TL;DR#
Video inpainting aims to restore corrupted video, but current methods struggle with generating fully masked objects, preserving background, and maintaining ID consistency in long videos. Existing approaches have limitations with limited pixel propagation or difficulties in balancing background preservation and foreground generation.
To address these issues, VideoPainter, an efficient dual-branch framework with a lightweight context encoder is proposed. This approach processes masked videos and injects background guidance into any pre-trained video diffusion transformer. A strategy to resample inpainting regions is also introduced for ID consistency in videos of any length. They also constructed VPData and VPBench: the largest video inpainting dataset.
Key Takeaways#
Why does it matter?#
This paper introduces VideoPainter, an innovative approach to video inpainting and editing. It will be important as it reduces the difficulties to restores corrupted video and edit videos with user-customized control. It also provides a large-scale dataset for future research.
Visual Insights#
🔼 This figure showcases VideoPainter’s capabilities in video inpainting and editing. The top half illustrates its ability to handle various inpainting tasks with different mask types (objects, landscapes, humans, animals, multiple regions, and random masks), consistently maintaining object identity even in long videos. The bottom half demonstrates VideoPainter’s video editing functionality, enabling users to add, remove, modify attributes of, and swap objects within videos, again showing consistent object identity over longer video sequences.
read the caption
Figure 1. VideoPainter enables plug-and-play text-guided video inpainting and editing for any video length and pre-trained Diffusion Transformer with masked video and video caption (user editing instruction). The upper part demonstrates the effectiveness of VideoPainter in various video inpainting scenarios, including object, landscape, human, animal, multi-region (Multi), and random masks. The lower section demonstrates the performance of VideoPainter in video editing, including adding, removing, changing attributes, and swapping objects. In both video inpainting and editing, we demonstrate strong ID consistency in generating long videos (Any Len.). Project Page: https://yxbian23.github.io/project/video-painter
Dataset | #Clips | Duration | Video Caption | Masked Region Desc. |
---|---|---|---|---|
DAVIS (Perazzi et al., 2016) | 0.4K | 0.1h | ✗ | ✗ |
YouTube-VOS (Xu et al., 2018) | 4.5K | 5.6h | ✗ | ✗ |
VOST (Tokmakov et al., 2023) | 1.5K | 4.2h | ✗ | ✗ |
MOSE (Ding et al., 2023) | 5.2K | 7.4h | ✗ | ✗ |
LVOS (Hong et al., 2023) | 1.0K | 18.9h | ✗ | ✗ |
SA-V (Ravi et al., 2024) | 642.6K | 196.0h | ✗ | ✗ |
Ours | 390.3K | 866.7h | âś“ | âś“ |
🔼 Table 1 compares several video inpainting datasets, highlighting key characteristics such as the number of video clips, total duration, and the availability of segmentation masks, video captions, and masked region descriptions. The table emphasizes that the newly introduced VPData dataset is significantly larger than existing datasets, containing over 390,000 high-quality video clips and including comprehensive annotations.
read the caption
Table 1. Comparison of video inpainting datasets. Our VPData is the largest video inpainting dataset to date, comprising over 390K high-quality clips with segmentation masks, video captions, and masked region descriptions.
In-depth insights#
Dual-Branch DiT#
The Dual-Branch Diffusion Transformer (DiT) architecture signifies an innovative approach to video inpainting by strategically decoupling background preservation from foreground generation. Traditional single-branch architectures often struggle to balance these competing objectives, leading to artifacts and inconsistencies. By employing two distinct branches, the model can dedicate one branch, the context encoder, to efficiently extracting and preserving background information from the masked video. This allows the primary DiT branch to focus on generating coherent and semantically relevant foreground content, guided by text prompts. This design choice is particularly crucial for handling complex scenarios with fully masked objects or intricate backgrounds, where maintaining contextual fidelity is paramount. The dual-branch approach enhances the overall quality and consistency of the inpainted video, leading to more realistic and visually appealing results.
ID Resampling#
The paper introduces an innovative ‘ID Resampling’ technique to maintain identity consistency across long videos, addressing a critical challenge in video inpainting. Traditional DiTs struggle with preserving object ID over extended durations, leading to inconsistent results. The ID Resampling technique tackles this by strategically resampling the tokens of masked regions, which inherently contain information about the desired identity. During training, the method freezes the DiT and context encoder, and introduces trainable ID-Resample Adapters(LoRA), effectively enabling the model to resample the relevant tokens. This process reinforces ID preservation within the inpainting region by enhancing ID sampling using token concatation to K,V vectors, which enables ID focus. During inference, the emphasis shifts to temporal consistency, resampling tokens from the inpainting region of the previous clip. This ensures a smoother transition and better visual coherence across frames, effectively mitigating the risk of visual discontinuities or identity shifts. The technique leverages the concept of maintaining a close visual relationship between adjacent frames during inpainting by prioritizing frame transitions. Ultimately, the ID Resampling is a nuanced approach with LoRA that enhances visual output.
Scalable VPData#
The concept of a ‘Scalable VPData’ dataset is crucial for advancing video inpainting. Scalability addresses the need for large datasets to effectively train deep learning models, especially generative ones. The emphasis is on creating diverse, high-quality training data without bottlenecks. It requires automating or semi-automating data creation, annotation, and cleaning. The ‘VP’ implies a focus on video inpainting, requiring both masked video sequences and the corresponding ground truth. It should have diverse scenes, object categories, motion patterns and text annotation to support text driven approach. Efficient object detection is required to generate a scalable data. SAM models are used to generate quality masks from the segmented video.
Context Encoder#
The context encoder appears to be a crucial component for efficiently processing and integrating background information into the video inpainting process. Given the limitations of existing single-branch architectures in balancing foreground generation and background preservation, a dedicated context encoder offers a promising solution. This encoder likely processes the masked video, extracting features that guide the diffusion transformer. The choice of a lightweight design, constituting only a small fraction of the backbone parameters, is significant. This suggests a focus on computational efficiency without sacrificing performance. Key considerations include how the encoder handles masked and unmasked regions, and whether it employs techniques like attention mechanisms to capture long-range dependencies within the video context. The plug-and-play nature implies modularity and flexibility, indicating compatibility with different pre-trained diffusion transformers. Its integration facilitates user-customized control over the inpainting process.
Plug-and-Play#
The research emphasizes the plug-and-play nature of their proposed framework, showcasing its adaptability and ease of integration with existing architectures. This suggests a modular design, allowing users to readily incorporate the system into various applications without extensive modifications. The system’s flexible nature is highlighted through support for diverse stylization backbones and both text-to-video (T2V) and image-to-video (I2V) diffusion transformer architectures, indicating a broad applicability. The focus on I2V compatibility enables integration with existing image inpainting, and the fact that the system can utilize various image inpainting techniques for the initial frame shows a high degree of adaptability. The ability to generate an initial frame with an image inpainting model highlights the systems flexibilities. Essentially it is adaptable to most existing models.
More visual insights#
More on figures
🔼 Figure 2 illustrates three different approaches to video inpainting: non-generative methods, generative methods, and VideoPainter. Non-generative methods use pixel propagation from the background to fill in missing parts of a video, but this approach struggles to inpaint fully masked objects. Generative methods adapt image inpainting models to videos by adding temporal attention, but they often struggle to maintain background fidelity and generate foreground content simultaneously. In contrast, VideoPainter uses a dual-branch architecture with a lightweight context encoder and a pre-trained Diffusion Transformer. This approach separates background preservation and foreground generation, resulting in better inpainting quality and allowing for plug-and-play control.
read the caption
Figure 2. Framework Comparison. Non-generative approaches, limited to pixel propagation from backgrounds, fail to inpaint fully segmentation-masked objects. Generative methods adapt single-branch image inpainting models to video by adding temporal attention, struggling to maintain background fidelity and generate foreground contents in one model. In contrast, VideoPainter implements a dual-branch architecture that leverages an efficient context encoder with any pre-trained DiT, decoupling video inpainting to background preservation and foreground generation, and enabling plug-and-play video inpainting control.
