TL;DR#
Current multimodal large language models (MLLMs) struggle with a significant discrepancy between their comprehension and generation abilities. This limitation hinders the development of truly versatile and powerful AI systems capable of seamlessly integrating understanding and creative content generation. Existing methods often focus on improving one aspect while neglecting the other, resulting in an imbalance that restricts overall performance.
The research introduces HermesFlow, a novel framework designed to resolve this issue. HermesFlow employs a technique called Pair-DPO (Direct Preference Optimization) which leverages paired preference data to simultaneously improve both understanding and generation capabilities. By using a self-play optimization strategy and iteratively refining the model’s understanding and generation preference, HermesFlow achieves significant improvement in closing the gap, outperforming existing state-of-the-art models in various benchmarks.
Key Takeaways#
Why does it matter?#
This paper is significant because it identifies and addresses a critical gap in the capabilities of current multimodal large language models (MLLMs). By proposing a novel framework, it offers practical solutions for improving the alignment between understanding and generation tasks, opening up new avenues for research and development in the field. The findings are highly relevant to the current focus on unified multimodal models and have implications for building more versatile and robust AI systems.
Visual Insights#
Model | # Params | POPE | MME | Flickr30k | VQAv2 | GQA | MMMU |
---|---|---|---|---|---|---|---|
Gemini-Nano-1 (Team et al., 2023) | 1.8B | - | - | - | 62.7 | - | 26.3 |
CoDI (Tang et al., 2024) | - | - | - | 12.8 | - | - | - |
Emu (Sun et al., 2024c) | 13B | - | - | 77.4 | 57.2 | - | - |
NExT-GPT (Wu et al., 2023) | 13B | - | - | 84.5 | 66.7 | - | - |
SEED-X (Ge et al., 2024) | 17B | 84.2 | 1435.7 | 52.3 | - | 47.9 | 35.6 |
DreamLLM (Dong et al., 2023) | 7B | - | - | - | 72.9 | - | - |
Chameleon (Team, 2024) | 34B | - | - | 74.7 | 66.0 | - | - |
Show-o (Xie et al., 2024a) | 1.3B | 80.0 | 1232.9 | 67.6 | 74.7 | 61.0 | 27.4 |
HermesFlow (Ours) | 1.3B | 81.4 | 1249.7 | 69.2 | 75.3 | 61.7 | 28.3 |
🔼 Table 1 presents a comparison of various multimodal large language models (MLLMs) on several benchmark tasks designed to evaluate multimodal understanding capabilities. The results show the performance of each model across different metrics, including the number of parameters used. The baseline data for comparison is taken from the Show-o model, as referenced in Xie et al. (2024a). This table allows readers to assess the relative strengths and weaknesses of different MLLMs in terms of their ability to understand multimodal inputs, highlighting the state-of-the-art performance in the field.
read the caption
Table 1: Evaluation on multimodal understanding benchmarks. The baseline data is quoted from Show-o (Xie et al., 2024a).
In-depth insights#
Multimodal Gap#
The concept of a “Multimodal Gap” in the context of large language models (LLMs) highlights a critical performance discrepancy between an LLM’s understanding and generation capabilities in multimodal contexts. The paper likely explores this gap by analyzing how these models perform differently on tasks requiring visual understanding (e.g., image captioning, visual question answering) versus visual generation (e.g., image synthesis from text prompts). This disparity suggests that current LLMs are more proficient at interpreting and analyzing multimodal inputs compared to actually creating novel multimodal outputs. The research probably investigates the root causes behind this gap and explores potential solutions. One key aspect might be the inherent differences in training data and methodologies used for understanding and generation tasks. Understanding often relies on large-scale datasets with labeled information, while generation relies on more complex approaches that might be more data-hungry. Ultimately, the findings will provide crucial insights into developing more balanced and powerful multimodal LLMs by addressing this gap, leading to significant advancements in various AI applications.
Pair-DPO Training#
The proposed Pair-DPO (Pairwise Direct Preference Optimization) training method is a novel approach designed to bridge the gap between multimodal understanding and generation capabilities within large language models (LLMs). Unlike traditional DPO, which focuses on optimizing either understanding or generation separately, Pair-DPO leverages homologous data (paired understanding and generation preferences) to simultaneously improve both. This simultaneous optimization aims to harmoniously align the model’s capabilities, reducing the inherent imbalance often observed where understanding surpasses generation. The effectiveness of Pair-DPO stems from its ability to learn from paired preferences, creating a more balanced and coherent training process. This technique appears particularly promising given the limitations of simply increasing training data for one capability without impacting the other. The iterative refinement within Pair-DPO further enhances this balance, progressively closing the performance gap between understanding and generation tasks, leading to a more robust and versatile multimodal LLM.
Iterative Alignment#
Iterative alignment, in the context of multimodal understanding and generation, represents a powerful strategy to progressively refine the model’s capabilities. It suggests a cyclical process where initial understanding and generation attempts are evaluated, and the feedback is used to iteratively improve both tasks. This iterative refinement is particularly valuable because understanding and generation are interdependent; improvements in one often lead to improvements in the other. The core idea is to use homologous data (data pairs with shared semantic meaning across modalities) to create a feedback loop. Through this, discrepancies between the model’s understanding and generation performance can be detected and addressed with each cycle. The key strength lies in its ability to learn from inherent model biases and data imbalances without requiring external high-quality data. This makes iterative alignment methods highly efficient and suitable for real-world applications where perfectly balanced datasets are hard to obtain. The self-play aspect often incorporated, further enhancing the learning process by leveraging the model’s own outputs as training material. This self-supervised refinement leads to a more robust and refined model, bridging the gap between understanding and generation for superior performance across various multimodal tasks.
Homologous Data#
The concept of “Homologous Data” in the context of multimodal understanding and generation is crucial. It refers to paired data points where both inputs (image and text) and outputs (captions and generated images) share a fundamental semantic similarity or relationship. This homologous nature allows for a direct comparison of understanding and generation capabilities within the same semantic space, revealing performance gaps and enabling targeted improvements. Curating homologous data involves carefully selecting paired inputs, generating multiple homologous outputs (e.g., captions, images), and filtering these outputs based on similarity metrics (like BERT similarity for captions or self-VQA scoring for images) to identify high-quality homologous pairs. This process ensures that model performance on both tasks is evaluated under comparable conditions, effectively measuring and mitigating inherent biases which would skew results if non-homologous data were used. This strategy helps to directly address the gap between understanding and generation by providing a framework for aligning the model’s capabilities across different modalities. The efficacy of the proposed approach hinges upon the quality and representativeness of the homologous data in capturing the nuances of multimodal understanding and generation tasks.
Future of MLLMs#
The future of Multimodal Large Language Models (MLLMs) is bright, but hinges on overcoming current limitations. Bridging the gap between understanding and generation capabilities is crucial; current models often excel at one while lagging in the other. Research focusing on data-efficient training and alignment techniques, such as the Pair-DPO method presented in the paper, will be key to creating more balanced and versatile models. Iterative optimization and self-improvement frameworks are promising avenues for enhancing both understanding and generative performance without needing massive datasets. Further exploration of homologous data curation and the synergy between understanding and generation tasks will be vital. The development of more effective evaluation metrics is crucial for assessing MLLM capabilities accurately. Addressing issues such as bias and ethical considerations will be paramount to ensuring responsible development and deployment. The integration of MLLMs into real-world applications will also drive future innovation, necessitating the development of robust and efficient architectures. Finally, unifying multimodal understanding and generation within a single, streamlined framework presents a significant challenge with immense potential rewards for advancing AI.
