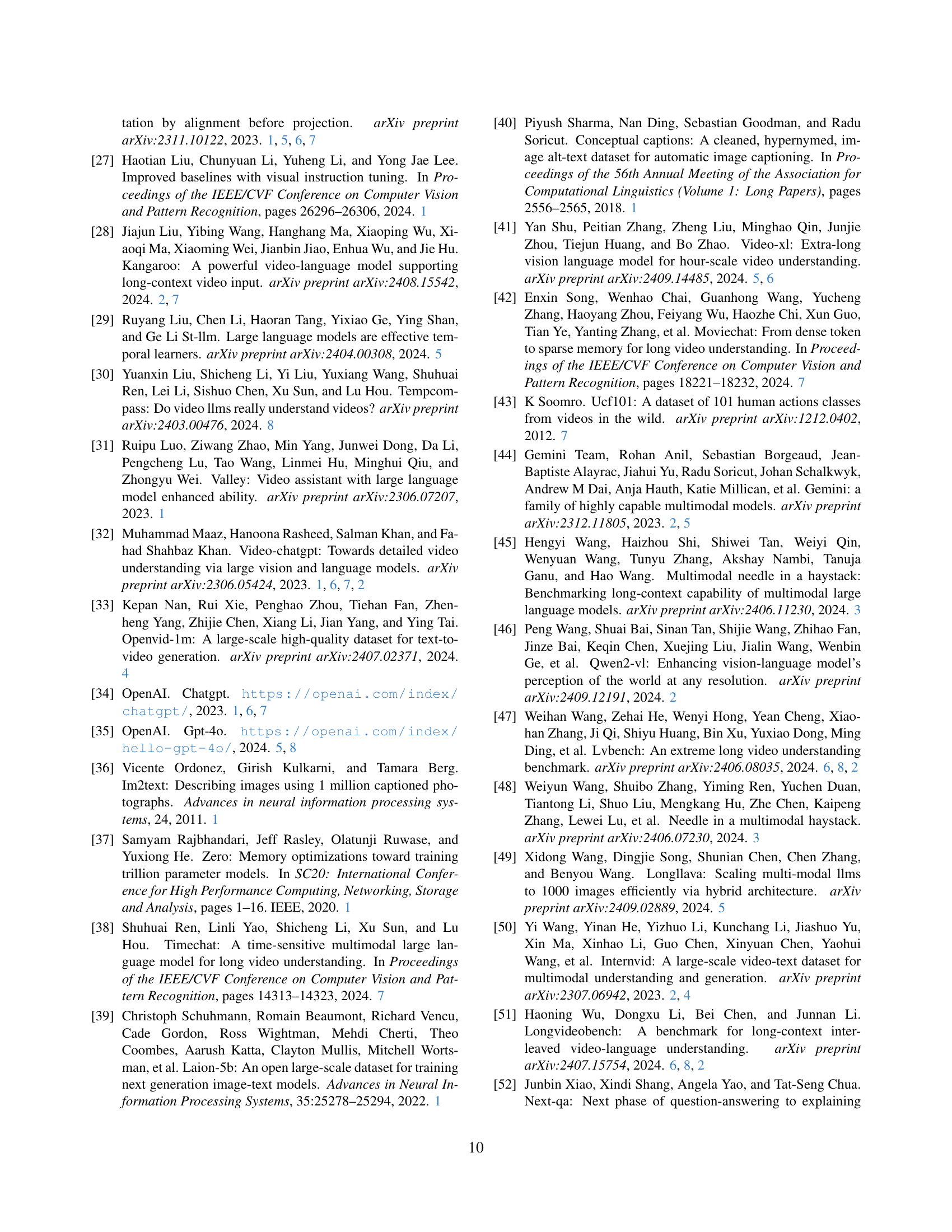
