TL;DR#
Current text-to-video models fall short of user expectations because they aren’t trained on relevant topics. Existing video datasets often lack alignment with real-world user interests. To address this, the paper introduces the VideoUFO dataset, which is specifically curated to focus on user preferences. It also features minimal overlap with existing datasets and uses Creative Commons licensed videos.
The paper identifies 1,291 user-focused topics, retrieves related YouTube videos, segments them into clips, generates captions, and verifies content. Experiments show that current models struggle with certain topics. A model trained on VideoUFO outperforms others in these areas, demonstrating the dataset’s value in enhancing text-to-video generation quality.
Key Takeaways#
Why does it matter?#
This work introduces VideoUFO, a new video dataset aligned with user interests, filling a gap in existing resources. It enables more relevant and effective text-to-video generation, addressing the limitations of current models and opening new research directions by providing high-quality, focused training data.
Visual Insights#
🔼 The figure shows a comparison between a firefly image generated by the Sora text-to-video model and a real-world firefly image. The generated firefly appears noticeably different from the real one, highlighting the limitations of current text-to-video models when dealing with topics (like glowing fireflies) not adequately represented in their training data. The model struggles to accurately represent the visual characteristics of the firefly, suggesting a need for datasets with broader topic coverage.
read the caption
Figure 1: The glowing firefly: (a) generated by Sora [2] and (b) captured in a real video. The generated firefly is noticeably different from its real-life counterpart and thus unsatisfying. We attribute this primarily to a lack of exposure to such topics.
In-depth insights#
User-Focused Data#
User-focused data in text-to-video generation emphasizes the need for datasets that reflect real-world user interests, addressing a key gap where current models often fall short of expectations. By curating data based on user-generated prompts, specifically leveraging platforms like YouTube with Creative Commons licenses, a user-focused approach aims to improve the alignment between generated content and actual user needs. The significance lies in creating a training dataset that prioritizes topics and aesthetics relevant to users, leading to more satisfying and applicable video outputs. Minimal overlap with existing datasets becomes crucial, ensuring that models trained on user-focused data gain new knowledge rather than simply reprocessing old information. Moreover, compliance with data usage regulations, achieved through Creative Commons licensing, ensures greater flexibility and accessibility for researchers.
VideoUFO vs Others#
When comparing VideoUFO to other video datasets, several key distinctions emerge. VideoUFO is curated with a specific focus on aligning with real user interests in text-to-video generation, setting it apart from datasets gathered from open-domain sources that may lack this user-centric approach. Additionally, VideoUFO introduces novel data obtained directly from YouTube, contrasting with datasets that primarily reprocess existing data. Moreover, VideoUFO prioritizes data compliance by exclusively using videos with Creative Commons licenses, offering researchers greater freedom in utilizing the data, an element often overlooked in other recent datasets. This strategic focus on user needs, new data, and compliance differentiates VideoUFO, making it a valuable resource for advancing text-to-video models that truly cater to user expectations.
BenchUFO Design#
BenchUFO design likely focuses on creating a benchmark for text-to-video models. It will probably involve selecting diverse prompts, generating videos using these prompts, and evaluating the quality of the generated videos. Key considerations would include the choice of evaluation metrics to accurately measure the alignment between the text prompt and the generated video content, diversity of prompts and prompt sources to fairly evaluate generalizability, the choice of the text-to-video generation model, and the resources required for the analysis.** The goal is to analyze effectiveness to generate videos that contain user-focused topics.
Topic Coverage Gap#
Analyzing the topic coverage gap reveals a critical disparity between existing video datasets and the actual focus of users in text-to-video generation. Current datasets, often sourced from broad, open-domain content, fail to adequately represent the specific topics users actively seek when creating videos. This misalignment results in text-to-video models that underperform in real-world scenarios because they are not trained on relevant data. The paper emphasizes the need for datasets curated with a user-centric approach to bridge this gap, ensuring models are exposed to a wider variety of niche interests and emerging trends. This gap highlights a need in more diverse training data to cover real user interests to improve model performance on realistic topics. VideoUFO is designed specifically to close this gap by collecting videos according to real users’ preferences and generating appropriate captions.
Towards Realism#
The push towards realism in text-to-video generation is a crucial step in bridging the gap between current model outputs and user expectations. The paper highlights Sora’s shortcomings in accurately depicting real-world phenomena like glowing fireflies, indicating a need for more realistic training data. A dataset curated to align with ‘users’ focus,’ as opposed to generic open-domain datasets, directly addresses this. Realism extends beyond visual fidelity; it encompasses contextual accuracy and capturing intricate details specific to user intents. Improving realism inherently enhances the applicability of generated videos across diverse sectors like film, gaming, education, and advertising, ultimately leading to more satisfying and useful creative outcomes. The paper’s exploration of user preferences is pivotal for moving away from creating impressive yet often disconnected content towards more meaningful and relatable video generation.
More visual insights#
More on figures
🔼 VideoUFO is the first dataset created specifically to align with real-world user interests for text-to-video generation. It contains over 1.09 million video clips covering 1,291 distinct topics identified from user prompts. The figure displays example images from the 20 most popular topics within the dataset to illustrate the diverse range of content included. Researchers can use VideoUFO to improve the performance of text-to-video models by training or fine-tuning them on this dataset.
read the caption
Figure 2: VideoUFO is the first dataset curated in alignment with real-world users’ focused topics for text-to-video generation. Specifically, the dataset comprises over 1.091.091.091.09 million video clips spanning 1,29112911,2911 , 291 topics. Here, we select the top 20202020 most popular topics for illustration. Researchers can use our VideoUFO to train or fine-tune their text-to-video generative models to better meet users’ needs.
🔼 Figure 3 visualizes the distribution of user-focused topics extracted from the VidProM dataset. It uses WizMap to represent these topics semantically, showing relationships and groupings between them. The figure is a visual representation of the clusters of user-provided prompts, illustrating the diversity and interconnectivity of the topics relevant to text-to-video generation. Due to its detailed nature, zooming in is recommended for a comprehensive view.
read the caption
Figure 3: The semantic distribution of users’ focused topics. It is visualized by WizMap [39]. Please \faSearch zoom in to see the details.
🔼 Figure 4 shows a sample data point from the VideoUFO dataset. Each data point contains a video clip, a unique ID, the topic of the video clip, the start and end timestamps of the clip within its original video, a brief caption summarizing the video, and a more detailed caption providing a more in-depth description. In addition to this textual information, VideoUFO provides six video quality scores for each clip using VBench, a video quality assessment tool. These scores evaluate aspects like subject consistency, background consistency, motion smoothness, and more.
read the caption
Figure 4: Each data point in our VideoUFO includes a video clip, an ID, a topic, start and end times, a brief caption, and a detailed caption. Beyond that, we evaluate each clip with six different video quality scores from VBench [7].
🔼 Figure 5 presents the statistical distributions of the lengths of brief captions, detailed captions, and video clip durations within the VideoUFO dataset. The histogram for brief captions shows a mean length of approximately 13.8 words. The histogram for detailed captions shows a mean length of roughly 155.5 words. Finally, a histogram of video clip durations shows an average length of around 12.6 seconds. These data provide insights into the characteristics of the text and video content within the VideoUFO dataset.
read the caption
Figure 5: The statistical information of captions and video clips in the proposed VideoUFO dataset. The average word count for brief and detailed captions is 13.813.8\mathbf{13.8}bold_13.8 and 155.5155.5\mathbf{155.5}bold_155.5, respectively, while the average clip duration is 12.612.6\mathbf{12.6}bold_12.6 seconds.
🔼 This table compares VideoUFO with other recent large-scale video datasets, highlighting key differences in their attributes. VideoUFO is distinguished by its focus on real user-centric topics derived from text-to-video prompts, unlike datasets which collect videos from open domains. Additionally, VideoUFO offers a more flexible Creative Commons license, unlike others with restrictive licenses. Despite its unique approach to data collection, VideoUFO maintains comparable video characteristics (number of videos, video length, caption length, resolution) to other prominent datasets. The abbreviations used are: ‘#’ for ’numbers’ and ‘–’ for ‘average’.
read the caption
Table 1: The comparison between the proposed VideoUFO with other recent video datasets based on their fundamental attributes. Unlike previous collections, our VideoUFO is derived directly from real user-focused topics and also offers a more flexible license, while remaining comparable to these datasets in other key aspects. “##\##” and “¯¯absent\ \bar{}over¯ start_ARG end_ARG ” are abbreviations for “numbers” and “average”, respectively.