🔼 This figure illustrates the pipeline used to create the VPData and VPBench datasets. The pipeline consists of five main stages: 1) Data Collection from sources like Videvo and Pexels; 2) Annotation using a multi-stage process involving object recognition, bounding box detection, mask generation, and scene splitting; 3) Splitting videos into shorter clips (10 seconds), discarding clips shorter than 6 seconds; 4) Selection of high-quality clips based on criteria such as aesthetic quality, motion strength, and content safety; and 5) Captioning, which uses advanced vision language models (CogVLM and GPT-4) to generate detailed captions and descriptions of masked regions in the videos. This pipeline allows for the creation of a large-scale, high-quality video inpainting dataset with detailed annotations.
read the caption
Figure 3. Dataset Construction Pipeline. It consists of five pre-processing steps: collection, annotation, splitting, selection, and captioning.
🔼 Figure 4 provides a detailed breakdown of the VideoPainter model’s architecture and operation. The upper half illustrates the model’s dual-branch structure. A context encoder processes a combination of noisy latent data, downscaled masks, and masked video latent representations (all processed through a Variational Autoencoder or VAE). The resulting features are then selectively integrated into a pre-trained Diffusion Transformer (DiT), with two encoder layers affecting the first and second halves of the DiT. Critically, only background tokens are added to maintain clarity. The lower half shows the inpainting ID region resampling, utilizing an ID Resample Adapter. During training, current masked region tokens are appended to key-value (KV) vectors for improved ID consistency. During inference, this process ensures consistency with the preceding video clip.
read the caption
Figure 4. Model overview. The upper figure shows the architecture of VideoPainter. The context encoder performs video inpainting based on concatenation of the noisy latent, downsampled masks, and masked video latent via VAE. Features extracted by the context encoder are integrated into the pre-trained DiT in a group-wise and token-selective manner, where two encoder layers modulate the first and second halves of the DiT, respectively, and only the background tokens will be integrated into the backbone to prevent information ambiguity. The lower figure illustrates the inpainting ID region resampling with the ID Resample Adapter. During training, tokens of the current masked region are concatenated to the KV vectors, enhancing ID preservation of the inpainting region. During inference, the ID tokens of the last clip are concatenated to the current KV vectors, maintaining ID consistency with the last clip by resampling.
🔼 Figure 5 presents a qualitative comparison of VideoPainter against several state-of-the-art video inpainting methods. It showcases the performance differences on both standard-length and long videos. The figure highlights VideoPainter’s superior ability to maintain video coherence, generate high-quality results, and achieve strong alignment with text captions. The results demonstrate VideoPainter’s effectiveness in preserving backgrounds, accurately filling in masked regions, and generating realistic content that is consistent with the provided text prompts. More detailed visualizations are available in the accompanying demo video.
read the caption
Figure 5. Comparison of previous inpainting methods and VideoPainter on standard and long video inpainting. More visualizations are in the demo video.
🔼 Figure 6 presents a qualitative comparison of VideoPainter against other state-of-the-art video editing methods. It showcases the results of standard and long-video editing tasks on various video clips. The figure visually demonstrates VideoPainter’s superior performance in terms of background preservation, text alignment, and overall video quality, highlighting its ability to handle both short and extended video sequences effectively. For a more comprehensive view of the results, the authors suggest referring to the accompanying demo video.
read the caption
Figure 6. Comparison of previous editing methods and VideoPainter on standard and long video editing. More visualizations are in the demo video.
🔼 Figure 7 demonstrates the versatility and plug-and-play nature of VideoPainter. It shows how easily VideoPainter integrates with pre-trained, community-developed models, specifically a Gromit-style LoRA (Cseti, 2024). This highlights VideoPainter’s ability to work with diverse styles and models, even those with a significant domain gap (in this case, an anime style LoRA applied to a dataset not trained on anime). The successful integration underscores VideoPainter’s adaptability and ease of use, proving that users can leverage various pre-trained models and customize their video inpainting results.
read the caption
Figure 7. Integrating VideoPainter to Gromit-style LoRA (Cseti, 2024).
🔼 Figure 8 presents supplementary examples of video inpainting results produced by VideoPainter. Each row showcases a different scenario: object inpainting, landscape inpainting, human inpainting, animal inpainting, multi-object inpainting, and random inpainting. The figure demonstrates the model’s ability to effectively inpaint diverse content within videos, preserving temporal coherence and maintaining the overall quality of the video.
read the caption
Figure 8. More video inpainting results.
More on tables
Metrics | Masked Region Preservation | Text Alignment | Video Quality | ||||||
---|---|---|---|---|---|---|---|---|---|
Models | PSNR | SSIM | LPIPS | MSE | MAE | CLIP Sim | CLIP Sim (M) | FVID | |
VPBench-S | ProPainter | 20.97 | 0.87 | 9.89 | 1.24 | 3.56 | 7.31 | 17.18 | 0.44 |
COCOCO | 19.27 | 0.67 | 14.80 | 1.62 | 6.38 | 7.95 | 20.03 | 0.69 | |
Cog-Inp | 22.15 | 0.82 | 9.56 | 0.88 | 3.92 | 8.41 | 21.27 | 0.18 | |
Ours | 23.32 | 0.89 | 6.85 | 0.82 | 2.62 | 8.66 | 21.49 | 0.15 | |
VPBench-L | ProPainter | 20.11 | 0.84 | 11.18 | 1.17 | 3.71 | 9.44 | 17.68 | 0.48 |
COCOCO | 19.51 | 0.66 | 16.17 | 1.29 | 6.02 | 11.00 | 20.42 | 0.62 | |
Cog-Inp | 19.78 | 0.73 | 12.53 | 1.33 | 5.13 | 11.47 | 21.22 | 0.21 | |
Ours | 22.19 | 0.85 | 9.14 | 0.71 | 2.92 | 11.52 | 21.54 | 0.17 | |
Davis | ProPainter | 23.99 | 0.92 | 5.86 | 0.98 | 2.48 | 7.54 | 16.69 | 0.12 |
COCOCO | 21.34 | 0.66 | 10.51 | 0.92 | 4.99 | 6.73 | 17.50 | 0.33 | |
Cog-Inp | 23.92 | 0.79 | 10.78 | 0.47 | 3.23 | 7.03 | 17.53 | 0.17 | |
Ours | 25.27 | 0.94 | 4.29 | 0.45 | 1.41 | 7.21 | 18.46 | 0.09 |
🔼 Table 2 presents a quantitative comparison of VideoPainter’s performance against three other video inpainting models: ProPainter, COCOCO, and Cog-Inp. The models were evaluated on three datasets: VPBench (for segmentation masks, with results shown for both standard-length and long videos) and Davis (for random masks). The evaluation metrics cover three aspects of video inpainting: masked region preservation (PSNR, SSIM, LPIPS, MSE, MAE), text alignment (CLIP Sim, CLIP Sim (M)), and video quality (FVID). The best performing model for each metric is highlighted in red, while the second best is in blue. This table provides a comprehensive performance evaluation of VideoPainter across various video inpainting tasks and allows for a direct comparison with existing state-of-the-art techniques.
read the caption
Table 2. Quantitative comparisons among VideoPainter and other video inpainting models in VPBench for segmentation mask (Standard (S) and Long (L) Video) and Davis for random mask: ProPainter (Zhou et al., 2023), COCOCO (Zi et al., 2024), and Cog-Inp (Yang et al., 2024). Metrics include masked region preservation, text alignment, and video quality. Red stands for the best, Blue stands for the second best.
