TL;DR#
User-generated content (UGC) communities benefit from integrating visual and textual information into search and recommendation (S&R). However, the progress on multimodal S&R is limited by the lack of high-quality datasets. To address this, the paper introduces Qilin, a multimodal information retrieval dataset collected from Xiaohongshu. Unlike existing datasets, Qilin offers user sessions with image-text notes, video notes, commercial notes, and direct answers, facilitating advanced multimodal neural retrieval models.
To better model user satisfaction and support analysis, Qilin collects APP-level contextual signals and user feedback. It includes user-favored answers and referred results for search requests triggering the Deep Query Answering (DQA) module, enabling training and evaluation of a Retrieval-augmented Generation (RAG) pipeline and exploration of the module’s effect on user search behavior. The paper provides findings and insights for improving S&R systems, highlighting Qilin’s contribution to multimodal content platforms with S&R services.
Key Takeaways#
Why does it matter?#
This paper introduces Qilin, a new multimodal dataset for information retrieval, addressing the scarcity of high-quality resources. It offers diverse data including user sessions and APP-level contextual signals, enabling the development of advanced S&R systems. Qilin’s insights and resources can significantly impact future research and industry innovations.
Visual Insights#
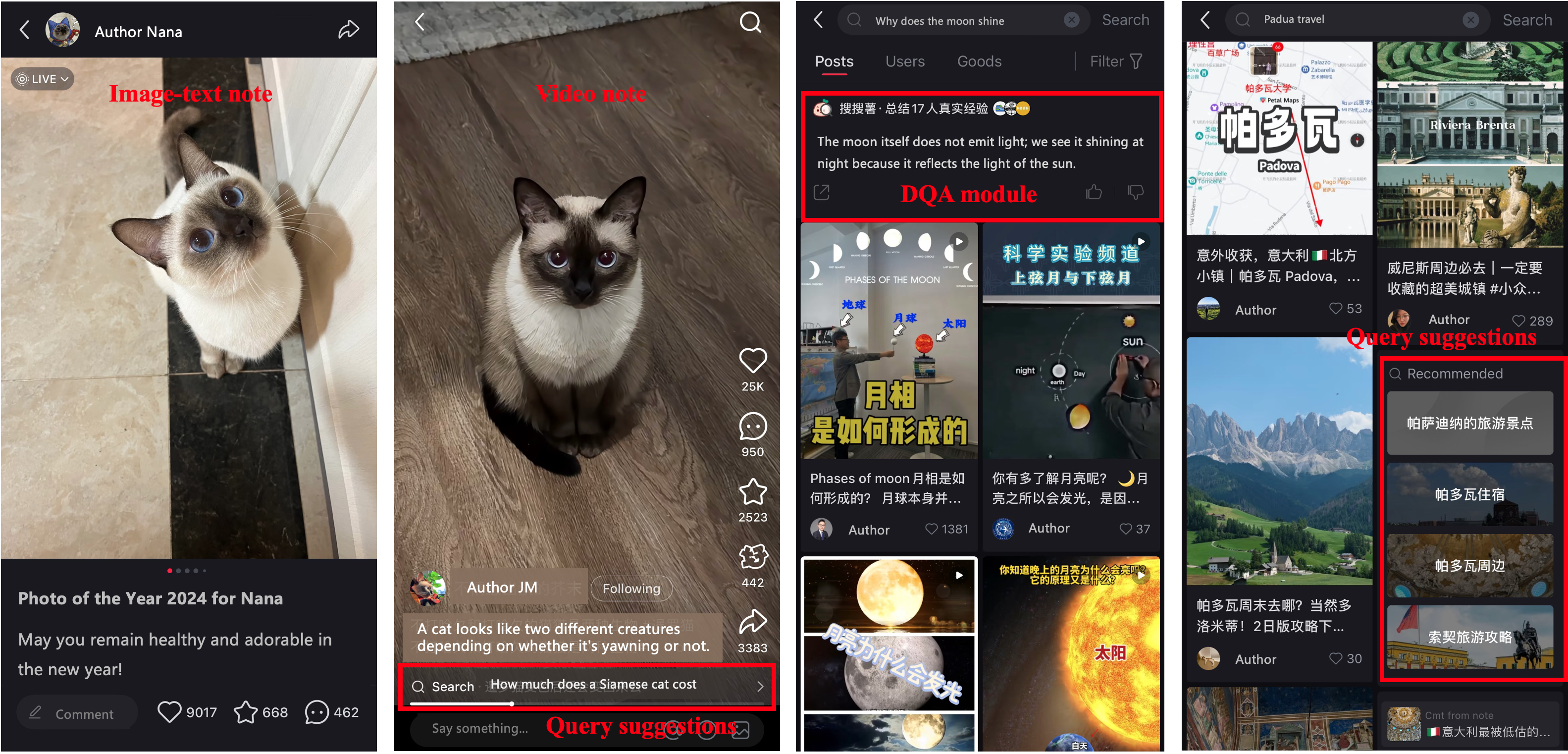
🔼 The figure shows Xiaohongshu’s search results page, which uses a two-column layout. The results are diverse, including image-text posts, videos, and commercial listings. A key feature is the inclusion of a Deep Query Answering (DQA) module that offers direct answers to user queries, in addition to the standard list of results. Also shown are various modules designed to suggest new topics and encourage further searches from users.
read the caption
Figure 1. Xiaohongshu leverages a two-column result list for S&R services, retrieving heterogeneous results like image-text, video, and commercial notes. The search system is equipped with a DQA module to provide direct answers for users. There are also various modules to stimulate users to search for any topics they might be interested in.
MODEL: Qwen2.5-14B-Instruct (Qwen2-VL-7B-Instruct) |
PROMPT_PREFIX: Imagine you are a content safety reviewer for text (or images). Please examine the following text (or image) and assess whether it contains any illegal or sensitive information: 1. Pornographic/Obscene: Includes explicit sexual descriptions, obscene language, etc.; 2. Violence: Includes bloody, terrifying, extreme violence, etc.; 3. Political Figures: Mentions or discusses information related to political figures; 4. Private Information of Ordinary Individuals: Involves privacy such as names, identifiers, contact information, addresses, etc.; 5. Portraits: Includes any real human faces. Note that the face of a virtual character (e.g., animation, comic, or game roles) is not a portrait; Additional Notes: Advertising content does not count as illegal or sensitive information. Mentioning public figures (such as actors, singers, entrepreneurs, etc.) does not count as illegal or sensitive information unless they are political figures. Return True only if the text clearly contains any of the specified illegal or sensitive content; otherwise, return False. Any emoji is not pornographic content. Normal romantic-related content or sexual education content is not considered pornographic; but if the content is overly explicit or detailed, then it counts. Expressions of negative emotions, when not coupled with violent content, should not be classified as acts of violence. Do not be too strict, as minor issues being marked as illegal or sensitive can result in false positives. If you are uncertain, it is acceptable to refrain from labeling the content as illegal or sensitive. Only when you are very sure, mark it as a positive instance and return True. Text (Image) to be checked: [ |
PROMPT_SUFFIX: ] Please only return True or False without any explanations. |
🔼 This table details the prompts used for filtering textual and image data in the Qilin dataset. The prompts are designed to identify and remove inappropriate content from the dataset, which includes identifying instances of pornography, violence, references to political figures, personal information, and real human faces. Different prompts are used for text and images, reflecting the unique ways each data type can be inappropriate. The prompts are aimed at providing explicit instructions for LLMs (Large Language Models) to accurately classify content while balancing accuracy and minimizing false positives.
read the caption
Table 1. Prompts used for filtering textual and image data.
In-depth insights#
Multimodal S&R#
Based on the provided research paper, ‘Multimodal S&R’ seems crucial for modern information retrieval, especially in user-generated content platforms. The paper addresses the deficiency in existing datasets, which often lack the multimodal information needed for advanced search and recommendation systems. Qilin, the dataset introduced, aims to bridge this gap by incorporating diverse data types like image-text notes and videos, which facilitates the development of neural retrieval models. The ability to analyze user behavior at the application level, considering factors such as session search and query suggestion modules, offers a holistic view of user engagement. This is vital as traditional methods might not suffice in scenarios where users interact with heterogeneous functional modules, like those incorporating deep query answering techniques. Exploring user intent understanding and cross-modal matching is vital and the complexity Qilin brings is beneficial for advancing multimodal systems.
