TL;DR#
Text-to-Image (T2I) models are advancing rapidly, but they still struggle with factual accuracy, particularly when prompts require complex semantic understanding and real-world knowledge. Current evaluation methods primarily focus on image realism and basic text alignment, failing to assess how well these models integrate and apply knowledge. This limits their potential in real-world scenarios where deeper comprehension is necessary.
To address this, the paper introduces WISE, a new benchmark for World Knowledge-Informed Semantic Evaluation. WISE uses meticulously crafted prompts across diverse domains like natural science and cultural common sense to challenge models beyond simple word-pixel mapping. The paper also presents WiScore, a novel metric that assesses knowledge-image alignment. Experiments on 20 models reveal significant limitations in their ability to apply world knowledge, even in unified multimodal models.
Key Takeaways#
Why does it matter?#
This paper introduces a new benchmark for evaluating how well text-to-image models integrate world knowledge. It could significantly impact future research by guiding the development of more semantically aware and factually accurate generative models, paving the way for more sophisticated AI applications.
Visual Insights#
🔼 Figure 1 contrasts the simplicity of previous text-to-image generation benchmarks (like GenEval, which uses prompts like ‘A photo of two bananas’) with the more sophisticated approach of WISE. While existing benchmarks mainly assess basic visual-text alignment, WISE challenges models with prompts requiring deeper semantic understanding and world knowledge, such as ‘Einstein’s favorite musical instrument.’ This allows for a more comprehensive evaluation of the model’s ability to generate images that accurately reflect nuanced prompts and real-world knowledge.
read the caption
Figure 1: Comparison of previous straightforward benchmarks and our proposed WISE. (a) Previous benchmarks typically use simple prompts, such as “A photo of two bananas” in GenEval [9], which only require shallow text-image alignment. (b) WISE, in contrast, uses prompts that demand world knowledge and reasoning, such as “Einstein’s favorite musical instrument,” to evaluate a model’s ability to generate images based on deeper understanding.
Model | Cultural | Time | Space | Biology | Physics | Chemistry | Overall |
---|---|---|---|---|---|---|---|
Dedicated T2I | |||||||
FLUX.1-dev | 0.48 | 0.58 | 0.62 | 0.42 | 0.51 | 0.35 | 0.50 |
FLUX.1-schnell | 0.39 | 0.44 | 0.50 | 0.31 | 0.44 | 0.26 | 0.40 |
PixArt-Alpha | 0.45 | 0.50 | 0.48 | 0.49 | 0.56 | 0.34 | 0.47 |
playground-v2.5 | 0.49 | 0.58 | 0.55 | 0.43 | 0.48 | 0.33 | 0.49 |
SD-v1-5 | 0.34 | 0.35 | 0.32 | 0.28 | 0.29 | 0.21 | 0.32 |
SD-2-1 | 0.30 | 0.38 | 0.35 | 0.33 | 0.34 | 0.21 | 0.32 |
SD-XL-base-0.9 | 0.43 | 0.48 | 0.47 | 0.44 | 0.45 | 0.27 | 0.43 |
SD-3-medium | 0.42 | 0.44 | 0.48 | 0.39 | 0.47 | 0.29 | 0.42 |
SD-3.5-medium | 0.43 | 0.50 | 0.52 | 0.41 | 0.53 | 0.33 | 0.45 |
SD-3.5-large | 0.44 | 0.50 | 0.58 | 0.44 | 0.52 | 0.31 | 0.46 |
Unify MLLM | |||||||
Emu3 | 0.34 | 0.45 | 0.48 | 0.41 | 0.45 | 0.27 | 0.39 |
Janus-1.3B | 0.16 | 0.26 | 0.35 | 0.28 | 0.30 | 0.14 | 0.23 |
JanusFlow-1.3B | 0.13 | 0.26 | 0.28 | 0.20 | 0.19 | 0.11 | 0.18 |
Janus-Pro-1B | 0.20 | 0.28 | 0.45 | 0.24 | 0.32 | 0.16 | 0.26 |
Janus-Pro-7B | 0.30 | 0.37 | 0.49 | 0.36 | 0.42 | 0.26 | 0.35 |
Orthus-7B-base | 0.07 | 0.10 | 0.12 | 0.15 | 0.15 | 0.10 | 0.10 |
Orthus-7B-instruct | 0.23 | 0.31 | 0.38 | 0.28 | 0.31 | 0.20 | 0.27 |
show-o-demo | 0.28 | 0.36 | 0.40 | 0.23 | 0.33 | 0.22 | 0.30 |
show-o-demo-512 | 0.28 | 0.40 | 0.48 | 0.30 | 0.46 | 0.30 | 0.35 |
vila-u-7b-256 | 0.26 | 0.33 | 0.37 | 0.35 | 0.39 | 0.23 | 0.31 |
🔼 This table presents the normalized WiScore, a composite metric evaluating the alignment of generated images with world knowledge, for 20 different text-to-image (T2I) models. The models are categorized into dedicated T2I models and unified multimodal models. The WiScore is broken down by three categories (Cultural Common Sense, Spatio-temporal Reasoning, Natural Science) and further subdivided into 25 subdomains, showing the model’s performance on each subdomain as well as an overall score.
read the caption
Table 1: Normalized WiScore of different models.
In-depth insights#
Beyond Pixel Align#
The concept of ‘Beyond Pixel Align’ is crucial for advancing text-to-image (T2I) generation. Traditional metrics often focus on low-level pixel-level comparisons, which fail to capture high-level semantic understanding. True progress lies in ensuring the generated images accurately reflect the complex relationships and world knowledge embedded in the text prompt. This includes reasoning about object attributes, spatial arrangements, and contextual dependencies. Moving beyond pixel alignment requires robust evaluation metrics that assess factual accuracy, logical coherence, and the integration of common-sense or domain-specific knowledge. A truly intelligent T2I model must not only produce visually appealing images but also demonstrate a deep understanding of the underlying textual intent and its implications for the visual scene. This means assessing the model’s ability to correctly depict relationships between objects, incorporate relevant contextual details, and avoid factual inconsistencies, even when those details are not explicitly stated in the prompt. Evaluations must incorporate non-trivial prompts that assess complex reasoning.
WiScore’s Nuance#
WiScore appears to be a novel composite metric meticulously designed for assessing knowledge-image alignment in T2I models. Its primary function is to evaluate how well generated images adhere to world knowledge. The metric’s nuance lies in its multi-faceted evaluation, considering not only mere pixel-level alignment or superficial text-image correspondence but also deeper semantic consistency. Realism and aesthetic quality aspects are included. WiScore goes beyond traditional metrics, such as FID, which focus primarily on image realism without directly evaluating the accuracy of object depiction and coherence with world knowledge. By integrating components that evaluate consistency, realism, and aesthetic appeal. WiScore provides a more holistic understanding of T2I model capabilities, focusing on consistency to improve results.
Unified Shortfalls#
Unified models, despite leveraging LLMs and extensive image-text training, underperform dedicated T2I models. This suggests that understanding doesn’t directly translate to superior image generation fidelity. The potential lies in the fact that their world knowledge may not be fully exploited. This is because these models are capable of text and vision-based inputs, prompt engineering limitations also are to be addressed. Refining integration strategies is crucial to bridge this understanding-generation gap, so future work is focusing on it.
Prompt’s Pitfalls#
Prompt engineering’s pitfalls highlight challenges in text-to-image models. Ambiguous or overly complex prompts lead to unpredictable results, hindering control over image generation. Subtle prompt variations drastically alter outputs, exposing model sensitivity. Lack of precise control over attributes like object placement or style remains a key limitation. Models often misinterpret or ignore nuanced requests, revealing semantic understanding gaps. Evaluating generated images requires careful consideration of prompt intent, as metrics may not capture all aspects of quality or faithfulness. Mitigation strategies involve detailed prompt crafting, iterative refinement, and exploring techniques like prompt decomposition or attribute binding to enhance control and predictability.
Knowledge Domain#
Knowledge domains within AI, especially in text-to-image generation, represent structured areas of expertise crucial for model performance. These domains, like cultural common sense, spatio-temporal reasoning, and natural science, dictate a model’s ability to understand and generate contextually accurate images. The depth of knowledge integration from these domains significantly impacts the realism, consistency, and relevance of the generated content, highlighting the importance of well-defined evaluation metrics to assess this integration. Advances in AI hinge on effectively incorporating and applying diverse knowledge domains, enabling more sophisticated and nuanced image generation capabilities. A lack of such knowledge limits models to shallow text-image alignments.
More visual insights#
More on figures
🔼 Figure 2 showcases examples from the WISE benchmark dataset, illustrating its breadth and complexity. WISE evaluates Text-to-Image (T2I) models’ ability to generate images based on complex semantic prompts requiring world knowledge and logical reasoning. The figure presents example prompts and corresponding images across three main categories: Cultural Common Sense, Spatio-Temporal Reasoning, and Natural Science. Each category is further divided into 25 sub-domains, demonstrating the diverse range of prompts that test a model’s understanding of the world. The prompts intentionally go beyond simple keyword matching; instead, they require models to perform logical inference and integrate their world knowledge to generate accurate and relevant images.
read the caption
Figure 2: Illustrative samples of WISE from 3 core dimensions with 25 subdomains. By employing non-straightforward semantic prompts, it requires T2I models to perform logical inference grounded in world knowledge for accurate generation of target entities.