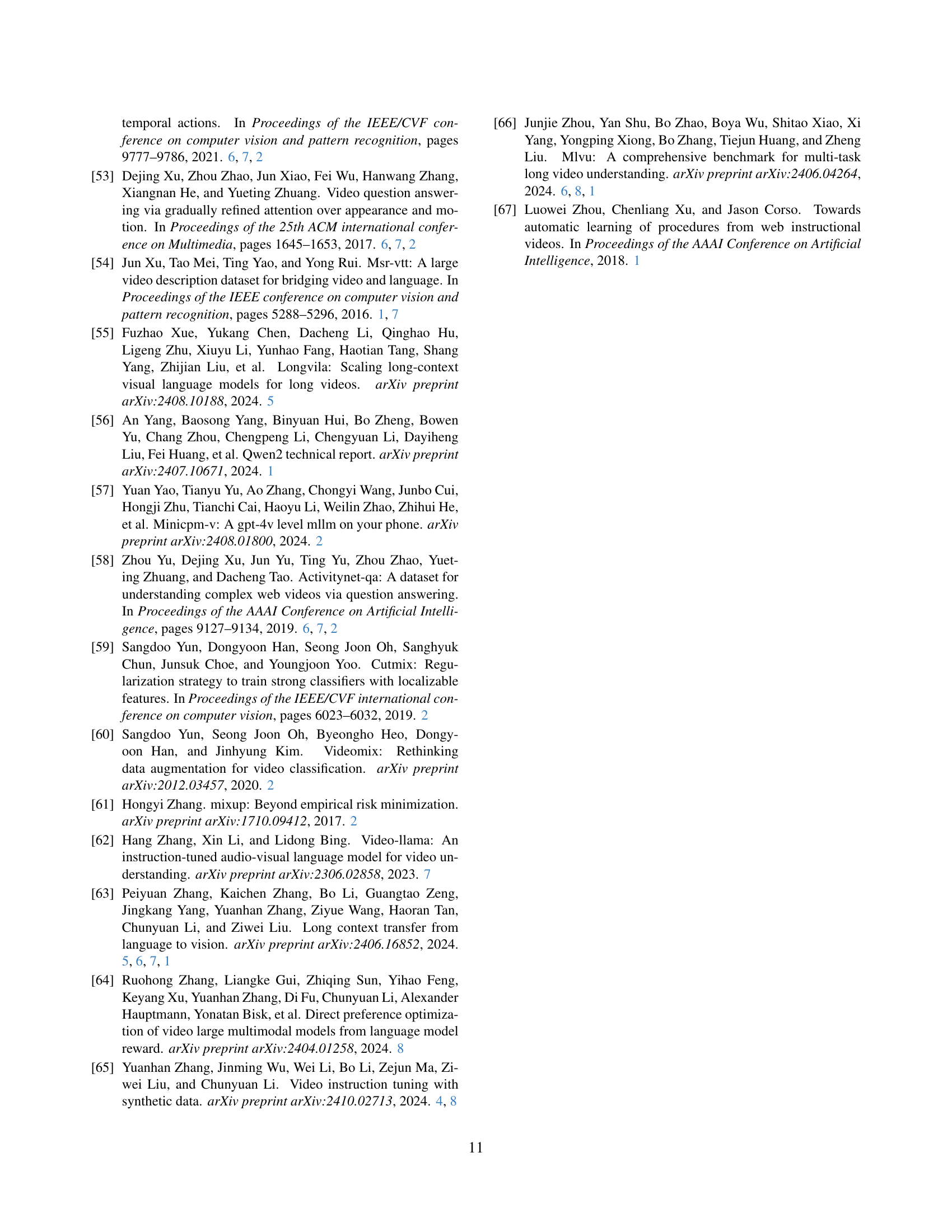