Metrics | Masked Region Preservation | Text Alignment | Video Quality | ||||||
---|---|---|---|---|---|---|---|---|---|
Models | PSNR | SSIM | LPIPS | MSE | MAE | CLIP Sim | CLIP Sim (M) | FVID | |
Standard | UniEdit | 9.96 | 0.36 | 56.68 | 11.08 | 25.78 | 8.46 | 14.23 | 1.36 |
DitCtrl | 9.30 | 0.33 | 57.42 | 12.73 | 27.45 | 8.52 | 15.59 | 0.57 | |
ReVideo | 15.52 | 0.49 | 27.68 | 3.49 | 11.14 | 9.34 | 20.01 | 0.42 | |
Ours | 22.63 | 0.91 | 7.65 | 1.02 | 2.90 | 8.67 | 20.20 | 0.18 | |
Long | UniEdit | 10.37 | 0.30 | 54.61 | 10.25 | 24.89 | 10.85 | 15.42 | 1.00 |
DitCtrl | 9.76 | 0.28 | 62.49 | 11.50 | 26.64 | 11.78 | 16.52 | 0.56 | |
ReVideo | 15.50 | 0.46 | 28.57 | 3.92 | 12.24 | 11.22 | 20.50 | 0.35 | |
Ours | 22.60 | 0.90 | 7.53 | 0.86 | 2.76 | 11.85 | 19.38 | 0.11 |
🔼 Table 3 presents a quantitative comparison of VideoPainter against three other video editing models: UniEdit, DitCtrl, and ReVideo. The comparison is based on the VPBench dataset, using both standard-length and long videos. Evaluation metrics encompass masked region preservation (how well the model maintains the integrity of the masked areas), text alignment (how well the generated video aligns semantically with the text caption describing the edits), and overall video quality. The best-performing model in each metric is highlighted in red, with the second-best in blue. This table helps illustrate VideoPainter’s performance relative to existing approaches in video editing tasks.
read the caption
Table 3. Quantitative comparisons among VideoPainter and other video editing models in VPBench (Standard and Long Video): UniEdit (Bai et al., 2024), DitCtrl (Cai et al., 2024), and ReVideo (Mou et al., 2024). Metrics include masked region preservation, text alignment, and video quality. Red stands for the best, Blue stands for the second best.
Task | Video Inpainting | Video Editing | ||||
---|---|---|---|---|---|---|
Background | Text | Video | Background | Text | Video | |
Preservation | Alignment | Quality | Preservation | Alignment | Quality | |
Ours |
🔼 This table presents the results of a user study comparing VideoPainter’s performance against other video inpainting and editing baselines. Participants rated videos generated by different models based on three criteria: background preservation, text alignment, and overall video quality. The preference ratios show the percentage of users who preferred VideoPainter for each criterion in both inpainting and editing tasks.
read the caption
Table 4. User Study: User preference ratios comparing VideoPainter with video inpainting and editing baselines.
Metrics | Masked Region Preservation | Text Alignment | Video Quality | |||||
Models | PSNR | SSIM | LPIPS | MSE | MAE | CLIP Sim | CLIP Sim (M) | FVID |
Single-Branch | 20.54 | 0.79 | 10.48 | 0.94 | 4.16 | 8.19 | 19.31 | 0.22 |
VideoPainter (1) | 21.92 | 0.81 | 8.78 | 0.89 | 3.26 | 8.44 | 20.79 | 0.17 |
VideoPainter (4) | 22.86 | 0.85 | 6.51 | 0.83 | 2.86 | 9.12 | 20.49 | 0.16 |
w/o Select | 20.94 | 0.74 | 7.90 | 0.95 | 3.87 | 8.26 | 17.84 | 0.25 |
VideoPainter (T2V) | 23.01 | 0.87 | 6.94 | 0.89 | 2.65 | 9.41 | 20.66 | 0.16 |
VideoPainter | 23.32 | 0.89 | 6.85 | 0.82 | 2.62 | 8.66 | 21.49 | 0.15 |
w/o Resample (L) | 21.79 | 0.84 | 8.65 | 0.81 | 3.10 | 11.35 | 20.68 | 0.19 |
VideoPainter (L) | 22.19 | 0.85 | 9.14 | 0.71 | 2.92 | 11.52 | 21.54 | 0.17 |
🔼 This ablation study analyzes the impact of various design choices in VideoPainter on the VPBench dataset. It compares a baseline single-branch model (where input channels are added to adapt masked video and the backbone is fine-tuned) against several VideoPainter variants. These variants systematically remove or alter key components, such as varying the depth of the context encoder (from one to four layers), removing the selective token integration step (integrating all context encoder tokens into the DiT), switching the backbone from image-to-video (I2V) to text-to-video (T2V) DiTs, and removing the target region ID resampling. Results for both standard and long video subsets are included. The best performing variant in each metric is highlighted in red.
read the caption
Table 5. Ablation Studies on VPBench. Single-Branch: We add input channels to adapt masked video and finetune the backbone. Layer Configuration (VideoPainter (*)): We vary the context encoder depth from one to four layers. w/o Selective Token Integration (w/o Select):: We bypass the token pre-selection step and integrate all context encoder tokens into DiT. T2V Backbone (VideoPainter (T2V)): We replace the backbone from image-to-video DiTs to text-to-video DiTs. w/o target region ID resampling (w/o Resample): We ablate on the target region ID resampling. (L) denotes evaluation on the long video subset. Red stands for the best result.