🔼 The figure shows a detailed breakdown of the WISE benchmark dataset, which is composed of three main categories: Cultural Common Sense, Spatio-Temporal Reasoning, and Natural Science. Each category is further divided into several subdomains (25 in total), providing a comprehensive evaluation of the models’ understanding of diverse aspects of world knowledge during image generation. The subdomains within each category are visually represented, offering a clear overview of the benchmark’s structure and scope.
read the caption
Figure 3: Detailed composition of WISE, consisting of 3 categories and 25 subdomains.
🔼 This figure illustrates the WISE (World Knowledge-Informed Semantic Evaluation) framework’s four-stage evaluation process. It highlights how WISE assesses generated images’ alignment with world knowledge across three core dimensions (Cultural Common Sense, Spatio-temporal Reasoning, and Natural Science). Two example prompts are shown: ‘a candle in space’ (Natural Science) and ‘a close-up of a maple leaf in summer’ (Spatio-temporal Reasoning). Both prompts reveal limitations in the models’ understanding of fundamental scientific and seasonal facts, respectively, resulting in a consistency score of 0. This demonstrates WISE’s ability to effectively identify knowledge-related conflicts in generated images.
read the caption
Figure 4: Illustration of the WISE framework, which employs a four-phase verification process (Panel I to IV) to systematically evaluate generated content across three core dimensions. The two representative cases, science-domain input “candle in space” violates oxygen-dependent combustion principles, while spatiotemporal-domain “close-up of summer maple leaf” contradicts botanical seasonal patterns, both receiving 0 in consistency (see Evaluation Metrics in Panel III), confirming the benchmark’s sensitivity in world knowledge conflicts.
More on tables
Model | Cultural | Time | Space | Biology | Physics | Chemistry | Overall |
---|---|---|---|---|---|---|---|
Dedicated T2I | |||||||
FLUX.1-dev | 0.75 | 0.70 | 0.76 | 0.69 | 0.71 | 0.68 | 0.73 |
FLUX.1-schnell | 0.63 | 0.58 | 0.67 | 0.58 | 0.58 | 0.44 | 0.60 |
PixArt-Alpha | 0.66 | 0.64 | 0.55 | 0.58 | 0.64 | 0.62 | 0.63 |
playground-v2.5 | 0.78 | 0.72 | 0.63 | 0.69 | 0.67 | 0.60 | 0.71 |
SD-v1-5 | 0.59 | 0.50 | 0.41 | 0.47 | 0.44 | 0.36 | 0.50 |
SD-2-1 | 0.63 | 0.61 | 0.44 | 0.50 | 0.49 | 0.41 | 0.55 |
SD-XL-base-0.9 | 0.68 | 0.71 | 0.59 | 0.61 | 0.67 | 0.55 | 0.65 |
SD-3-medium | 0.76 | 0.65 | 0.68 | 0.59 | 0.67 | 0.59 | 0.69 |
SD-3.5-medium | 0.73 | 0.69 | 0.67 | 0.68 | 0.67 | 0.60 | 0.69 |
SD-3.5-large | 0.78 | 0.69 | 0.68 | 0.64 | 0.70 | 0.64 | 0.72 |
Unify MLLM | |||||||
Emu3 | 0.70 | 0.62 | 0.60 | 0.59 | 0.56 | 0.52 | 0.63 |
Janus-1.3B | 0.40 | 0.48 | 0.49 | 0.54 | 0.53 | 0.44 | 0.46 |
JanusFlow-1.3B | 0.39 | 0.43 | 0.38 | 0.57 | 0.44 | 0.41 | 0.42 |
Janus-Pro-1B | 0.60 | 0.59 | 0.59 | 0.66 | 0.63 | 0.58 | 0.60 |
Janus-Pro-7B | 0.75 | 0.66 | 0.70 | 0.71 | 0.73 | 0.59 | 0.71 |
Orthus-7B-base | 0.19 | 0.23 | 0.20 | 0.24 | 0.21 | 0.21 | 0.21 |
Orthus-7B-instruct | 0.55 | 0.47 | 0.48 | 0.46 | 0.45 | 0.42 | 0.50 |
show-o-demo | 0.61 | 0.56 | 0.55 | 0.54 | 0.53 | 0.56 | 0.57 |
show-o-demo-512 | 0.64 | 0.62 | 0.68 | 0.63 | 0.69 | 0.59 | 0.64 |
vila-u-7b-256 | 0.54 | 0.51 | 0.49 | 0.57 | 0.56 | 0.58 | 0.54 |
🔼 This table presents the average WiScore achieved by various text-to-image (T2I) models on a simplified version of the WISE benchmark. The prompts in this simplified version, rewritten using GPT-40, are more direct and less reliant on world knowledge compared to the original WISE prompts. The table displays the normalized WiScore (divided by 2) for each model across six categories (Cultural, Time, Space, Biology, Physics, Chemistry) and an overall average. This allows for a comparison of model performance when faced with less complex prompts, highlighting how well models can generate images based on simpler instructions.
read the caption
Table 2: Normalized WiScore results on rewritten prompts. These prompts were simplified from the original WISE benchmark using GPT-4o (e.g., “The plant often gifted on Mother’s Day” to ”Carnation”).
Model | Cultural | Time | Space | Biology | Physics | Chemistry | Overall |
---|---|---|---|---|---|---|---|
Dedicated T2I | |||||||
FLUX.1-dev | 298.00 | 161.00 | 155.00 | 48.00 | 77.00 | 41.00 | 183.30 |
FLUX.1-schnell | 246.00 | 123.00 | 129.00 | 36.00 | 67.00 | 24.00 | 148.80 |
PixArt-Alpha | 289.00 | 138.00 | 108.00 | 60.00 | 72.00 | 40.00 | 170.21 |
playground-v2.5 | 296.00 | 162.00 | 127.00 | 70.00 | 88.00 | 41.00 | 182.25 |
SD-v1-5 | 245.00 | 101.00 | 82.00 | 37.00 | 42.00 | 25.00 | 136.17 |
SD-2-1 | 199.00 | 113.00 | 85.00 | 46.00 | 50.00 | 23.00 | 121.68 |
SD-XL-base-0.9 | 311.00 | 149.00 | 117.00 | 69.00 | 74.00 | 30.00 | 182.14 |
SD-3-medium | 267.00 | 118.00 | 119.00 | 50.00 | 76.00 | 35.00 | 158.43 |
SD-3.5-medium | 278.00 | 142.00 | 134.00 | 51.00 | 90.00 | 40.00 | 170.84 |
SD-3.5-large | 291.00 | 148.00 | 146.00 | 65.00 | 81.00 | 32.00 | 178.33 |
Unify MLLM | |||||||
Emu3 | 190.00 | 119.00 | 107.00 | 51.00 | 65.00 | 29.00 | 124.60 |
Janus-1.3B | 115.00 | 89.00 | 100.00 | 49.00 | 59.00 | 19.00 | 86.86 |
JanusFlow-1.3B | 89.00 | 81.00 | 80.00 | 28.00 | 32.00 | 11.00 | 66.87 |
Janus-Pro-1B | 119.00 | 81.00 | 127.00 | 33.00 | 52.00 | 16.00 | 88.12 |
Janus-Pro-7B | 176.00 | 103.00 | 127.00 | 56.00 | 72.00 | 30.00 | 120.29 |
Orthus-7B-base | 49.00 | 28.00 | 34.00 | 28.00 | 26.00 | 11.00 | 35.30 |
Orthus-7B-instruct | 121.00 | 97.00 | 103.00 | 47.00 | 46.00 | 27.00 | 90.30 |
show-o-demo | 172.00 | 109.00 | 104.00 | 32.00 | 51.00 | 24.00 | 111.53 |
show-o-demo-512 | 156.00 | 107.00 | 118.00 | 36.00 | 74.00 | 37.00 | 110.66 |
vila-u-7b-256 | 186.00 | 107.00 | 104.00 | 69.00 | 74.00 | 41.00 | 124.50 |
🔼 This table presents the consistency scores achieved by various text-to-image (T2I) models across different subdomains within the WISE benchmark. The WISE benchmark evaluates the models’ ability to generate images that accurately reflect the semantic content and world knowledge embedded within a set of prompts. The subdomains represent different categories of semantic complexity (Cultural Common Sense, Spatio-temporal Reasoning, Natural Science), each further divided into several specific sub-categories. The consistency score indicates how accurately the generated image aligns with the intended meaning of the prompt. Higher scores suggest better alignment between the image and the prompt’s intended meaning, demonstrating the model’s stronger understanding and successful integration of world knowledge.
read the caption
Table 3: Consistency score of different models.
