TL;DR#
Recent diffusion models have improved video editing capabilities, but multi-grained editing remains difficult. The major issues are semantic misalignment of text-to-region control and feature coupling within the diffusion model. Editing at different levels (class, instance, part) requires precise control, challenging current methods due to feature mixing and semantic ambiguity.
To address these issues, the paper presents a zero-shot approach that modulates space-time attention mechanisms for fine-grained control. It enhances text-to-region control by amplifying local prompt attention and minimizing irrelevant interactions. It improves feature separation by increasing intra-region awareness and reducing inter-region interference. The method achieves state-of-the-art performance in real-world scenarios.
Key Takeaways#
Why does it matter?#
This work is important because it introduces a novel approach to video editing with multi-level granularity, offering a way to control and modify video content with greater precision. It addresses the critical challenges of semantic misalignment and feature coupling
Visual Insights#
πΌ This figure showcases VideoGrain’s capability to perform multi-grained video editing. It demonstrates three levels of editing: class-level (editing objects within the same class, for example, changing multiple men into Spidermen), instance-level (editing individual distinct objects, such as transforming one man into Spiderman and another into Batman), and part-level (modifying attributes of objects or adding new ones, for instance, adding a hat to Spiderman). The figure visually illustrates these three levels with examples from videos, highlighting the precision and semantic understanding achieved by VideoGrain.
read the caption
Figure 1: VideoGrain enables multi-grained video editing across class, instance, and part levels.
Β | Automatic Metric | Human Evaluation | |||||
---|---|---|---|---|---|---|---|
Method | CLIP-F | CLIP-T | Warp-Err | Q-edit | Edit-Acc | Temp-Con | Overall |
FateZero | 95.75 | 33.78 | 3.08 | 10.96 | 59.8 | 78.6 | 59.6 |
ControlVideo | 97.71 | 34.41 | 4.73 | 7.27 | 53.2 | 50.0 | 43.6 |
TokenFlow | 96.48 | 34.59 | 2.82 | 12.28 | 45.4 | 50.4 | 39.8 |
Ground-A-Video | 95.17 | 35.09 | 4.43 | 7.92 | 69.0 | 72.0 | 63.2 |
DMT | 96.34 | 34.09 | 2.05 | 16.63 | 58.7 | 79.4 | 64.5 |
VideoGrain(ours) | 98.63 | 36.56 | 1.42 | 25.75 | 88.4 | 85.0 | 83.0 |
πΌ This table presents a quantitative comparison of VideoGrain against other state-of-the-art methods for multi-grained video editing. The comparison uses four automatic metrics (CLIP-F, CLIP-T, Warp-Err, Q-edit) to assess the quality of the generated videos, along with human evaluation scores for edit accuracy, temporal consistency, and overall quality. Higher values for CLIP-F, CLIP-T and Q-edit, and lower values for Warp-Err indicate better performance. The best results for each metric are highlighted in bold.
read the caption
Table 1: Quantitative comparison of automatic metrics and human evaluation. The best results are bolded.
In-depth insights#
Multi-Granular Edit#
Multi-granular editing represents a significant leap in content manipulation, moving beyond simple, uniform adjustments to allow for nuanced control at different levels of detail. This means that a user could potentially modify broad categorical aspects, refine specific instances, and even adjust minute elements. This framework is useful because of its adaptable nature, making it a strong tool for many creative and content-related tasks. It presents new difficulties, mostly around preserving logical integrity and preventing unintended consequences as changes cascade across scales. Successfully implementing multi-granular editing necessitates advanced algorithms that grasp the relationships between levels and handle edits accordingly. The potential rewards, including more precise creative control and efficiency.
ST-Layout Attn#
ST-Layout Attn (Spatial-Temporal Layout-Guided Attention) is a core element. It modulates cross-attention for precise text-to-region control by emphasizing relevant spatial areas, thus enhancing the alignment between textual prompts and visual regions. Additionally, it modulates self-attention to enhance intra-region focus and minimize inter-region interference. The goal is to avoid feature coupling and mixing between different objects or parts of objects within the video. This modulation is achieved through unified mechanisms. In essence, it combines what to attend to in the region to maintain focus for better and relevant extraction of text from input prompts to avoid hallucination.
Zero-Shot Editing#
Zero-shot video editing aims to modify videos based on textual prompts without requiring task-specific training. This is achieved by leveraging pre-trained diffusion models and manipulating their attention mechanisms. Challenges include preserving temporal consistency, maintaining high fidelity, and accurately controlling edits across different granularities (class, instance, part level). Methods like attention modulation, masking, and feature blending are employed to achieve these goals. The key is to balance semantic control with temporal coherence, ensuring that the edited video remains realistic and consistent.
Feature Seperation#
Feature separation in diffusion models is crucial for multi-grained video editing, preventing unwanted blending of attributes between edited regions. Without explicit mechanisms, models tend to homogenize features, leading to artifacts and inaccurate edits. Techniques modulating self-attention can enhance intra-region focus while suppressing inter-region interference, ensuring distinct objects retain unique characteristics and preventing texture mixing. Proper feature separation is essential for achieving high-fidelity, controlled video manipulation, especially when dealing with multiple instances or part-level modifications, enabling precise and semantically meaningful edits.
Limited Generalize#
Addressing the “Limited Generalize” aspect is crucial for advancing video editing models. The current method relies on zero-shot learning, which inherently limits its ability to generate high-quality results if the base model produces artifacts or fails to capture ideal generation priors. Scenarios involving significant shape deformations or appearance changes pose a challenge, as the model struggles to adapt due to its dependence on the T2I framework. Incorporating motion priors from T2V models presents a promising avenue for future research, potentially enabling the model to overcome these limitations and achieve more robust and versatile video editing capabilities. This will enhance the model’s ability to generalize across diverse scenarios, improving the realism and consistency of edited videos. The focus on zero-shot learning in this research introduces inherent limitations due to the foundational generation quality being constrained by the inherent capacity of the underlying model.
More visual insights#
More on figures
πΌ The figure illustrates the concept of multi-grained video editing, which involves modifications at three levels: class, instance, and part. Class-level editing changes all objects within a specific class (e.g., changing all men to Spiderman). Instance-level editing modifies individual objects separately (e.g., changing one man to Spiderman and another to a polar bear). Finally, part-level editing focuses on modifying specific attributes of an object (e.g., adding sunglasses to a polar bear). The figure also highlights the challenges of existing instance editing methods, which often mix features of different instances during editing, leading to artifacts. This contrasts with the goal of multi-grained editing to provide precise control over each level of modification.
read the caption
Figure 2: Definition of multi-grained video editing and comparison on instance editing
πΌ This figure analyzes the failure of a diffusion model in instance-level video editing. The objective was to transform the left man into Iron Man, the right man into Spiderman, and the trees into cherry blossoms. Subfigure (a) shows the input video. Subfigure (b) demonstrates the application of K-Means clustering to the self-attention features, revealing a semantic layout but failing to distinguish between distinct instances. Subfigure (d) visualizes the 32x32 cross-attention map generated when the model attempts the edit using the prompt βAn Iron Man and a Spiderman are jogging under cherry blossoms,β highlighting the issue of feature mixing and misalignment between textual prompts and corresponding visual regions.
read the caption
Figure 3: Analysis of why the diffusion model failed in instance-level video editing. Our goal is to edit left man into βIron Man,β right man into βSpiderman,β and trees into βcherry blossoms.β In (b), we apply K-Means on self-attention, and in (d), we visualize the 32x32 cross-attention map.
πΌ Figure 4 illustrates the VideoGrain pipeline, a novel framework for multi-grained video editing. It shows how spatial-temporal layout-guided attention (ST-Layout Attn) is integrated into a pre-trained Stable Diffusion model. This integration modulates both cross-attention and self-attention mechanisms for finer control. Cross-attention is modulated to ensure that each local prompt focuses on its correct spatial region (positive pairs), while ignoring irrelevant areas (negative pairs), achieving precise text-to-region control. Self-attention is modulated to amplify intra-region interactions and suppress inter-region interactions across frames. This improves feature separation and helps maintain temporal consistency. The bottom of the figure visually explains the modulation process, showing how attention weights are adjusted using positive and negative pair scores.
read the caption
Figure 4: VideoGrain pipeline. (1) we integrate ST-Layout Attn into the frozen SD for multi-grained editing, where we modulate self- and cross-attention in a unified manner. (2) In cross-attention, we view each local prompt and its location as positive pairs, while the prompt and outside-location areas are negative pairs, enabling text-to-region control. (3) In self-attention, we enhance positive awareness within intra-regions and restrict negative interactions between inter-regions across frames, making each query only attend to the target region and keep feature separation. In the bottom two figures, pπpitalic_p denotes original attention score and w,iπ€πw,iitalic_w , italic_i denotes the word and frame index.
πΌ Figure 5 presents example results demonstrating VideoGrain’s capability for multi-grained video editing. It showcases edits at three levels of granularity: class-level (modifying objects within the same class), instance-level (modifying specific instances of objects), and part-level (adding new objects or modifying attributes of existing objects). The figure visually demonstrates the versatility and precision of the proposed VideoGrain model. For a more comprehensive view of the editing results including video clips and more examples, refer to the project page linked in the paper.
read the caption
Figure 5: Qualitative results. VideoGrain achieves multi-grained video editing, including class-level, instance-level, and part-level. We refer the reader to our project page for full-video results.
πΌ Figure 6 presents a qualitative comparison of VideoGrain’s performance against other state-of-the-art methods across class, instance, and part levels of video editing. The figure showcases examples of animal and human instance edits, and part-level modifications, highlighting VideoGrain’s ability to achieve more precise and accurate results compared to baselines. For a detailed analysis of the results, including video demonstrations, please refer to the project page.
read the caption
Figure 6: Qualitative comparisons. We refer the reader to our project page for detailed assessment.
πΌ This figure visualizes the attention weight distribution before and after applying the Spatial-Temporal Layout-Guided Attention (ST-Layout Attn) module. It showcases how ST-Layout Attn refines attention weights to improve the precision of multi-grained text-to-region control and feature separation. The ‘before’ visualization demonstrates attention leakage, highlighting the feature mixing problem prevalent in diffusion models without ST-Layout Attn. The ‘after’ visualization, in contrast, shows how ST-Layout Attn focuses attention to relevant regions for each target while suppressing attention to irrelevant areas, thus effectively addressing feature mixing and enhancing the accuracy and quality of the edits.
read the caption
Figure 7: Attention weight distribution.
πΌ This figure shows an ablation study on the impact of cross-attention and self-attention modulation within the Spatial-Temporal Layout Attention (ST-Layout Attn) module of the VideoGrain model. It demonstrates how modulating these attention mechanisms improves the model’s ability to perform multi-grained video editing. The results show that both cross-attention and self-attention modulation are essential for accurate and high-quality edits, particularly when handling multiple instances or complex edits. The figure presents several edited video frames alongside quantitative metrics to support the findings.
read the caption
Figure 8: Ablation of cross- and self-modulation in ST-Layout Attn.