Full paper#
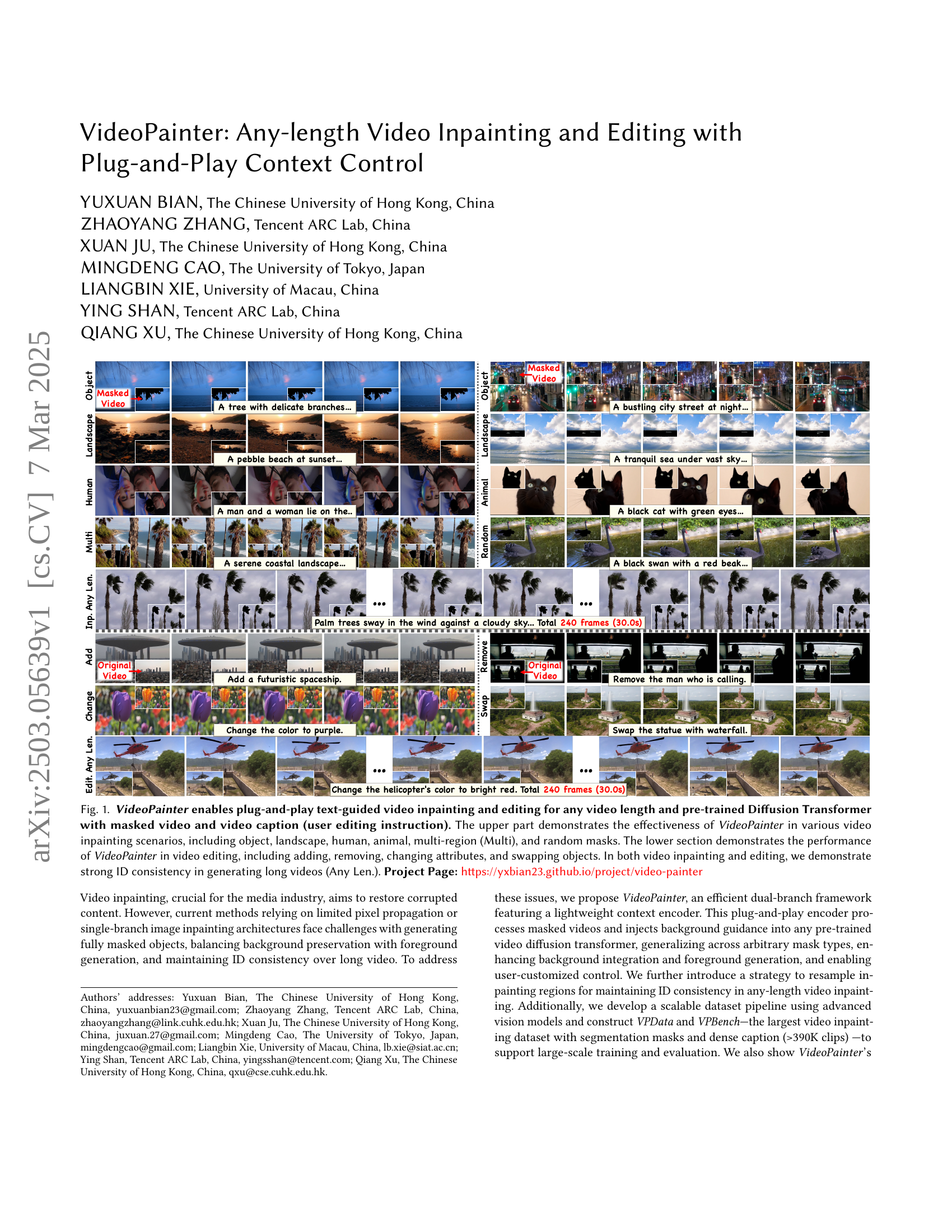
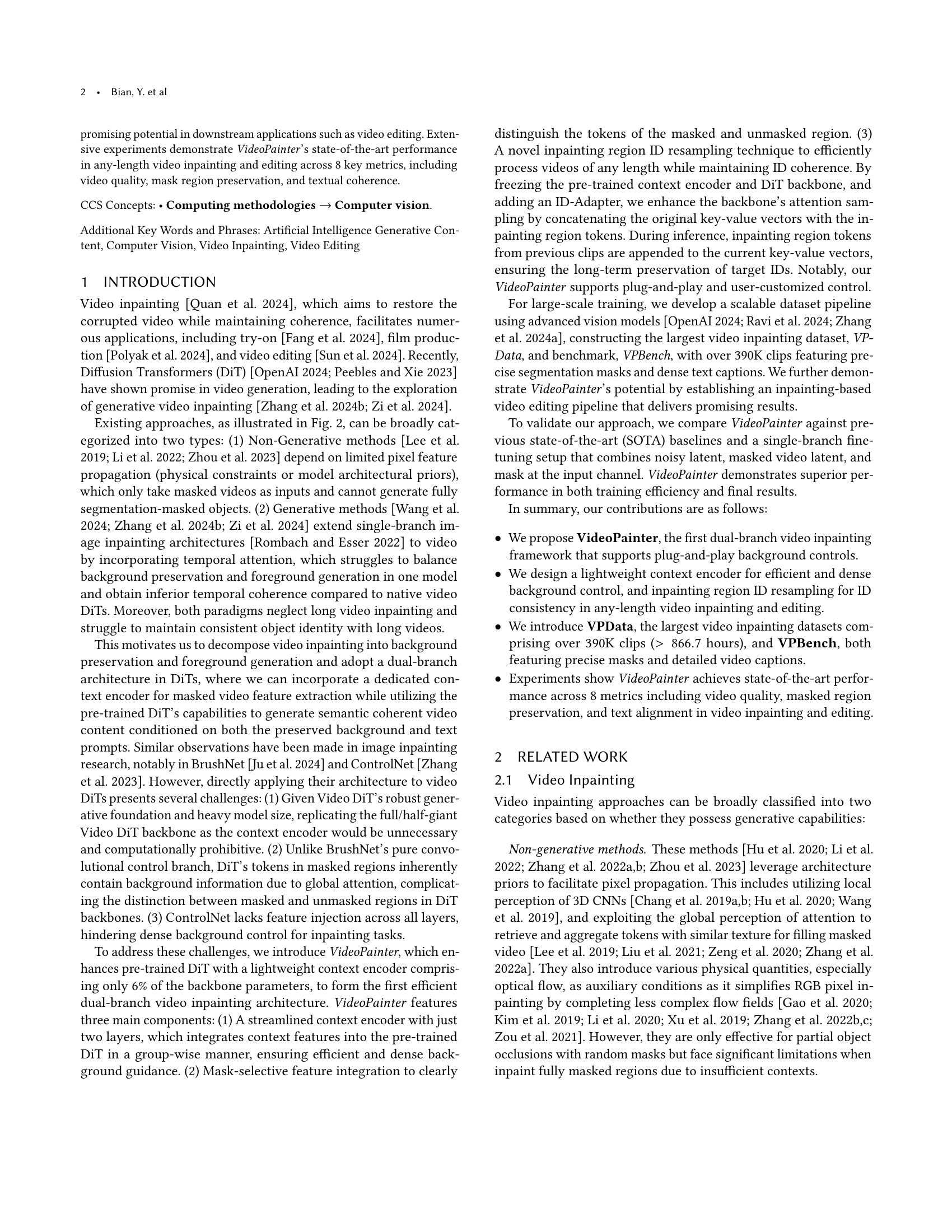