More visual insights#
More on tables
GenEval | DPG-Bench | |||||||||
Methods | #params | Single Obj. | Two Obj. | Counting | Colors | Position | Color Attri. | Overall | Average | |
Diffusion Model | ||||||||||
LDM (Rombach et al., 2022) | 1.4B | 0.92 | 0.29 | 0.23 | 0.70 | 0.02 | 0.05 | 0.37 | - | |
DALL-E 2 (Ramesh et al., 2022) | 4.2B | 0.94 | 0.66 | 0.49 | 0.77 | 0.10 | 0.19 | 0.52 | - | |
SD 1.5 (Rombach et al., 2022) | 860M | 0.94 | 0.37 | 0.27 | 0.72 | 0.05 | 0.07 | 0.40 | 63.18 | |
SD 2.1 (Rombach et al., 2022) | 865M | 0.97 | 0.50 | 0.46 | 0.80 | 0.07 | 0.14 | 0.49 | 68.09 | |
Autoregressive Model | ||||||||||
LlamaGen (Sun et al., 2024b) | 775M | 0.87 | 0.25 | 0.23 | 0.51 | 0.06 | 0.04 | 0.32 | 65.16 | |
Emu (Sun et al., 2024c) | 14B | 0.87 | 0.34 | 0.26 | 0.56 | 0.07 | 0.06 | 0.36 | - | |
Chameleon (Team, 2024) | 34B | 0.89 | 0.39 | 0.28 | 0.66 | 0.08 | 0.07 | 0.40 | - | |
LWM (Liu et al., 2024b) | 7B | 0.93 | 0.41 | 0.46 | 0.79 | 0.09 | 0.15 | 0.47 | - | |
SEED-X (Ge et al., 2024) | 17B | 0.97 | 0.58 | 0.26 | 0.80 | 0.19 | 0.14 | 0.49 | - | |
Show-o (Xie et al., 2024a) | 1.3B | 0.95 | 0.52 | 0.49 | 0.82 | 0.11 | 0.28 | 0.53 | 67.48 | |
Janus (Wu et al., 2024a) | 1.3B | 0.97 | 0.68 | 0.30 | 0.84 | 0.46 | 0.42 | 0.61 | - | |
HermesFlow (Ours) | 1.3B | 0.98 | 0.84 | 0.66 | 0.82 | 0.32 | 0.52 | 0.69 | 70.22 |
🔼 Table 2 presents a comprehensive evaluation of various models’ performance on two visual generation benchmarks: GenEval and DPG-Bench. GenEval assesses multiple aspects of image generation quality, including the handling of single and multiple objects, color accuracy, positional accuracy, and attribute representation. DPG-Bench provides an overall assessment of image generation capabilities. The table lists various models, their parameter counts, and their scores on each sub-task and the overall performance metrics of the two benchmarks. This allows for a comparison of different models across various visual generation aspects.
read the caption
Table 2: Evaluation on visual generation benchmarks: GenEval (Ghosh et al., 2024) and DPG-Bench (Hu et al., 2024).
Method | # Params | FID | CLIP-Score |
---|---|---|---|
LDM (Rombach et al., 2022) | 1.4B | 12.64 | - |
DALL·E 2 (Ramesh et al., 2022) | 6.5B | 10.39 | - |
SD 1.5 (Rombach et al., 2022) | 860M | 9.62 | 30.23 |
SD 2.1 (Rombach et al., 2022) | 865M | 8.03 | 30.87 |
LlamaGen (Sun et al., 2024b) | 775M | 9.45 | 29.12 |
Emu (Sun et al., 2024c) | 14B | 11.02 | 28.98 |
LWM (Liu et al., 2024b) | 7B | 12.68 | - |
SEED-X (Ge et al., 2024) | 17B | 14.99 | - |
Show-o (Xie et al., 2024a) | 1.3B | 9.24 | 30.63 |
HermesFlow (Ours) | 1.3B | 9.07 | 31.08 |
🔼 This table presents the results of evaluating different models on the MSCOCO dataset using two metrics: Fréchet Inception Distance (FID) and CLIP score. Lower FID scores indicate better image quality (more similar to real images), and higher CLIP scores indicate better image-text alignment. The table compares the performance of HermesFlow against several other models and shows its FID and CLIP score for zero-shot generation.
read the caption
Table 3: MSCOCO zero-shot FID and CLIP-Score.
Method | # Params | Understanding Score | Generation Score | Gap |
---|---|---|---|---|
VILA-U (Wu et al., 2024c) (Xie et al., 2024a) | 7B | 0.646 | 0.477 | 0.169 |
Janus (Wu et al., 2024a) | 1.3B | 0.599 | 0.417 | 0.182 |
Show-o (Xie et al., 2024a) | 1.3B | 0.520 | 0.433 | 0.087 |
HermesFlow (Ours) | 1.3B | 0.533 | 0.497 | 0.036 |
🔼 This table quantitatively assesses the performance gap between multimodal understanding and generation capabilities in various Multimodal Large Language Models (MLLMs). It presents the understanding and generation scores for several MLLMs, calculated using a standardized pipeline. The ‘Gap’ column shows the difference between the understanding and generation scores, highlighting the relative strengths and weaknesses of each model in these two aspects. A smaller gap indicates a more balanced performance between understanding and generation. The data in the table helps illustrate the common phenomenon observed in the paper, that understanding capabilities of MLLMs typically outperform their generation capabilities.
read the caption
Table 4: Quantitative assess of MLLM’s Understanding and Generation Gap.
Understanding Bench | Generation Bench | |||||
Methods | POPE | MME | MMMU | GenEval (Overall) | DPG-Bench (Average) | |
Show-o (Xie et al., 2024a) | 80.0 | 1232.9 | 27.4 | 0.53 | 67.48 | |
DPO (Understanding) | 80.8 | 1242.2 | 27.8 | 0.58 | 67.88 | |
DPO (Generation) | 80.5 | 1239.3 | 27.5 | 0.70 | 70.03 | |
Pair-DPO (Iter. 0) (Show-o) | 80.0 | 1232.9 | 27.4 | 0.53 | 67.48 | |
Pair-DPO (Iter. 1) | 81.1 | 1246.7 | 28.0 | 0.68 | 70.19 | |
Pair-DPO (Iter. 2) | 81.3 | 1248.3 | 28.1 | 0.69 | 70.21 | |
Pair-DPO (Iter. 3) | 81.4 | 1249.7 | 28.3 | 0.69 | 70.22 |
🔼 This table presents a comparison of the performance of three different optimization methods: standard DPO focusing solely on understanding, standard DPO focusing solely on generation, and the proposed Pair-DPO method. It shows the impact of applying Pair-DPO iteratively, demonstrating performance improvements across multiple multimodal understanding and generation benchmarks (POPE, MME, MMMU, GenEval, and DPG-Bench) with each iteration.
read the caption
Table 5: Comparison of Pair-DPO vs. DPO and the Effect of Pair-DPO Iterations.