πΌ This figure compares the performance of VideoP2P, a video editing method using SAM-Track instance masks, with the proposed VideoGrain method. It demonstrates two editing approaches: (1) joint editing, where multiple regions are modified simultaneously in a single denoising step, and (2) sequential editing, where each region is modified sequentially in separate denoising steps. The results show that VideoGrain outperforms VideoP2P in both accuracy and consistency, particularly in scenarios with complex edits.
read the caption
Figure 9: VideoP2P joint and sequential edit with SAM-Track masks
πΌ This figure demonstrates the limitations of the Ground-A-Video method in performing joint edits with instance information. Despite providing instance-level grounding (information about the location and identity of objects), Ground-A-Video struggles to correctly modify multiple instances simultaneously in a single edit pass. The figure highlights the failures of this approach in contrast to the capabilities of the VideoGrain model, which successfully handles multi-grained video editing.
read the caption
Figure 10: Ground-A-Video joint edit with instance information
πΌ Figure 11 demonstrates the capability of the proposed VideoGrain method to perform multi-grained video editing without relying on additional instance segmentation masks from SAM-Track. It showcases the results of editing a video of Batman playing tennis on a snowy court before an iced wall, using three different approaches: (1) The input video, (2) Results obtained using Ground-A-Video, showing its limitations in multi-grained editing, (3) Results from applying DDIM inversion cluster masks to identify regions for editing, demonstrating the methodβs reliance on inherent semantic layout information rather than external masks. Finally, (4) displays the results from VideoGrain, illustrating its ability to successfully edit the video. This illustrates that VideoGrain does not strictly depend on SAM-Track, but leverages the diffusion modelβs self-attention features for multi-grained video editing.
read the caption
Figure 11: Our method without additional SAM-Track masks
πΌ This figure demonstrates the capability of the VideoGrain model to edit specific subjects within a video while leaving the background unchanged. The leftmost image shows the original video frame. The next three images show the results of editing only the left subject, only the right subject, and both subjects simultaneously. This showcases the model’s ability to perform selective edits with precision.