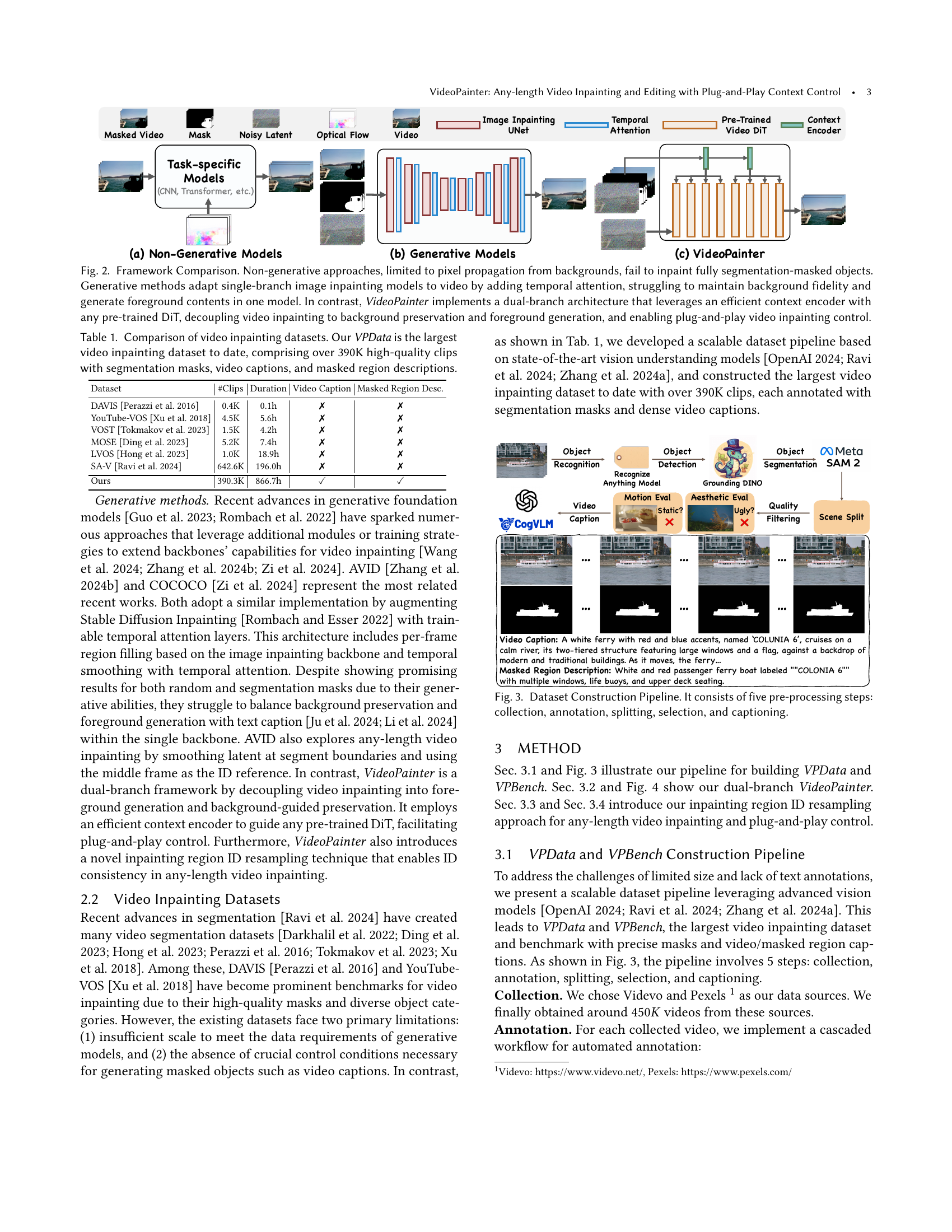
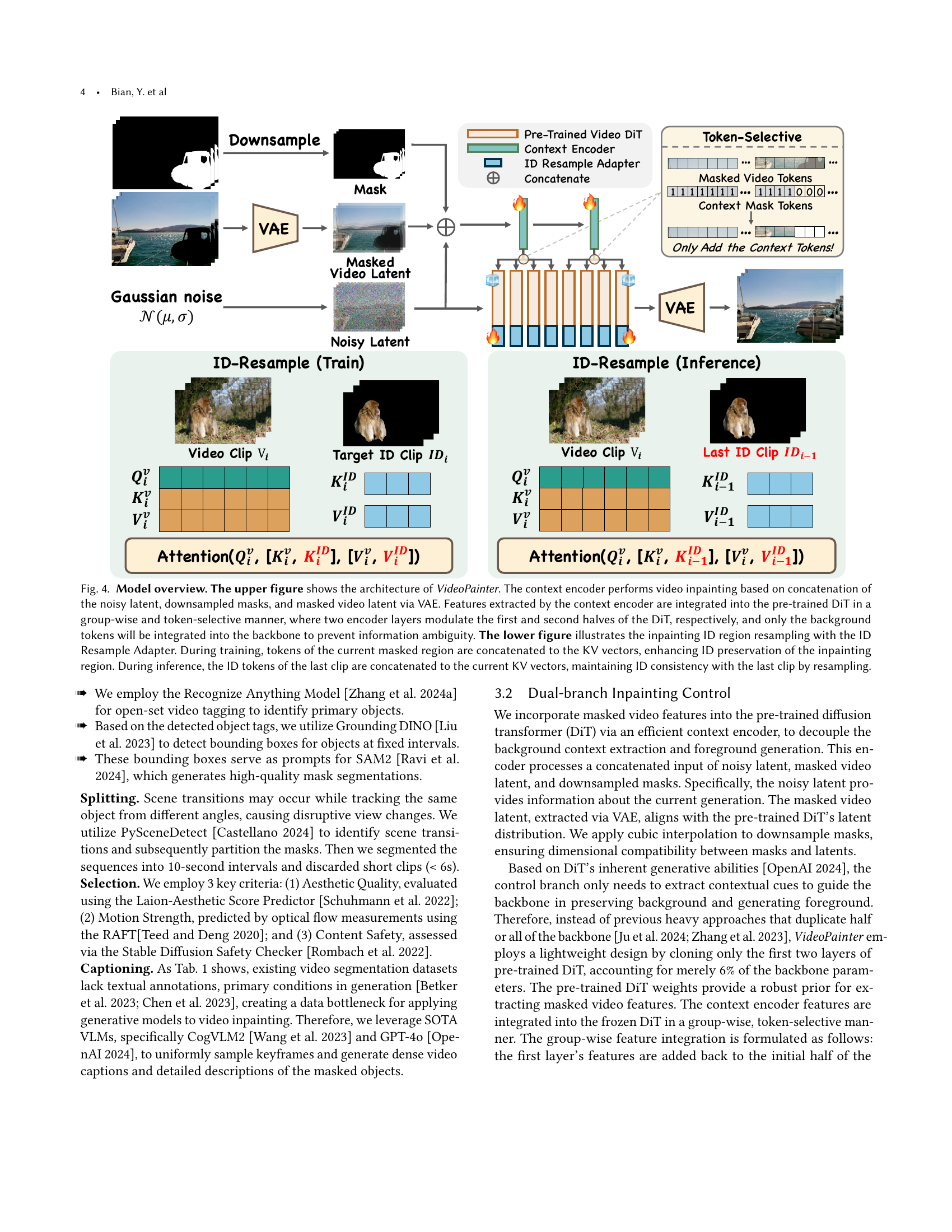