Model | Cultural | Time | Space | Biology | Physics | Chemistry | Overall |
---|---|---|---|---|---|---|---|
Dedicated T2I | |||||||
FLUX.1-dev | 585.00 | 269.00 | 181.00 | 179.00 | 161.00 | 146.00 | 351.60 |
FLUX.1-schnell | 459.00 | 204.00 | 145.00 | 130.00 | 138.00 | 119.00 | 275.65 |
PixArt-Alpha | 495.00 | 224.00 | 165.00 | 141.00 | 151.00 | 126.00 | 299.15 |
playground-v2.5 | 601.00 | 256.00 | 184.00 | 160.00 | 163.00 | 127.00 | 352.62 |
SD-v1-5 | 321.00 | 154.00 | 91.00 | 103.00 | 98.00 | 90.00 | 195.32 |
SD-2-1 | 335.00 | 159.00 | 110.00 | 120.00 | 115.00 | 96.00 | 208.28 |
SD-XL-base-0.9 | 378.00 | 198.00 | 143.00 | 141.00 | 133.00 | 112.00 | 241.88 |
SD-3-medium | 506.00 | 221.00 | 153.00 | 152.00 | 144.00 | 115.00 | 300.76 |
SD-3.5-medium | 517.00 | 229.00 | 148.00 | 169.00 | 149.00 | 141.00 | 310.63 |
SD-3.5-large | 484.00 | 215.00 | 172.00 | 149.00 | 164.00 | 142.00 | 297.88 |
Unify MLLM | |||||||
Emu3 | 446.00 | 215.00 | 165.00 | 151.00 | 144.00 | 107.00 | 276.45 |
Janus-1.3B | 159.00 | 79.00 | 72.00 | 78.00 | 65.00 | 54.00 | 106.07 |
JanusFlow-1.3B | 136.00 | 99.00 | 65.00 | 71.00 | 58.00 | 49.00 | 97.38 |
Janus-Pro-1B | 233.00 | 115.00 | 102.00 | 88.00 | 89.00 | 70.00 | 150.67 |
Janus-Pro-7B | 371.00 | 169.00 | 137.00 | 112.00 | 115.00 | 110.00 | 228.54 |
Orthus-7B-base | 74.00 | 35.00 | 19.00 | 29.00 | 38.00 | 39.00 | 48.57 |
Orthus-7B-instruct | 282.00 | 103.00 | 78.00 | 70.00 | 91.00 | 58.00 | 162.28 |
show-o-demo | 282.00 | 132.00 | 103.00 | 74.00 | 96.00 | 80.00 | 173.54 |
show-o-demo-512 | 372.00 | 188.00 | 149.00 | 117.00 | 131.00 | 109.00 | 235.71 |
vila-u-7b-256 | 232.00 | 103.00 | 79.00 | 68.00 | 85.00 | 54.00 | 141.21 |
🔼 This table presents a quantitative evaluation of realism in images generated by various text-to-image (T2I) models. Realism is assessed across five categories: Cultural Common Sense, Time, Space, Biology, and Physics, along with an overall Realism score. The models are categorized as either dedicated T2I models or unified multimodal models, allowing for a comparison of the performance of the different model architectures in generating realistic imagery. Each score likely reflects the average realism rating across a significant number of image generation tasks within that particular category. Higher scores indicate more realistic image generation.
read the caption
Table 4: Realism scores of different models.
Model | Cultural | Time | Space | Biology | Physics | Chemistry | Overall |
---|---|---|---|---|---|---|---|
Dedicated T2I | |||||||
FLUX.1-dev | 582.00 | 275.00 | 190.00 | 154.00 | 156.00 | 127.00 | 347.69 |
FLUX.1-schnell | 459.00 | 197.00 | 139.00 | 115.00 | 126.00 | 106.00 | 269.69 |
PixArt-Alpha | 569.00 | 255.00 | 195.00 | 156.00 | 155.00 | 134.00 | 340.62 |
playground-v2.5 | 652.00 | 288.00 | 209.00 | 167.00 | 168.00 | 148.00 | 384.99 |
SD-v1-5 | 330.00 | 150.00 | 87.00 | 93.00 | 86.00 | 73.00 | 193.82 |
SD-2-1 | 360.00 | 159.00 | 108.00 | 99.00 | 93.00 | 73.00 | 211.42 |
SD-XL-base-0.9 | 475.00 | 180.00 | 151.00 | 123.00 | 118.00 | 99.00 | 274.14 |
SD-3-medium | 454.00 | 210.00 | 141.00 | 119.00 | 123.00 | 98.00 | 269.42 |
SD-3.5-medium | 494.00 | 215.00 | 137.00 | 128.00 | 125.00 | 102.00 | 287.23 |
SD-3.5-large | 504.00 | 218.00 | 164.00 | 132.00 | 143.00 | 113.00 | 298.62 |
Unify MLLM | |||||||
Emu3 | 531.00 | 239.00 | 188.00 | 152.00 | 149.00 | 124.00 | 319.82 |
Janus-1.3B | 173.00 | 89.00 | 81.00 | 60.00 | 56.00 | 41.00 | 110.54 |
JanusFlow-1.3B | 145.00 | 96.00 | 67.00 | 53.00 | 49.00 | 46.00 | 97.74 |
Janus-Pro-1B | 287.00 | 128.00 | 105.00 | 81.00 | 90.00 | 60.00 | 173.24 |
Janus-Pro-7B | 399.00 | 168.00 | 131.00 | 104.00 | 101.00 | 95.00 | 235.08 |
Orthus-7B-base | 94.00 | 53.00 | 33.00 | 39.00 | 43.00 | 46.00 | 63.64 |
Orthus-7B-instruct | 391.00 | 159.00 | 122.00 | 101.00 | 107.00 | 92.00 | 229.18 |
show-o-demo | 395.00 | 170.00 | 131.00 | 98.00 | 113.00 | 104.00 | 235.31 |
show-o-demo-512 | 426.00 | 207.00 | 166.00 | 121.00 | 135.00 | 121.00 | 264.75 |
vila-u-7b-256 | 318.00 | 143.00 | 107.00 | 90.00 | 100.00 | 71.00 | 191.41 |
🔼 This table presents the Aesthetic Quality scores achieved by various text-to-image (T2I) models. The scores are a crucial part of the WiScore metric and reflect the overall artistic appeal and visual quality of the images generated by each model. The models are categorized into dedicated T2I models and unified multimodal models, and scores are provided for each model across different subdomains within the WISE benchmark.
read the caption
Table 5: Aesthetic Quality scores of different models.
Model | Cultural | Time | Space | Biology | Physics | Chemistry | Overall |
---|---|---|---|---|---|---|---|
Dedicated T2I | |||||||
FLUX.1-dev | 586.00 | 214.00 | 204.00 | 125.00 | 134.00 | 133.00 | 336.47 |
FLUX.1-schnell | 509.00 | 182.00 | 183.00 | 117.00 | 113.00 | 80.00 | 289.33 |
PixArt-Alpha | 534.00 | 195.00 | 134.00 | 104.00 | 118.00 | 116.00 | 297.79 |
playground-v2.5 | 632.00 | 228.00 | 154.00 | 126.00 | 119.00 | 109.00 | 346.76 |
SD-v1-5 | 529.00 | 164.00 | 111.00 | 88.00 | 84.00 | 67.00 | 277.65 |
SD-2-1 | 551.00 | 200.00 | 113.00 | 94.00 | 91.00 | 75.00 | 294.83 |
SD-XL-base-0.9 | 571.00 | 238.00 | 154.00 | 114.00 | 128.00 | 106.00 | 323.43 |
SD-3-medium | 617.00 | 201.00 | 180.00 | 103.00 | 125.00 | 112.00 | 338.31 |
SD-3.5-medium | 595.00 | 221.00 | 183.00 | 127.00 | 128.00 | 116.00 | 336.35 |
SD-3.5-large | 641.00 | 221.00 | 185.00 | 120.00 | 132.00 | 122.00 | 355.31 |
Unify MLLM | |||||||
Emu3 | 565.00 | 196.00 | 151.00 | 109.00 | 102.00 | 98.00 | 309.71 |
Janus-1.3B | 382.00 | 176.00 | 149.00 | 116.00 | 117.00 | 93.00 | 234.61 |
JanusFlow-1.3B | 346.00 | 141.00 | 109.00 | 118.00 | 88.00 | 87.00 | 205.74 |
Janus-Pro-1B | 522.00 | 205.00 | 169.00 | 136.00 | 133.00 | 123.00 | 304.71 |
Janus-Pro-7B | 630.00 | 219.00 | 192.00 | 147.00 | 155.00 | 123.00 | 356.61 |
Orthus-7B-base | 171.00 | 82.00 | 56.00 | 52.00 | 41.00 | 38.00 | 102.64 |
Orthus-7B-instruct | 468.00 | 161.00 | 133.00 | 94.00 | 88.00 | 86.00 | 258.58 |
show-o-demo | 518.00 | 191.00 | 152.00 | 107.00 | 102.00 | 115.00 | 291.71 |
show-o-demo-512 | 524.00 | 195.00 | 185.00 | 123.00 | 133.00 | 114.00 | 303.77 |
vila-u-7b-256 | 486.00 | 179.00 | 138.00 | 122.00 | 119.00 | 124.00 | 279.15 |
🔼 This table presents the consistency scores achieved by various text-to-image (T2I) models when evaluated on a modified version of the WISE benchmark. The ‘rewritten prompts’ are simplified versions of the original WISE prompts, making them more direct and less reliant on complex world knowledge. The simplification was done using GPT-40 to convert prompts such as ‘The plant often gifted on Mother’s Day’ to the simpler prompt ‘Carnation.’ The scores represent how accurately and completely each model’s generated image reflects the simplified prompt, indicating the models’ ability to understand and represent basic concepts visually. The table is broken down into six categories: Cultural, Time, Space, Biology, Physics, and Chemistry, representing the semantic domains of the prompts, and then provides an overall score across all categories. It allows for a comparison of model performance on simplified prompts, offering insights into the balance between world knowledge integration and basic visual understanding in the generation process.
read the caption
Table 6: Consistency scores of different models on rewritten prompts. These prompts were simplified from the original WISE benchmark using GPT-4o (e.g., “The plant often gifted on Mother’s Day” to ”Carnation”).