read the caption
Figure 12: Soely edit on specific subjects, without background changed
πΌ Figure 13 presents examples of part-level modifications achievable with the VideoGrain method. The left side shows modifications on humans, demonstrating changes such as altering a shirt’s color from gray to blue, or changing the shirt style from a half-sleeve to a full suit. The right side shows modifications on animals, specifically changing a cat’s head and body color while retaining other features. These examples showcase VideoGrain’s capability to make fine-grained edits to specific parts of objects within a video frame, enhancing its versatility for detailed video manipulation.
read the caption
Figure 13: Part-level modifications on humans and animals
πΌ This figure compares the results of different approaches to handling temporal consistency in video editing. Specifically, it contrasts using a per-frame approach, a sparse-causal approach (considering only the current and immediately preceding frame), and the proposed ST-Layout Attention (ST-Layout Attn) method. The goal is to show how ST-Layout Attn effectively avoids flickering and maintains consistent visual details across frames during multi-grained video editing, unlike the other methods that might produce inconsistencies.
read the caption
Figure 14: Temporal Focus of ST-Layout Attn
πΌ This figure demonstrates the impact of ControlNet on the model’s performance. ControlNet is a technique that uses additional information, such as depth or pose maps, to guide the image generation process. The figure shows that even without ControlNet (using only the textual descriptions), the model can still edit videos with multiple regions simultaneously. However, there might be some inconsistencies between edited and original objects without ControlNet due to lack of explicit structural guidance.
read the caption
Figure 15: ControlNet ablation
πΌ This figure showcases the versatility of the VideoGrain model in handling more complex editing tasks involving general objects and shape changes. The top row demonstrates instance editing on animals, successfully replacing animals with others while maintaining the background context. The bottom row demonstrates shape editing on cars, effectively changing the make and model of a vehicle while preserving the overall scene. This highlights the model’s ability to perform multi-grained editing, seamlessly integrating changes into existing video content, regardless of the complexity of the scene or the type of objects involved.