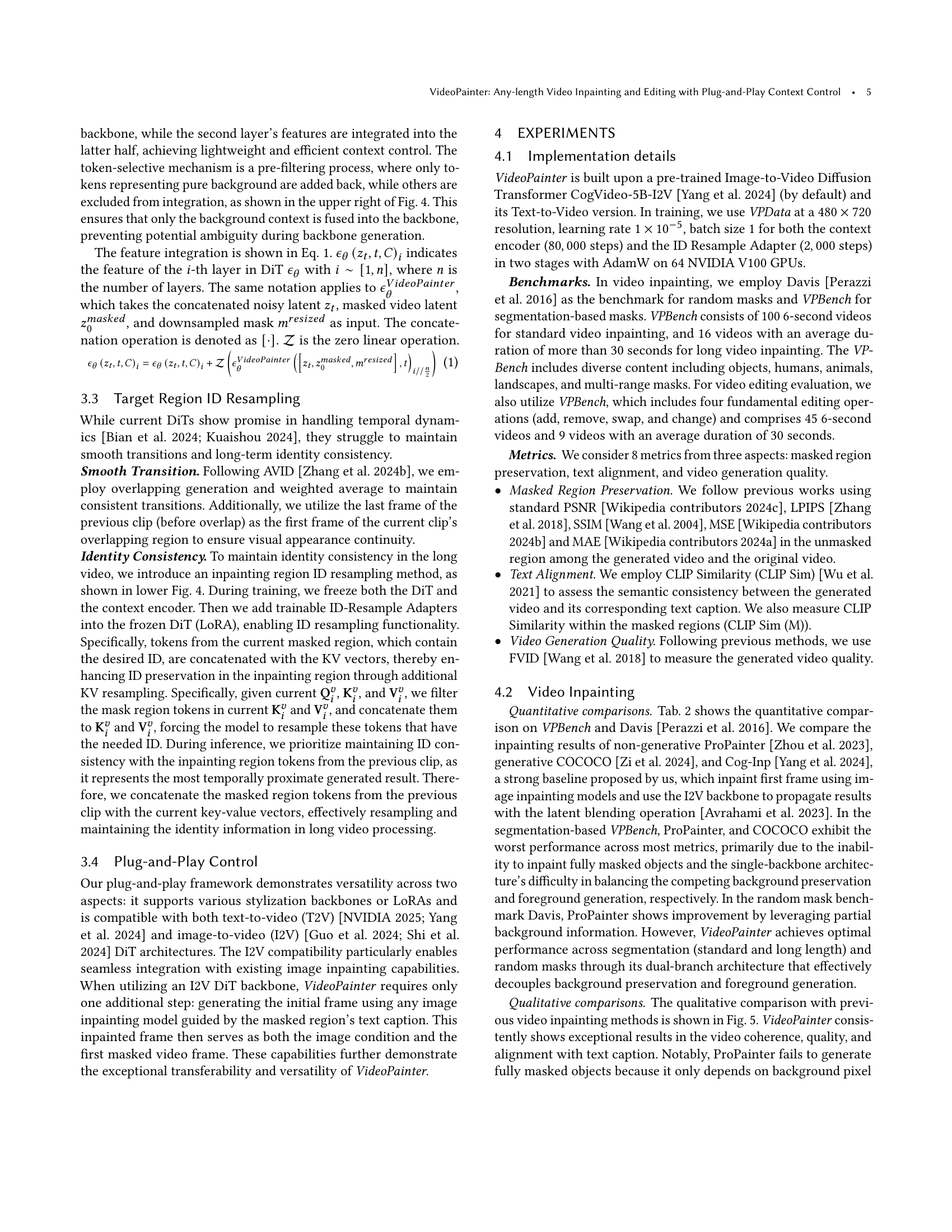
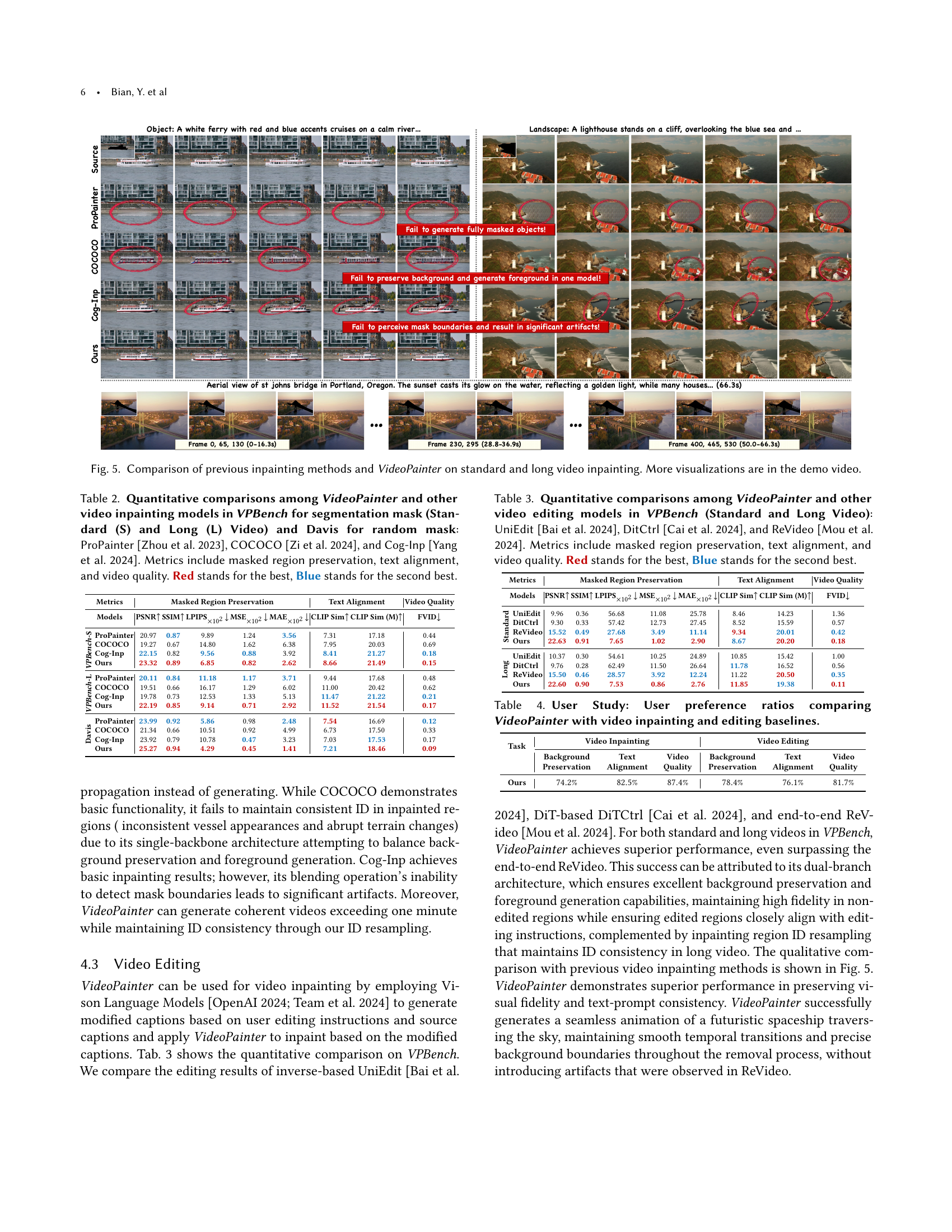