APP-level Tracks#
The concept of “APP-level Tracks” in the context of multimodal information retrieval is intriguing. It suggests focusing on user behavior within a specific application, analyzing not just individual queries but the sequence of interactions, time spent, and features used. This approach allows for a more holistic understanding of user intent and satisfaction. By tracking how users navigate and interact with various app features, including search, recommendation, and DQA modules, it is possible to uncover patterns and preferences that would be missed by analyzing isolated search sessions. Furthermore, the data helps to model user state transitions, revisits, and query reformulations thereby significantly improving user’s search experiences.
DQA Influence#
The paper highlights the growing importance of Deep Query Answering (DQA) modules in modern search engines. These modules, which employ Retrieval-Augmented Generation (RAG) pipelines, provide users with succinct, direct answers. While DQA modules enhance user experience, their influence on user satisfaction and retention remains underexplored. Presenting direct answers can significantly alter user browsing behavior, potentially leading to increased focus on top results and reduced interaction with organic listings. To address this gap, the paper introduces the Qilin dataset, which includes user feedback on DQA modules and contextual behaviors, enabling researchers to evaluate the impact of RAG on user-perceived experience and explore how DQA affects user search patterns. This exploration is key to optimizing search strategies in the era of increasingly sophisticated AI-powered answer engines, and for future research.
Qilin Dataset#
The Qilin dataset, a multimodal information retrieval resource with APP-level user sessions, appears to be a valuable contribution to the field. Its focus on user-generated content (UGC) from a popular social platform distinguishes it from existing datasets, which often lack the richness and heterogeneity of real-world user interactions. The dataset’s comprehensive collection of user sessions, encompassing diverse result types like image-text notes, video notes, commercial notes, and direct answers, enables the development of advanced multimodal neural retrieval models. Moreover, the inclusion of APP-level contextual signals and genuine user feedback allows for a deeper understanding of user satisfaction and behavior. The availability of user-favored answers and referred results for search requests triggering the Deep Query Answering (DQA) module opens up opportunities for training and evaluating Retrieval-augmented Generation (RAG) pipelines and exploring the impact of such modules on user search behavior. The dataset’s potential to advance multimodal content platforms with S&R services is significant.
Diverse Behaviors#
Analyzing diverse user behaviors within information retrieval systems reveals nuanced interaction patterns. Variations in search query formulation, influenced by user expertise and task complexity, significantly impact retrieval effectiveness. Understanding these behaviors can help in optimizing retrieval strategies and adapting interfaces to cater to different user needs. Session-level analysis provides further insights into user intent and satisfaction, enabling the development of personalized retrieval experiences. Recognizing diverse search approaches is crucial for improving relevance and user engagement in information retrieval systems. Furthermore, APP-level behaviors can also reveal insight on user engagement and result satisfaction that can inform strategies on improving the recommendation and search results.
More visual insights#
More on figures
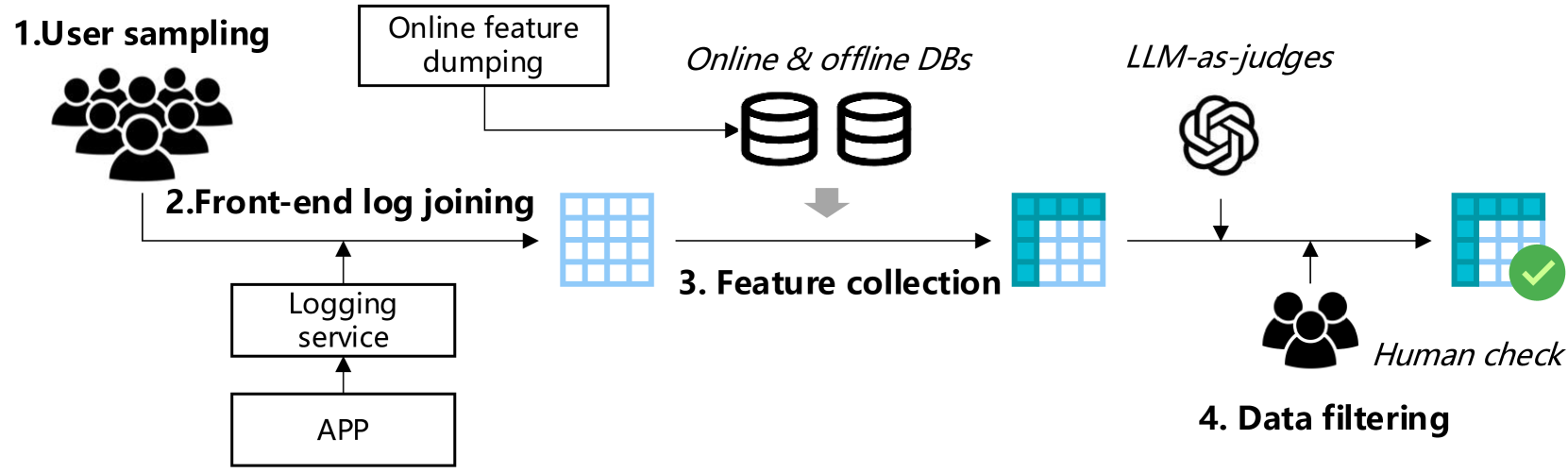
🔼 The figure illustrates the pipeline for creating the Qilin dataset. It begins with sampling user IDs from Xiaohongshu’s database. These IDs are then linked to the platform’s frontend logs to build the dataset’s foundation. Next, features are extracted from different databases: request details, user profiles, and note attributes (including image and text information). A crucial step is the filtering process, employing Large Language Models (LLMs) and manual expert review to eliminate unsafe or inappropriate content, resulting in a clean, high-quality multimodal dataset.
read the caption
Figure 2. The data construction process of Qilin. The front-end log is joined with sampled user IDs to obtain the dataset backbone. Then we collect features for the request, user, and note from various databases. Finally, all content features undergo rigorous filtering by LLMs and human experts.
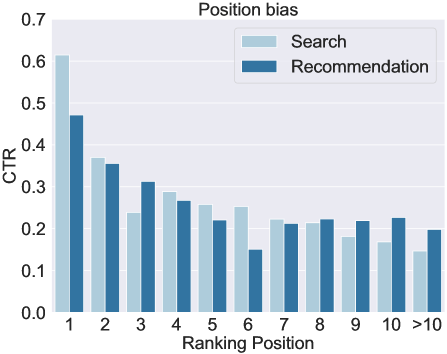
🔼 This figure shows the click-through rate (CTR) for search and recommendation results across different ranking positions. It illustrates the well-known ‘position bias,’ where higher-ranked results tend to receive more clicks. The figure also shows that the decay in CTR with ranking position is slower for recommendations than for search results. This difference is likely due to different user browsing behaviors in these two scenarios.
read the caption
(a) Position bias.

🔼 This figure shows the click-through rate (CTR) for search and recommendation results across multiple sessions. It demonstrates that CTR decreases as the session progresses, indicating a session bias effect. This means users are less likely to click on results later in their session, perhaps due to fatigue or satisfaction.
read the caption
(b) Session bias.
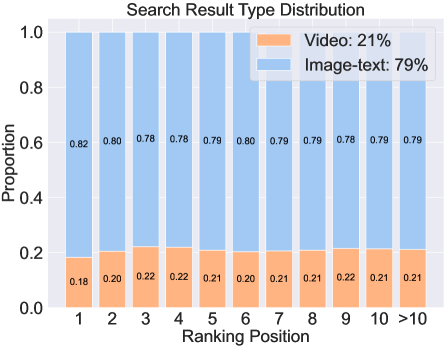
🔼 This figure shows the distribution of different result types (image-text and video notes) across ranking positions in search scenarios. The x-axis represents the ranking position, and the y-axis represents the proportion of each result type. The figure visualizes how the proportion of video notes and image-text notes varies across different positions in the search results.
read the caption
(c) Search result distribution.
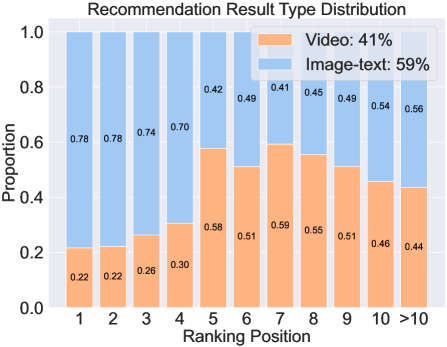
🔼 This figure shows the distribution of video and image-text notes in recommendation results across different ranking positions. The x-axis represents the ranking position, and the y-axis represents the proportion of each note type. It illustrates how the prevalence of video and image-text notes changes depending on where they appear in the recommendation list.
read the caption
(d) Recommendation result distribution.