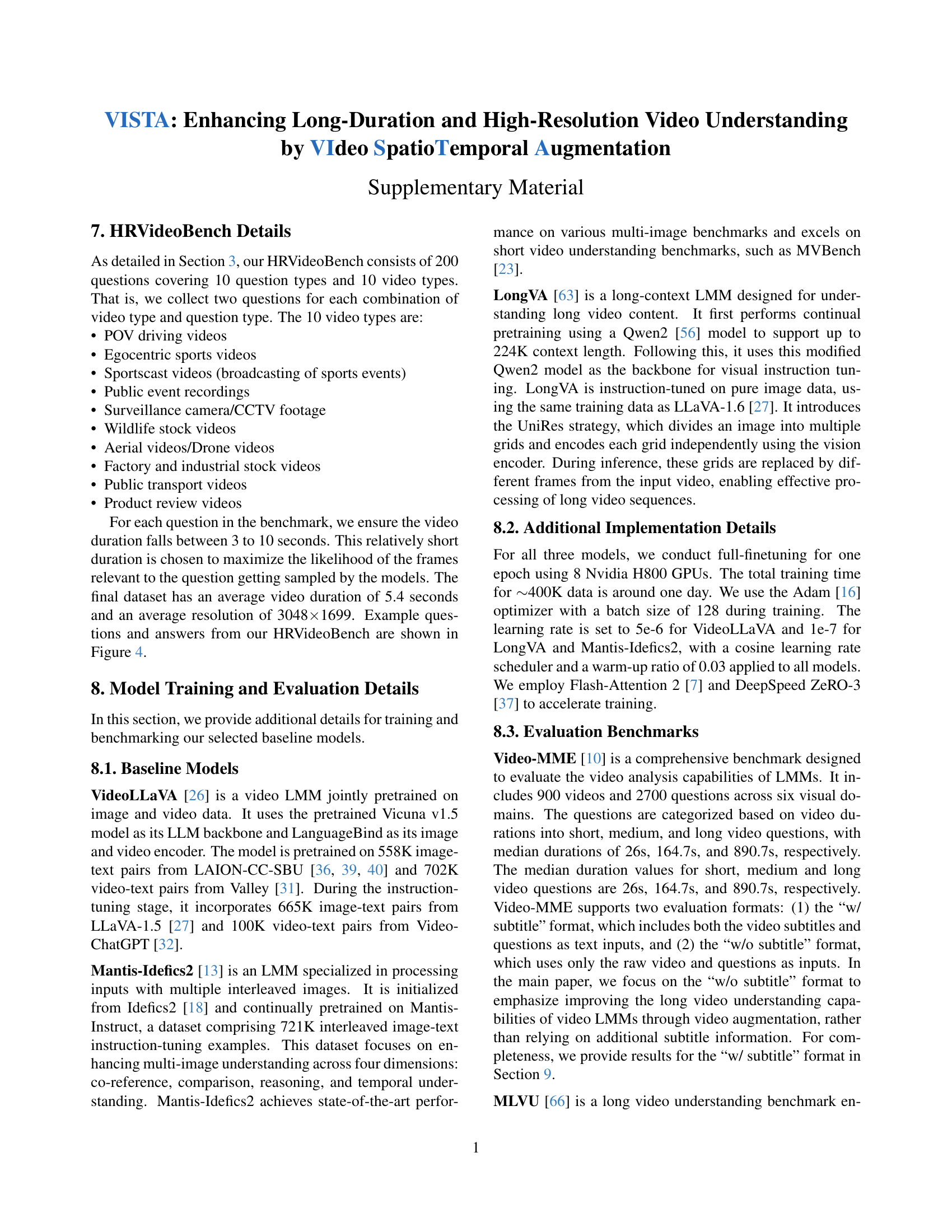