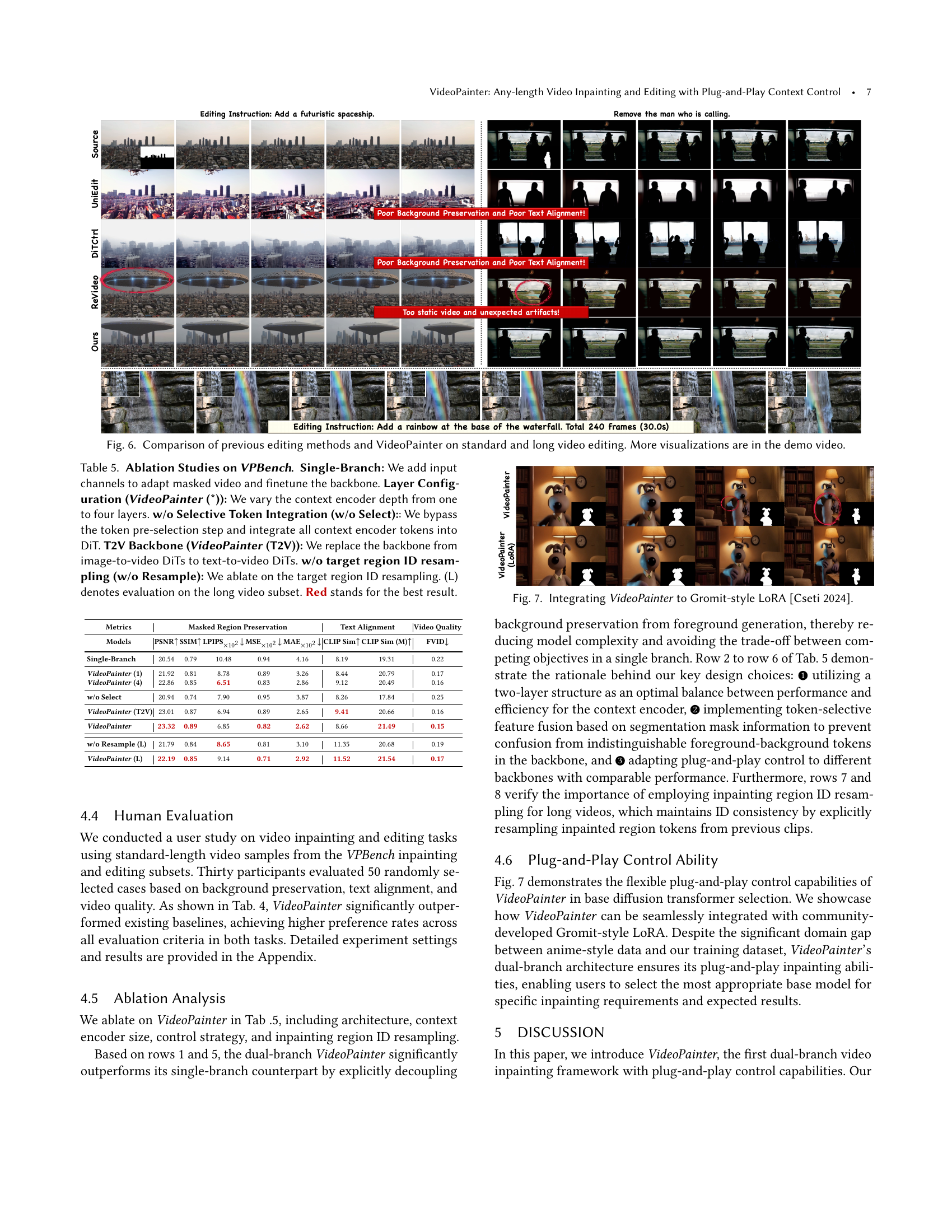
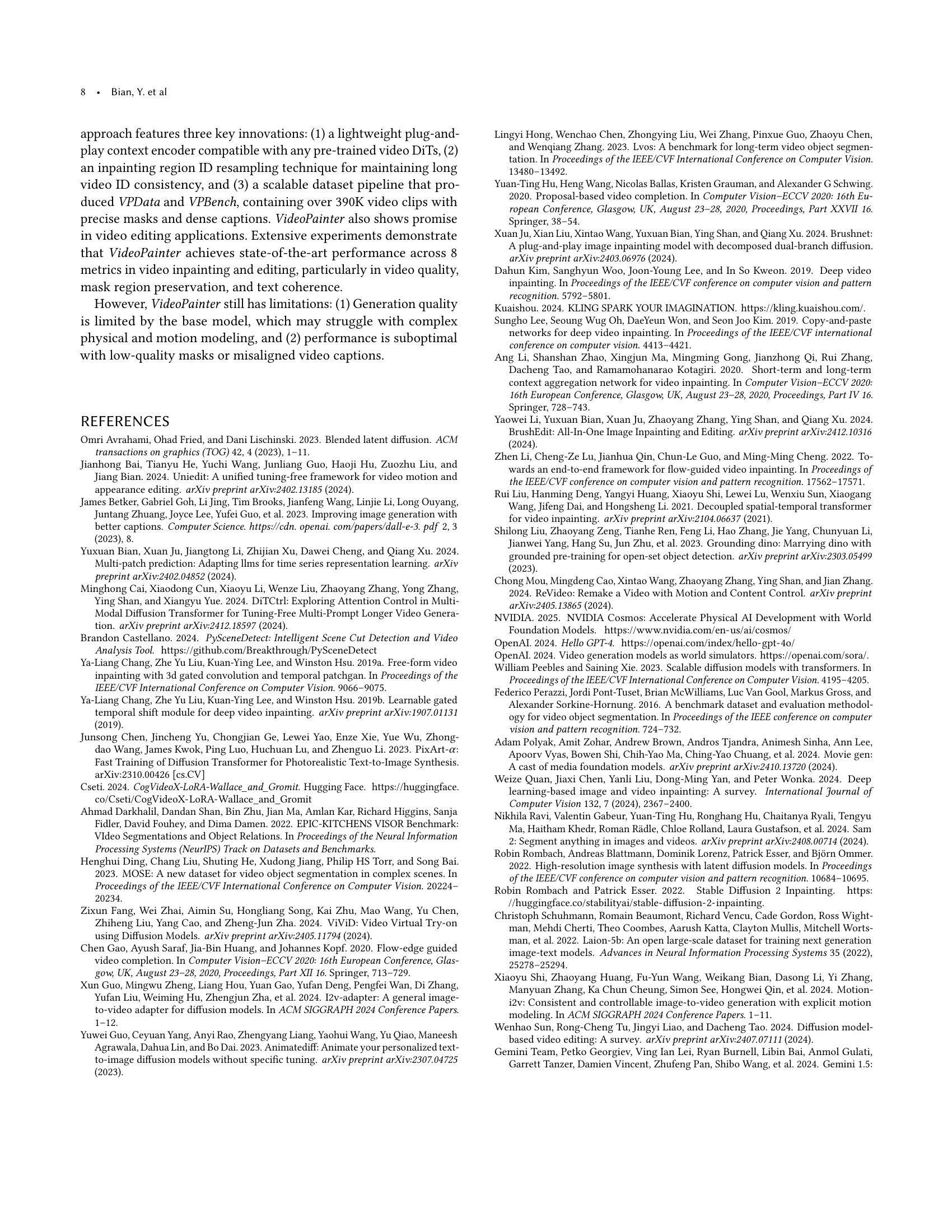
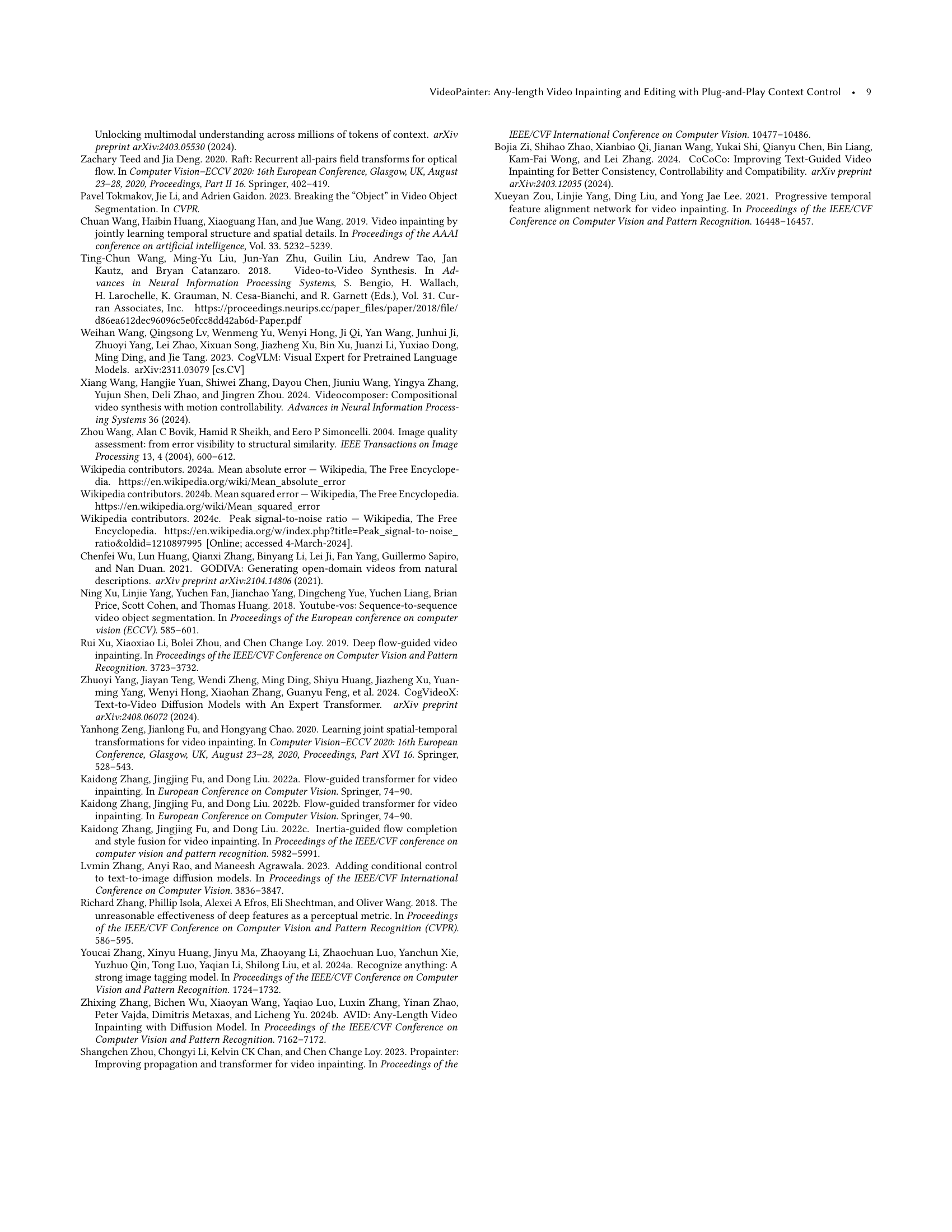