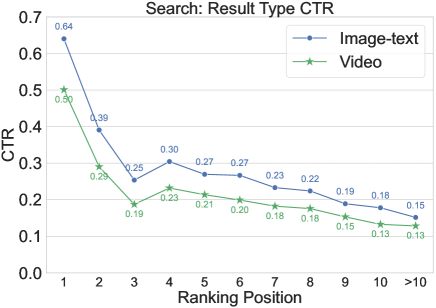
🔼 This figure shows the click-through rate (CTR) for search results plotted against ranking positions. The CTR shows how often users click on a result given its position. It demonstrates the relationship between the position of a search result on the page and the probability that it will be clicked. This helps understand position bias in search results (the tendency for higher-ranked results to receive more clicks regardless of relevance). The figure also shows the distribution of CTR for different types of search results (image-text and video notes).
read the caption
(e) Search result CTR.

🔼 This figure shows the click-through rate (CTR) for image-text and video notes across different ranking positions in recommendation scenarios. It illustrates how the CTR varies depending on the position of the result (position bias) in the recommendation list.
read the caption
(f) Recommendation result CTR.
More on tables
Property | Amazon | JD Search | KuaiSAR | Qilin |
# Users | 192,403 | 173,831 | 25,877 | 15,482 |
# Items | 63,001 | 12,872,736 | 6,890,707 | 1,983,938 |
# Queries | 3,221 | 171,728 | 453,667 | 57,1881 |
# Actions | 1,689,188 | 26,667,260 | 19,664,885 | 2,498,594 |
# Images2 | ? | – | – | 5,006,181 |
DQA info | ||||
Item text | title+review | anon’d | anon’d | title+body |
🔼 This table compares the Qilin dataset with three other existing datasets used for Search and Recommendation (S&R) tasks: Amazon, JD Search, and KuaiSAR. It highlights key differences in the number of users, items, queries, and actions recorded. Importantly, it notes that Amazon uses pseudo-queries (not real user queries), while JD Search and KuaiSAR provide only anonymized item content (not the actual item text). Qilin’s distinction is emphasized: it uniquely includes user actions related to the Deep Query Answering (DQA) module, offering a more complete picture of user interactions.
read the caption
Table 2. Comparison between Qilin and existing S&R datasets. Note that queries in Amazon are pseudo ones. JD Search and KuaiSAR only provide anonymized item content. Besides S&R, Qilin also include user actions on DQA.
Table | Key | Fields |
Search | search id | query, session id, user id, 20 recently clicked note ids, query source (1-8), search result details (list, each element is encapsulated as {note id, position, timestamp, six engage labels}, sorted by ascending timestamp), DQA details (if triggered); |
Rec | request id | session id, user id, 20 recently clicked note ids, recommendation result details (a list of encapsulated elements, ditto); |
DQA | search id | all search fields, answer content, referred note ids, four user engage labels; |
User | user id | gender, platform, age, fan number, follow number, 40 encrypted dense features; |
Note | note id | note type, note title, note content, image id list1, video duration, video height, video width, image num, content length, commercial flag, 1/2/3-level taxonomy ids, 30 statistical features (e.g., the number of monthly impressions or clicks); |
🔼 Table 3 presents a detailed schema of the Qilin dataset, outlining the structure and contents of each data field. It breaks down the dataset into key tables (search, recommendation, DQA, user, and note), detailing the attributes and data types within each table. This allows researchers to understand the organization and content of the data for improved usage and analysis.
read the caption
Table 3. Data schema of Qilin.
Scenario | Tasks |
Search | CTR prediction, click simulation, unbiased learning to rank, multimodal search, context-aware ranking, session search, (post) pre-training for web search, enhancing search by recommendation, pre-training for RAG, evaluation for RAG, multimodal RAG, query performance prediction, etc; |
Rec | content-based recommendation, multimodal recommendation, session-based recommendation, unbiased recommendation, query (intent) recommendation, enhancing recommendation by search, etc; |
General | multi-task learning, multi-scenario learning, heterogeneous user behavior analysis, multimodal alignment, multimodal LLM-as-judges, scaling laws fitting, etc; |
🔼 This table lists various potential research tasks enabled by the Qilin dataset. It categorizes these tasks by the type of retrieval system they apply to (Search, Recommendation, or General) and provides examples of specific research questions that could be addressed using Qilin’s data. This includes tasks related to ranking model training, bias mitigation, user behavior analysis, and multimodal learning.
read the caption
Table 4. Potential tasks that Qilin supports.
S | R | S-DQA | S+DQA | |
Avg. browsing depth | 22.75 | 18.80 | 23.41 | 10.61 |
Avg. first click rank | 3.01 | 4.97 | 3.03 | 2.50 |
Avg. click num | 3.88 | 3.67 | 3.99 | 2.50 |
Click-through rate | 21.01% | 24.13% | 21.02% | 20.73% |
Like rate | 4.11% | 7.07% | 4.19% | 1.29% |
Collect rate | 1.87% | 1.47% | 1.88% | 1.26% |
Share rate | 0.57% | 0.63% | 0.57% | 0.52% |
Comment rate | 0.32% | 0.99% | 0.32% | 0.21% |
# Samples | 57,188 | 94,552 | 54,256 | 2,932 |
🔼 This table presents a comparison of user engagement metrics across different search scenarios within a mobile application. The scenarios are categorized by the presence or absence of interactions with a Deep Query Answering (DQA) module. Metrics include average browsing depth, average click position, click-through rate, and various engagement rates (like, collect, share, comment). Engagement rates are calculated as conditional probabilities, showing the likelihood of an engagement action given a click has already occurred. This allows for a nuanced analysis of user behavior in relation to the DQA module and different search paradigms (with and without recommendations).
read the caption
Table 5. User engagements across scenarios, where S-DQA and S+DQA denote search requests without or with triggering the DQA module. Note that engagement rates such as like rate and collect rate are calculated based on the conditional probability P(engage=1|click=1)𝑃𝑒𝑛𝑔𝑎𝑔𝑒conditional1𝑐𝑙𝑖𝑐𝑘1P(engage=1|click=1)italic_P ( italic_e italic_n italic_g italic_a italic_g italic_e = 1 | italic_c italic_l italic_i italic_c italic_k = 1 ).
SS | RS | RR | SR | |
Proportion | 34.88% | 2.95% | 59.32% | 2.84% |
Avg. click num | 4.0014 | 3.9782 | 2.9745 | 4.4466 |
Click-through rate | 20.18% | 22.98% | 23.20% | 25.01% |
# Samples | 29,295 | 2,476 | 49,815 | 2,387 |
🔼 This table presents an analysis of user transitions between search (S) and recommendation (R) services within a mobile application. It shows the percentage of transitions between different service combinations (S→S, R→S, R→R, S→R), along with the average number of clicks and click-through rates for each transition type. The data reveals user behavior patterns related to switching between search and recommendation functionalities within the app.
read the caption
Table 6. User transition analysis. S and R stand for search and recommendation, respectively.
Proportion | CTR | Avg. click num | |
Length¡=3 | 0.32% | 0.1967 | 2.9892 |
Length=4 | 13.64% | 0.1962 | 4.0276 |
Length=5 | 13.94% | 0.2047 | 3.9303 |
Length=6 | 17.27% | 0.2051 | 4.0338 |
Length=7 | 14.07% | 0.2111 | 3.9446 |
Length=8 | 12.42% | 0.2150 | 3.8180 |
Length=9 | 9.54% | 0.2199 | 3.6971 |
Length=10 | 6.89% | 0.2272 | 3.7497 |
Length¿10 | 11.91% | 0.2216 | 3.7010 |
🔼 This table shows the distribution of query lengths (number of characters) in the Qilin dataset and how user engagement metrics, specifically click-through rate (CTR) and average click number, vary across different query lengths. The color intensity of the cells visually represents the magnitude of the CTR and average click number. Deeper colors indicate higher values for both metrics, providing a quick visual comparison of user engagement for different query lengths.
read the caption
Table 7. Query length distribution and corresponding user engagement. Note that a deeper color denotes a higher value of CTR or average click number.
Type | Prop. | Examples | ||
Add | 13.57% |
| ||
Delete | 2.53% |
| ||
Change | 47.16% |
| ||
Repeat | 7.57% |
| ||
Others | 29.17% |
| ||
🔼 This table showcases examples of various query reformulation types observed in user search sessions. It categorizes these reformulations into five types: Add (adding words), Delete (removing words), Change (modifying words), Repeat (repeating the same query), and Others (all other reformulation types). For each type, representative examples from the dataset are provided to illustrate the different ways users modify their search queries. The total number of reformulation actions included in the dataset is 29,875.
read the caption
Table 8. Examples of different query reformulation types. The total number of reformulating actions is 29,875.