🔼 Figure 6 is a bar chart comparing the number of user-focused topics covered by various recently published video datasets. The x-axis lists the dataset names, while the y-axis represents the count of topics covered. Each bar’s height visually indicates how many user-focused topics from the VideoUFO dataset are also present in that specific dataset. The chart highlights that no single existing dataset encompasses all the user-focused topics identified in the VideoUFO dataset. This emphasizes the novelty and unique contribution of VideoUFO, which addresses a gap in existing resources.
read the caption
Figure 6: The number of user-focused topics covered by recent video datasets. None successfully includes all user-focused topics.
🔼 The figure illustrates the BenchUFO process, a novel benchmark designed to assess the ability of text-to-video models to generate videos accurately reflecting user-focused topics. The process involves selecting 791 concrete noun topics, each represented by 10 real-world user-provided prompts. For each prompt, a text-to-video model generates a video, which is then described using a video understanding model. Finally, the similarity between the generated description and the original prompt is calculated to evaluate the model’s performance on that specific topic.
read the caption
Figure 7: The calculation process of BenchUFO. It is designed to evaluate whether a text-to-video model can effectively generate videos that contain user-focused topics. It comprises 791 concrete noun topics, each paired with 10101010 real-world user-provided prompts.
🔼 This table presents a benchmark comparing the performance of several text-to-video generation models on user-focused topics. It shows the average similarity scores between generated video descriptions and original prompts for the worst (Low N) and best (Top N) performing topics. The models compared include publicly