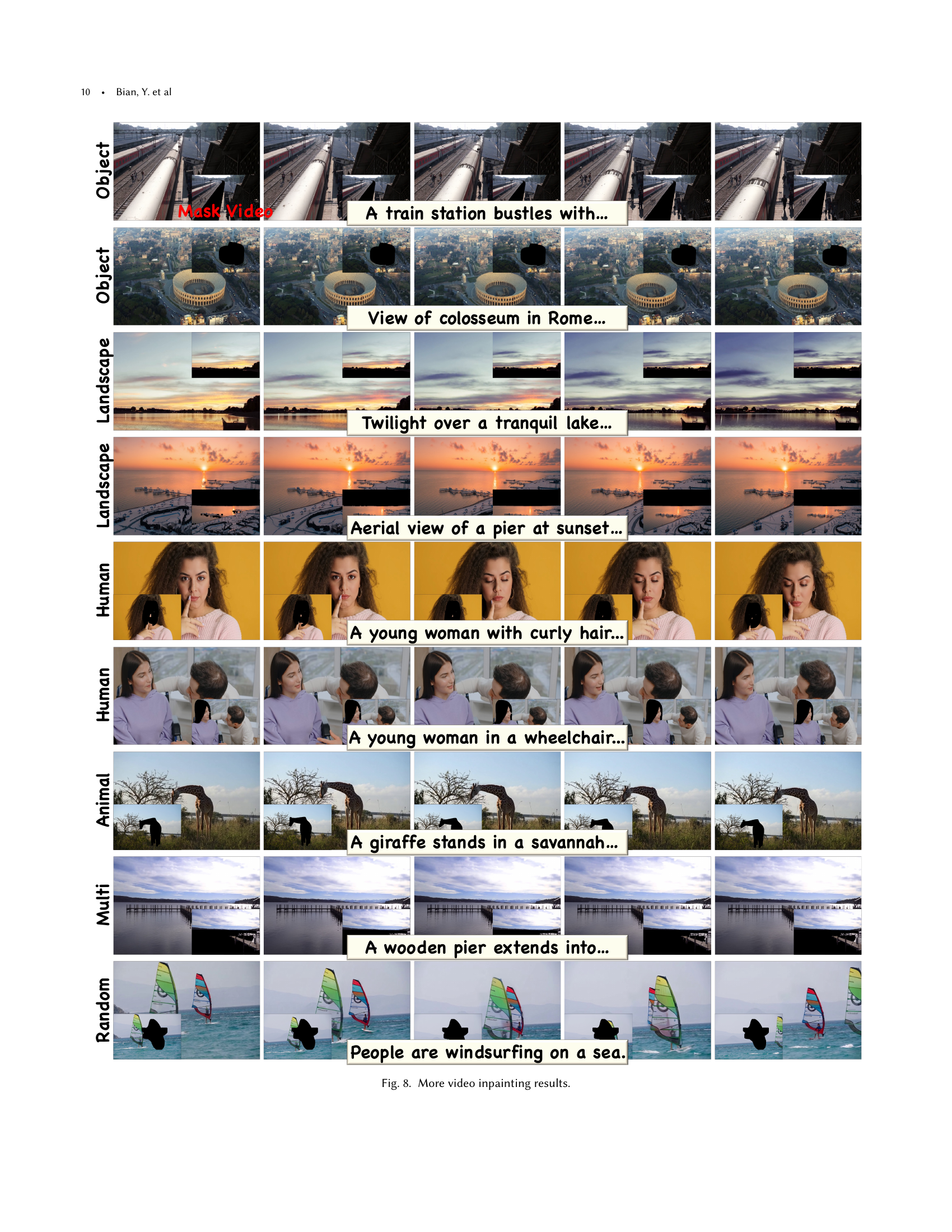
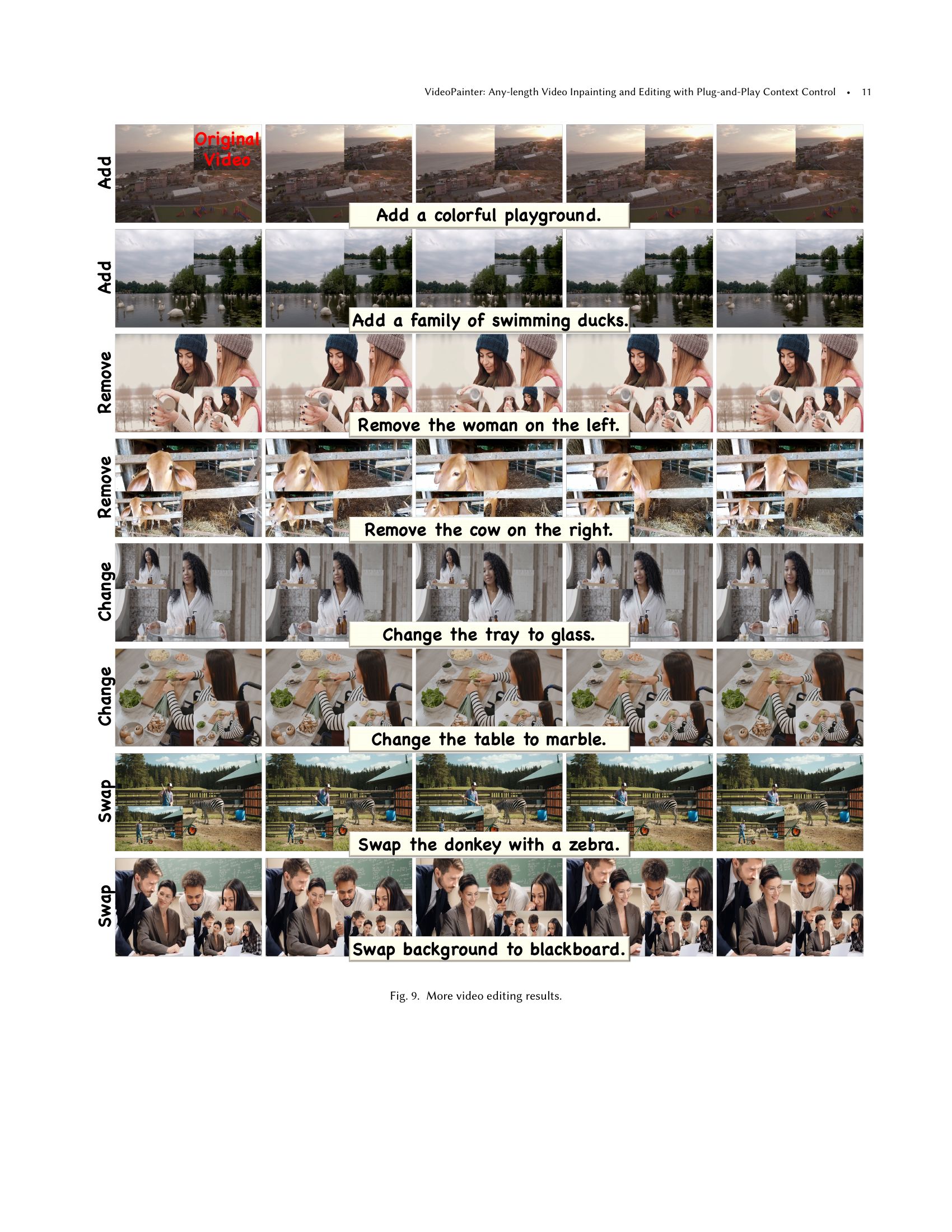