广州批发广州批发市场 (Guangzhou wholesaleGuangzhou wholesale market) |
五大文明五大文明发源地 (the five great civilizationsthe birthplaces of the five great civilizations) |
🔼 This table details the various sources from which user queries originate within the Xiaohongshu app. It lists eight categories of query sources, provides a concise definition for each, and presents the proportion of queries stemming from each source. This allows for an analysis of user behavior and how different entry points into the search function influence query patterns and overall search experience.
read the caption
Table 9. Query source definition and analysis.
ip设计插件ip设计 (intellectual property designing pluginintellectual property design) |
农业经营模式分析农业经营模式 (analysis of agricultural business modesagricultural business modes) |
🔼 This table presents a comparison of the performance of several different approaches for search and recommendation tasks. The approaches include various methods such as BM25, BERT (both bi-encoder and cross-encoder versions), DCN-V2, and a Visual Language Model (VLM). Performance is measured using Mean Reciprocal Rank (MRR) at 10 and 100, and Mean Average Precision (MAP) at 10 and 100. The results show the effectiveness of different models in ranking search results and recommending items to users, illustrating the impact of factors like visual information and model architecture.
read the caption
Table 10. Comparison of search and recommendation performances on various approaches.
文案破碎感文案自由松弛感 (writing sense with vulnerabilitywriting sense with freedom and relaxation) |
将数据分三分位四分位间距 (dividing data into tertilesInterquartile Range, IQR) |