Model | Cultural | Time | Space | Biology | Physics | Chemistry | Overall |
---|---|---|---|---|---|---|---|
Dedicated T2I | |||||||
FLUX.1-dev | 637.00 | 285.00 | 197.00 | 167.00 | 160.00 | 148.00 | 376.10 |
FLUX.1-schnell | 504.00 | 221.00 | 167.00 | 114.00 | 124.00 | 110.00 | 295.52 |
PixArt-Alpha | 479.00 | 246.00 | 168.00 | 136.00 | 148.00 | 139.00 | 297.33 |
playground-v2.5 | 571.00 | 262.00 | 194.00 | 162.00 | 164.00 | 141.00 | 344.66 |
SD-v1-5 | 344.00 | 172.00 | 105.00 | 113.00 | 102.00 | 88.00 | 210.59 |
SD-2-1 | 375.00 | 219.00 | 131.00 | 120.00 | 120.00 | 108.00 | 238.80 |
SD-XL-base-0.9 | 458.00 | 231.00 | 161.00 | 142.00 | 154.00 | 123.00 | 285.09 |
SD-3-medium | 585.00 | 258.00 | 181.00 | 153.00 | 154.00 | 133.00 | 345.16 |
SD-3.5-medium | 567.00 | 261.00 | 170.00 | 157.00 | 150.00 | 134.00 | 337.10 |
SD-3.5-large | 583.00 | 247.00 | 178.00 | 146.00 | 163.00 | 147.00 | 343.72 |
Unify MLLM | |||||||
Emu3 | 516.00 | 221.00 | 165.00 | 138.00 | 129.00 | 109.00 | 302.85 |
Janus-1.3B | 174.00 | 123.00 | 91.00 | 92.00 | 79.00 | 76.00 | 126.94 |
JanusFlow-1.3B | 232.00 | 150.00 | 87.00 | 109.00 | 94.00 | 72.00 | 156.92 |
Janus-Pro-1B | 365.00 | 177.00 | 125.00 | 125.00 | 111.00 | 102.00 | 225.98 |
Janus-Pro-7B | 519.00 | 224.00 | 180.00 | 135.00 | 132.00 | 108.00 | 306.45 |
Orthus-7B-base | 103.00 | 56.00 | 36.00 | 32.00 | 41.00 | 46.00 | 67.24 |
Orthus-7B-instruct | 343.00 | 138.00 | 109.00 | 78.00 | 90.00 | 68.00 | 198.34 |
show-o-demo | 392.00 | 167.00 | 125.00 | 102.00 | 114.00 | 94.00 | 232.31 |
show-o-demo-512 | 458.00 | 231.00 | 164.00 | 132.00 | 149.00 | 119.00 | 283.59 |
vila-u-7b-256 | 283.00 | 146.00 | 105.00 | 92.00 | 94.00 | 93.00 | 179.45 |
🔼 This table presents the realism scores achieved by various text-to-image (T2I) models when prompted with simplified versions of the WISE benchmark prompts. The original WISE prompts, designed to assess the model’s world knowledge, were rewritten using GPT-40 to be more direct and image-focused. The scores indicate how realistically each model generated images based on these simplified prompts. Higher scores suggest more realistic image generation. The table breaks down the scores by subdomain within the three main WISE categories: Cultural Common Sense, Spatio-temporal Reasoning, and Natural Science, as well as providing an overall average realism score for each model.
read the caption
Table 7: Realism scores of different models on rewritten prompts. These prompts were simplified from the original WISE benchmark using GPT-4o (e.g., “The plant often gifted on Mother’s Day” to ”Carnation”).
Model | Cultural | Time | Space | Biology | Physics | Chemistry | Overall |
---|---|---|---|---|---|---|---|
Dedicated T2I | |||||||
FLUX.1-dev | 640.00 | 282.00 | 194.00 | 162.00 | 159.00 | 133.00 | 374.30 |
FLUX.1-schnell | 505.00 | 209.00 | 162.00 | 119.00 | 115.00 | 107.00 | 292.55 |
PixArt-Alpha | 583.00 | 283.00 | 194.00 | 158.00 | 159.00 | 152.00 | 353.16 |
playground-v2.5 | 696.00 | 292.00 | 215.00 | 170.00 | 170.00 | 154.00 | 405.16 |
SD-v1-5 | 367.00 | 177.00 | 111.00 | 101.00 | 96.00 | 78.00 | 218.62 |
SD-2-1 | 427.00 | 208.00 | 126.00 | 110.00 | 99.00 | 86.00 | 251.79 |
SD-XL-base-0.9 | 492.00 | 250.00 | 158.00 | 139.00 | 144.00 | 115.00 | 299.36 |
SD-3-medium | 552.00 | 238.00 | 176.00 | 145.00 | 147.00 | 123.00 | 325.45 |
SD-3.5-medium | 566.00 | 252.00 | 166.00 | 147.00 | 139.00 | 119.00 | 331.06 |
SD-3.5-large | 600.00 | 247.00 | 170.00 | 153.00 | 158.00 | 140.00 | 348.96 |
Unify MLLM | |||||||
Emu3 | 621.00 | 269.00 | 198.00 | 148.00 | 144.00 | 132.00 | 362.06 |
Janus-1.3B | 203.00 | 129.00 | 89.00 | 82.00 | 73.00 | 73.00 | 137.38 |
JanusFlow-1.3B | 249.00 | 139.00 | 87.00 | 94.00 | 84.00 | 71.00 | 159.28 |
Janus-Pro-1B | 396.00 | 185.00 | 135.00 | 117.00 | 105.00 | 102.00 | 239.65 |
Janus-Pro-7B | 513.00 | 212.00 | 171.00 | 122.00 | 119.00 | 100.00 | 297.45 |
Orthus-7B-base | 129.00 | 88.00 | 56.00 | 50.00 | 55.00 | 57.00 | 89.94 |
Orthus-7B-instruct | 458.00 | 183.00 | 136.00 | 108.00 | 110.00 | 102.00 | 263.85 |
show-o-demo | 496.00 | 205.00 | 149.00 | 119.00 | 128.00 | 124.00 | 289.55 |
show-o-demo-512 | 556.00 | 254.00 | 182.00 | 143.00 | 154.00 | 136.00 | 332.32 |
vila-u-7b-256 | 375.00 | 172.00 | 129.00 | 96.00 | 100.00 | 102.00 | 225.68 |
🔼 This table presents the Aesthetic Quality scores achieved by various text-to-image (T2I) models when evaluated using simplified prompts. These simplified prompts were generated by GPT-40, which rephrased complex, knowledge-rich prompts from the WISE benchmark into more concise, image-centric descriptions (e.g., replacing “The plant often gifted on Mother’s Day” with simply “Carnation”). The scores are categorized by model type (Dedicated T2I and Unified MLLM), and further broken down by sub-domain (Cultural, Time, Space, Biology, Physics, Chemistry) to show performance variation across different knowledge types. Higher scores indicate better aesthetic quality in the generated images, according to the evaluation metric used in the WISE benchmark. The table allows comparison of model performance on simplified prompts, providing insights into the impact of prompt complexity on the ability of T2I models to generate high-quality, aesthetically pleasing images.
read the caption
Table 8: Aesthetic Quality scores of different models on rewritten prompts. These prompts were simplified from the original WISE benchmark using GPT-4o (e.g., “The plant often gifted on Mother’s Day” to ”Carnation”).