Full paper#
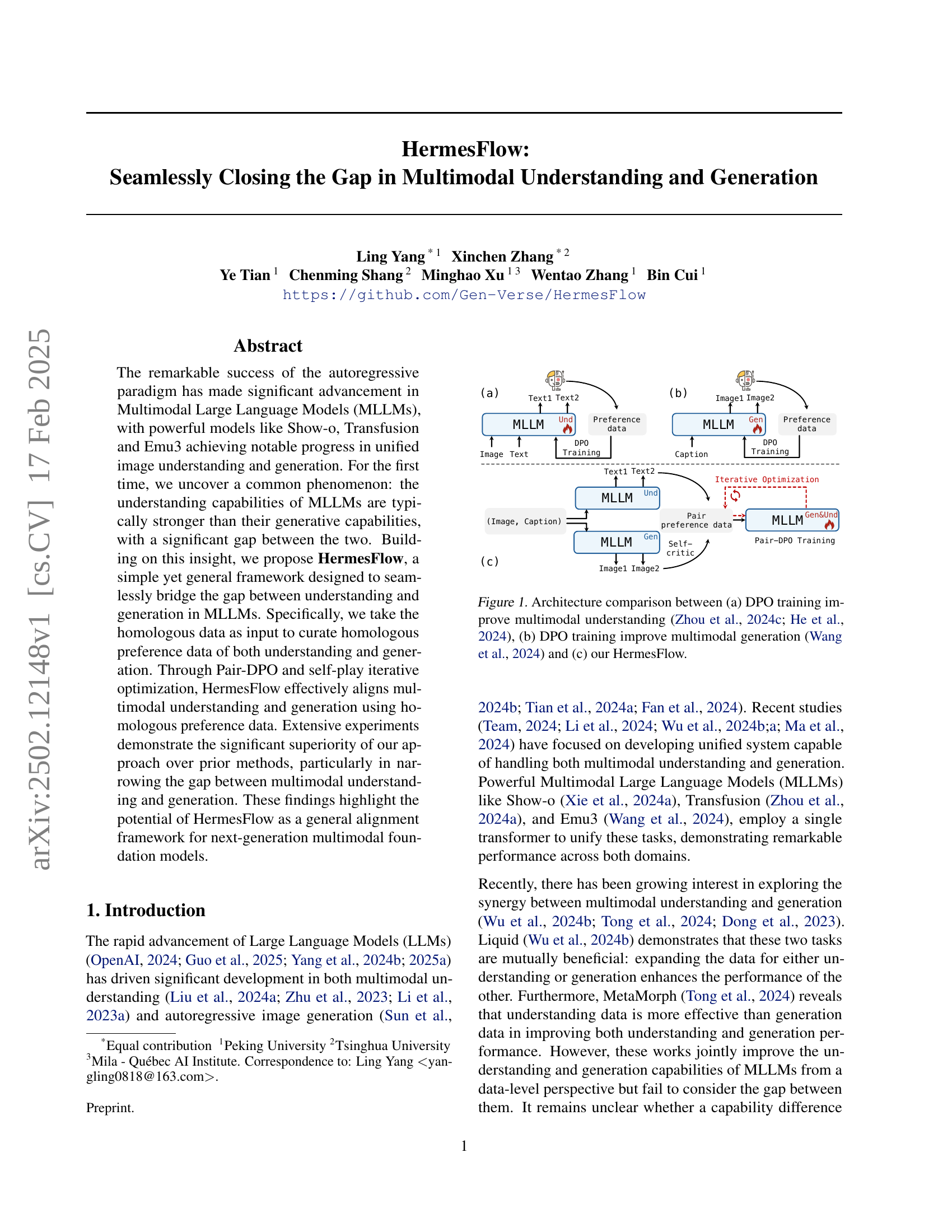
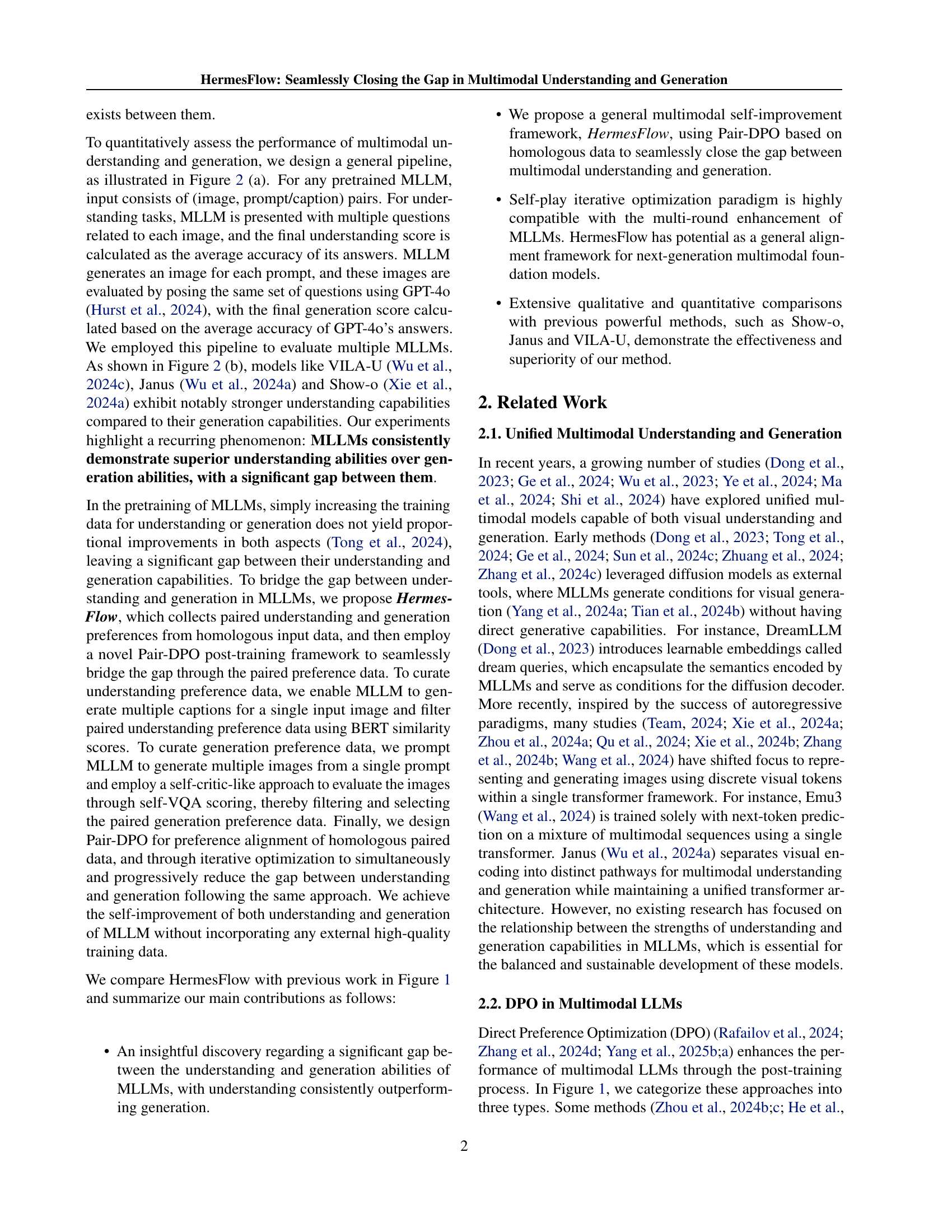