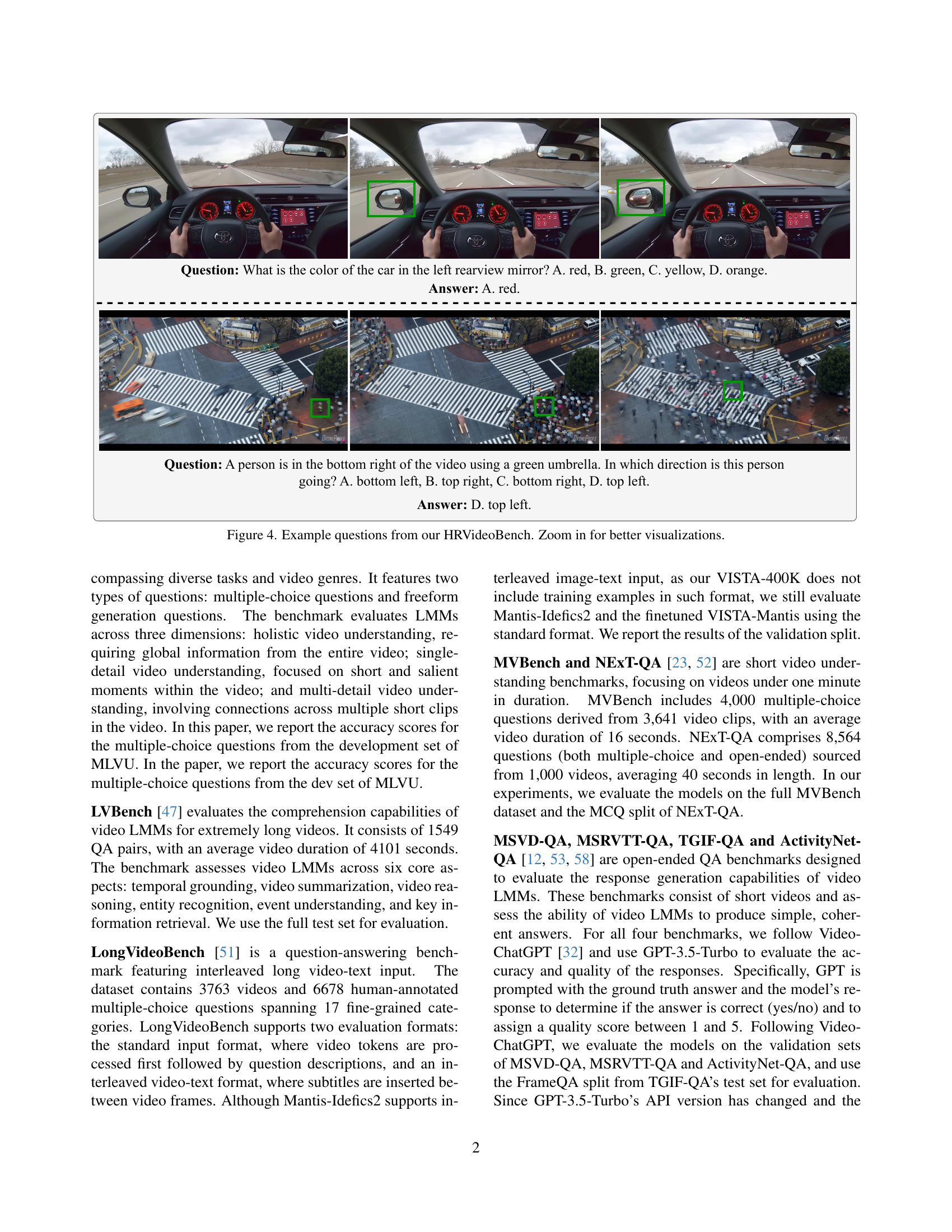
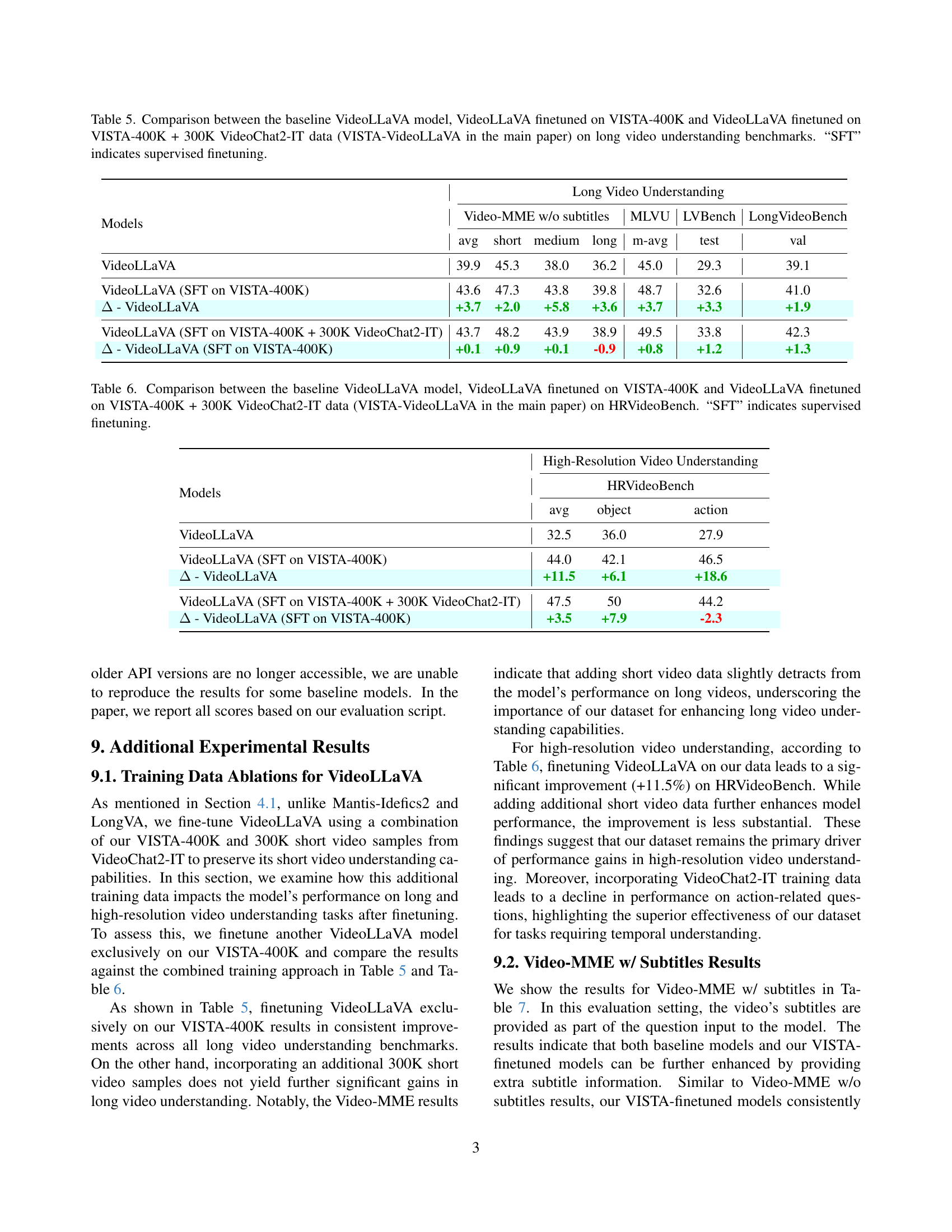