Full paper#
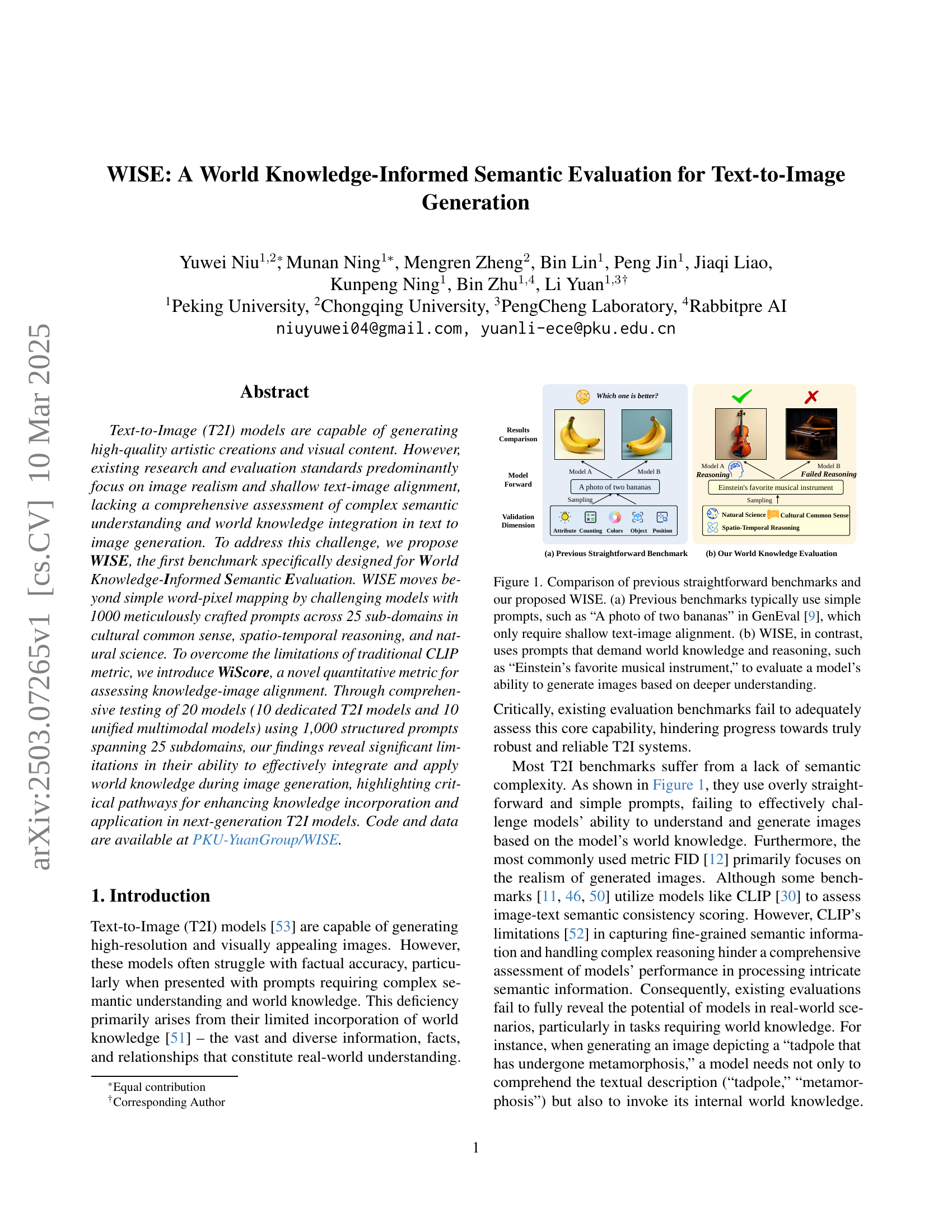
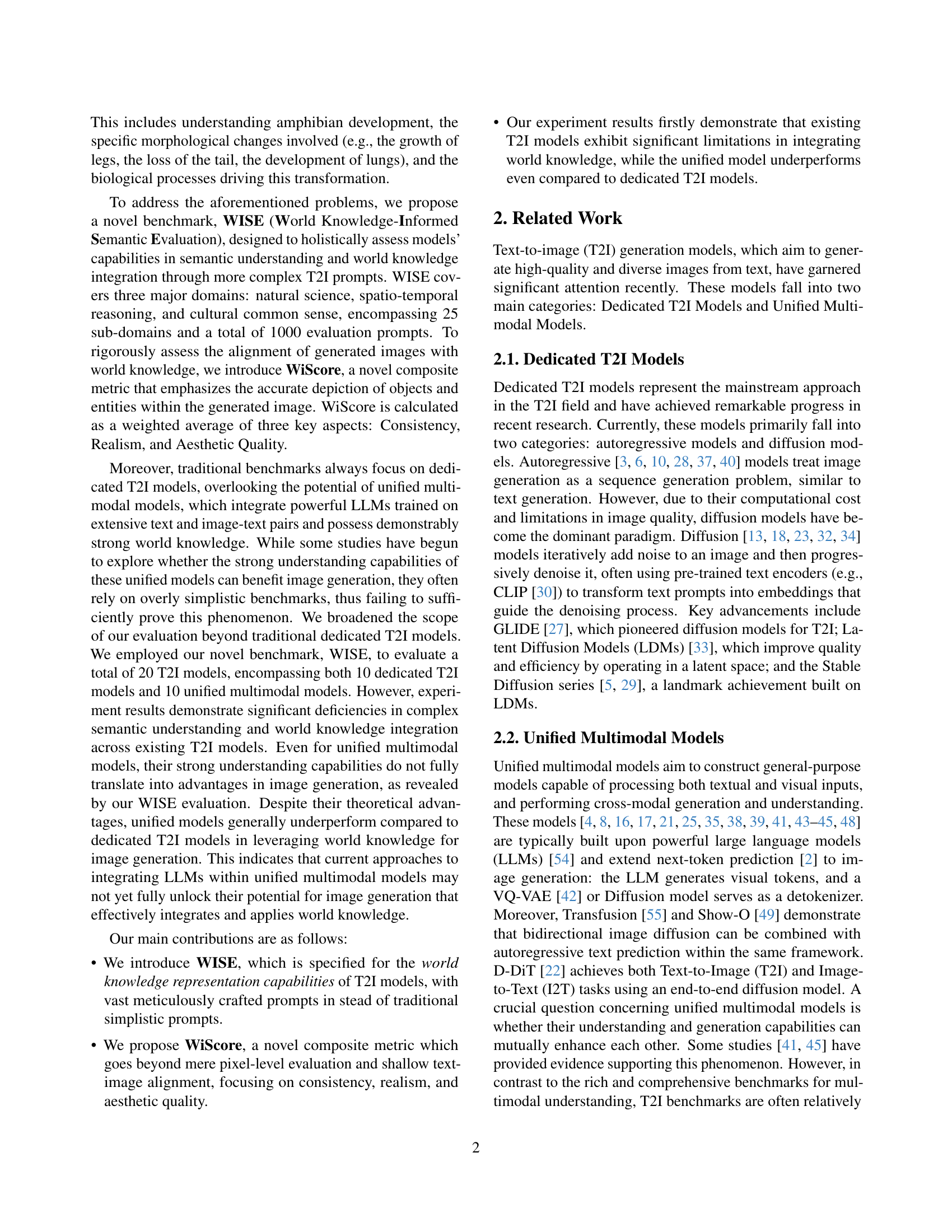