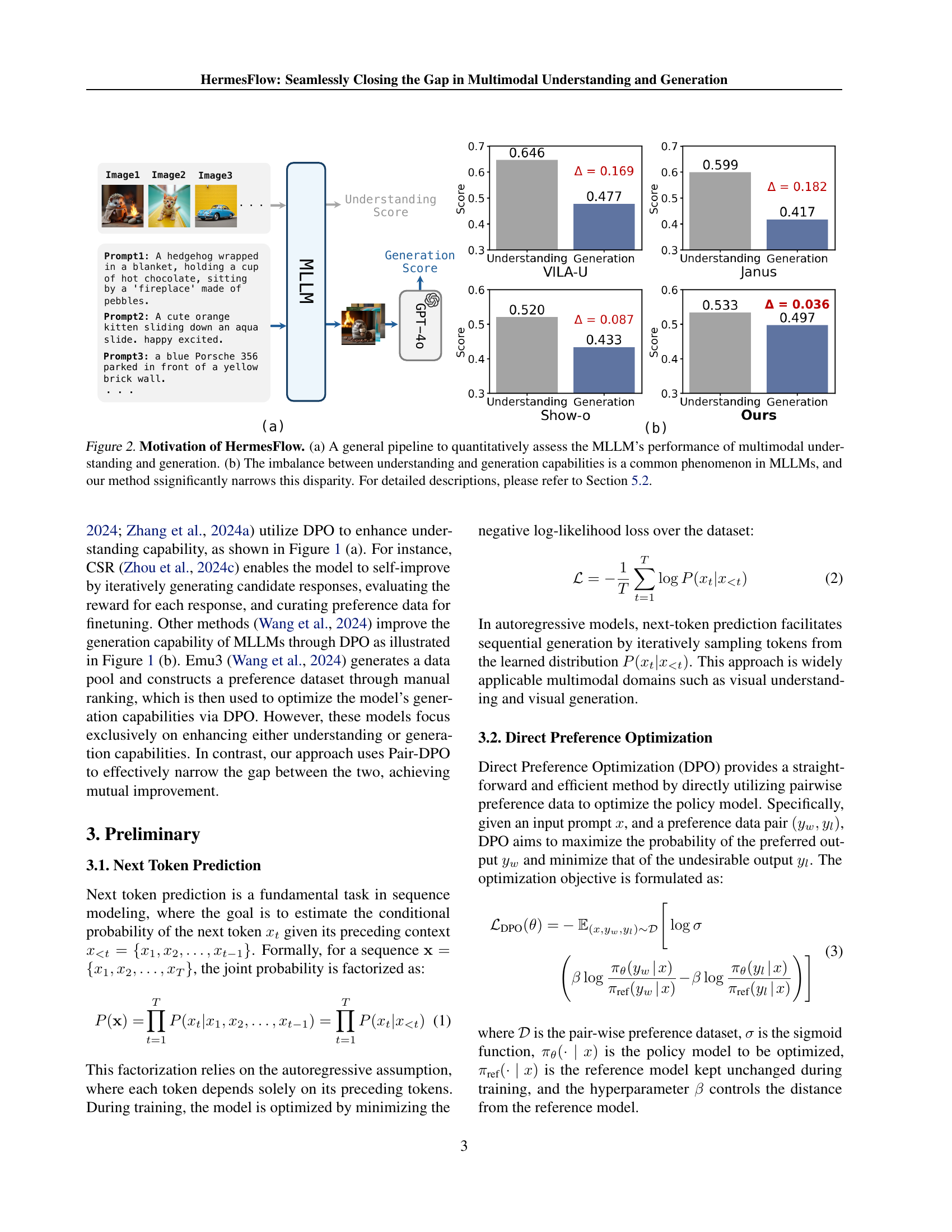
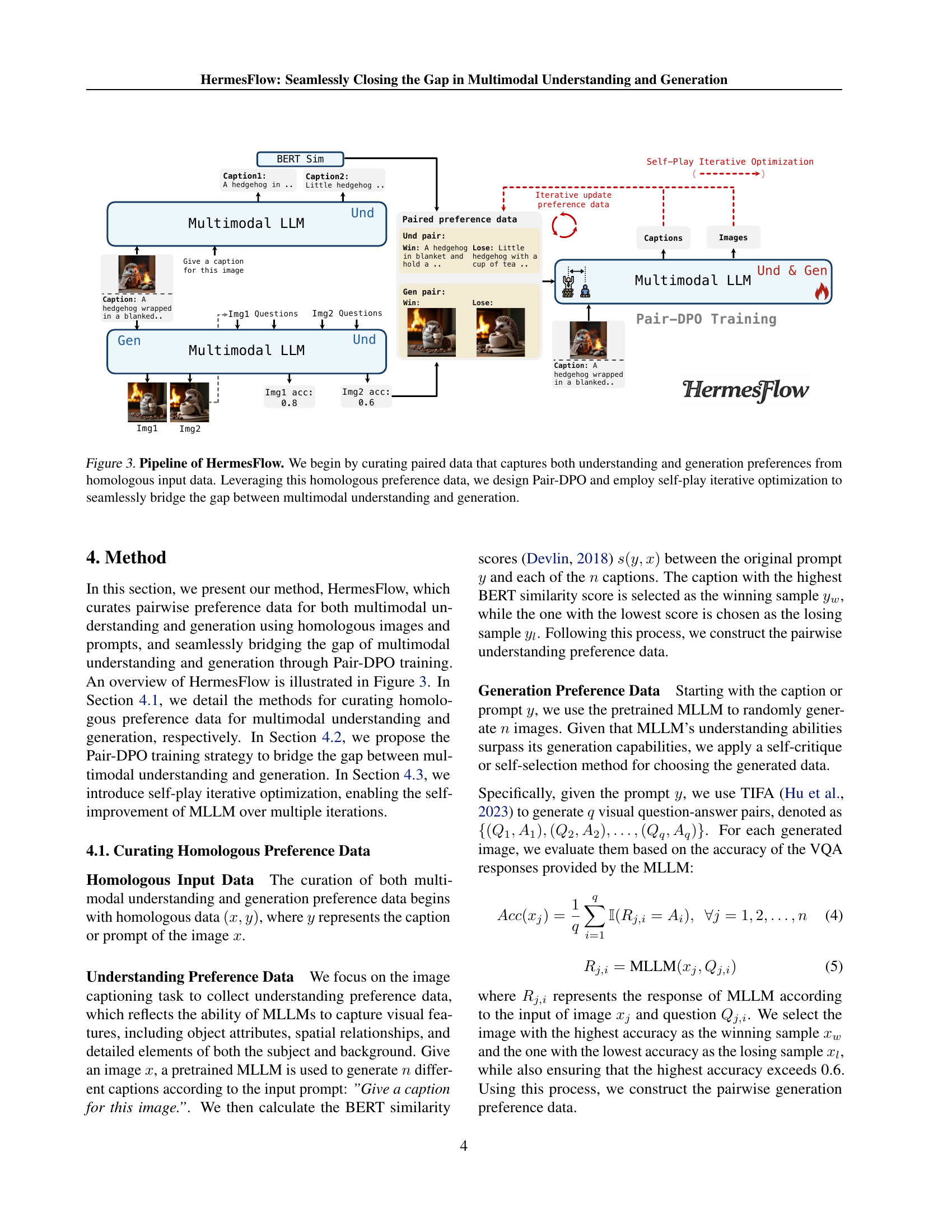