read the caption
Figure 16: More general objects instance editing (animals) and shape editing (cars) results.
πΌ This ablation study investigates the impact of using ST-Layout Attention (ST-Layout Attn) with varying numbers of frames on attention weight distribution. It compares the attention weight distribution when ST-Layout Attn is applied to (1) the first frame only, (2) each frame individually, and (3) across the entire video sequence. The goal is to demonstrate how ST-Layout Attn helps maintain consistency in attention weights across frames and improve the temporal coherence of the generated video, mitigating issues like flickering or inconsistencies that could arise from processing individual frames in isolation.
read the caption
Figure 17: More frames ablation of ST-Layout Attnβs effects on attention weight distribution.
More on tables
Β | Time(min) | Memory (GB) | RAM (GB) |
---|---|---|---|
FateZero | 8.68 | 27.35 | 144.22 |
ControlVideo | 4.41 | 16.15 | 7.03 |
TokenFlow | 4.56 | 17.84 | 5.35 |
Ground-A-Video | 5.81 | 17.31 | 9.96 |
DMT | 5.79 | 27.88 | 8.12 |
VideoGrain | 3.83 | 15.94 | 4.42 |
πΌ This table presents a comparison of the computational efficiency of different video editing methods. It shows the time taken to perform a single edit (in minutes), GPU memory usage (in GB), and RAM usage (in GB) for each method. The methods compared are FateZero, ControlVideo, TokenFlow, Ground-A-Video, DMT, and VideoGrain (the authors’ method). This allows for a direct comparison of the resource requirements of each approach.
read the caption
Table 2: Efficiency comparison.
Β Method | CLIP-F | CLIP-T | Warp-Err | |
---|---|---|---|---|
Baseline | 95.21 | 33.59 | 3.86 | 8.70 |
Baseline + Cross Modulation | 96.28 | 36.09 | 2.53 | 14.26 |
Baseline + Cross Modulation + Self Modulation | 98.63 | 36.56 | 1.42 | 25.75 |
πΌ This table presents a quantitative analysis of the impact of cross-attention and self-attention modulation within the ST-Layout Attention mechanism of the VideoGrain model. It compares the performance of the model under different configurations: a baseline model, a model with only cross-attention modulation, and a model with both cross-attention and self-attention modulation. The results are evaluated using four metrics: CLIP-F, CLIP-T, Warp-Err, and Q-edit, which assess different aspects of video editing quality, including feature similarity, temporal consistency, and overall editing quality. The goal is to demonstrate the individual and combined contributions of cross-attention and self-attention modulation to the overall performance of the VideoGrain model.
read the caption
Table 3: Quantitative ablation of cross- and self-modulation in ST-Layout Attn.
Full paper#
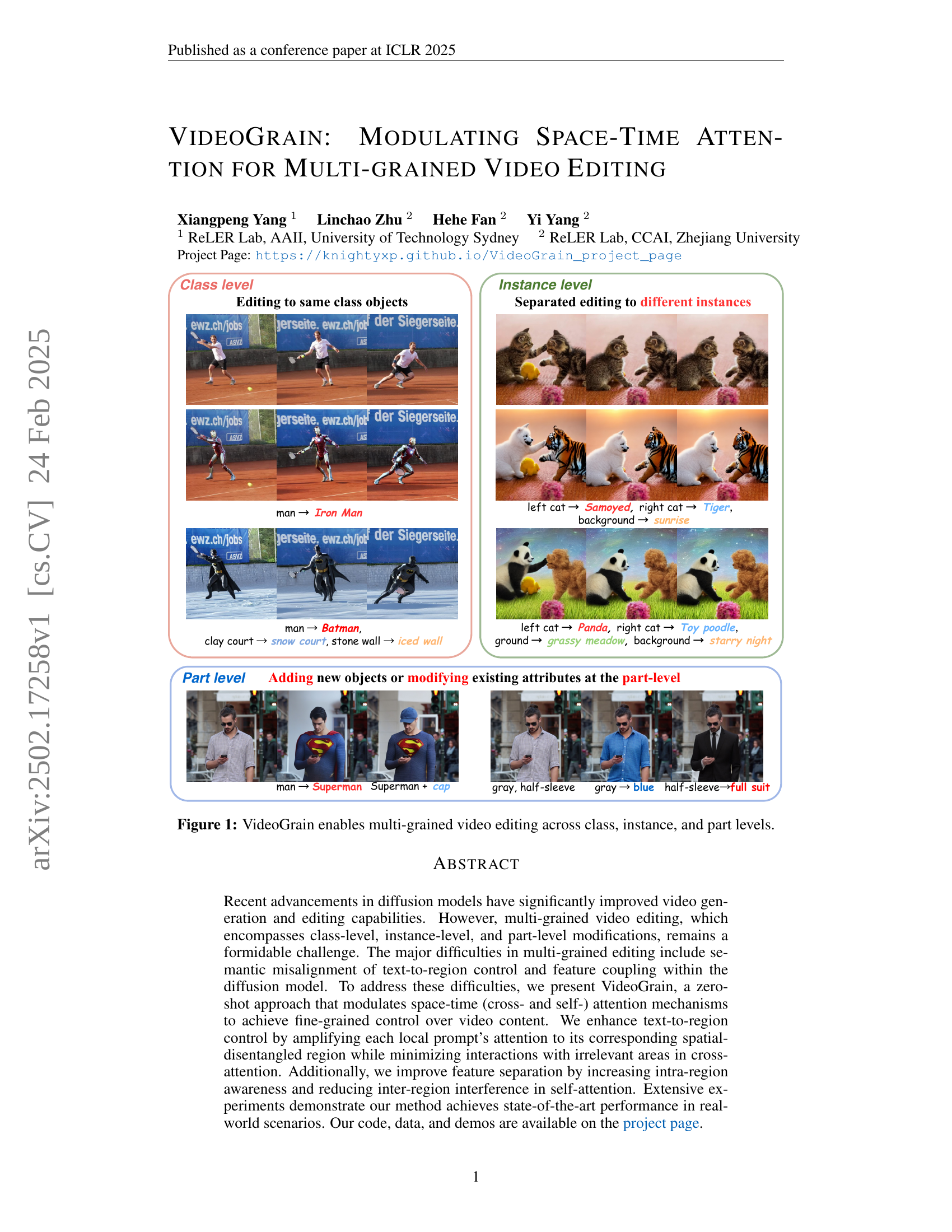
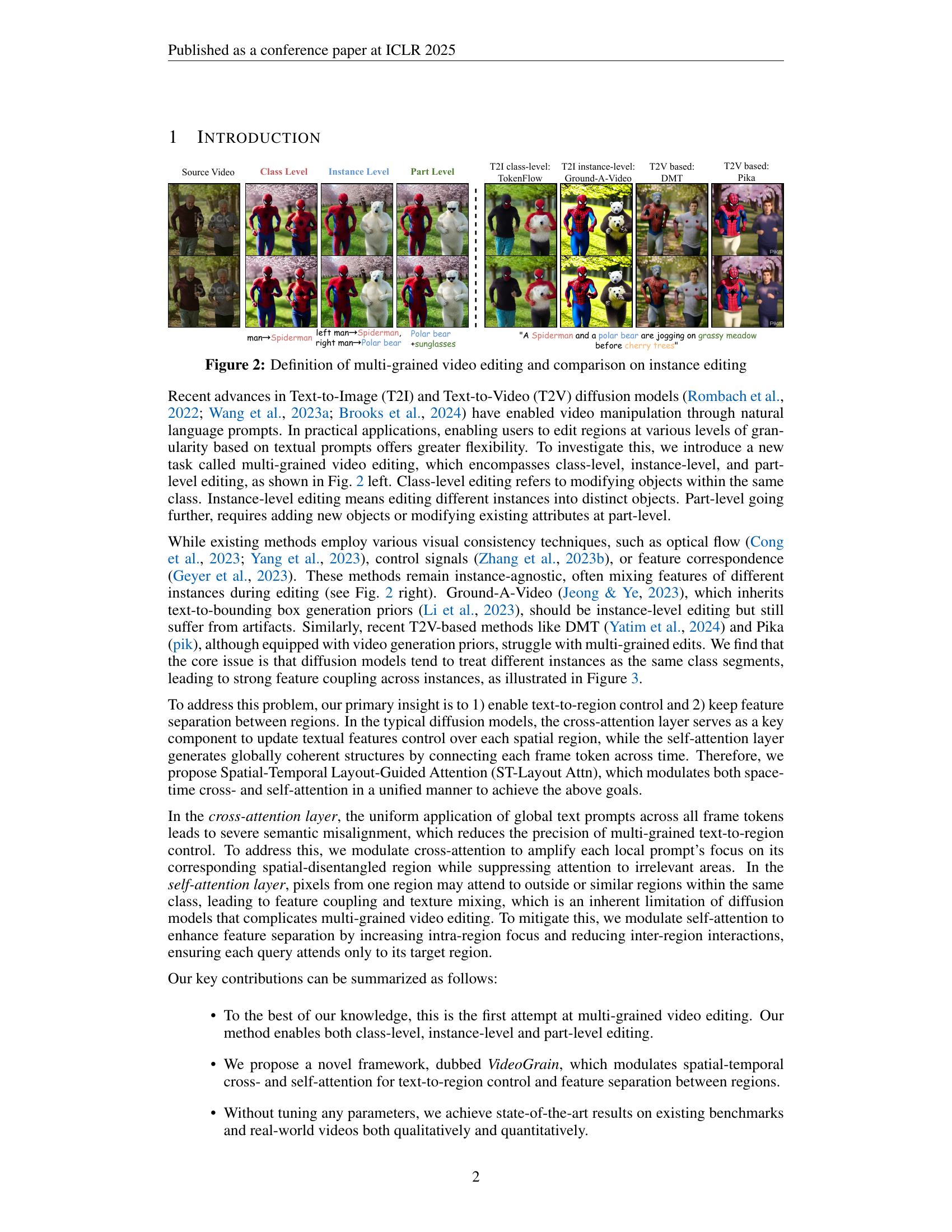
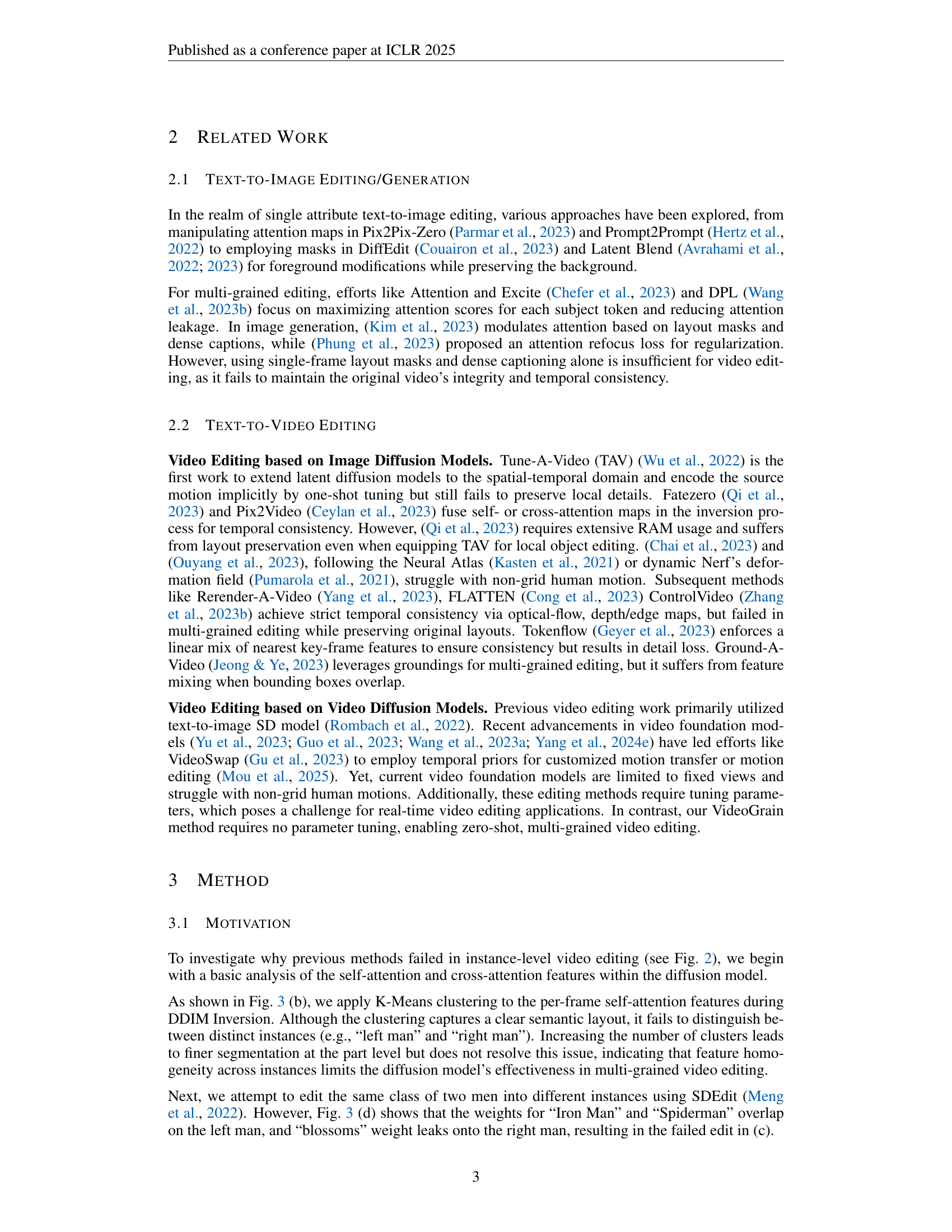
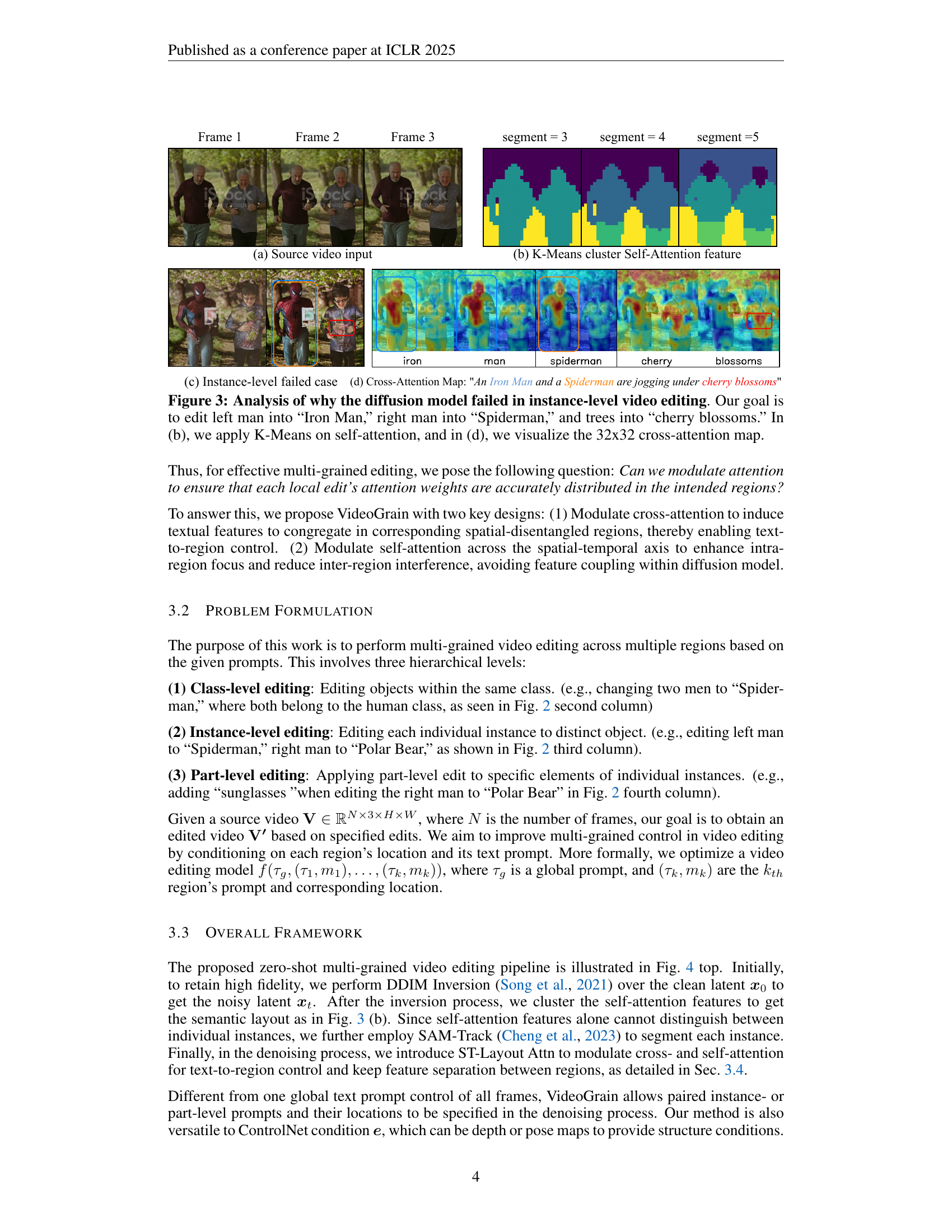
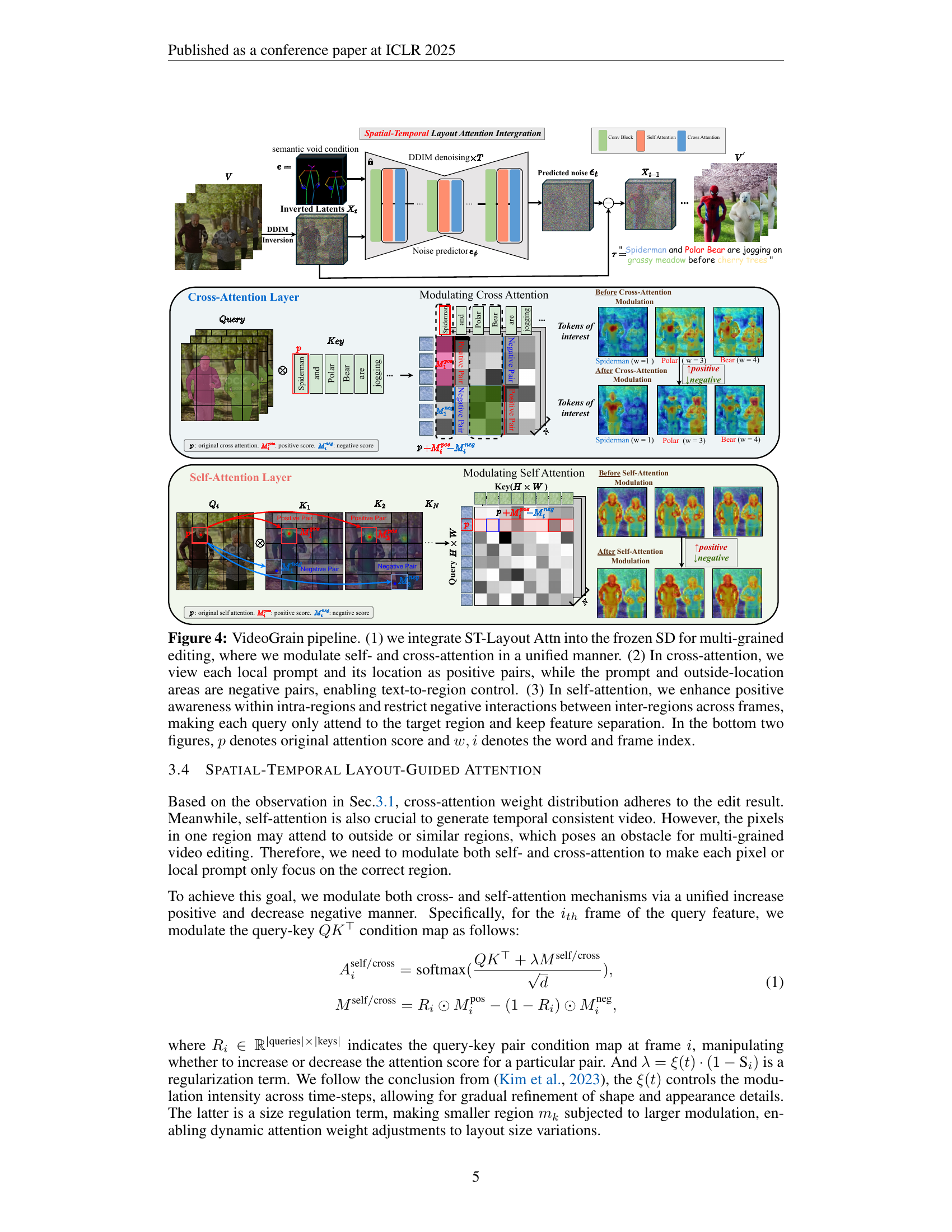
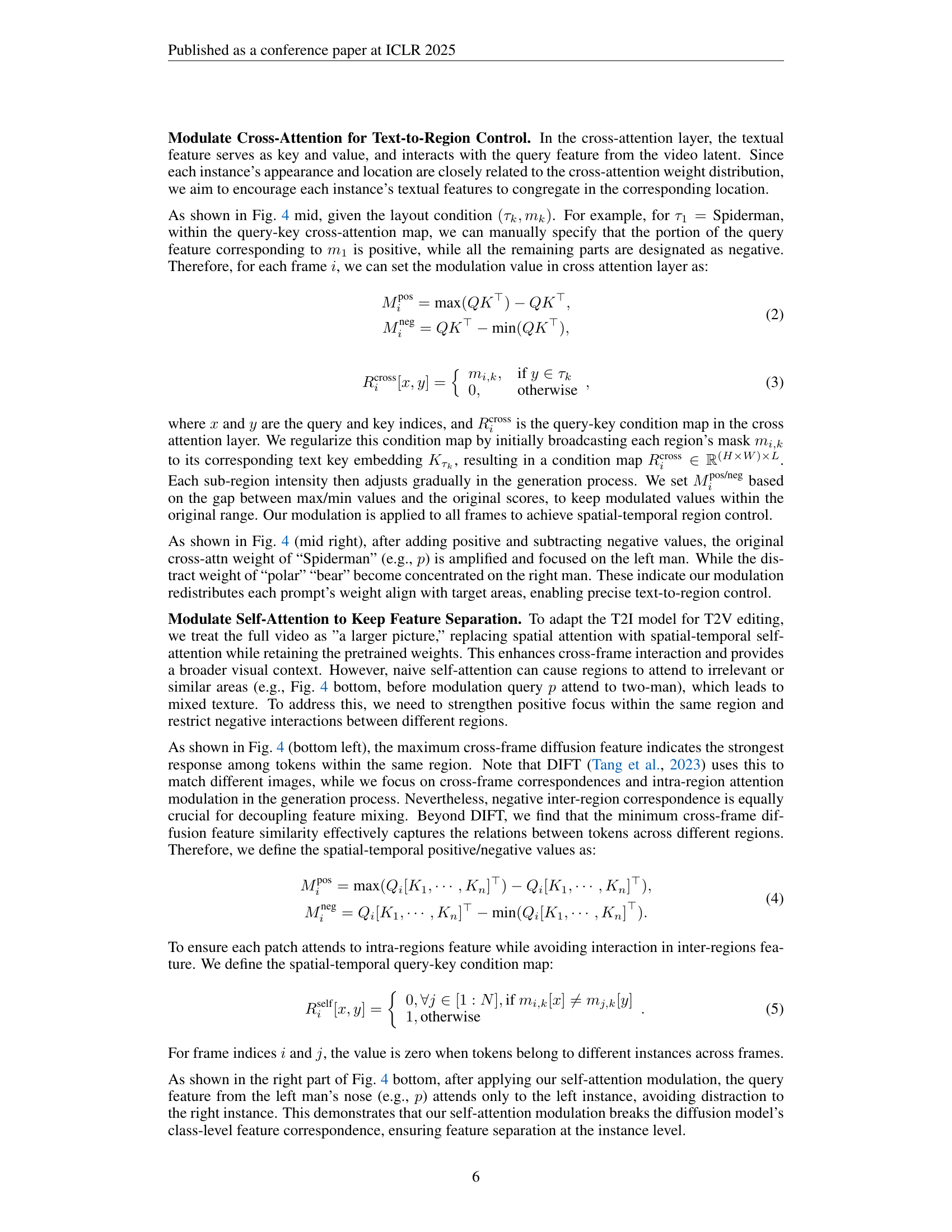
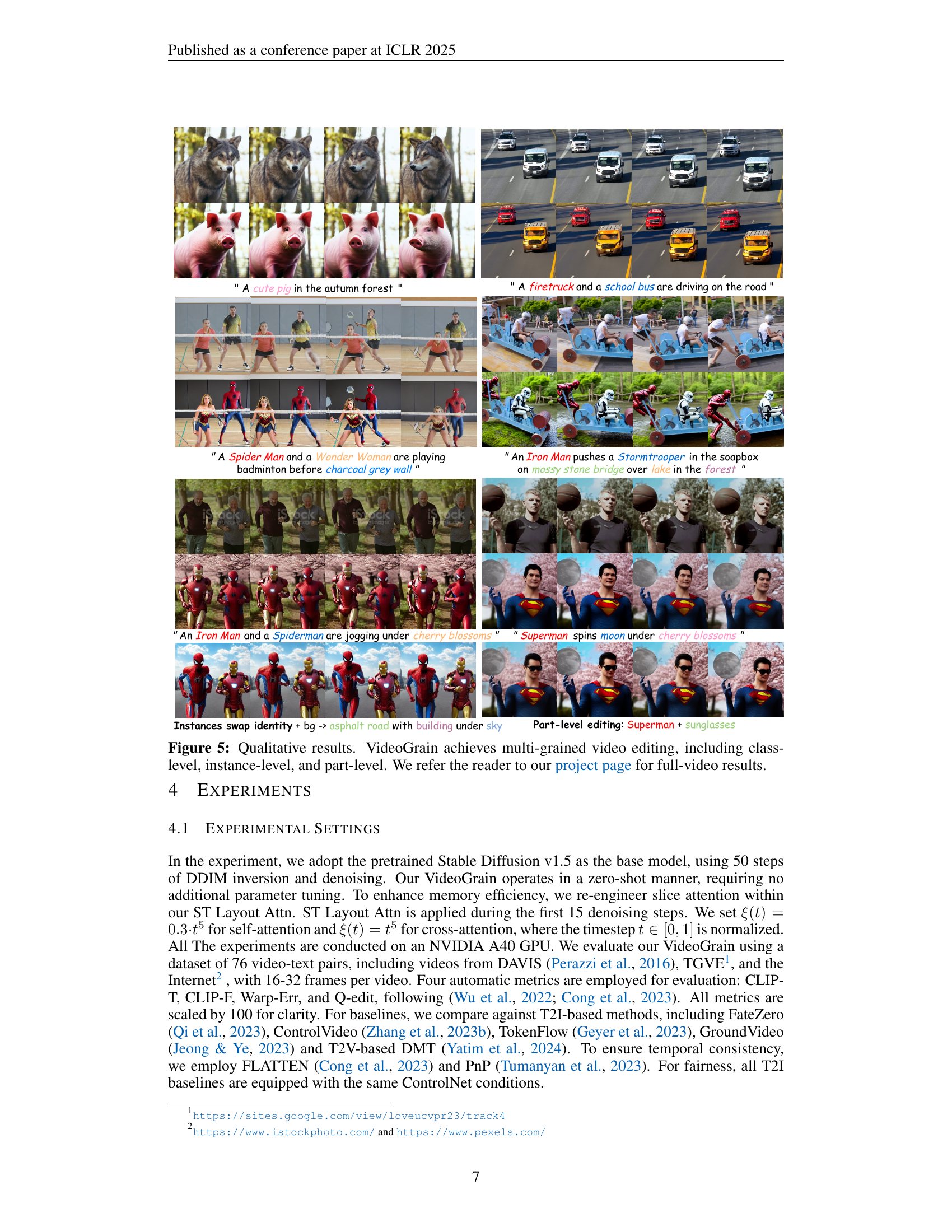
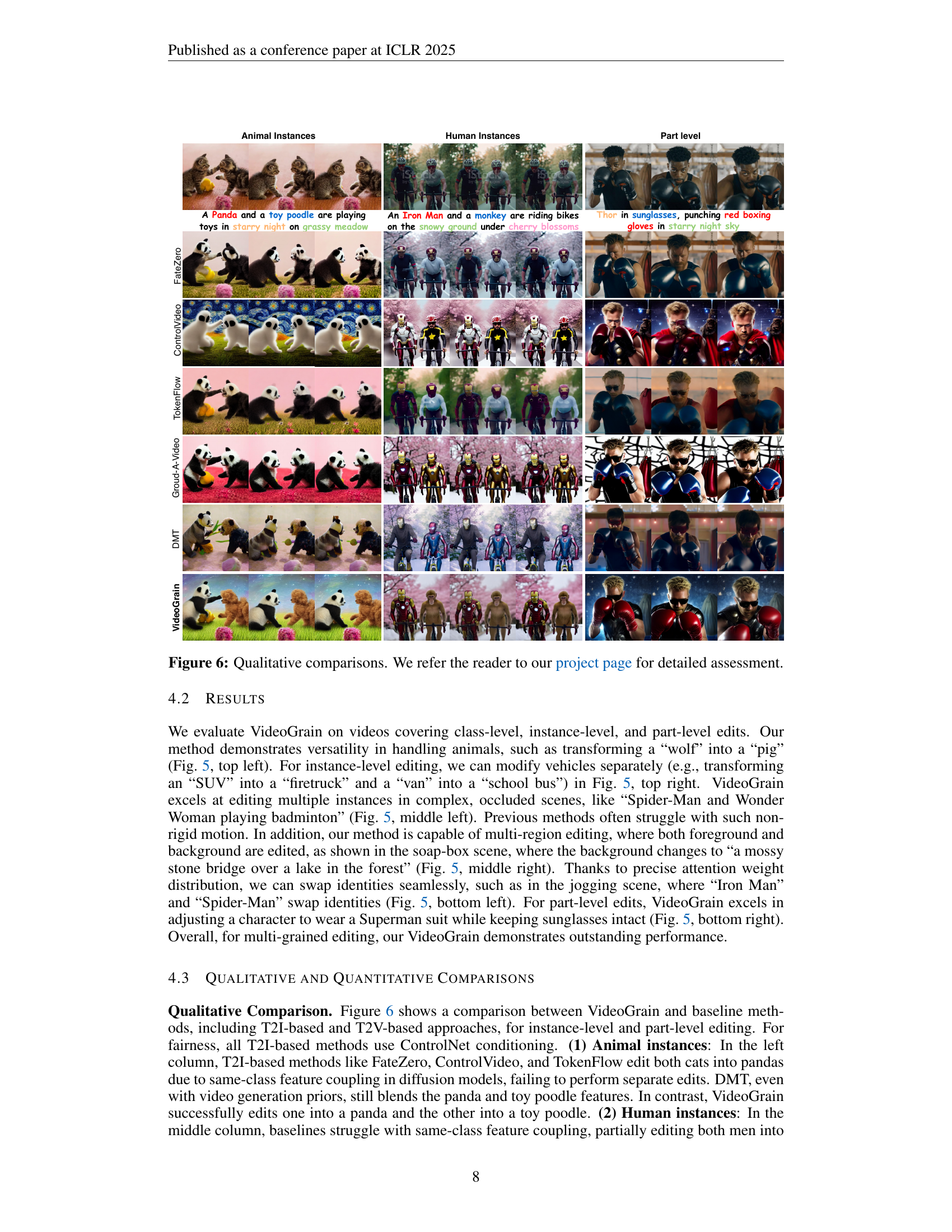
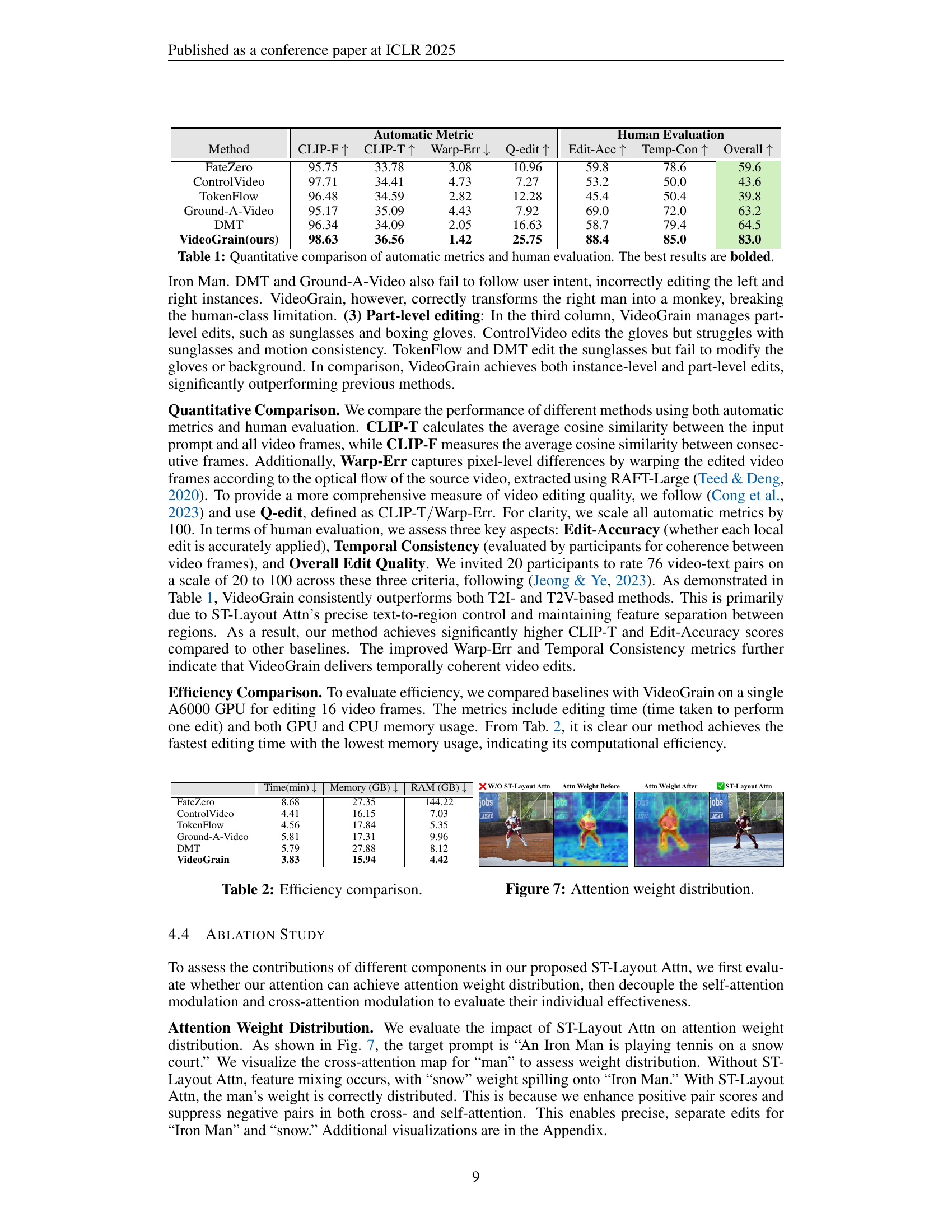
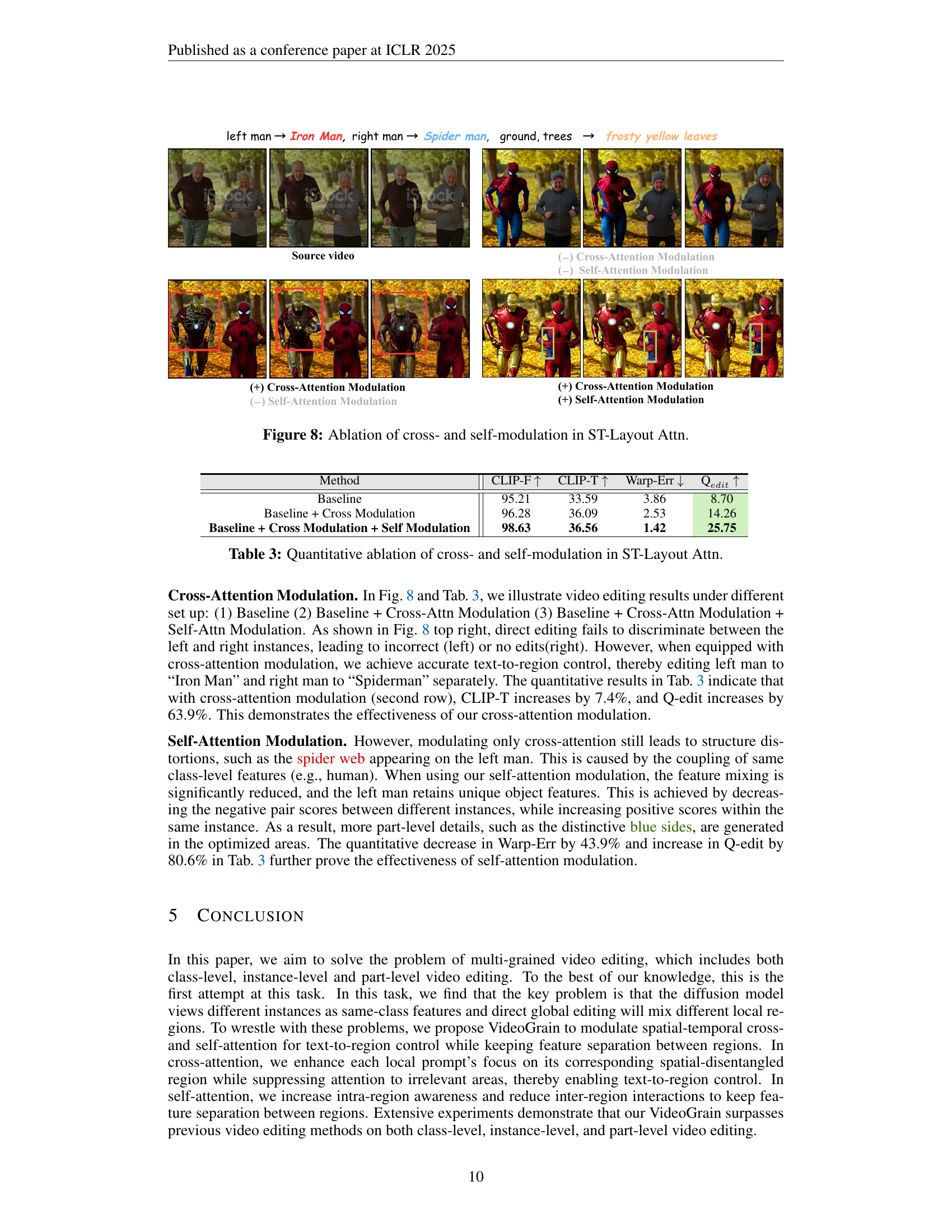
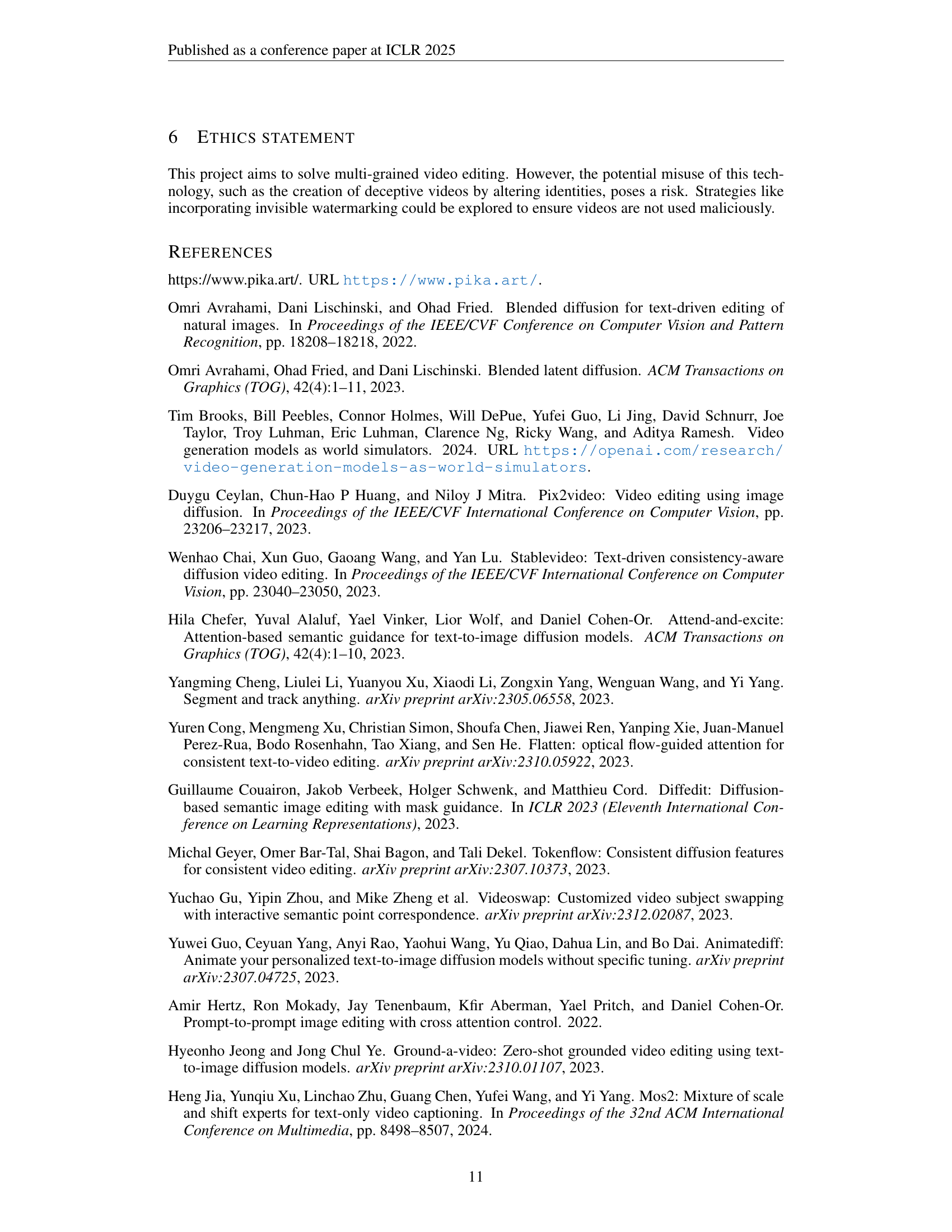
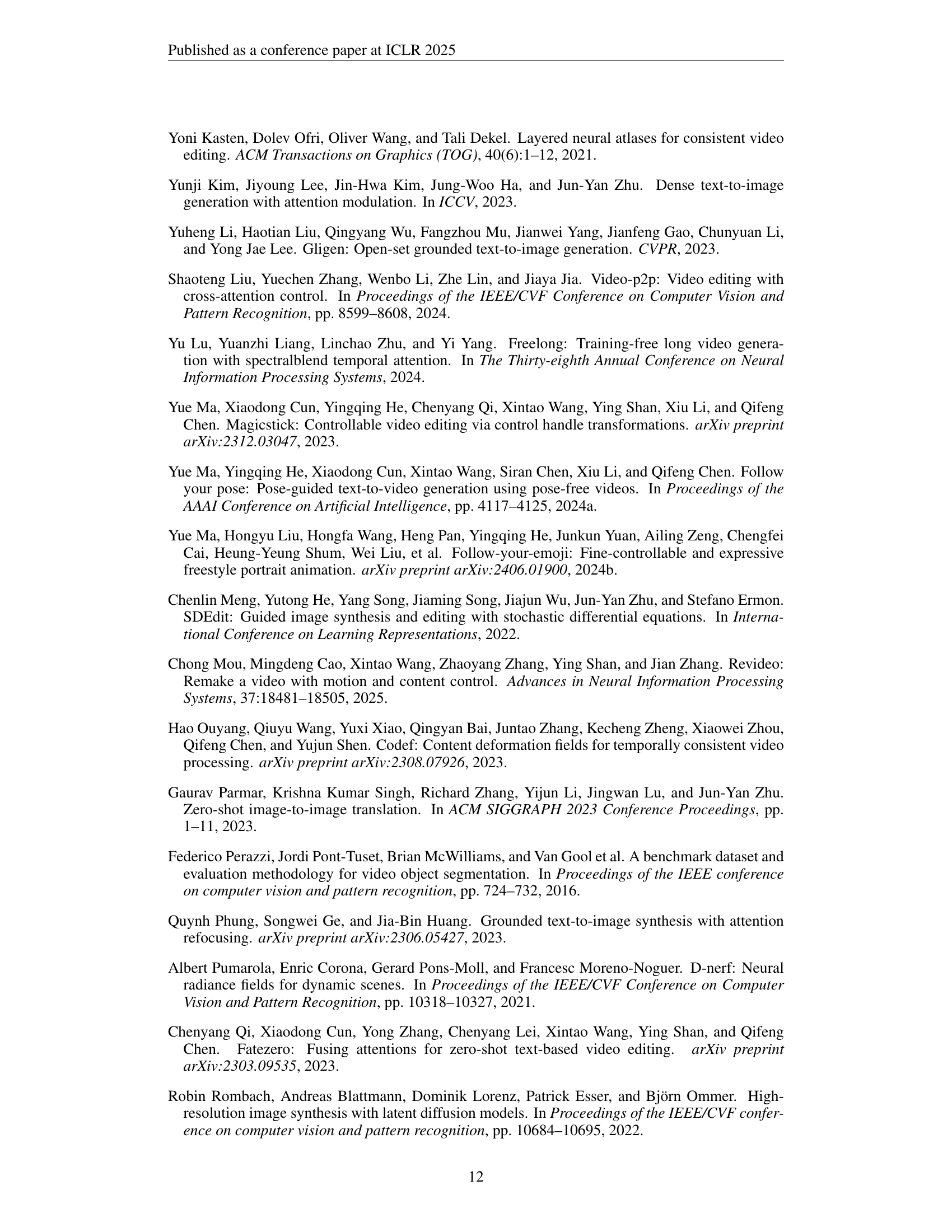
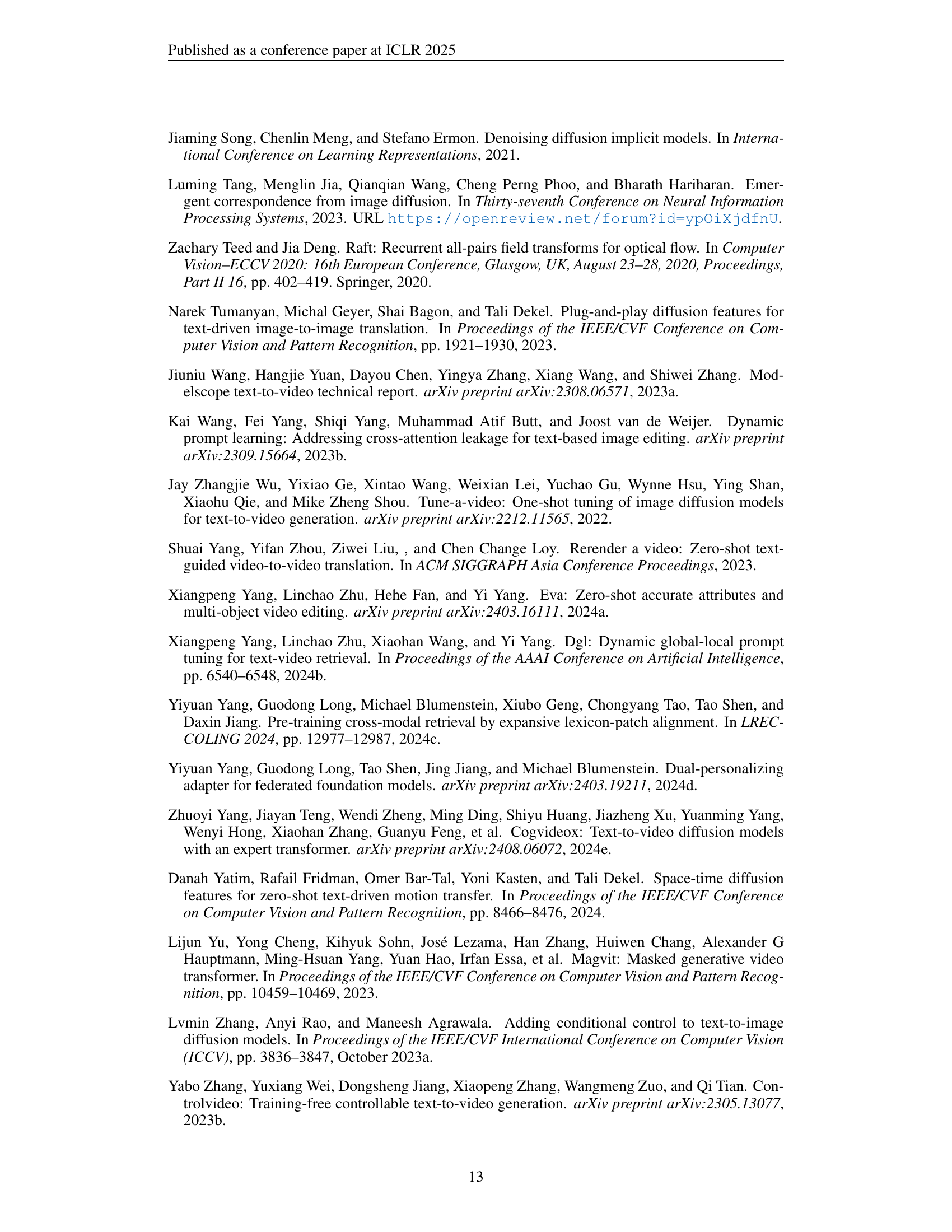
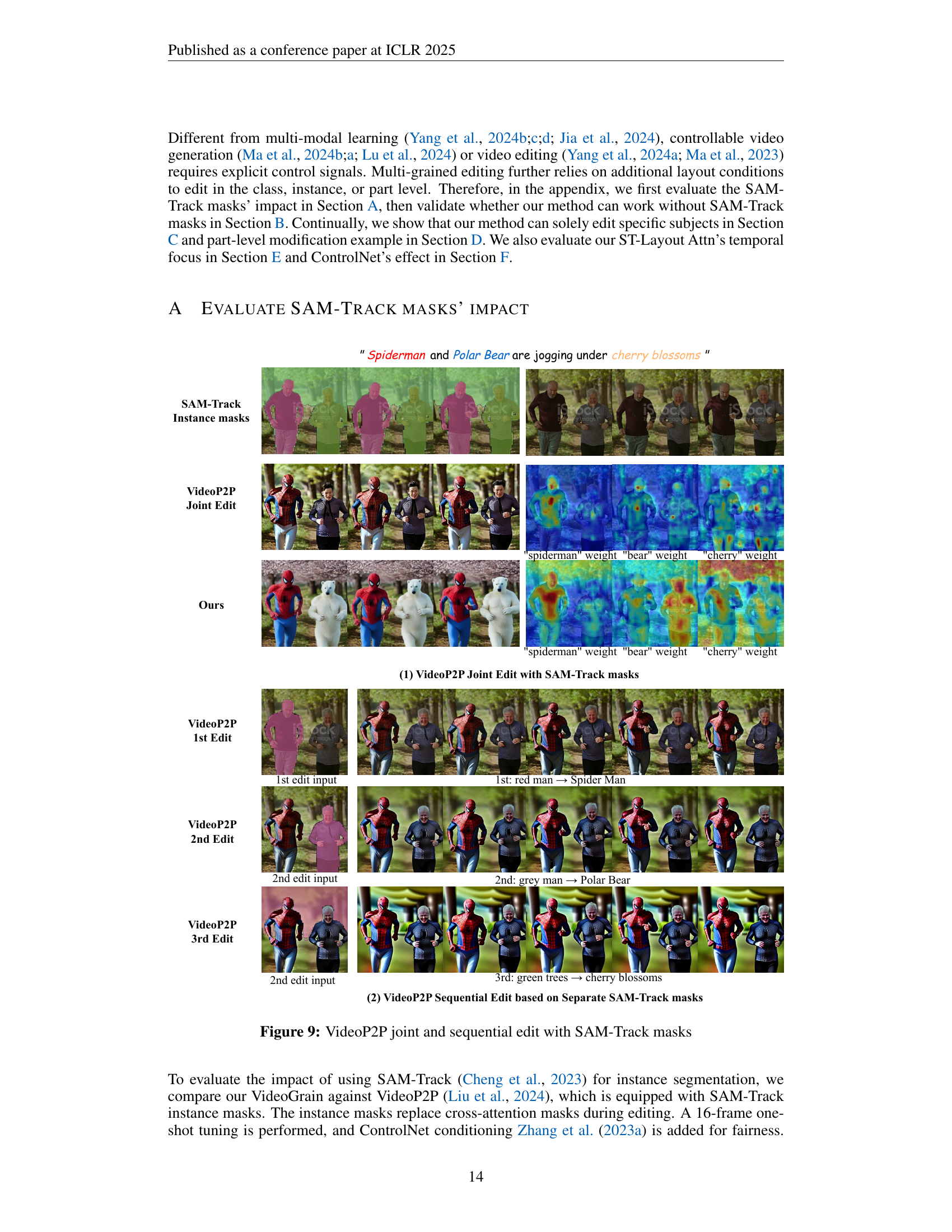
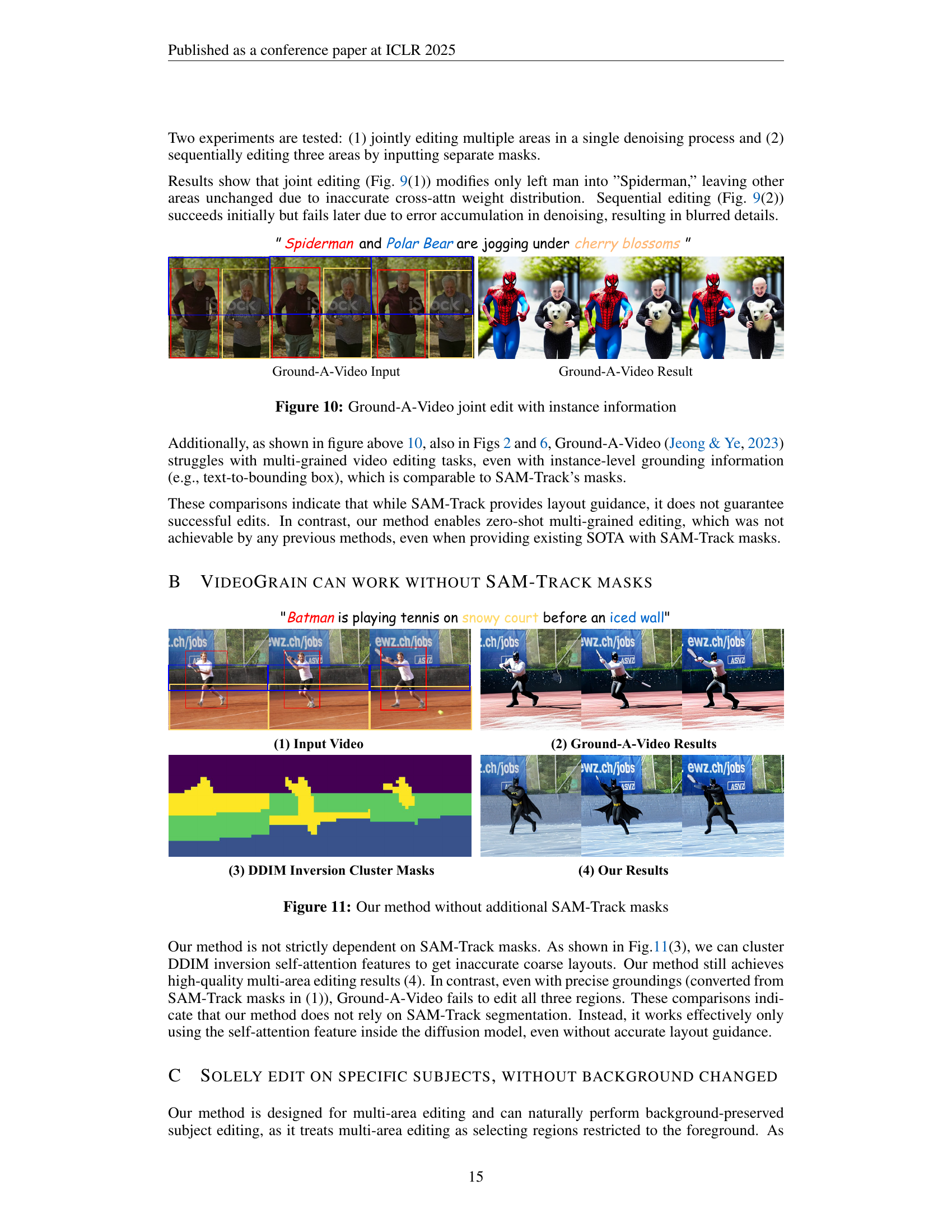
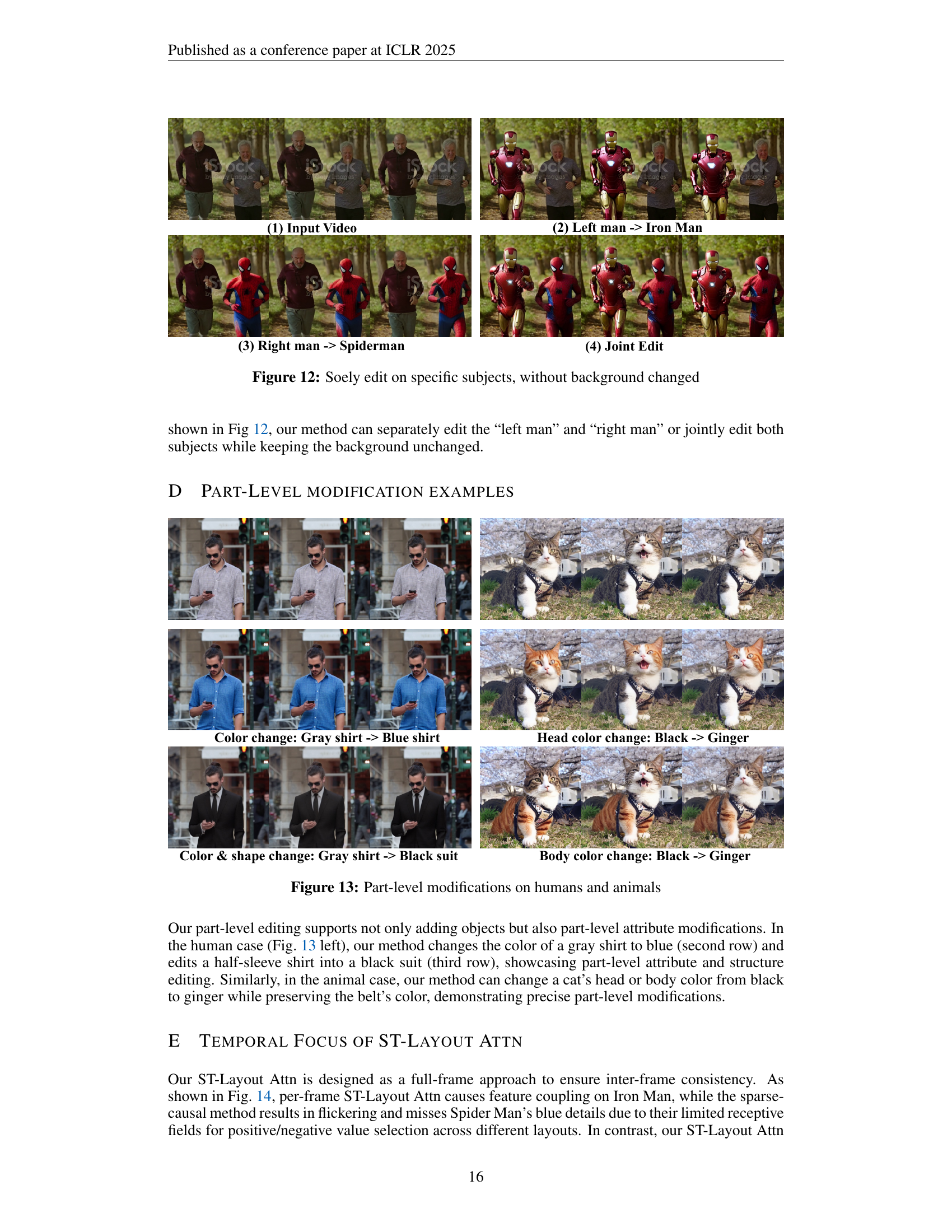
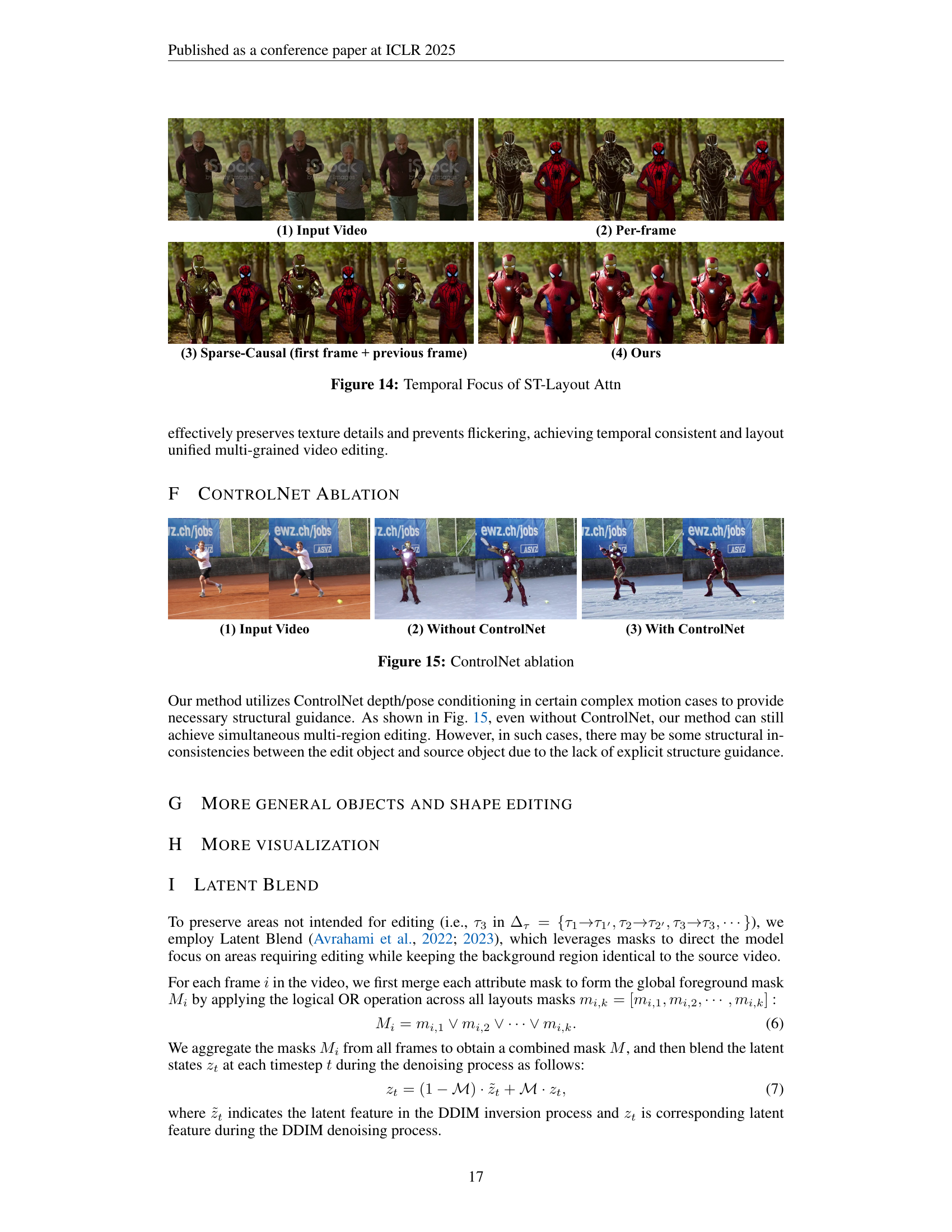
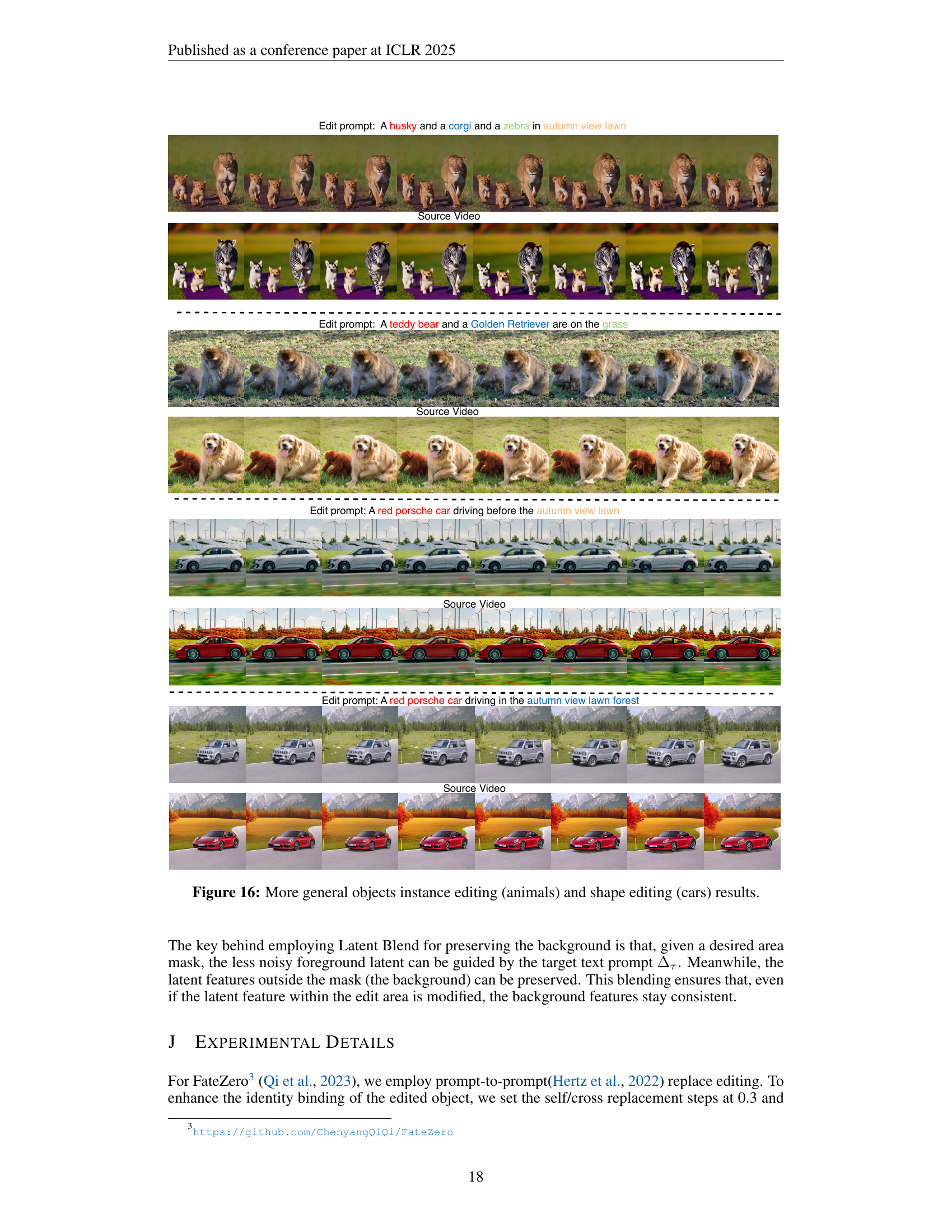
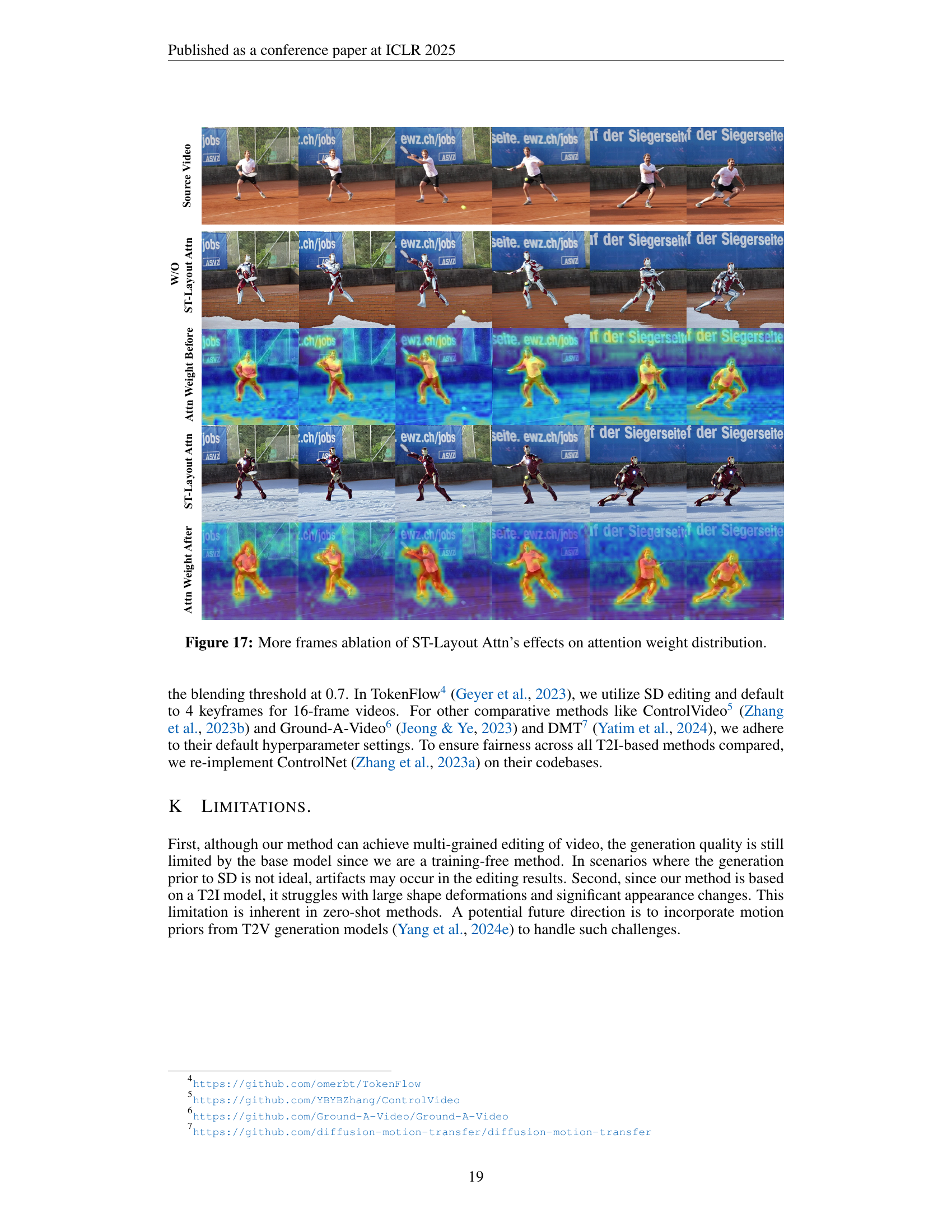