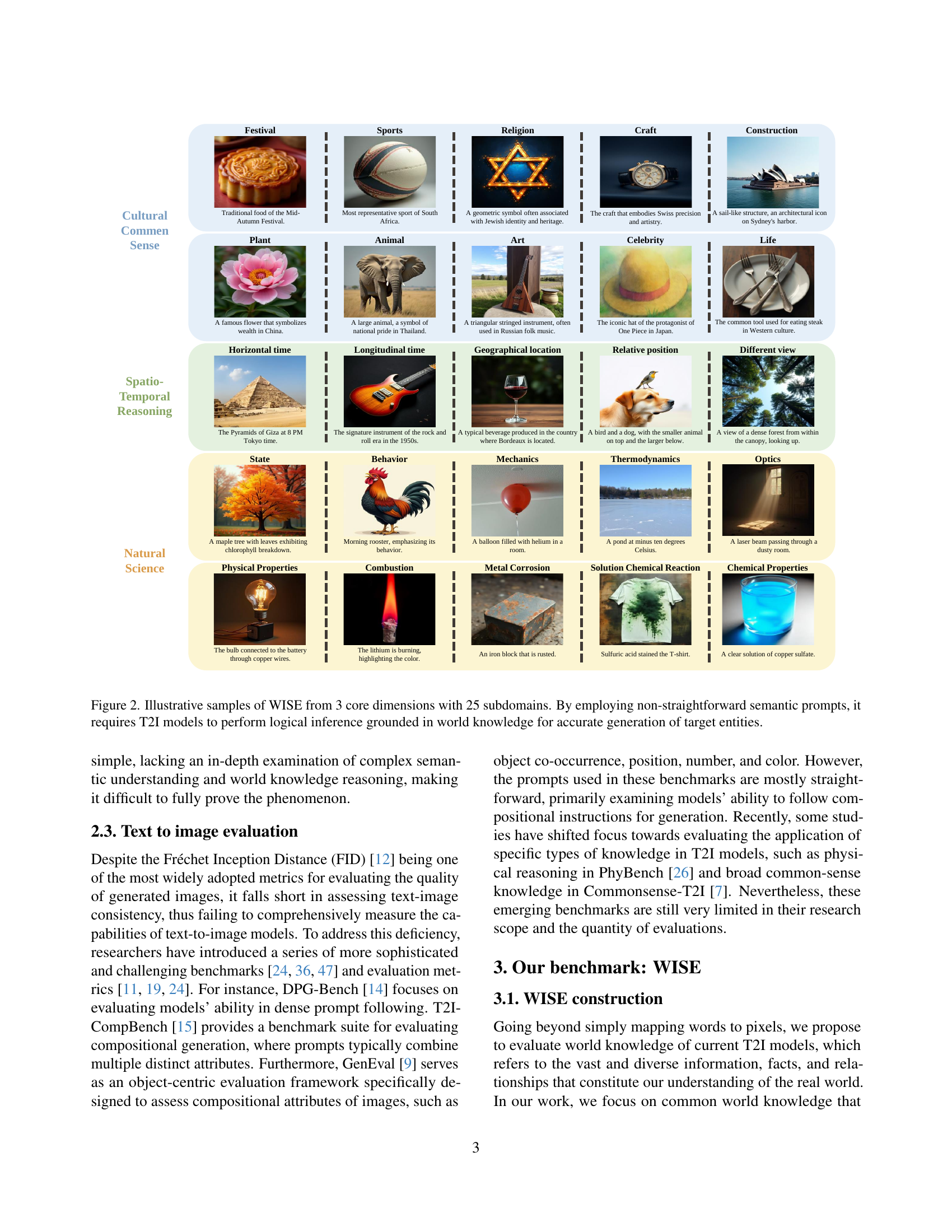
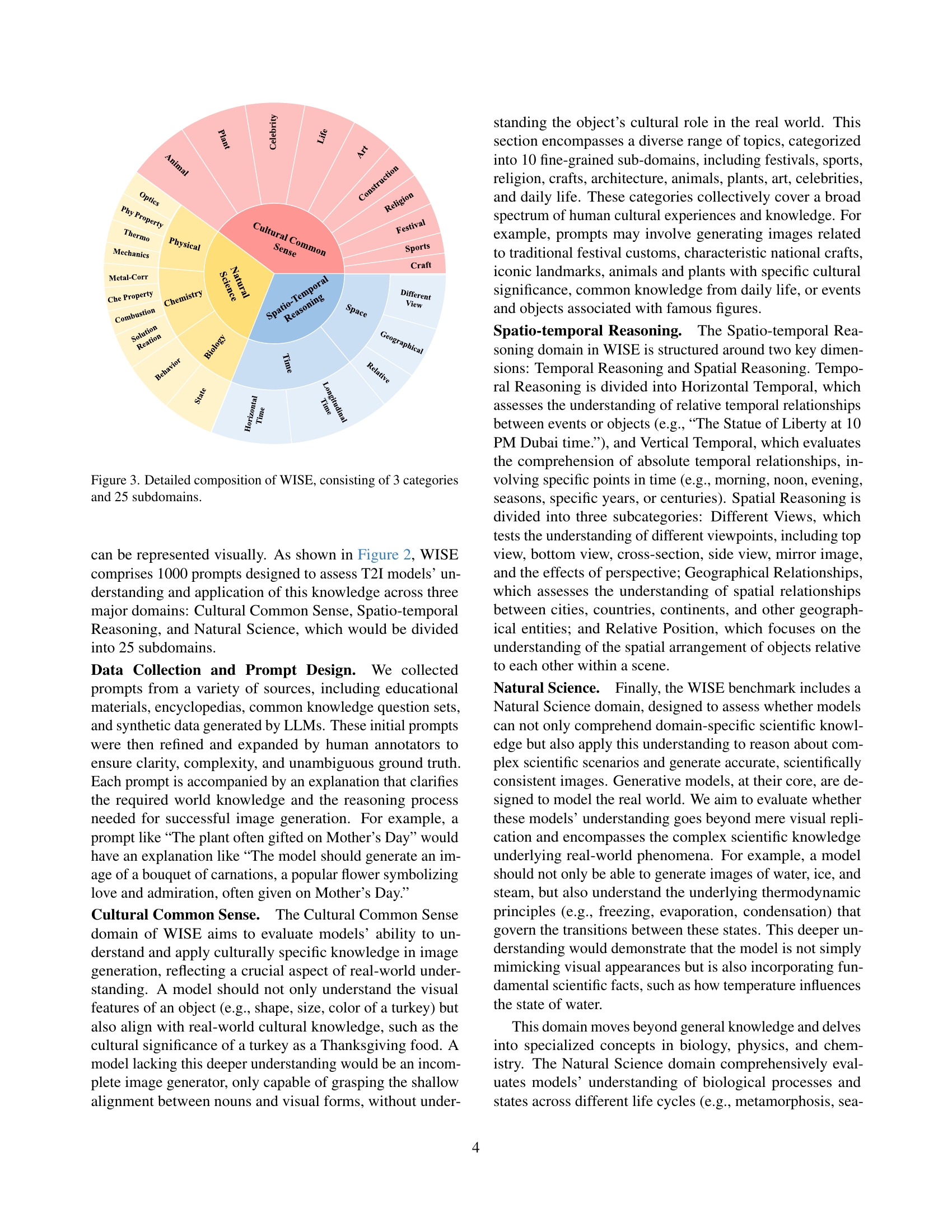
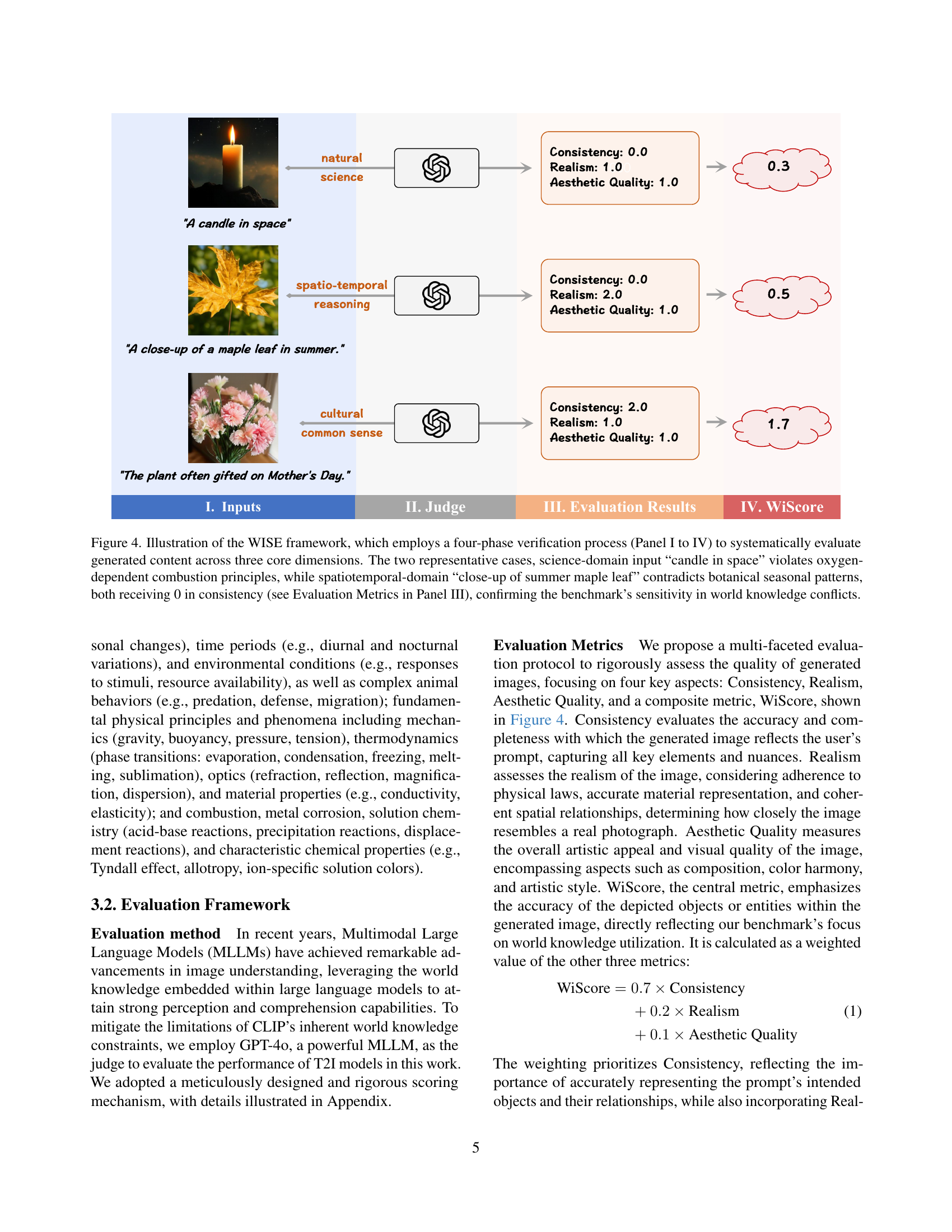