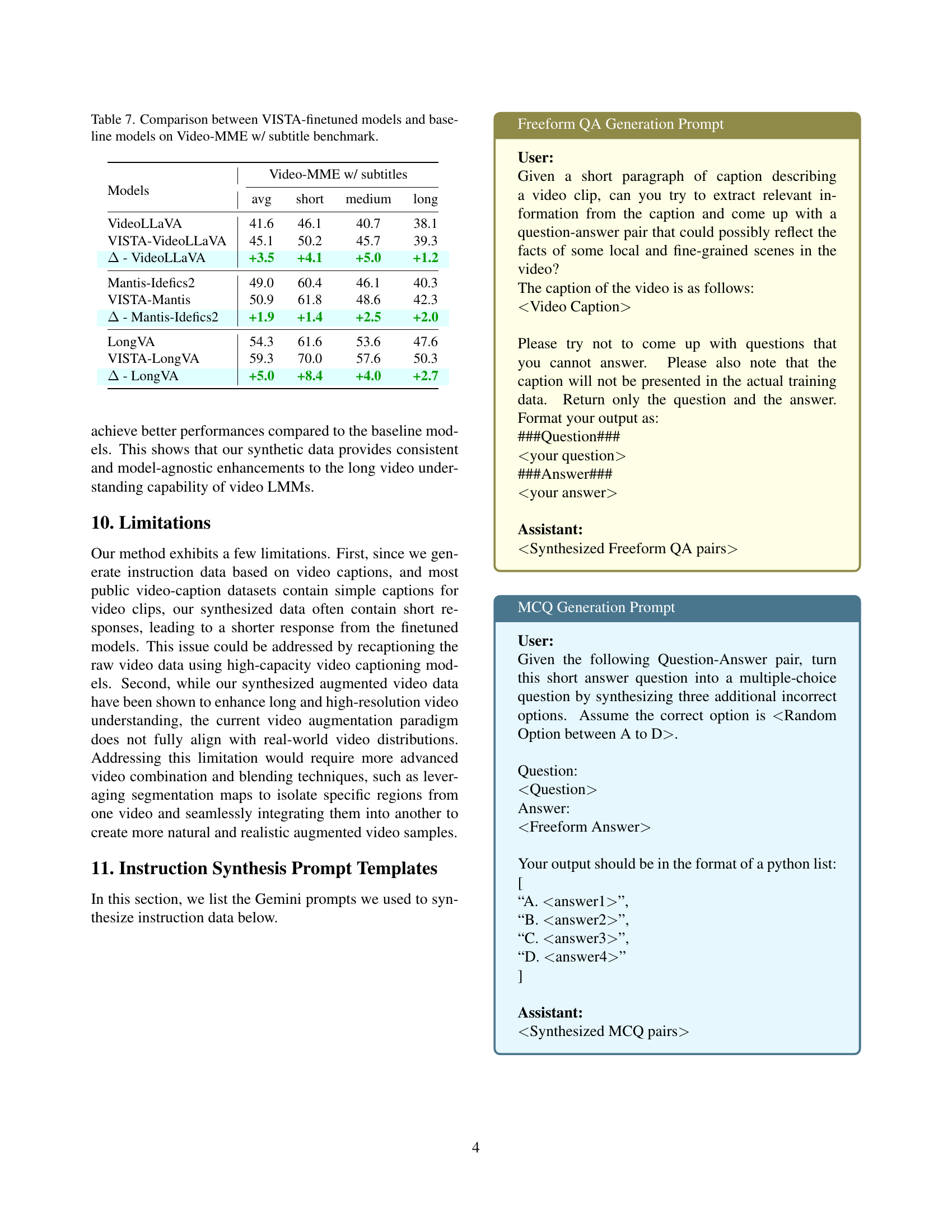