available models trained on various datasets (both public and private) and a model (MVDiT) specifically trained on three different datasets: VidGen, OpenVid, and the authors’ VideoUFO dataset. This allows for an assessment of how well different models perform on a variety of topics, with a focus on identifying those where models struggle.
read the caption
Table 2: The performance of both publicly available text-to-video models and our trained models on the proposed BenchUFO. The publicly available models are trained on various datasets, including both public and private ones. MVDiT [20] is trained on VidGen [29], OpenVid [20], and VideoUFO, respectively. “Low/Top N𝑁Nitalic_N” denotes the average score of the worst/best-performing N𝑁Nitalic_N topics.
Full paper#
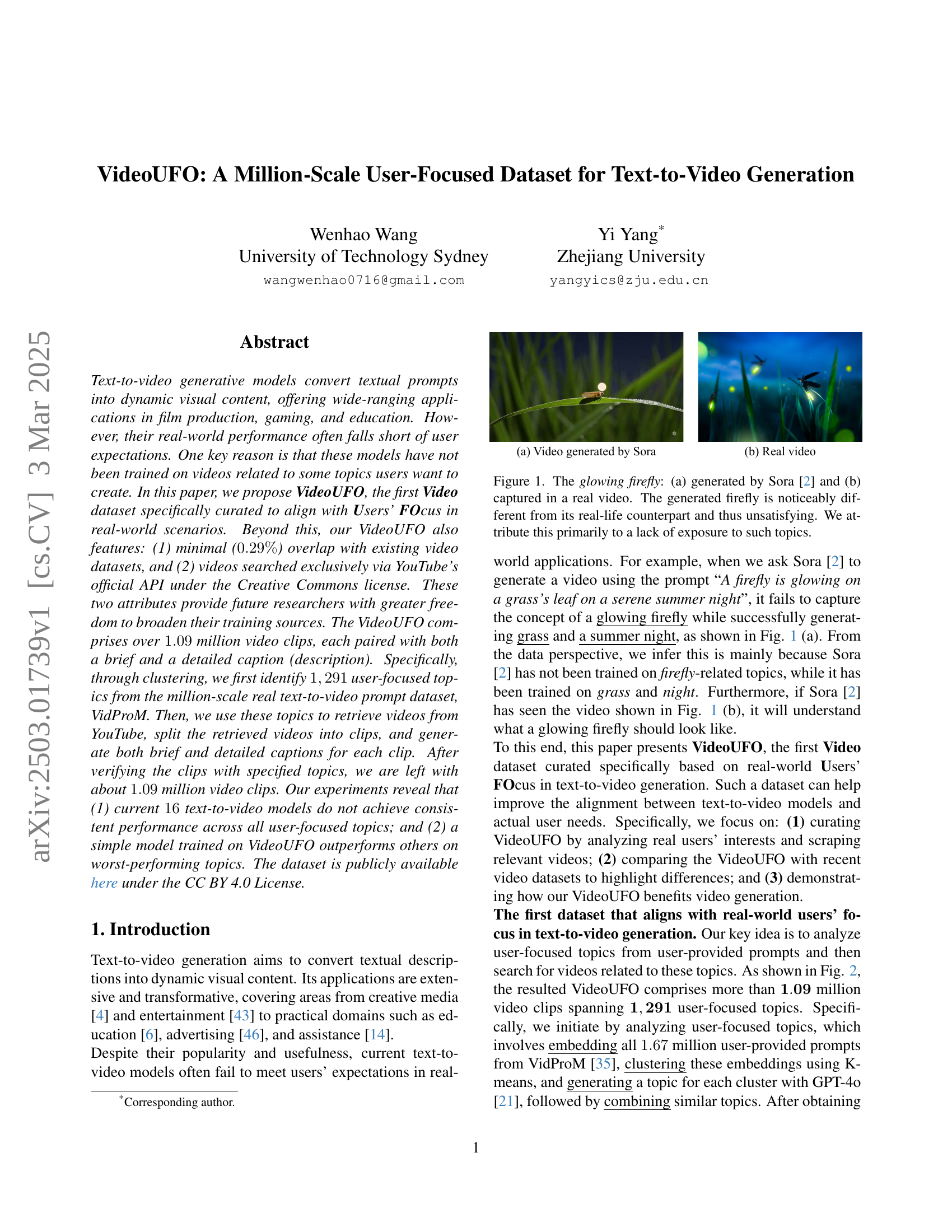
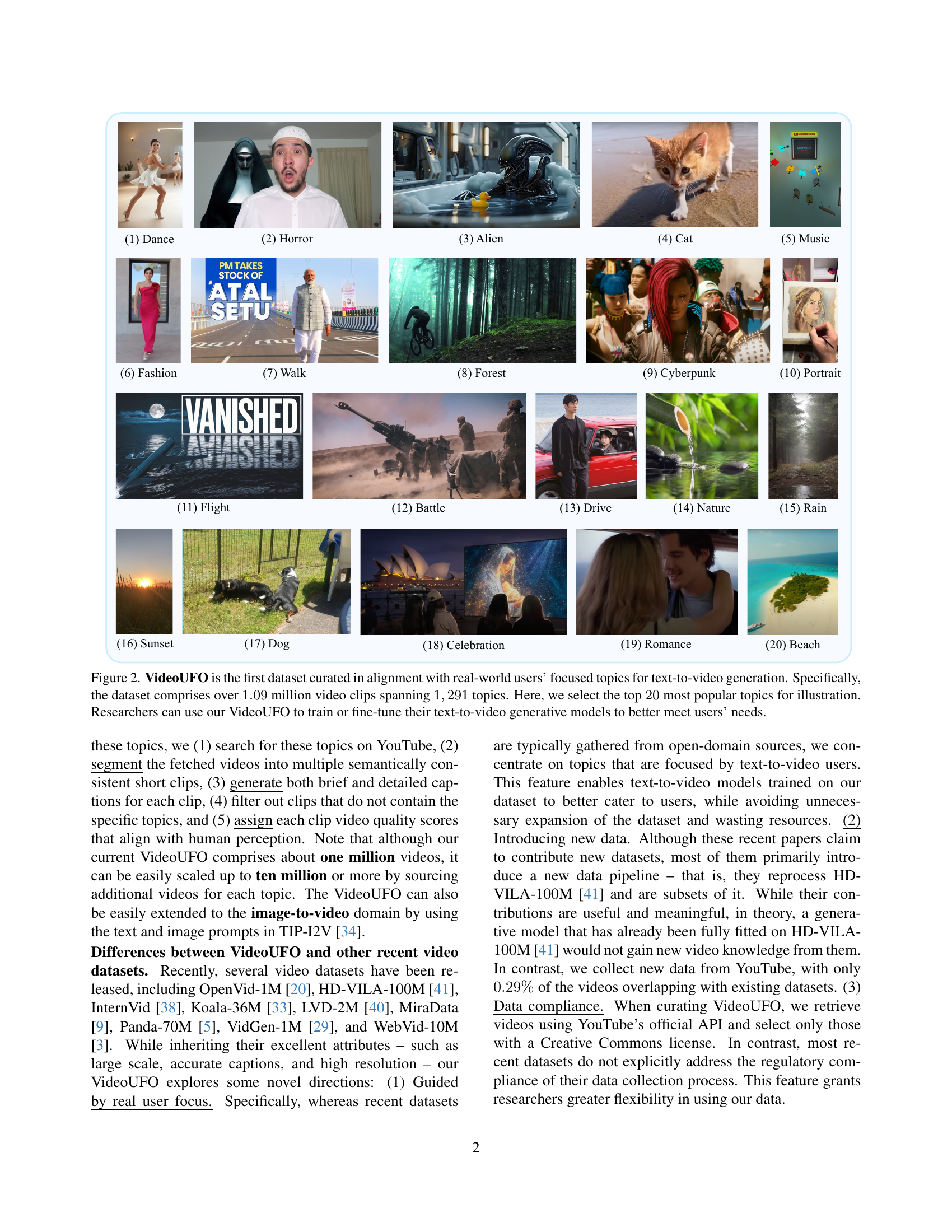
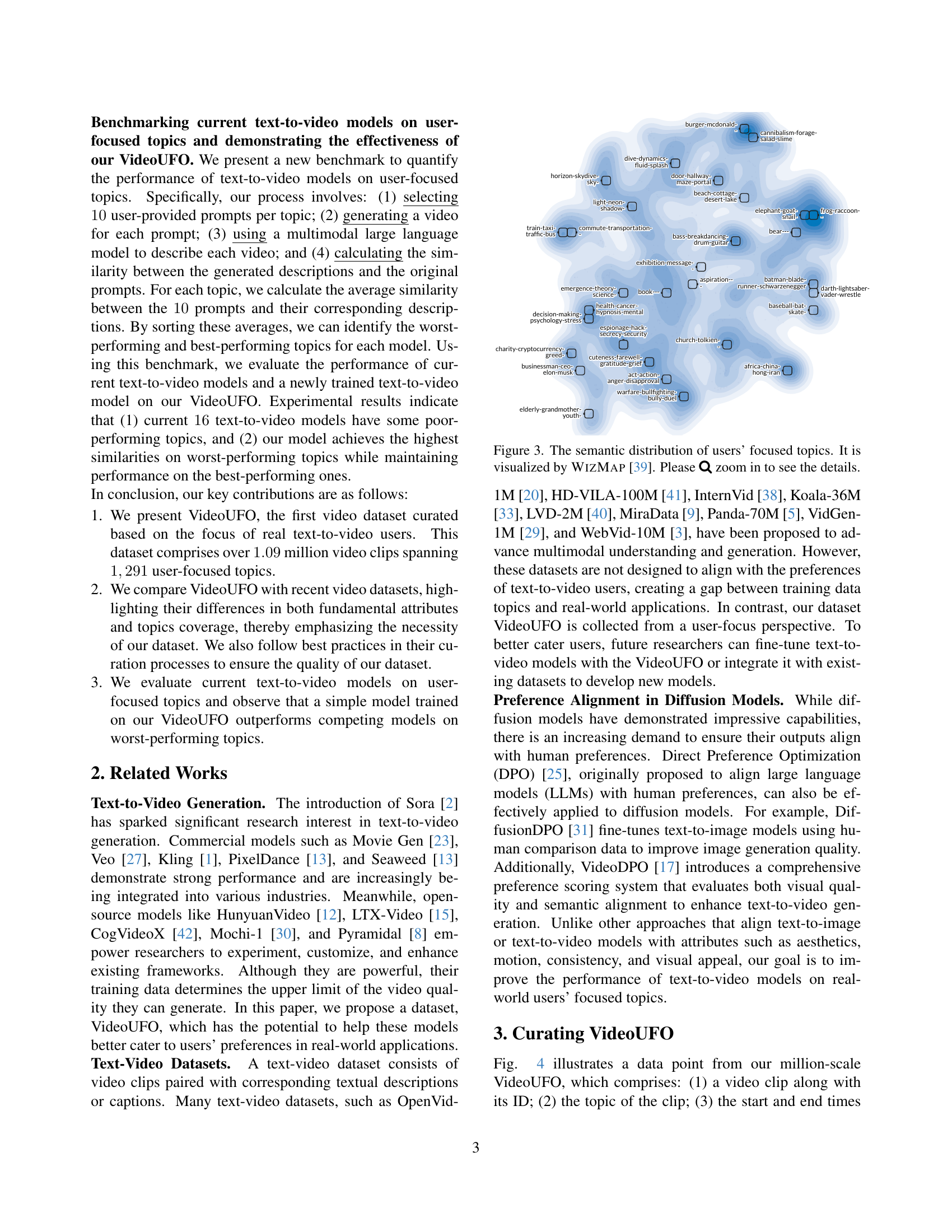
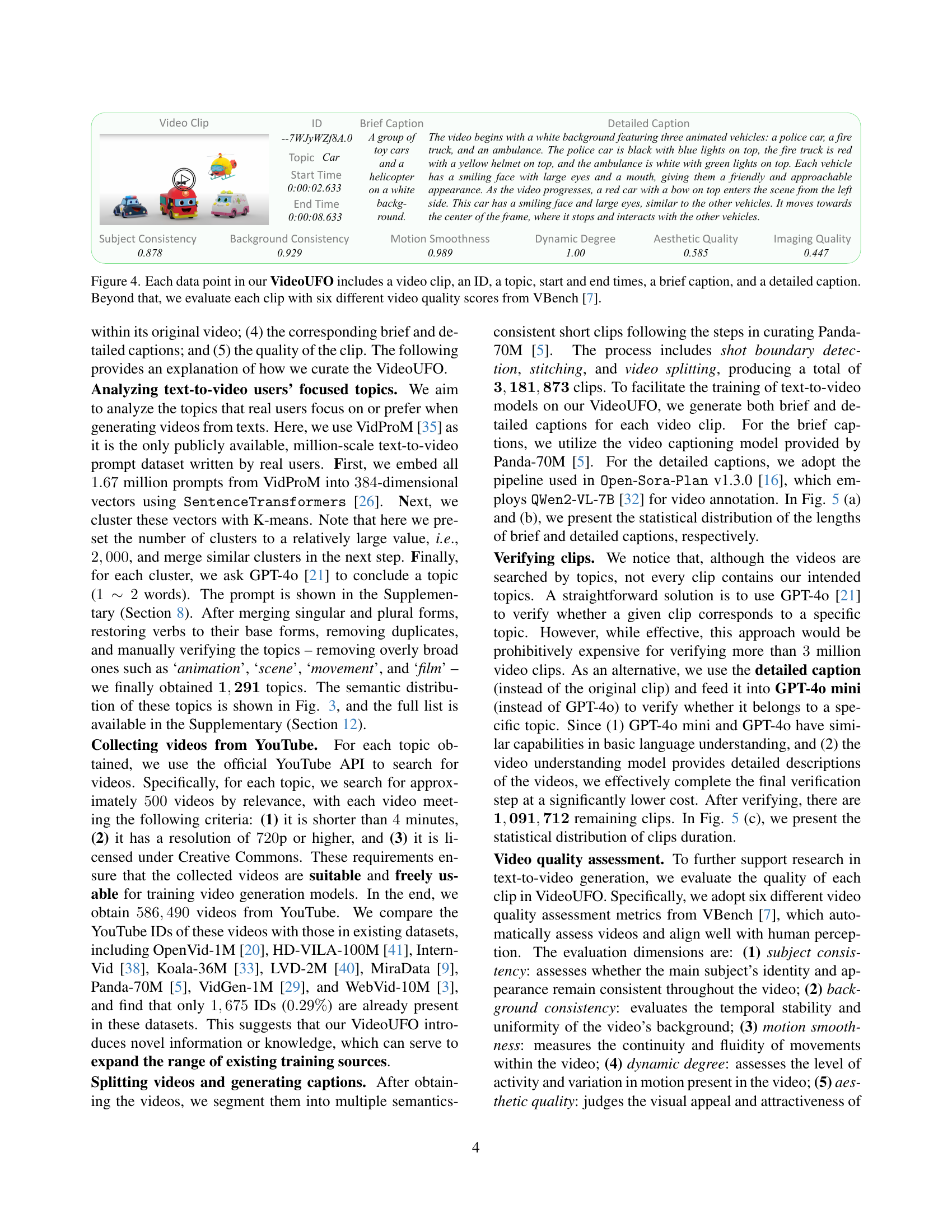