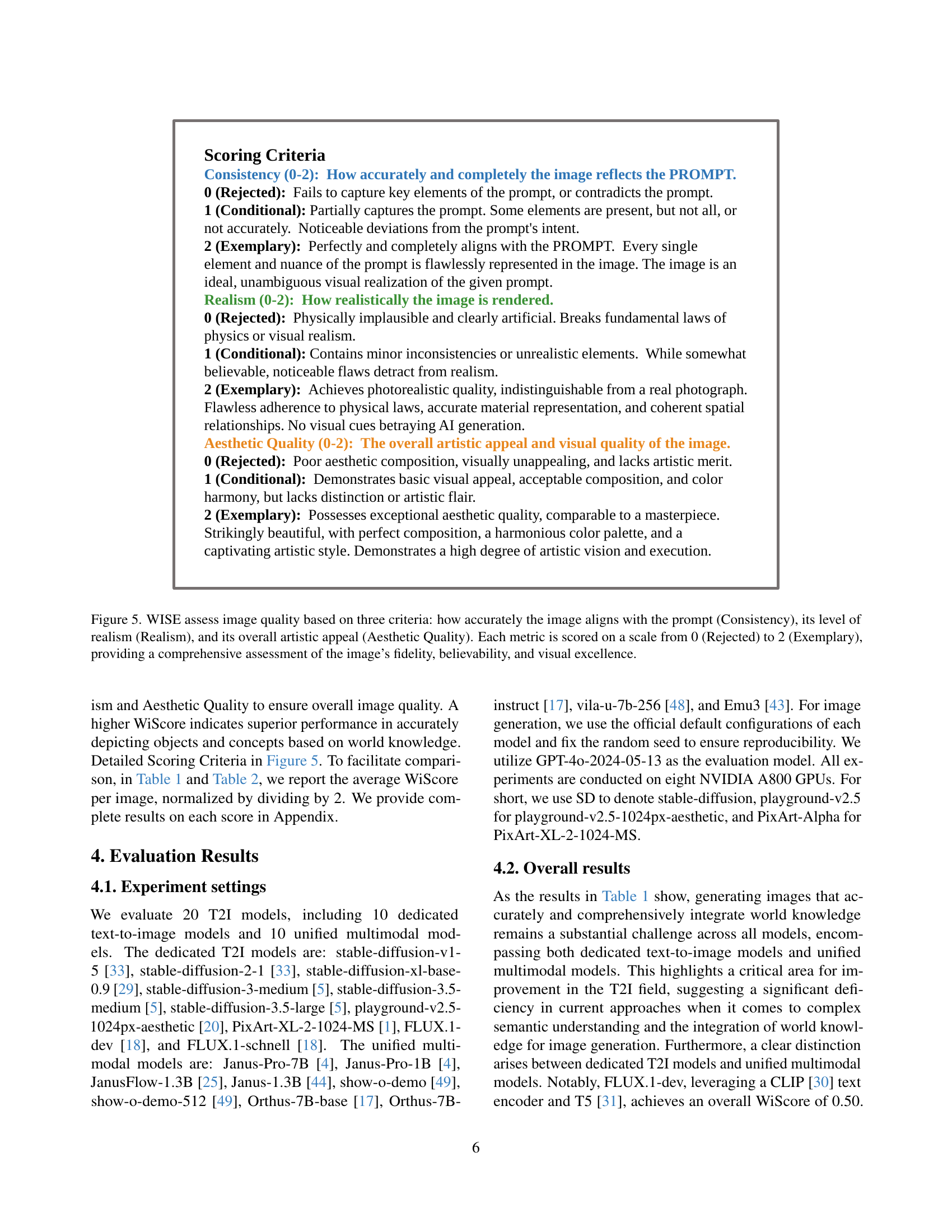
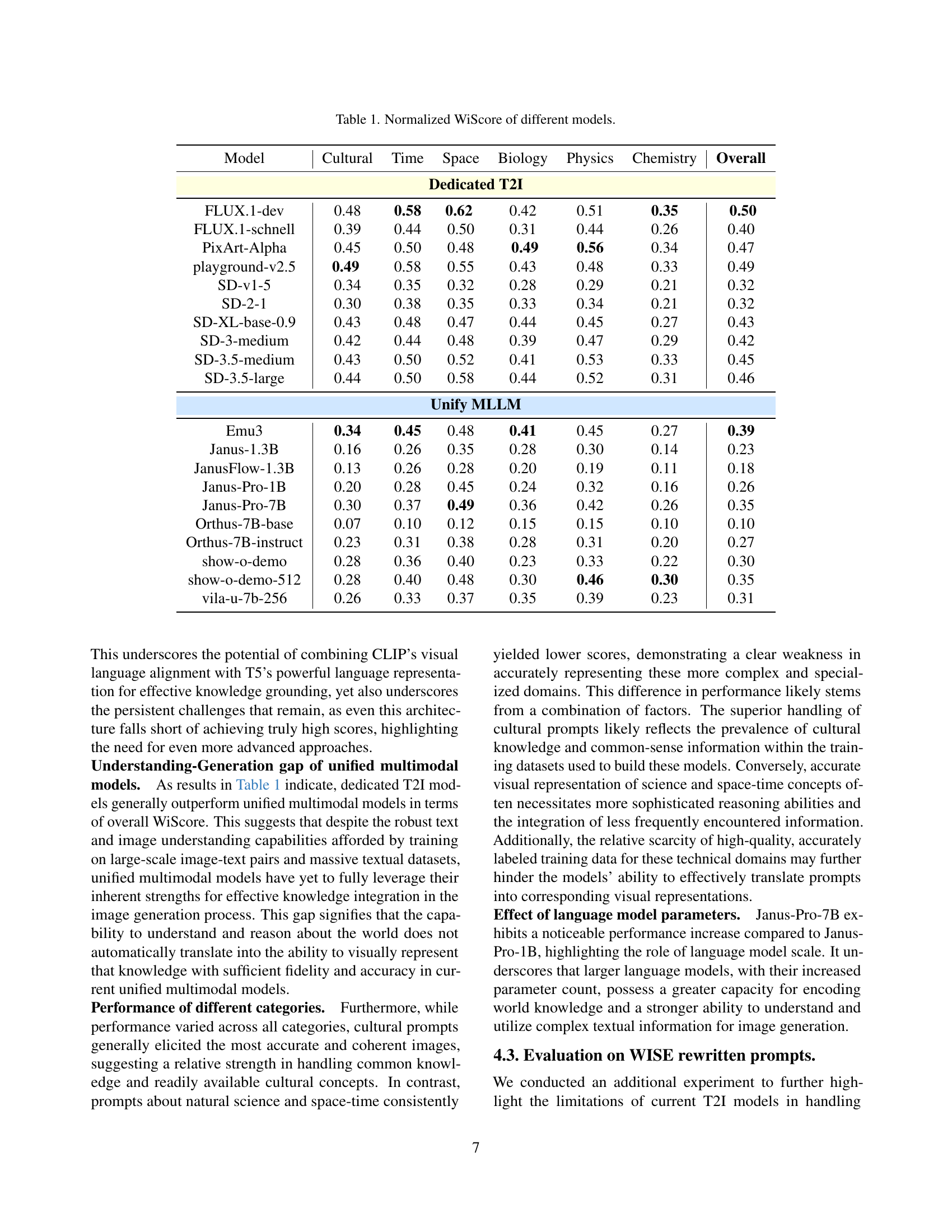