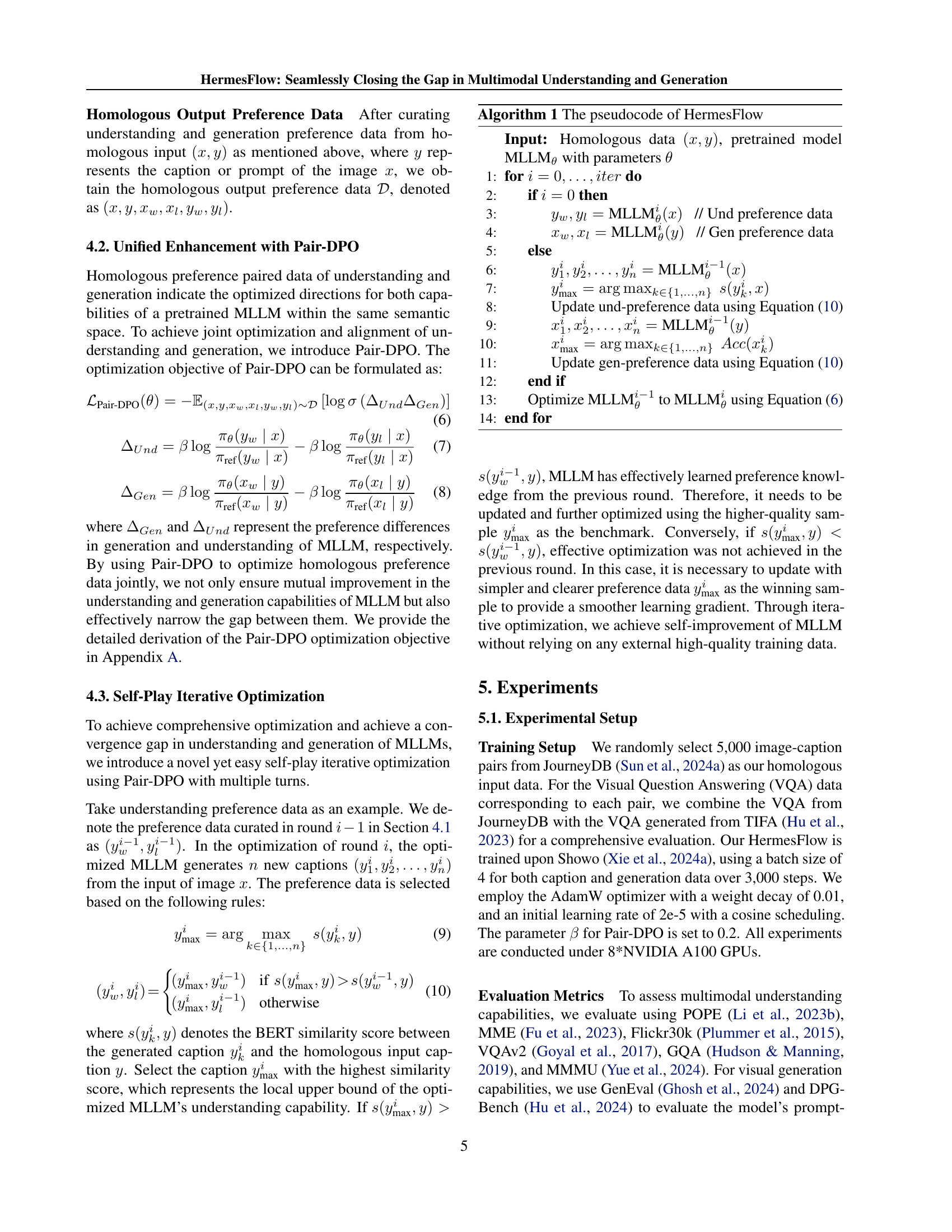
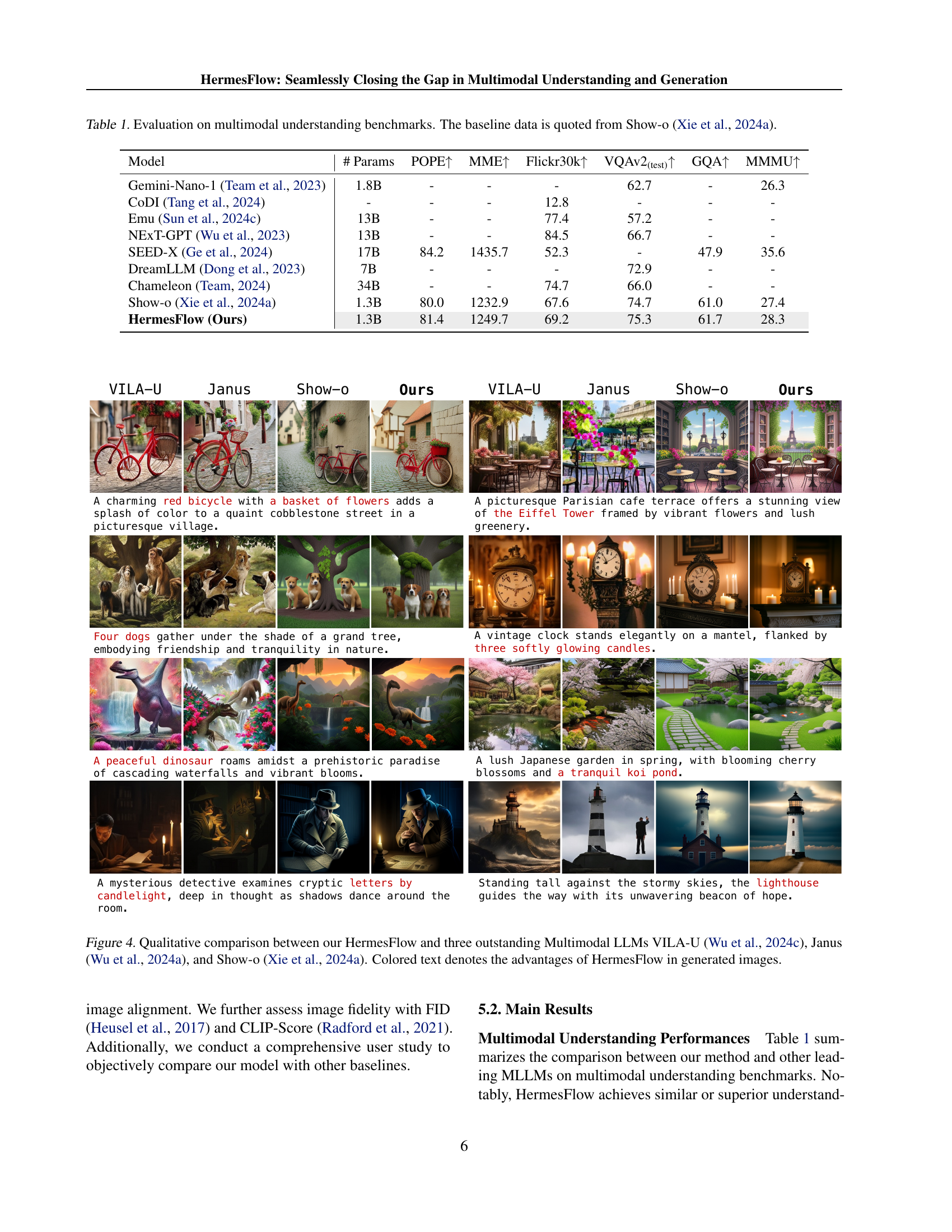
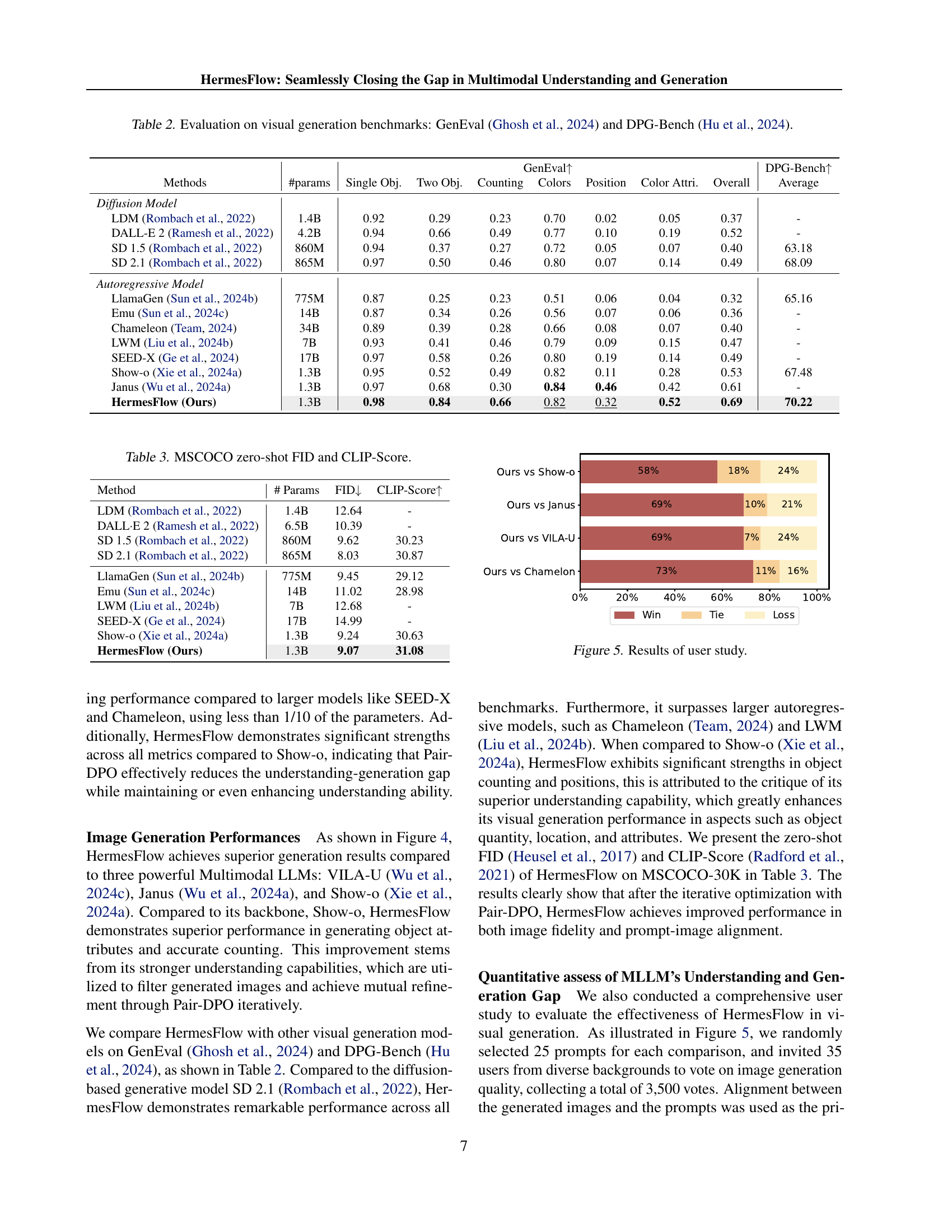