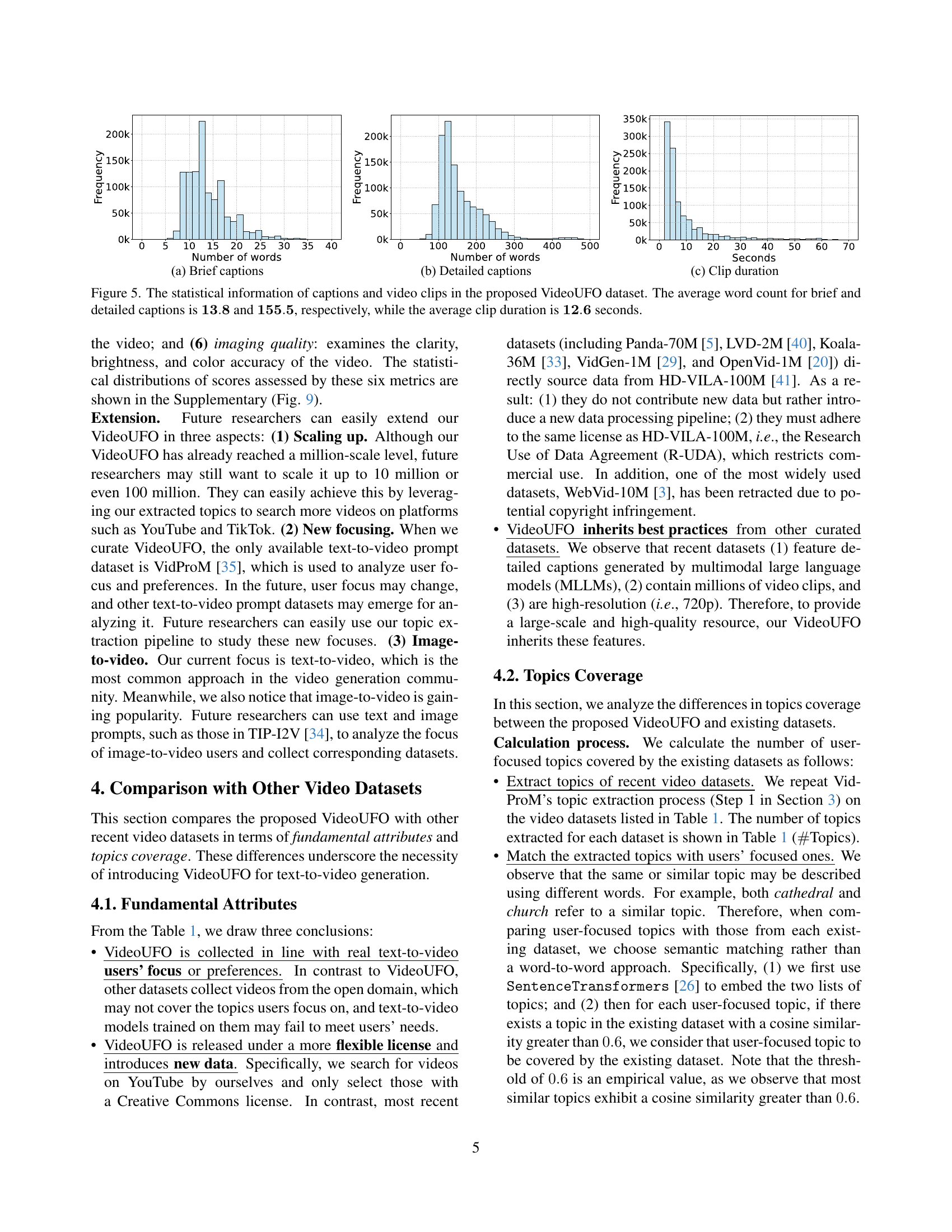
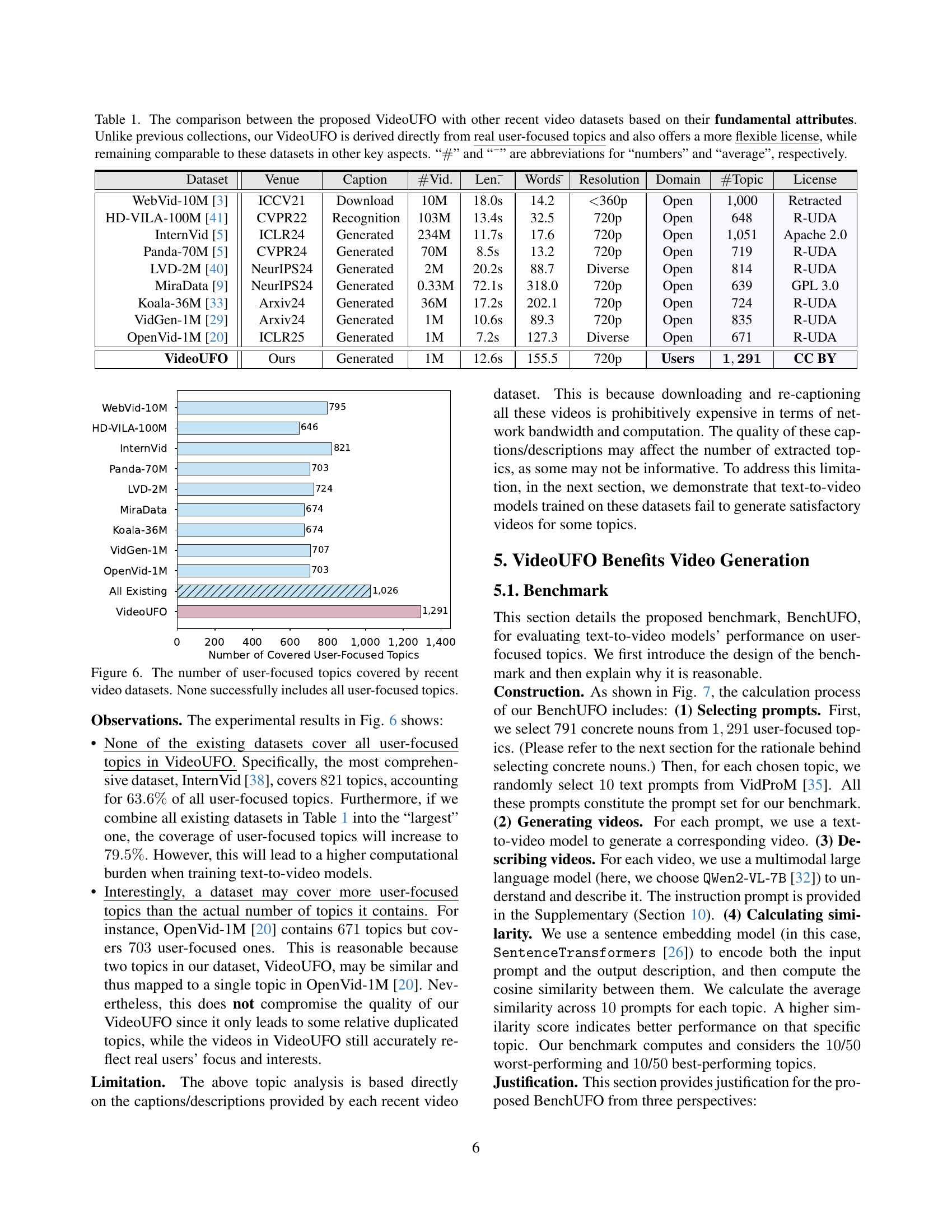
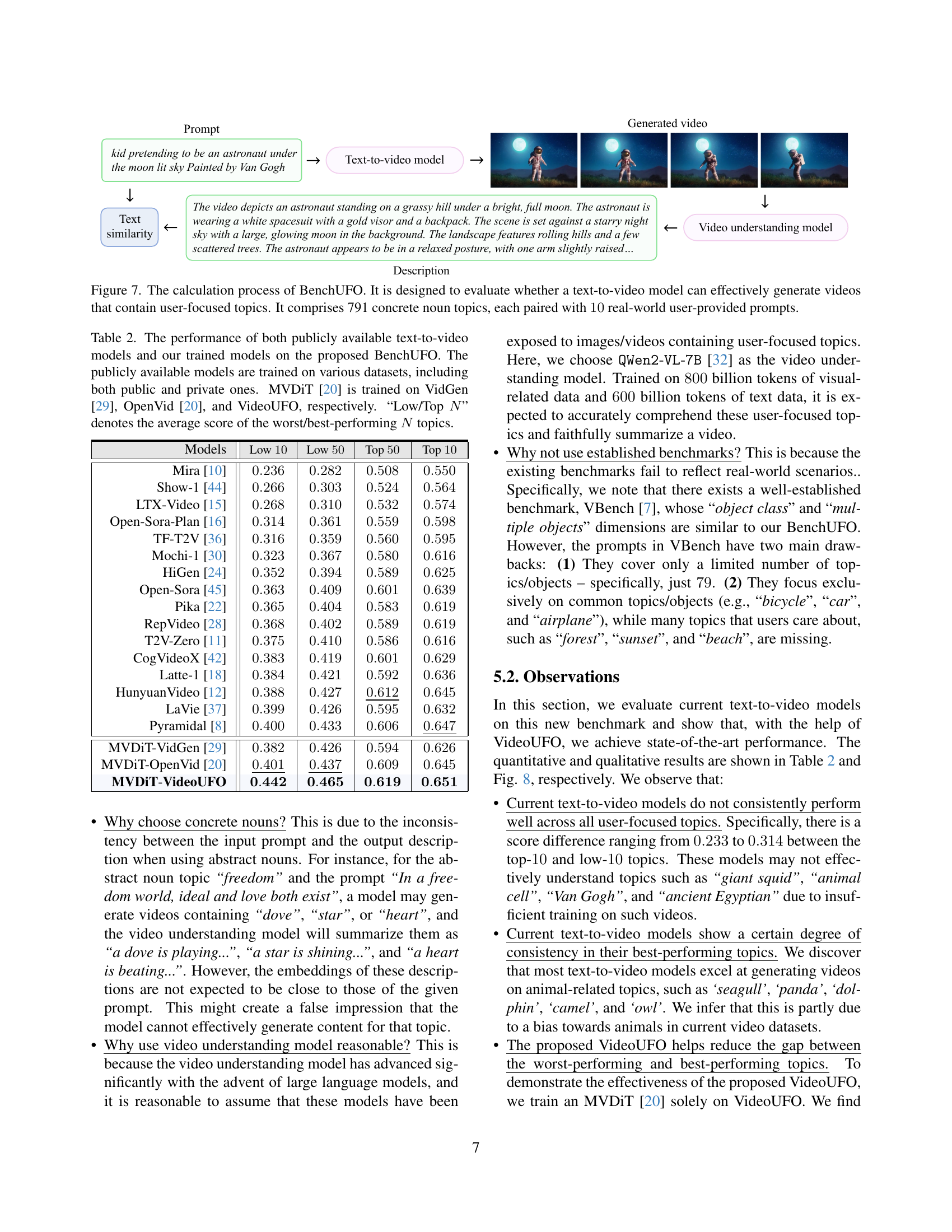
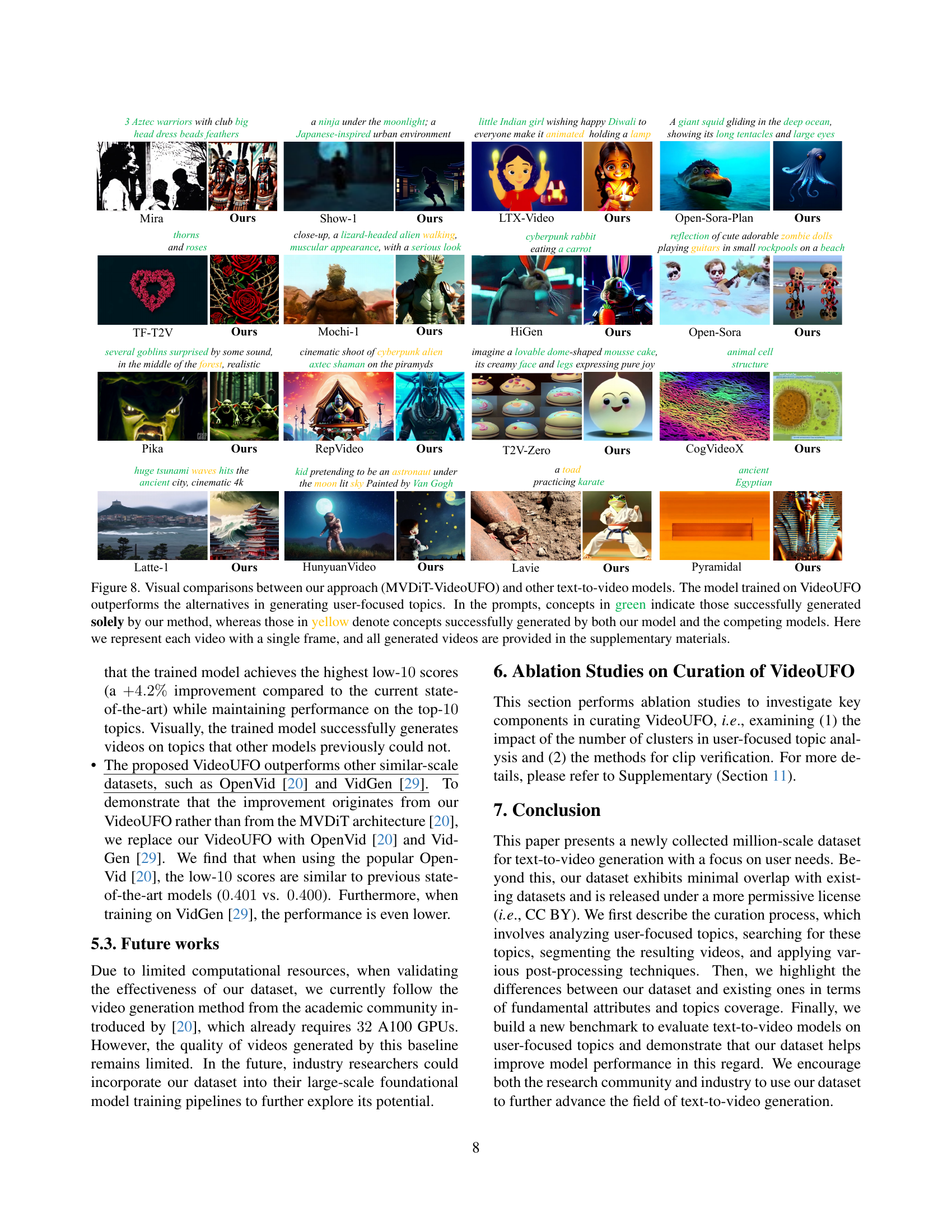
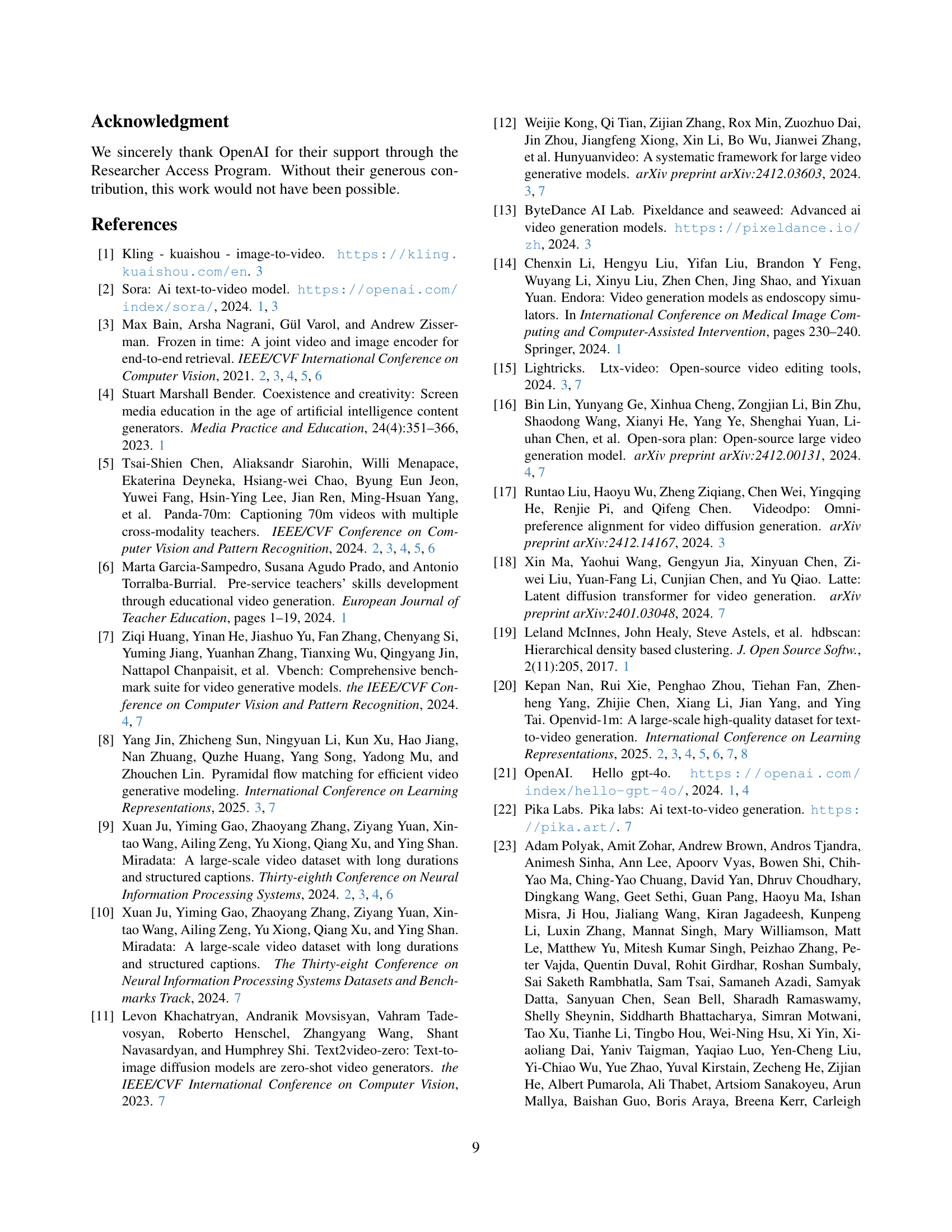
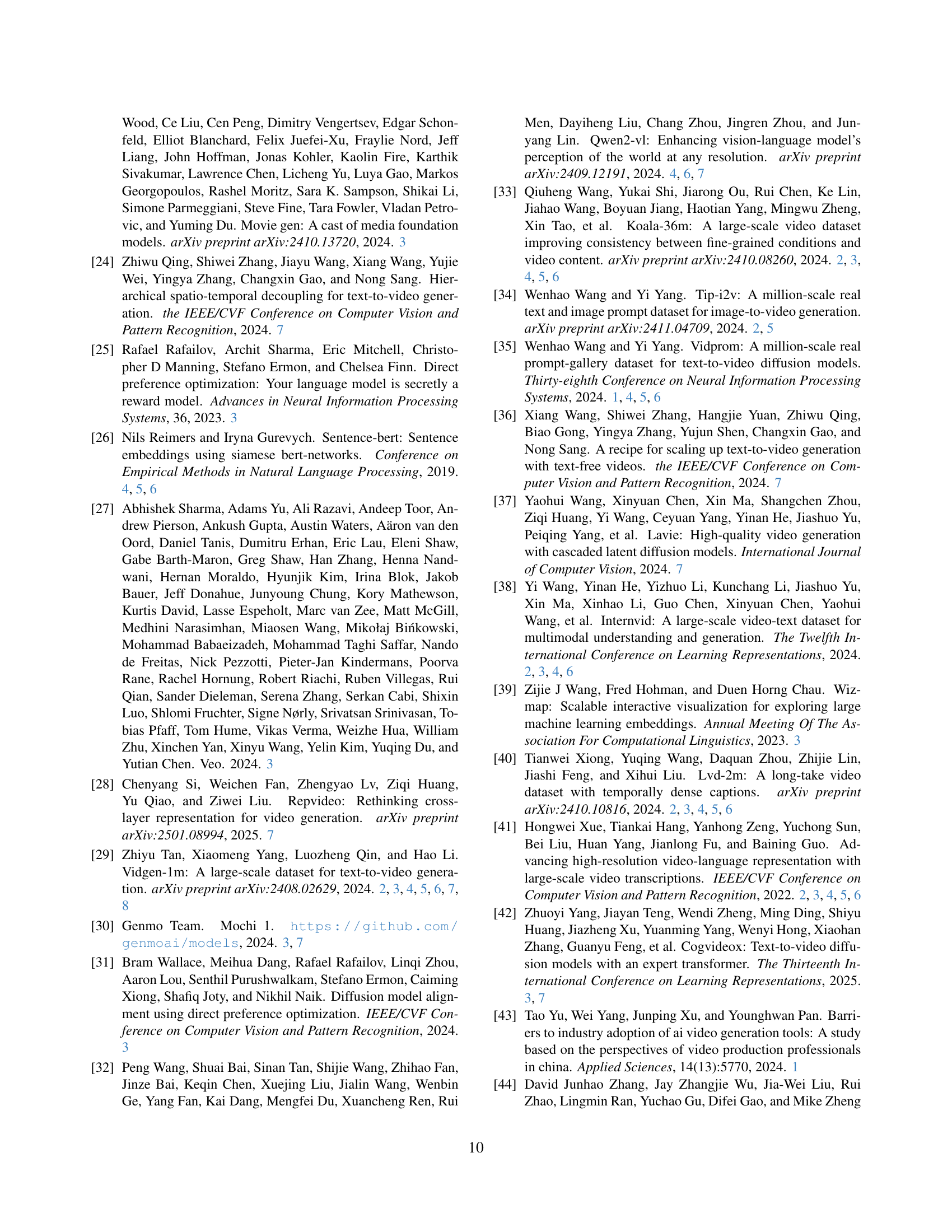
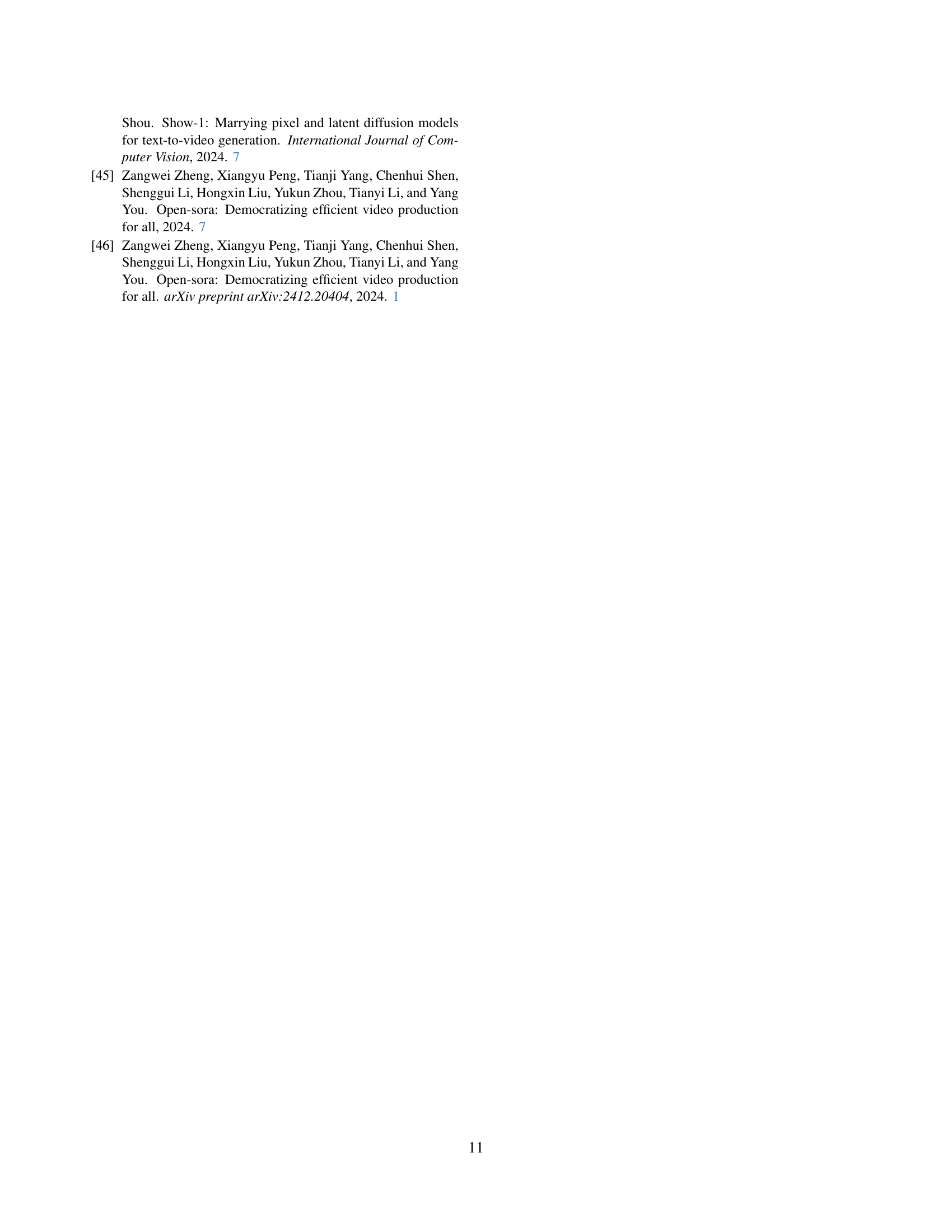
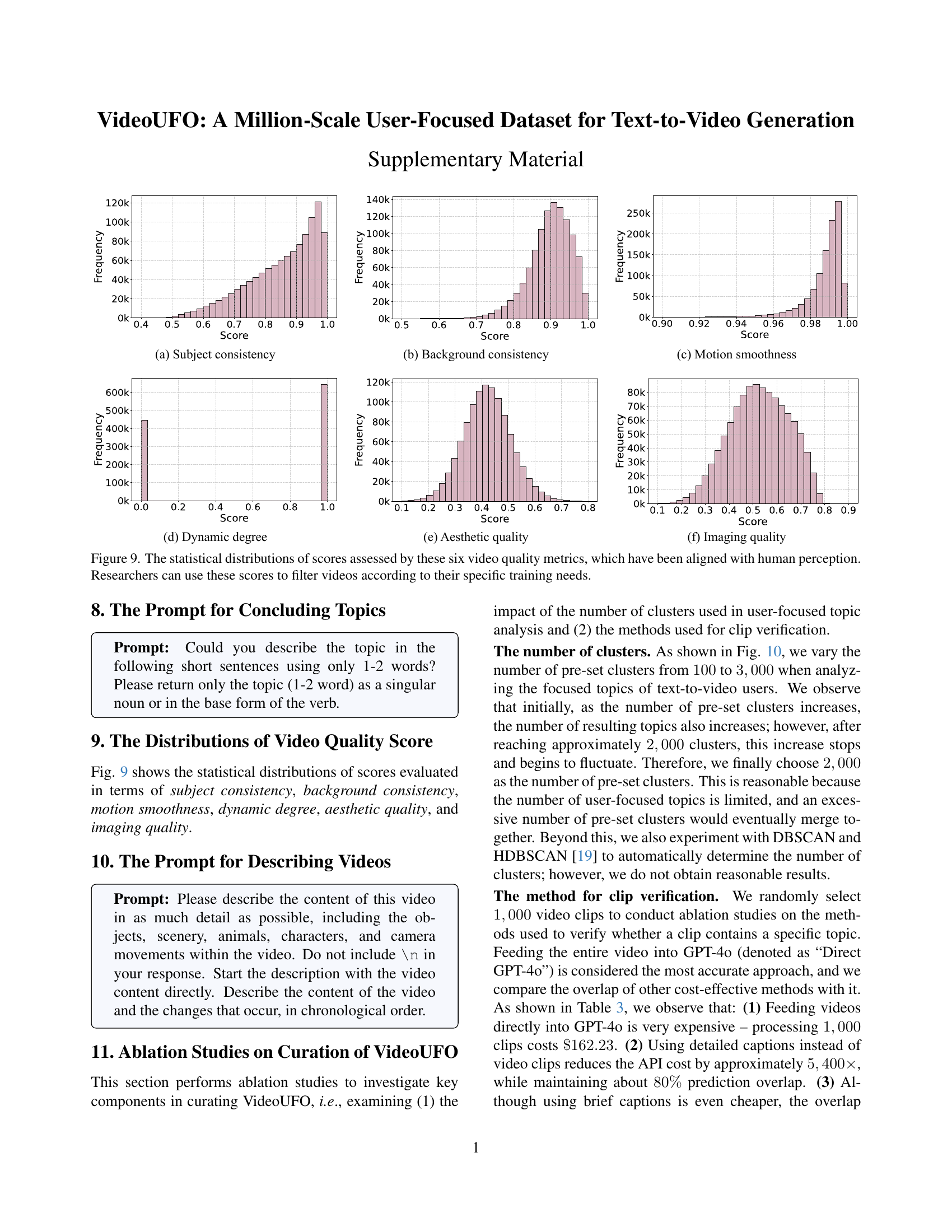
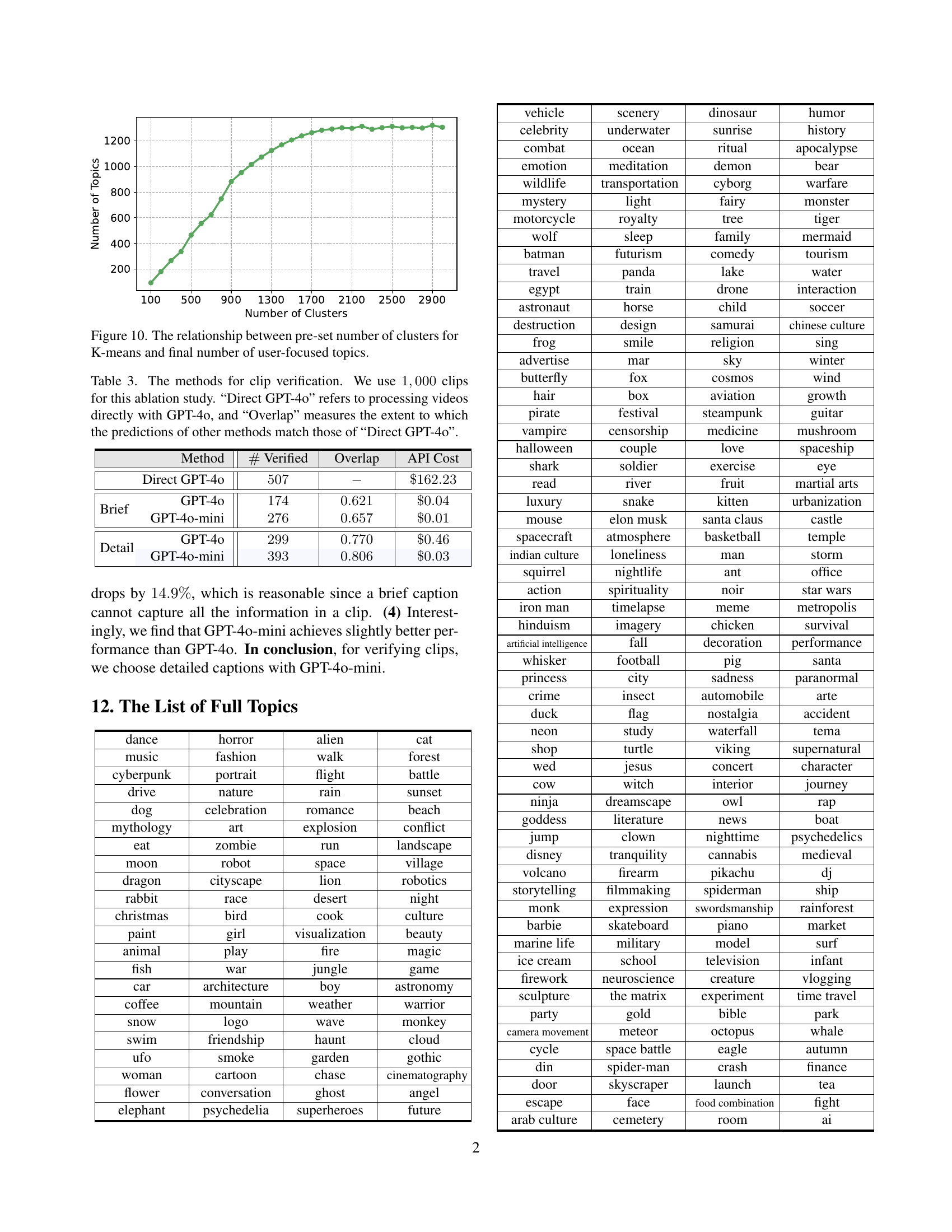
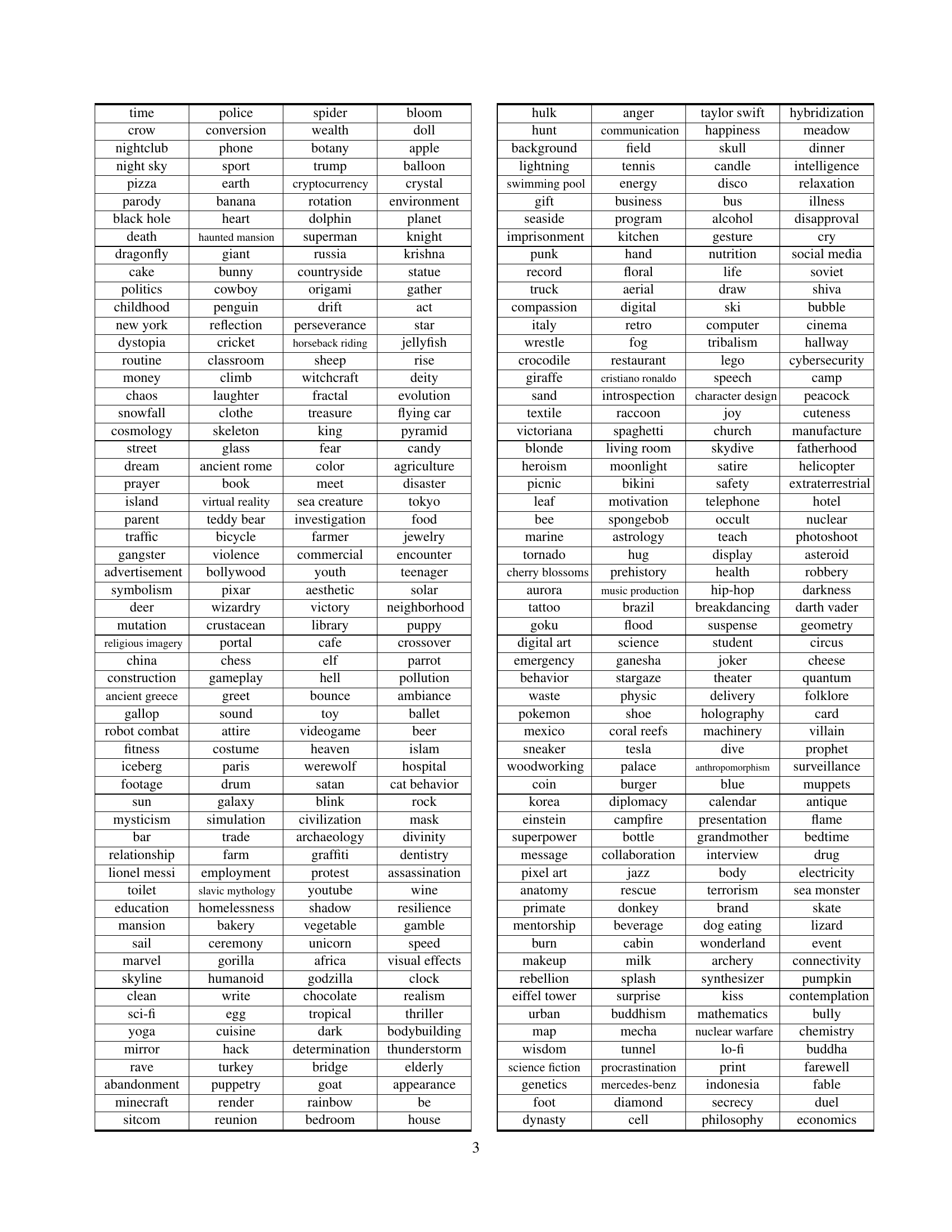
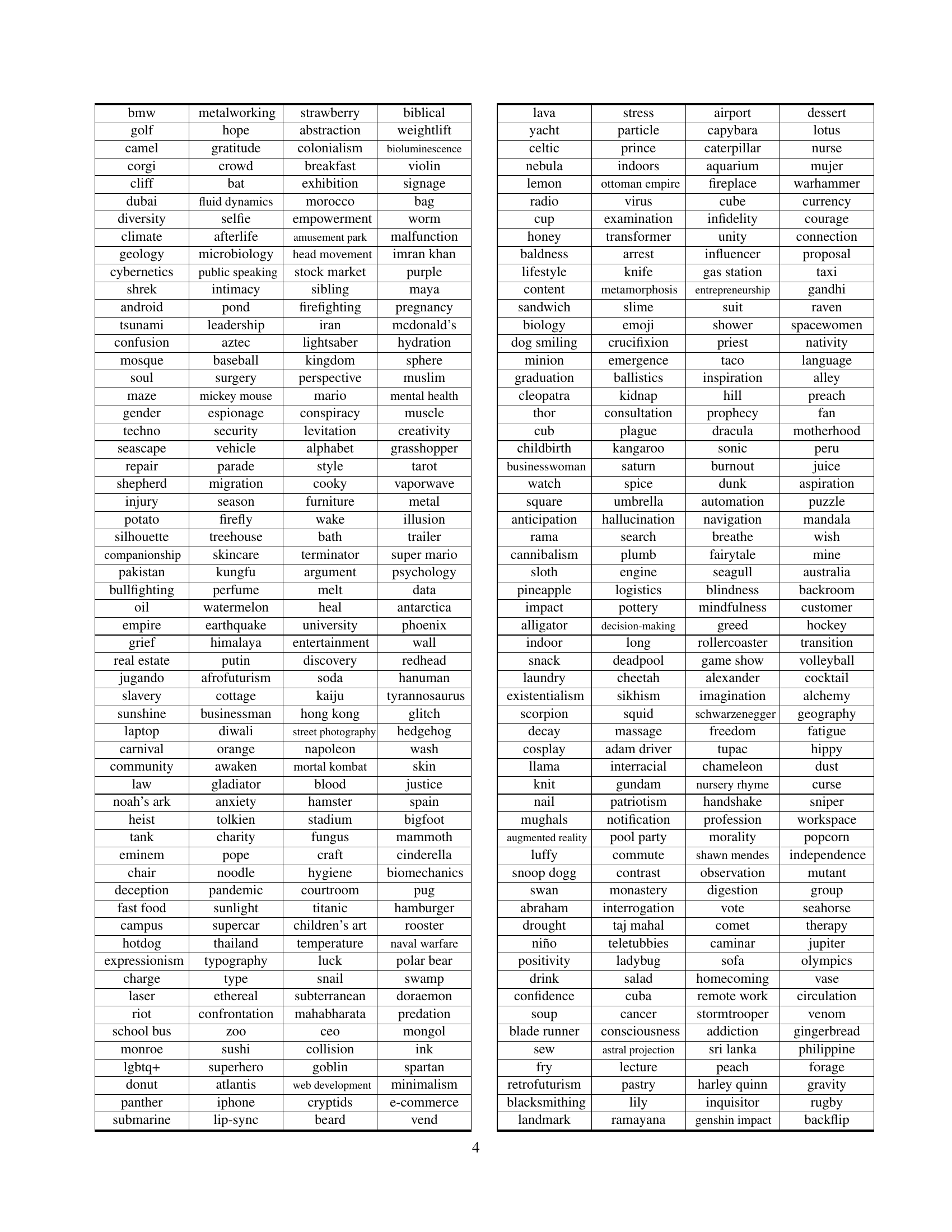
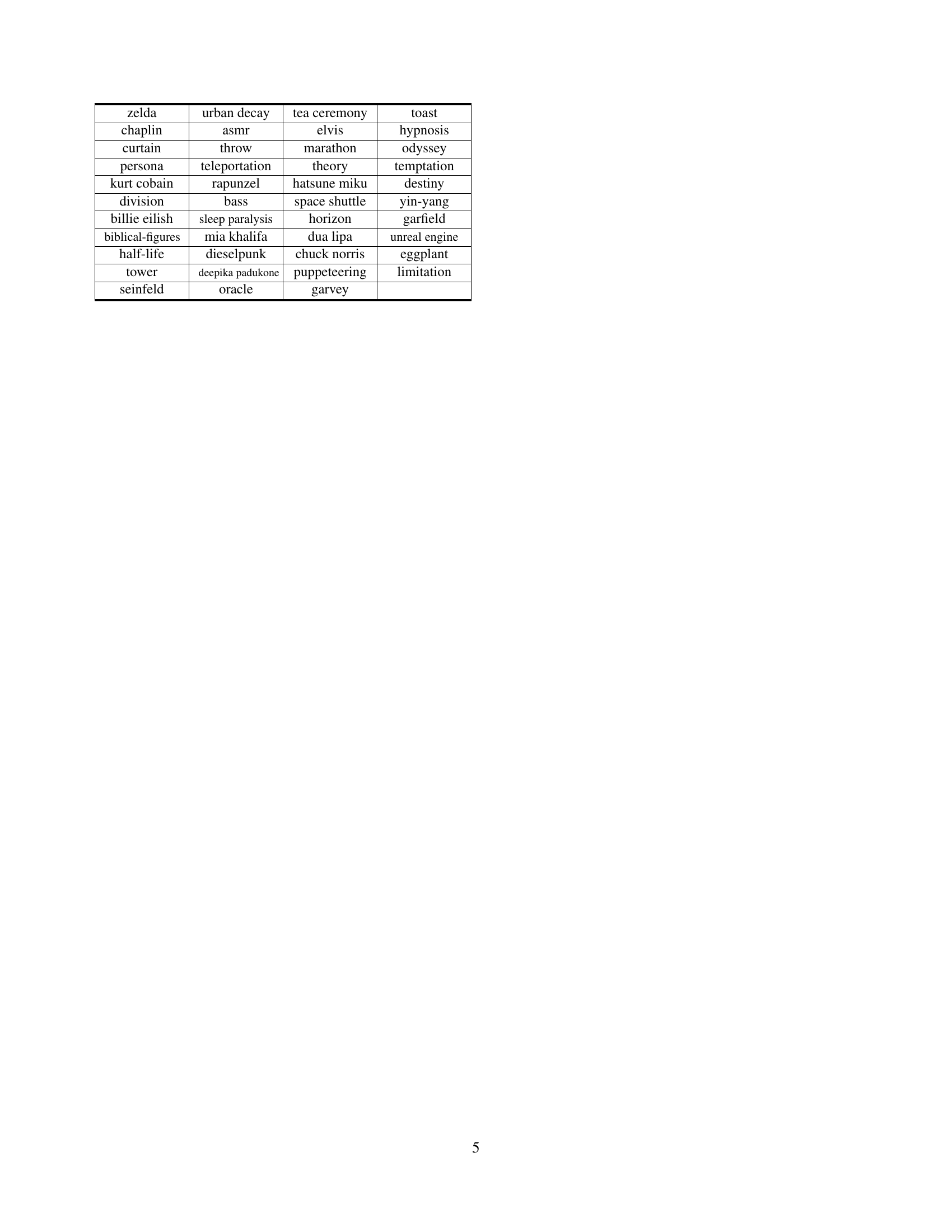