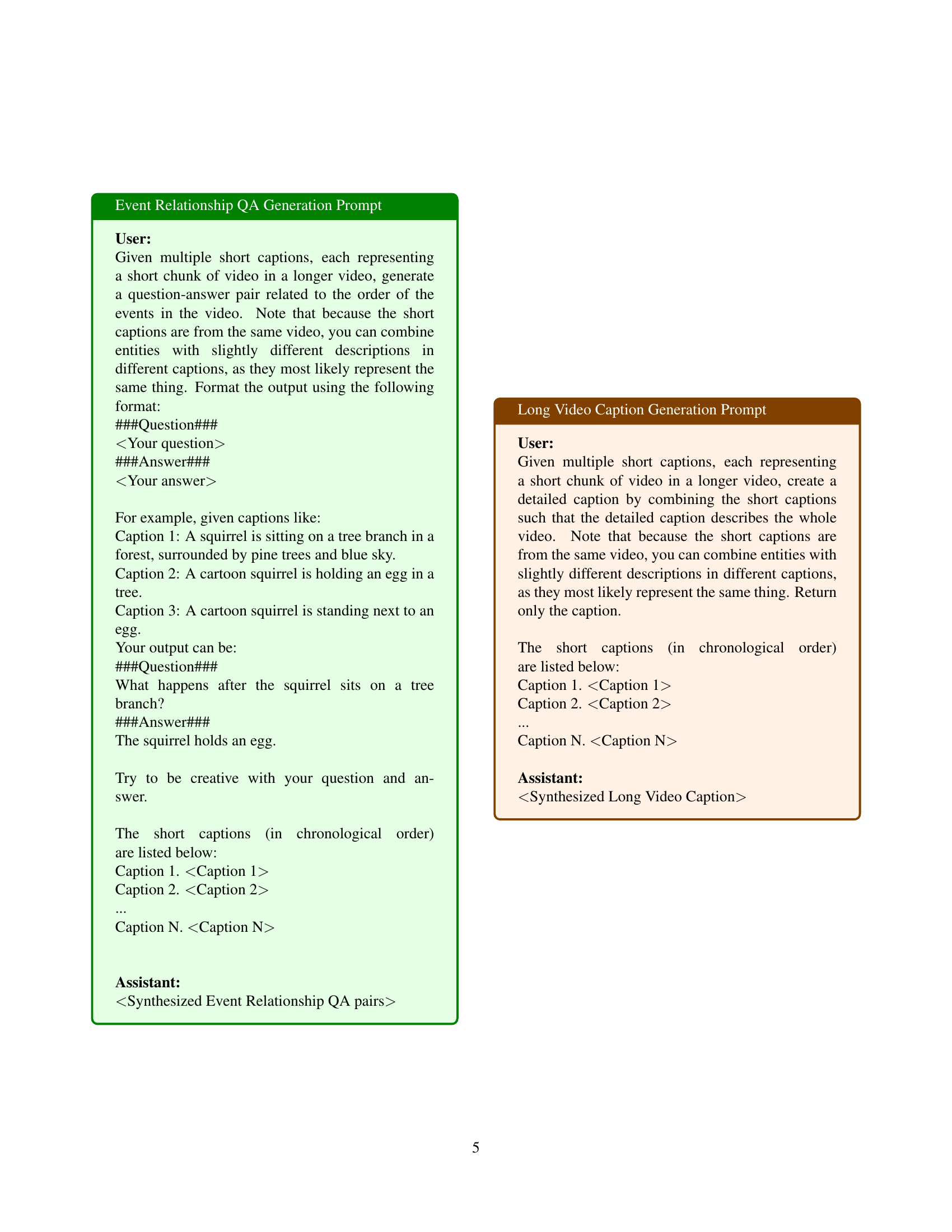