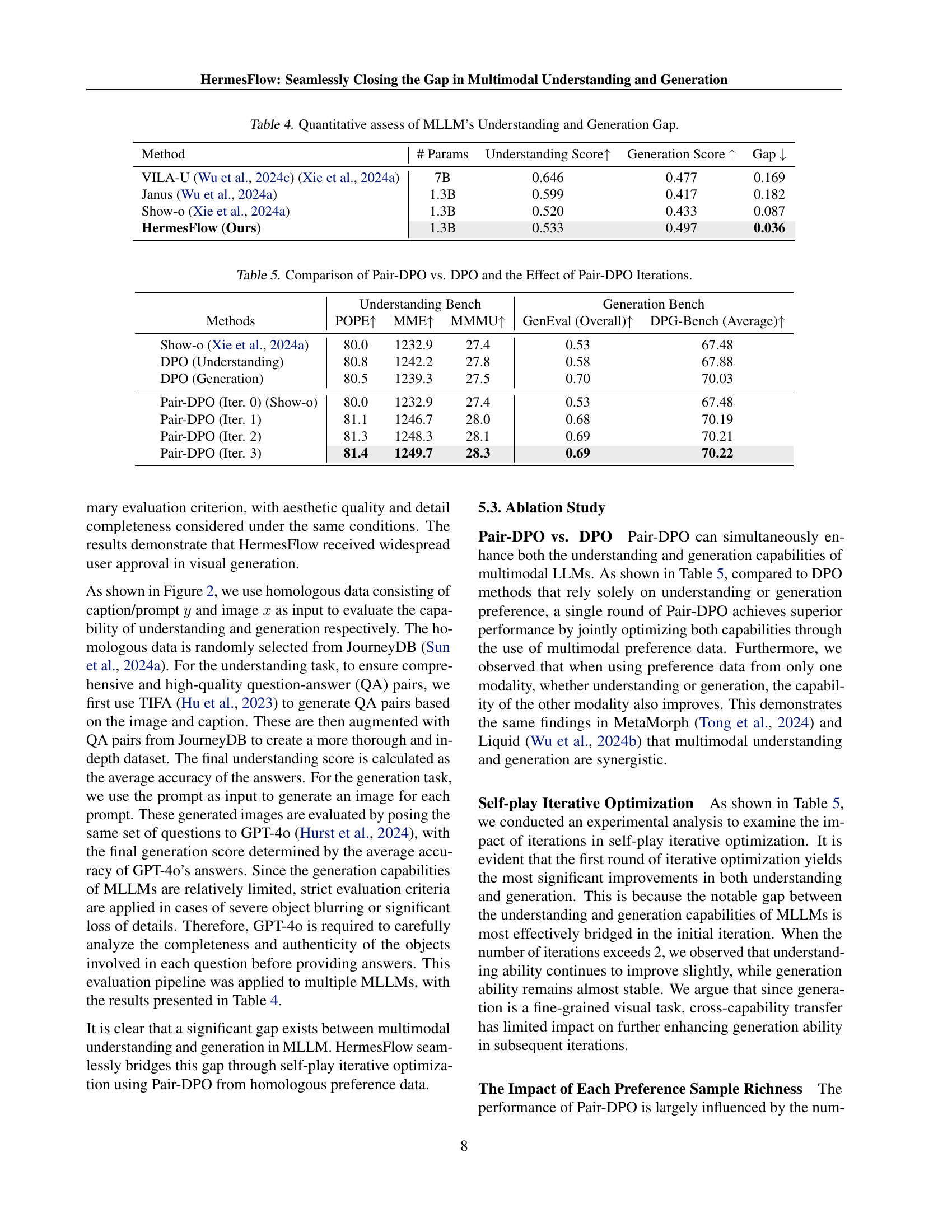
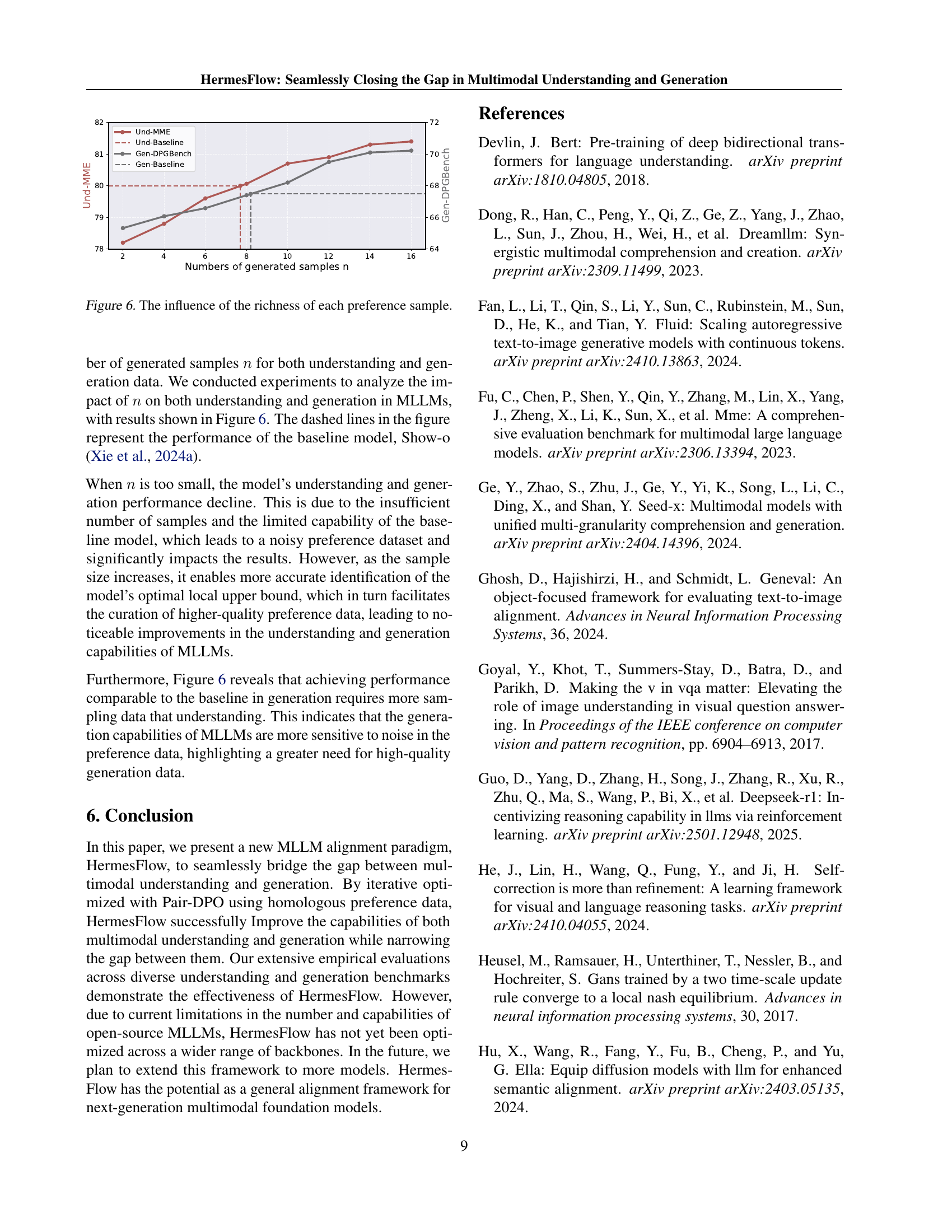