🔼 This table compares the performance of different Large Language Models (LLMs) in a Retrieval-Augmented Generation (RAG) pipeline. The RAG pipeline uses retrieved documents to aid in the generation of answers. The table shows the performance of each LLM using four different retrieval methods: no retrieval, retrieval with BM25, retrieval with BERT bi-encoders, and retrieval using the ground truth documents from the Qilin dataset (Oracle). Performance is measured using ROUGE-L and BERTScore (F1), which assess the quality of the generated answers.
read the caption
Table 11. Comparison of Retrieval-augmented generation (RAG) performance across various LLMs.
Full paper#
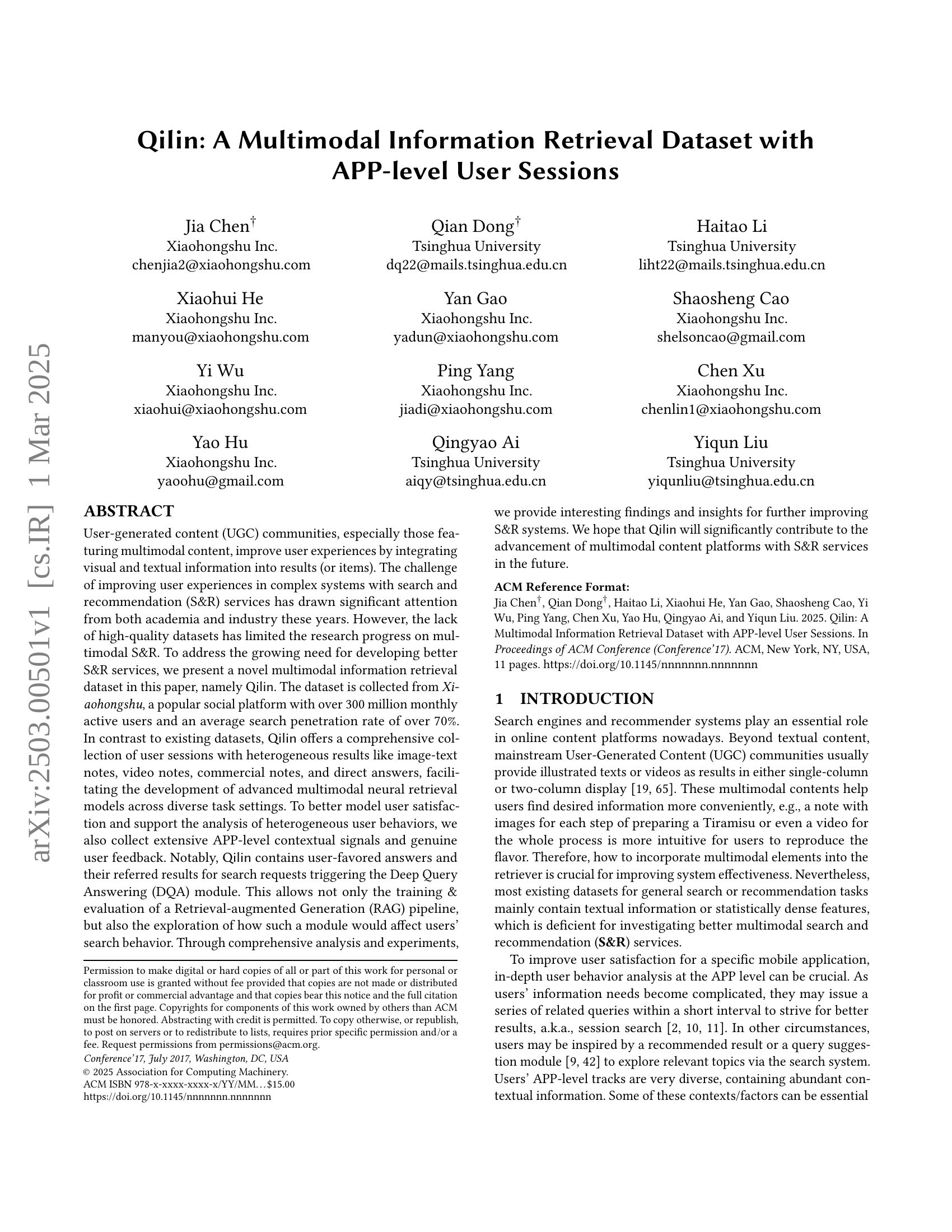
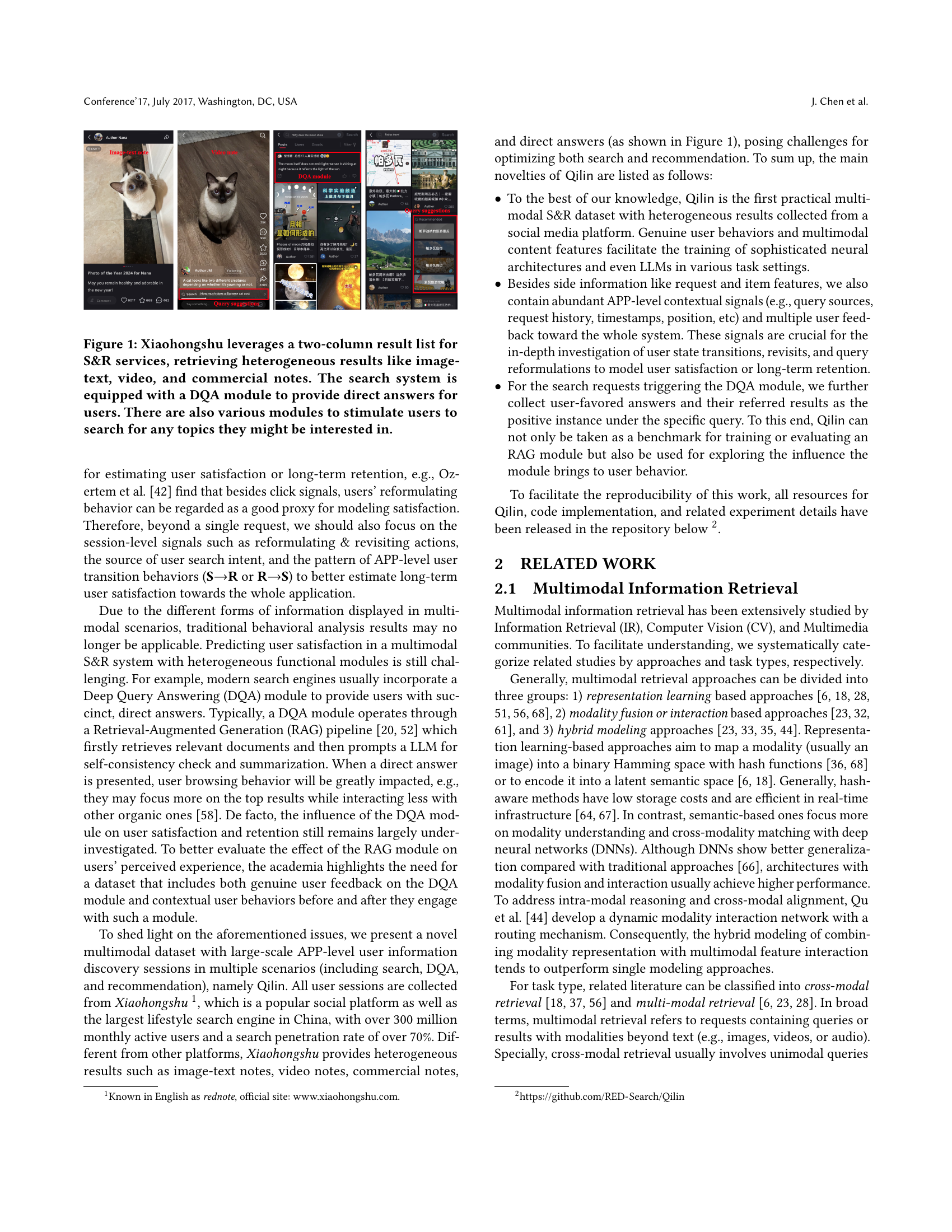
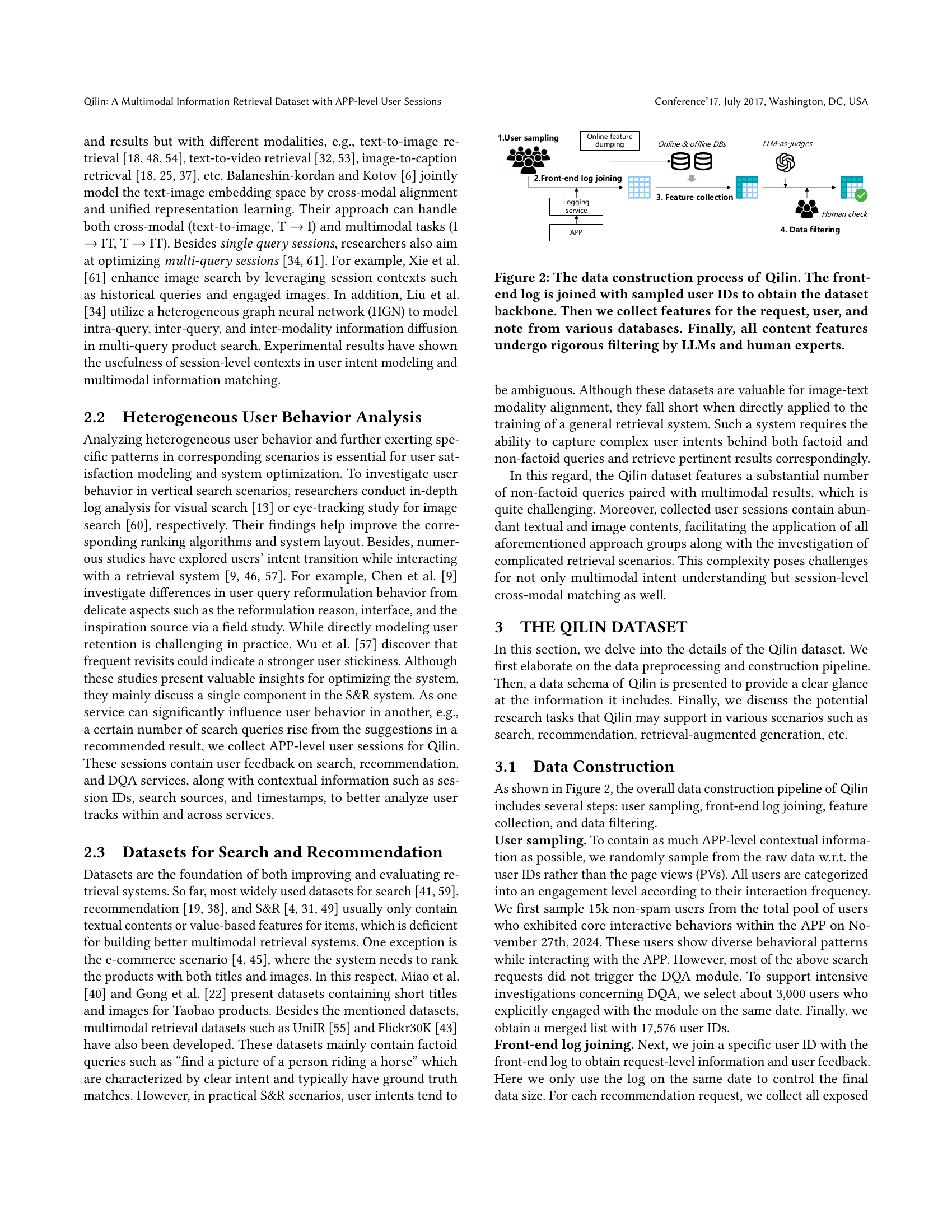
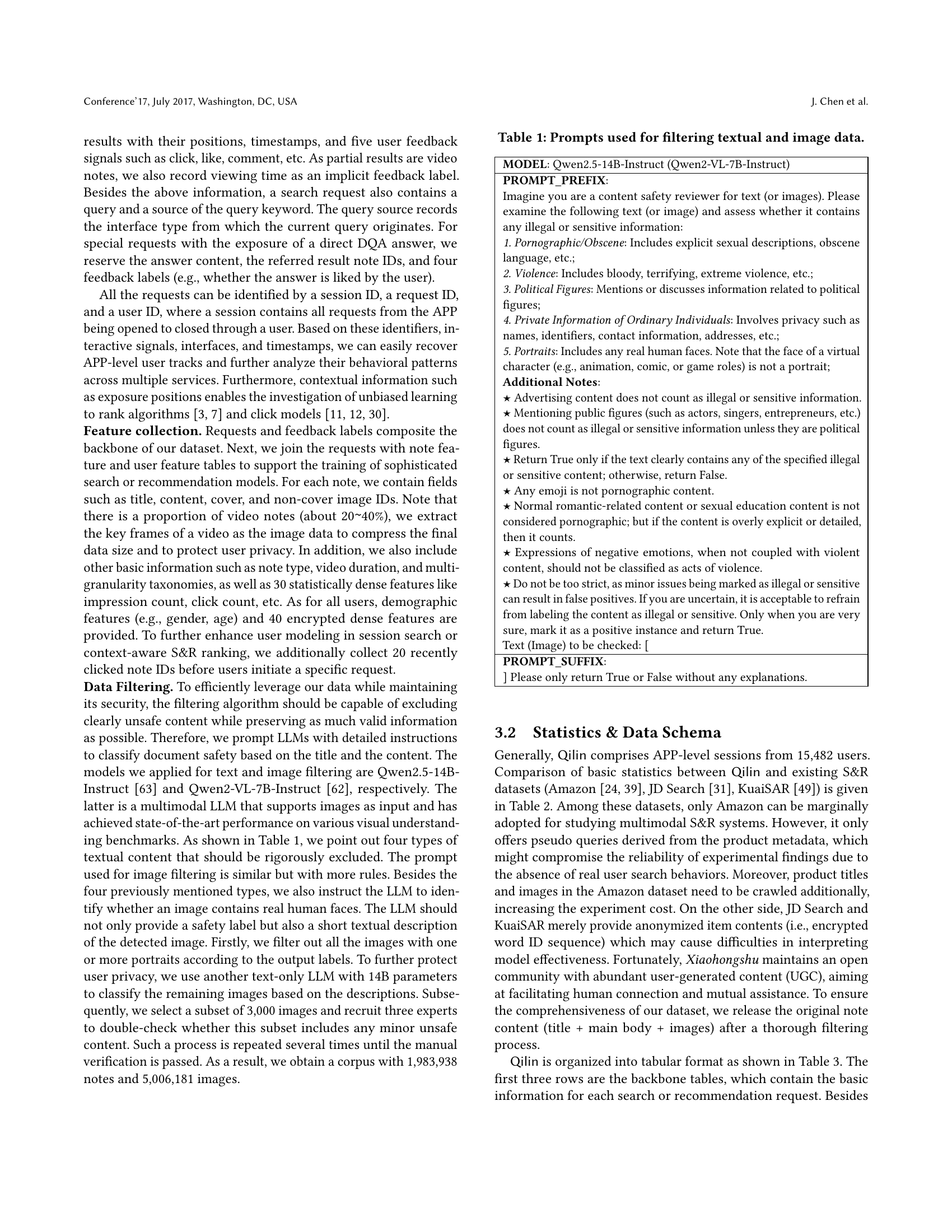
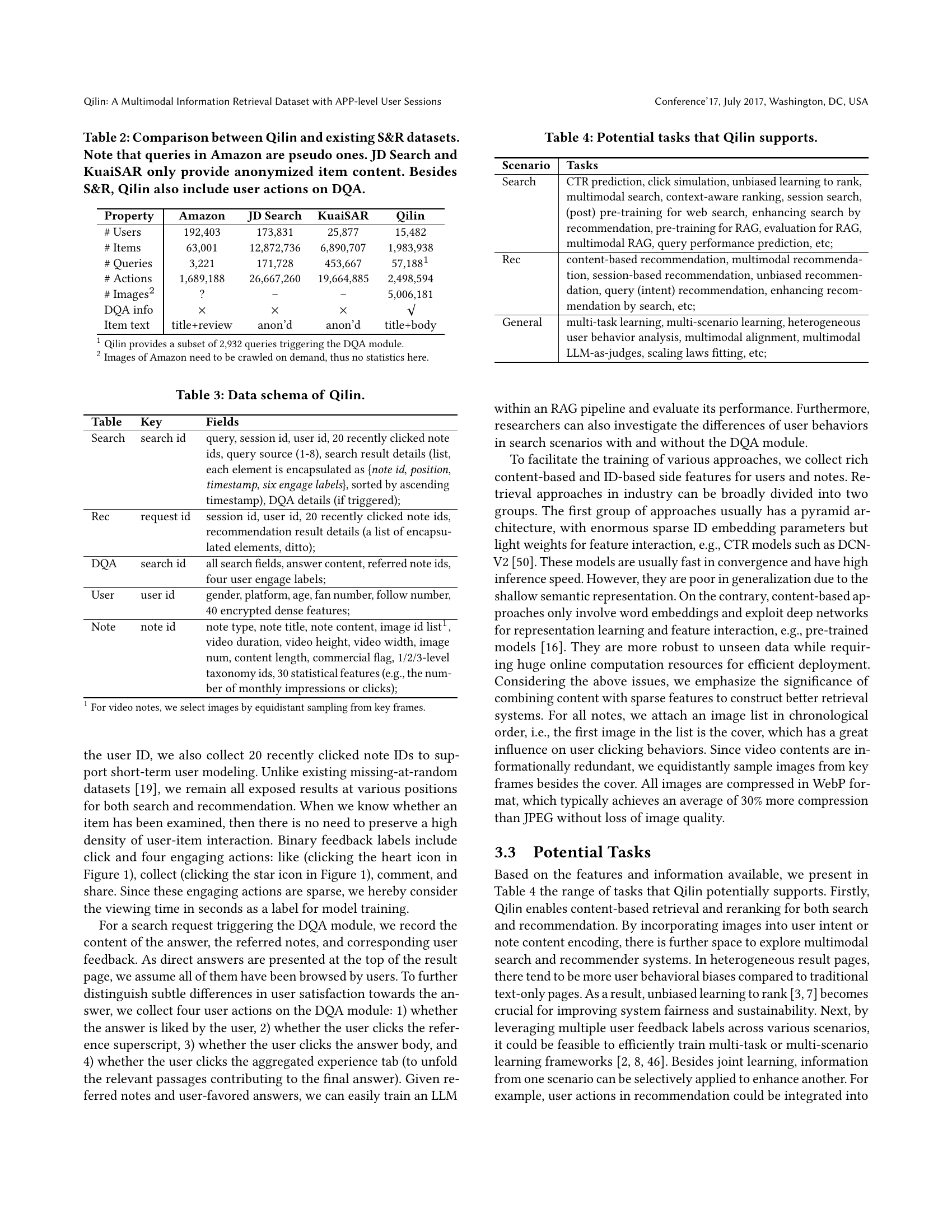
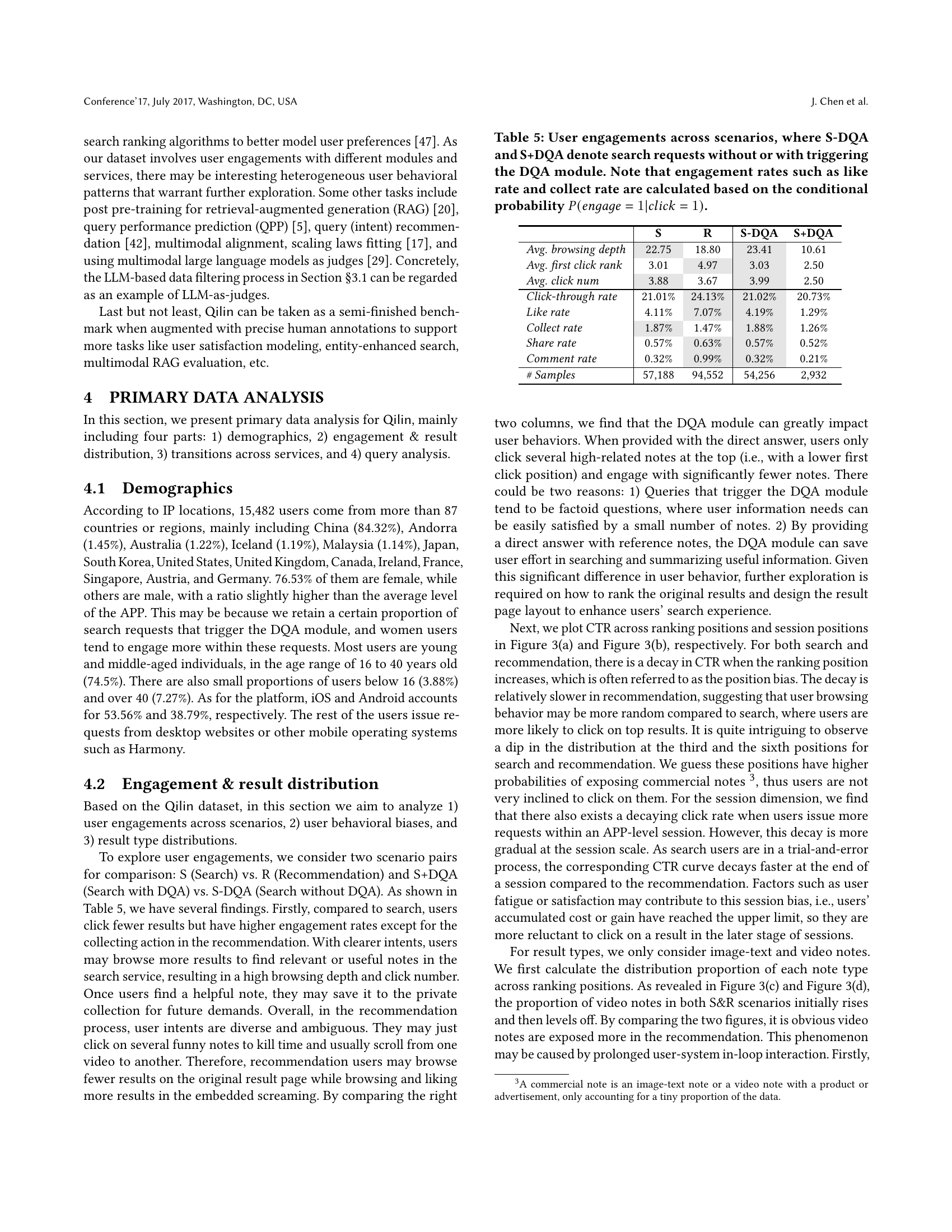
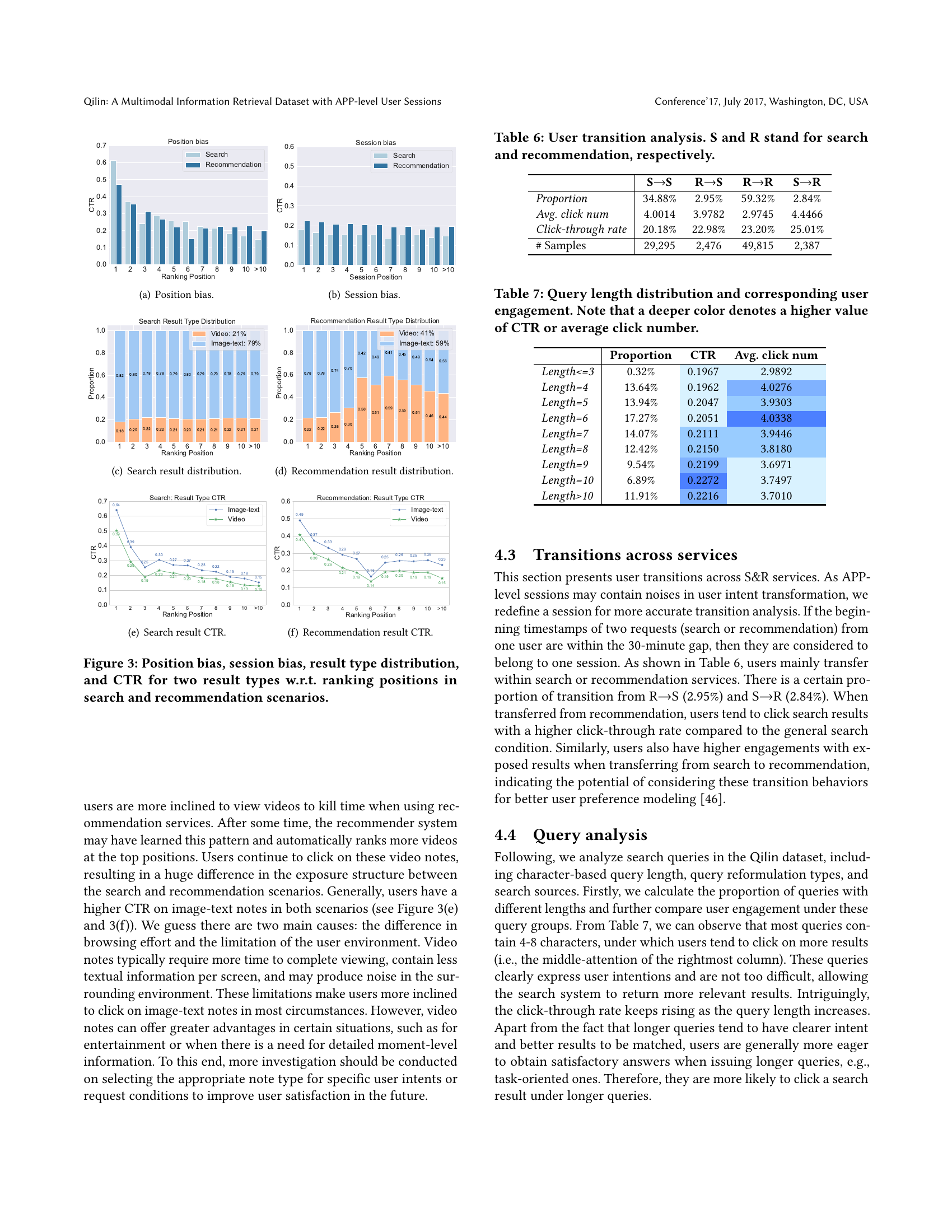
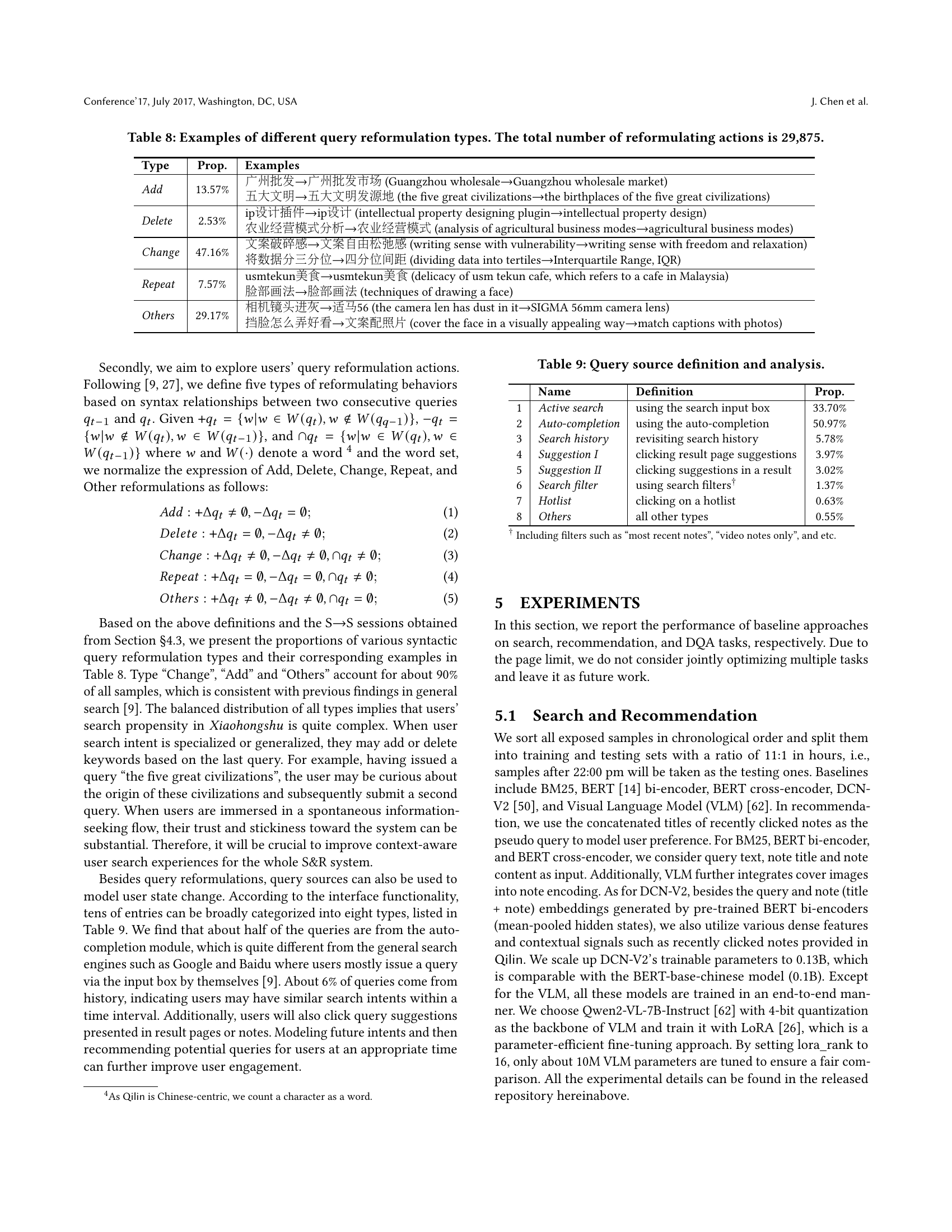
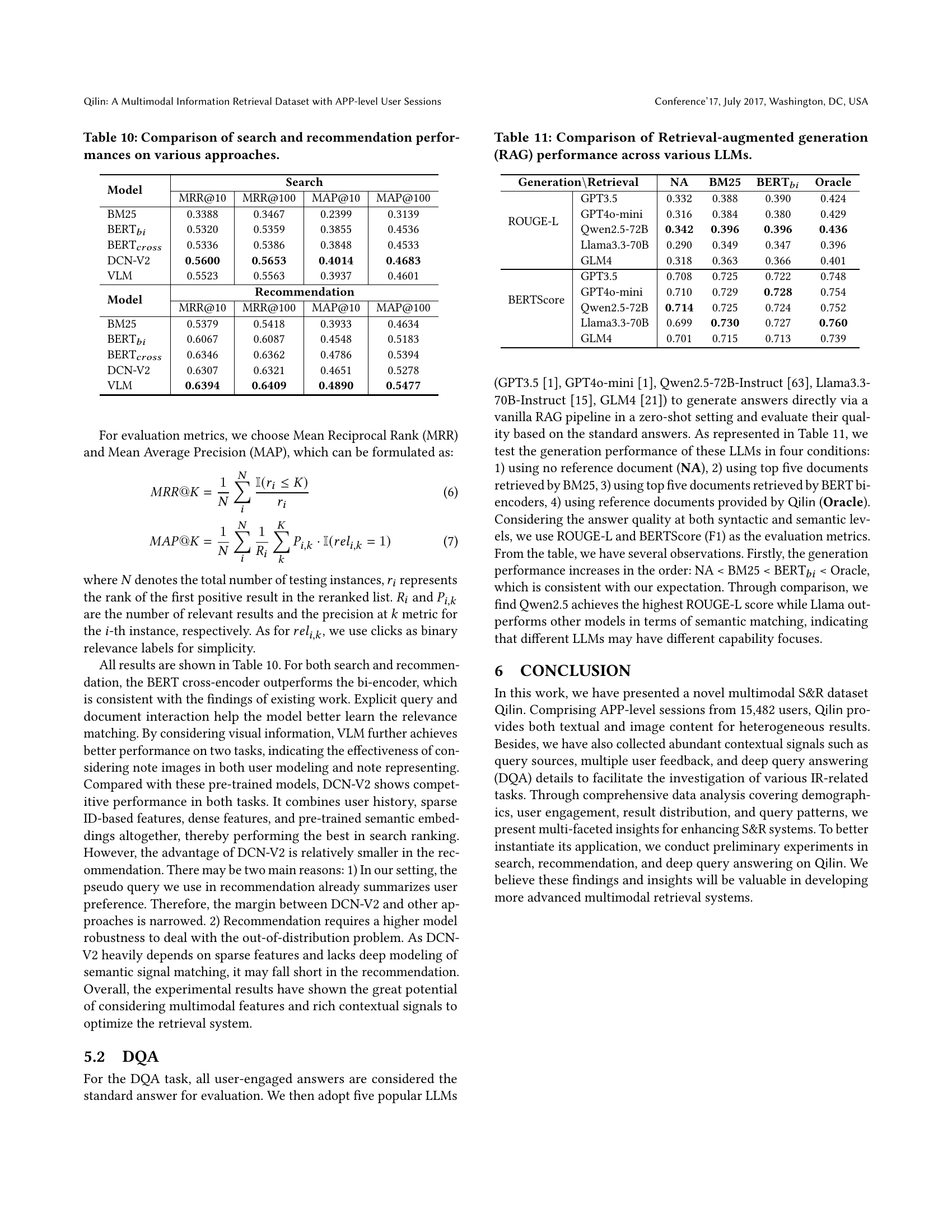
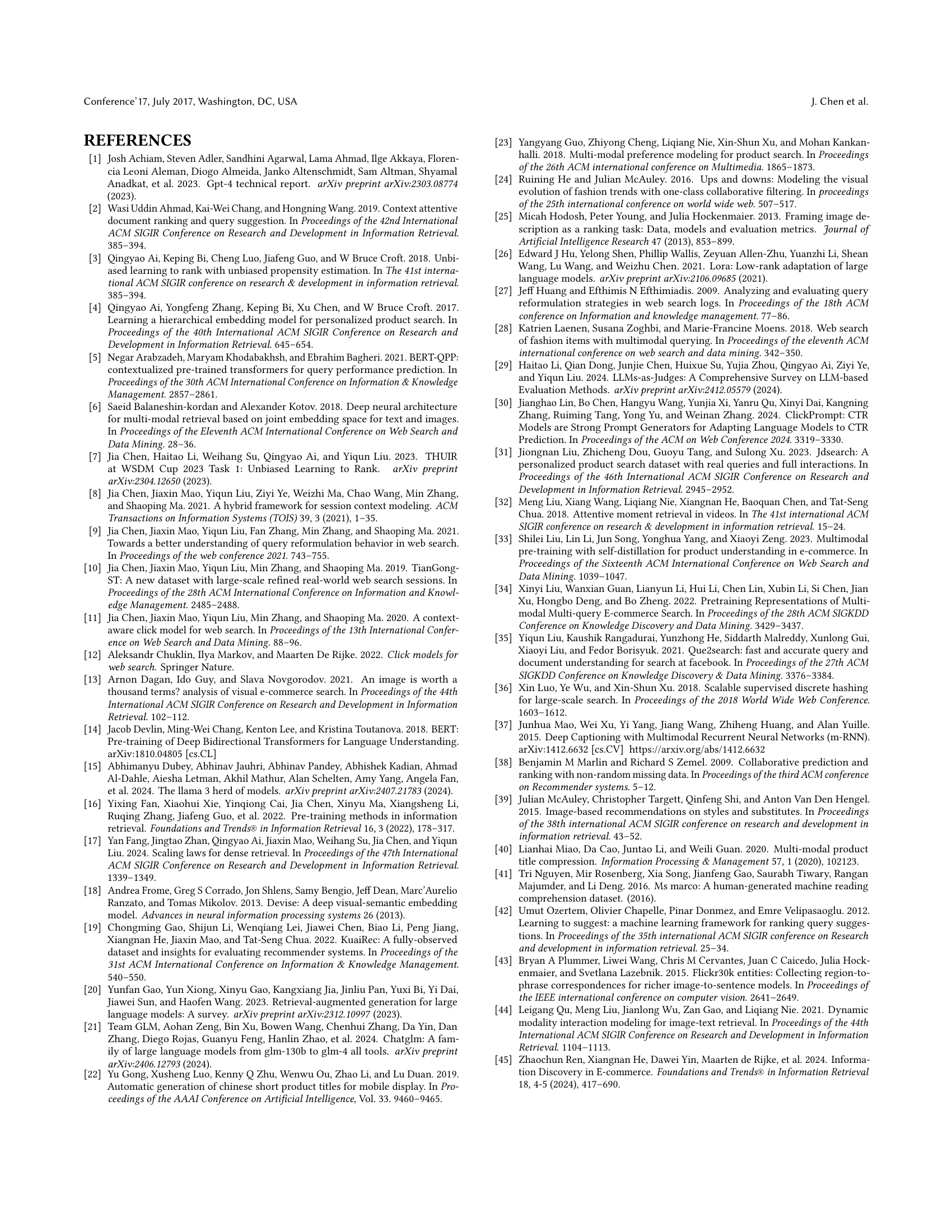
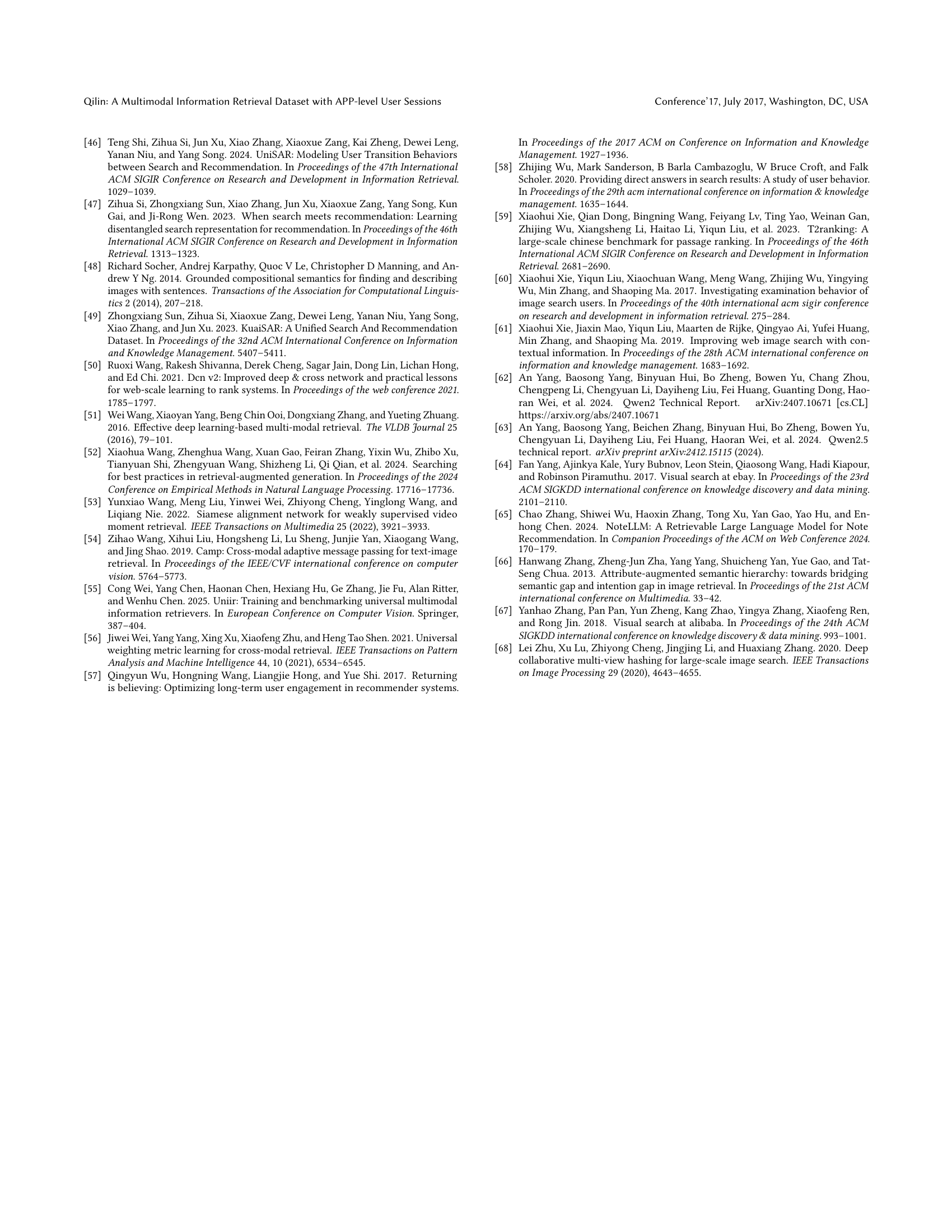