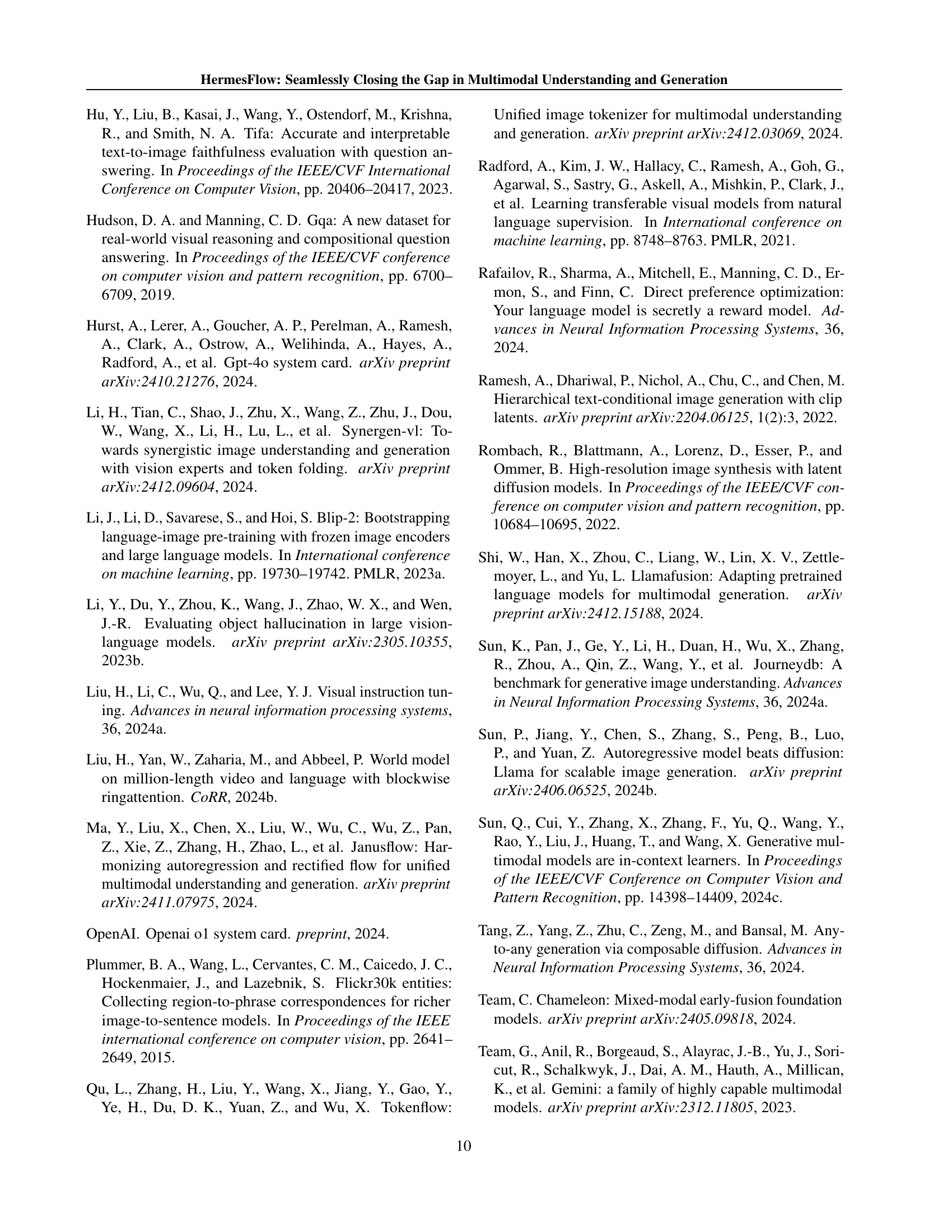
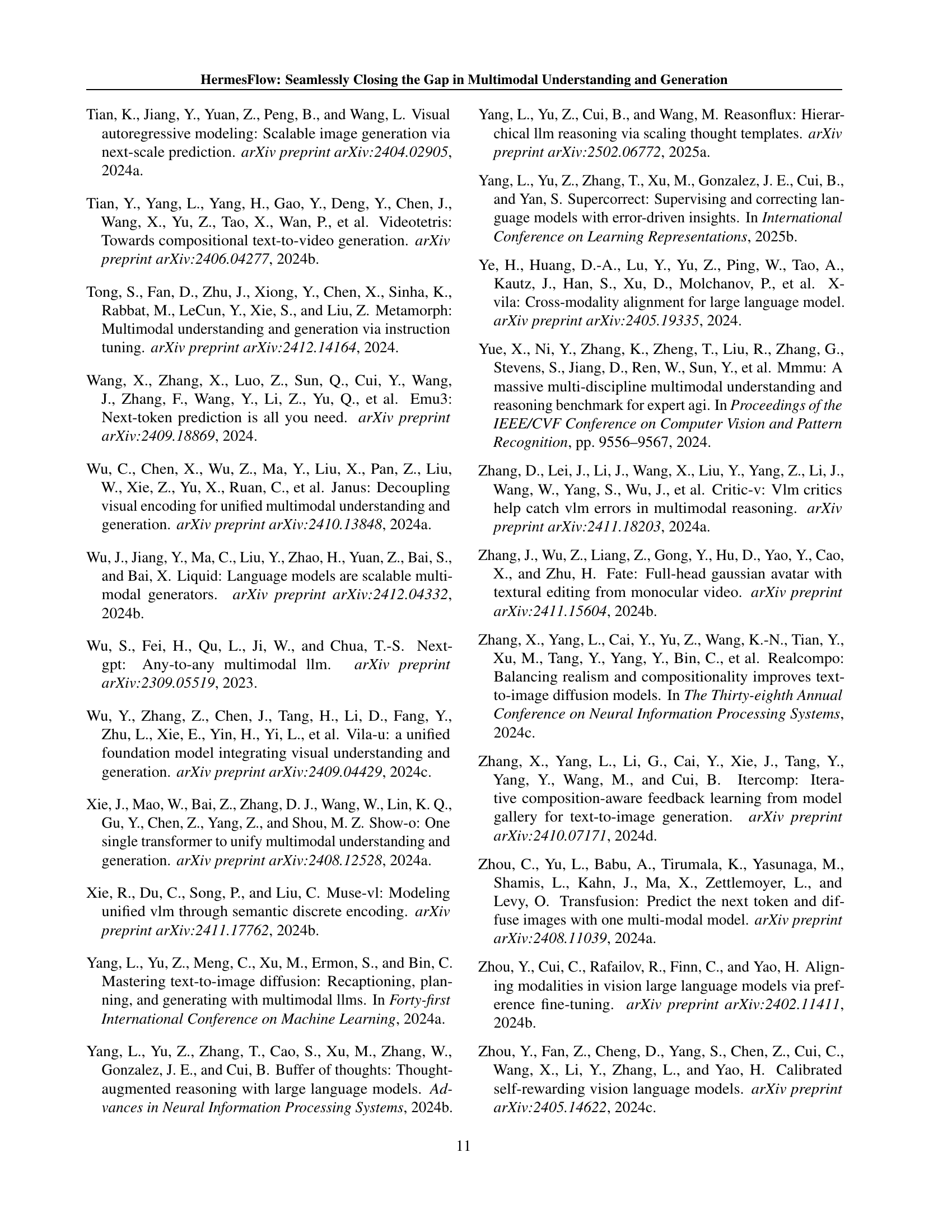
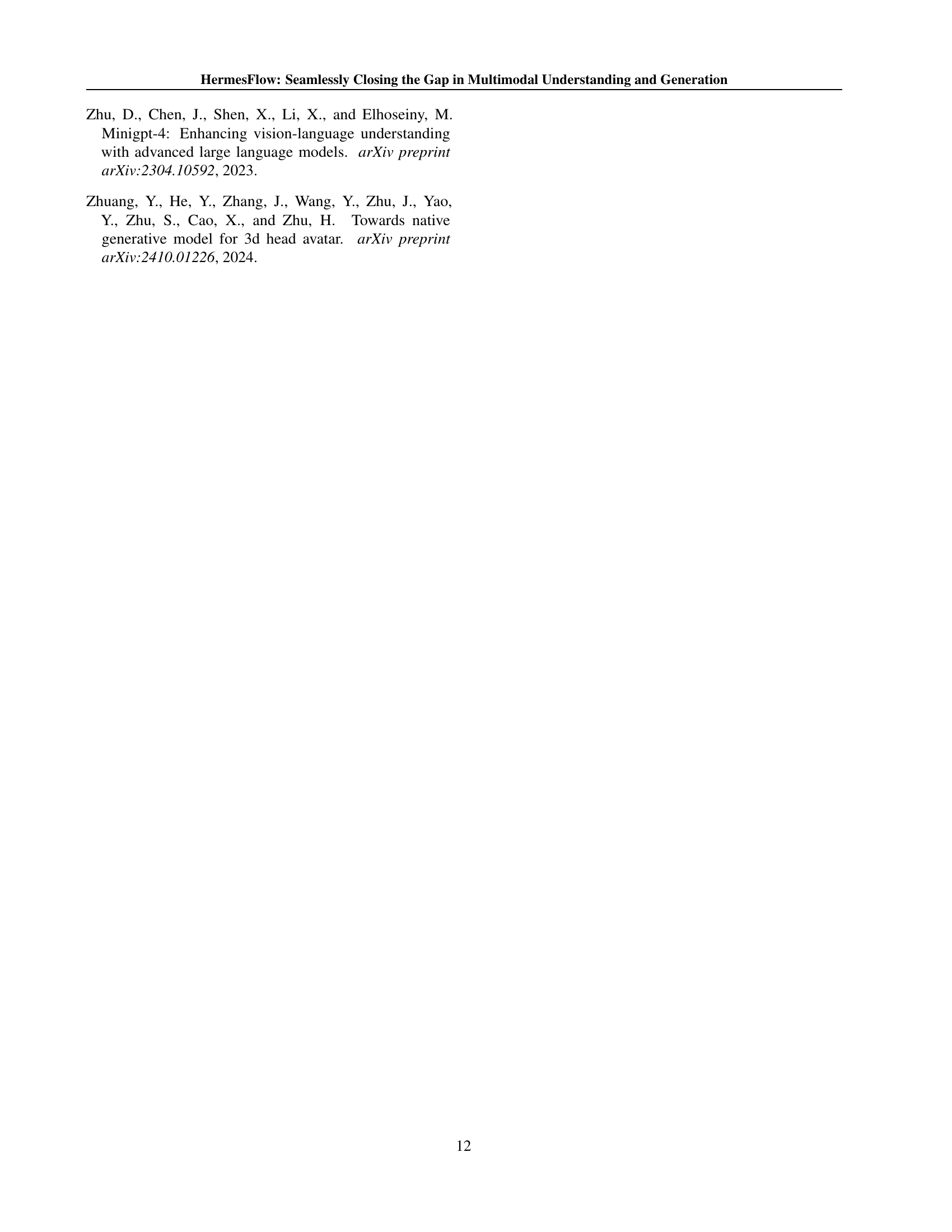
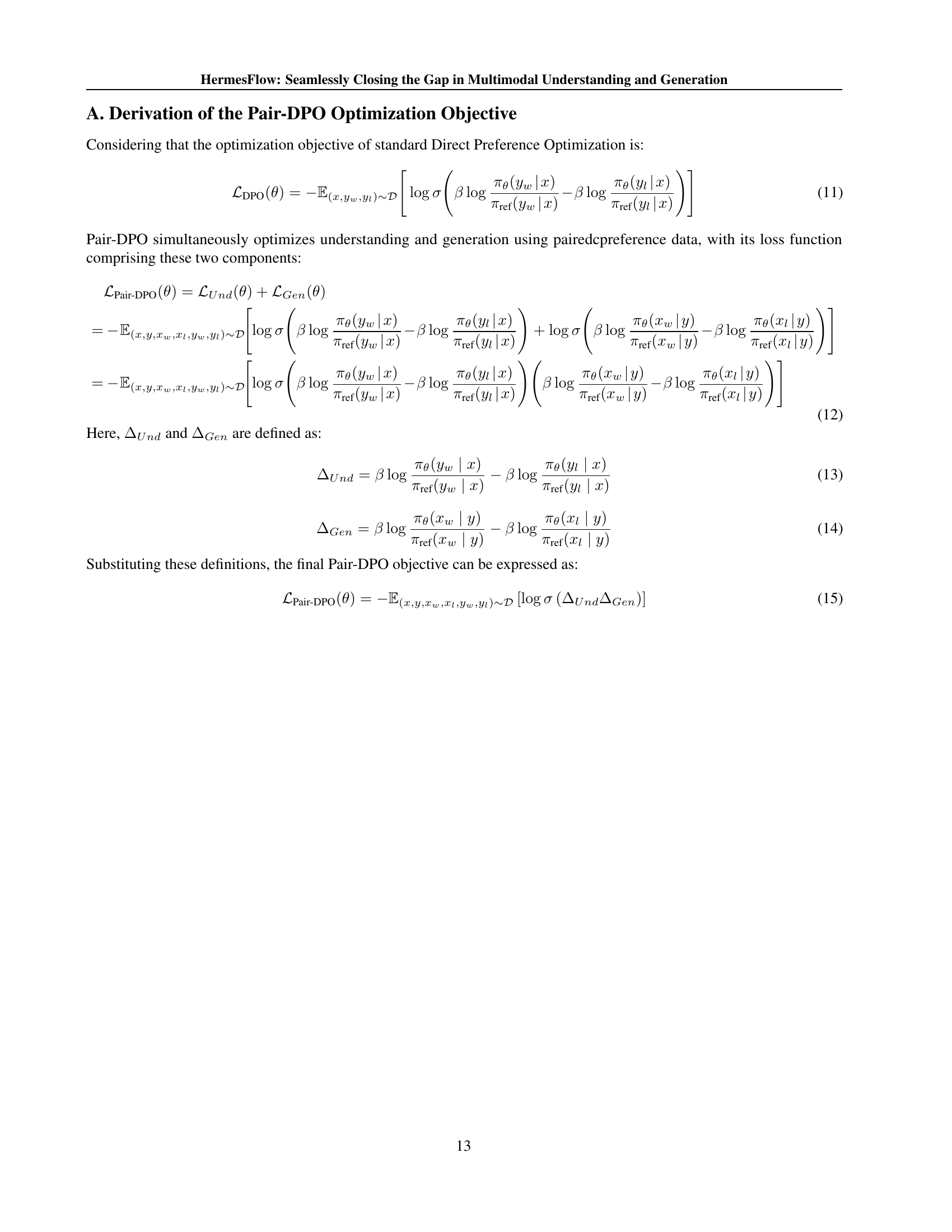
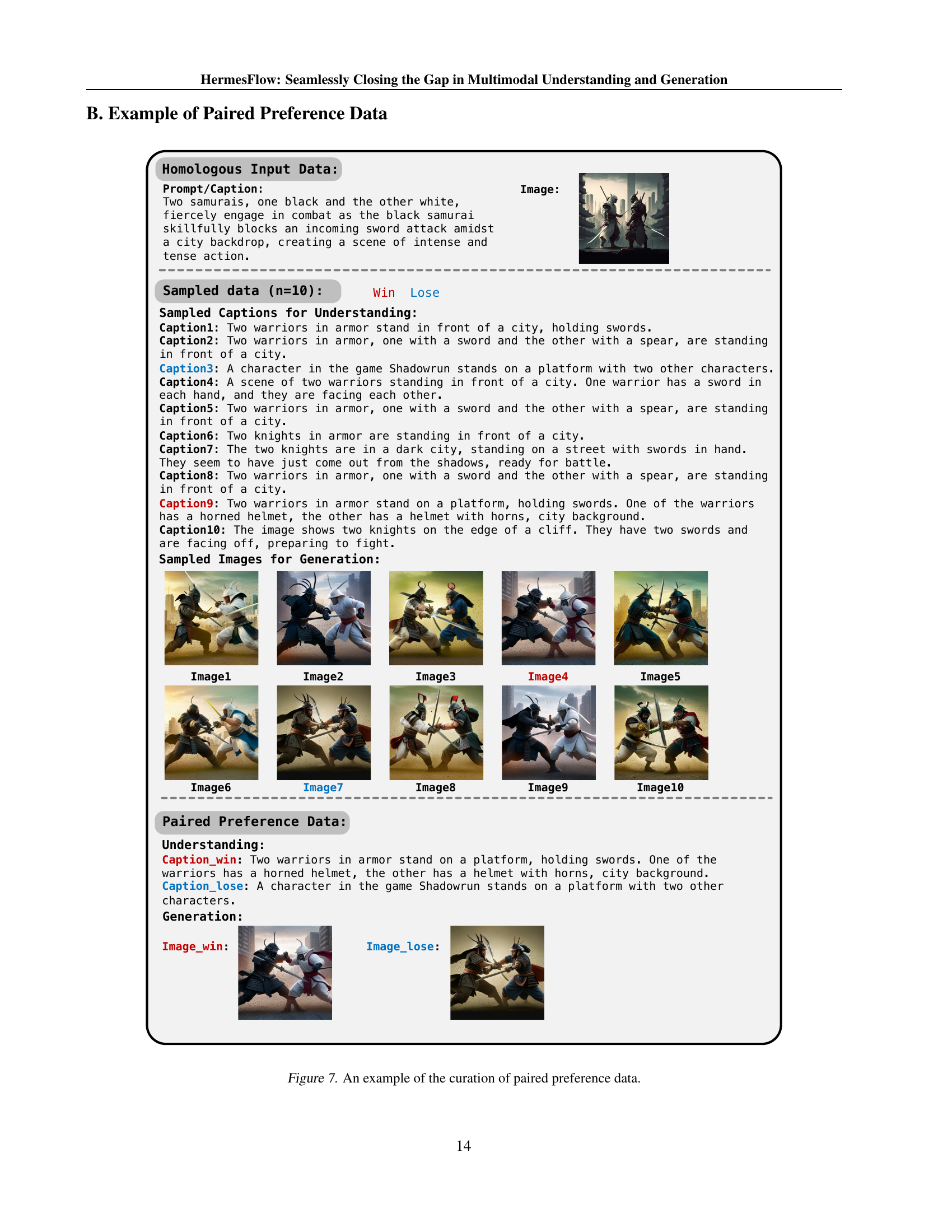