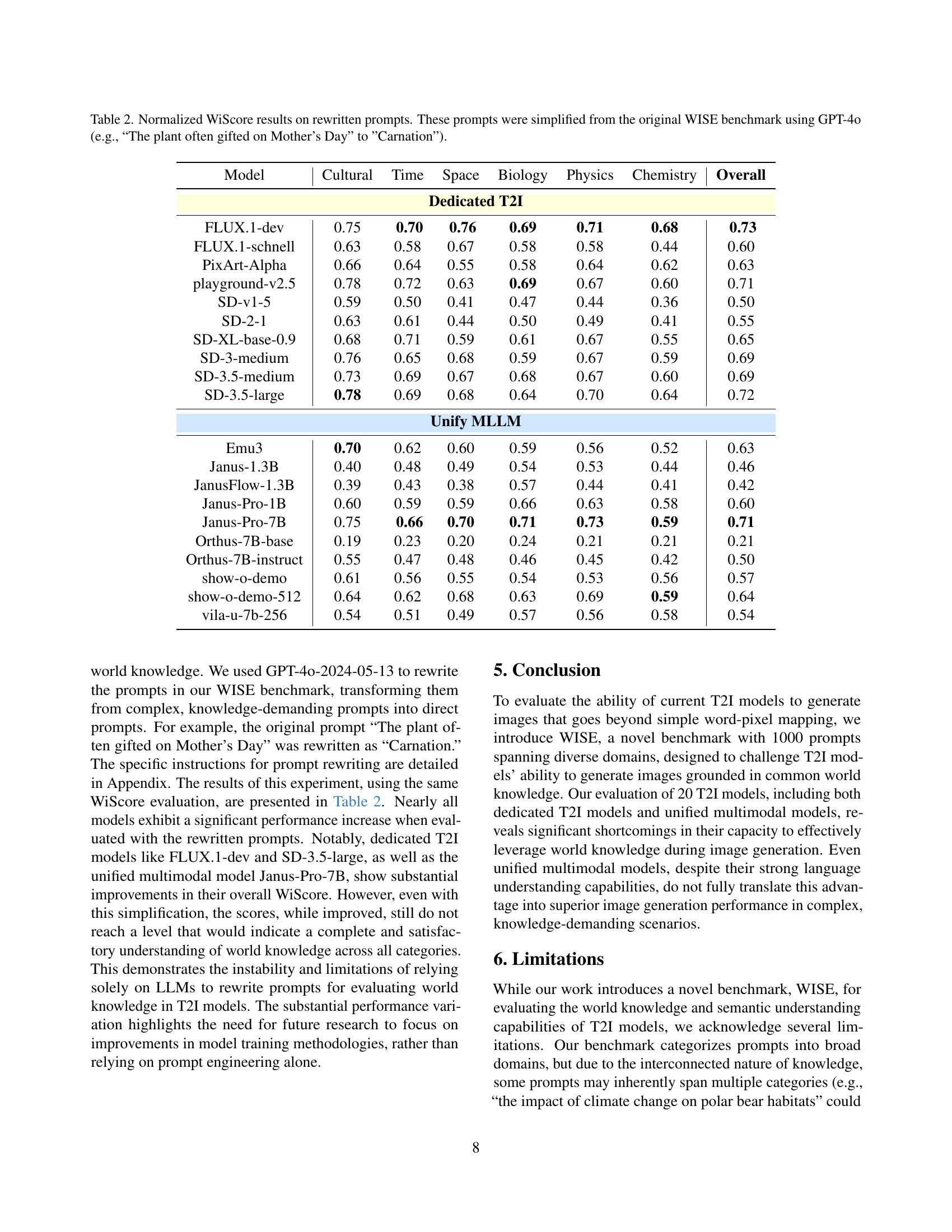
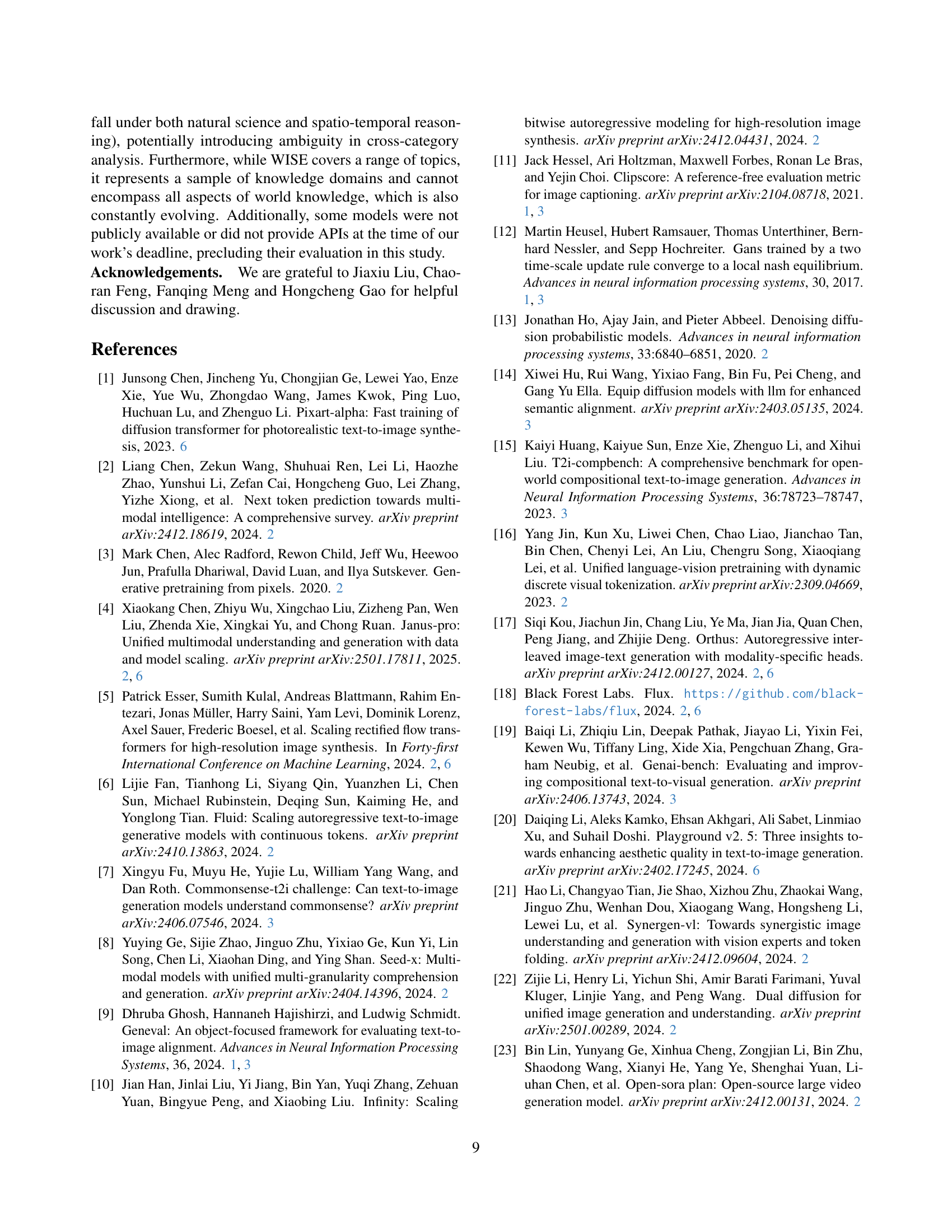
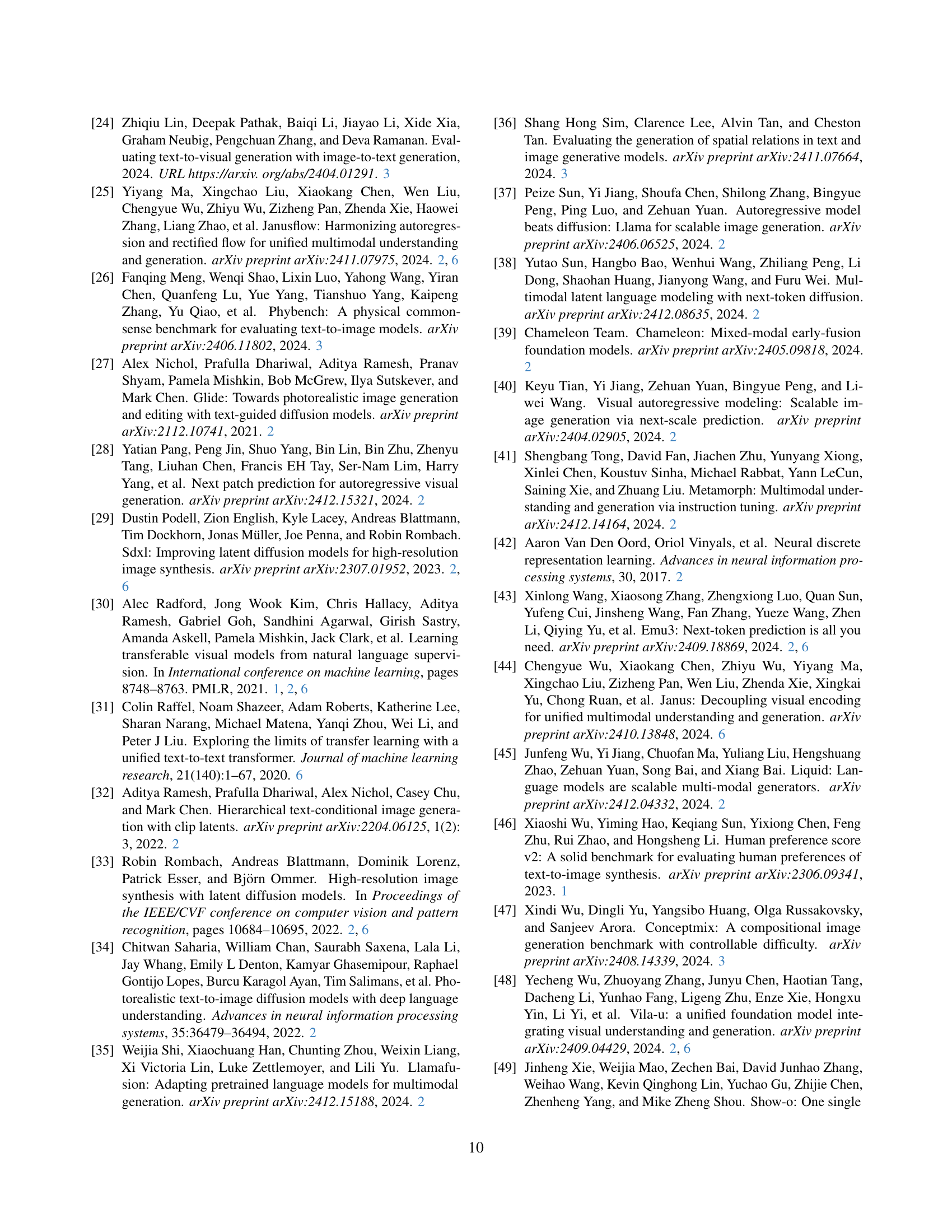
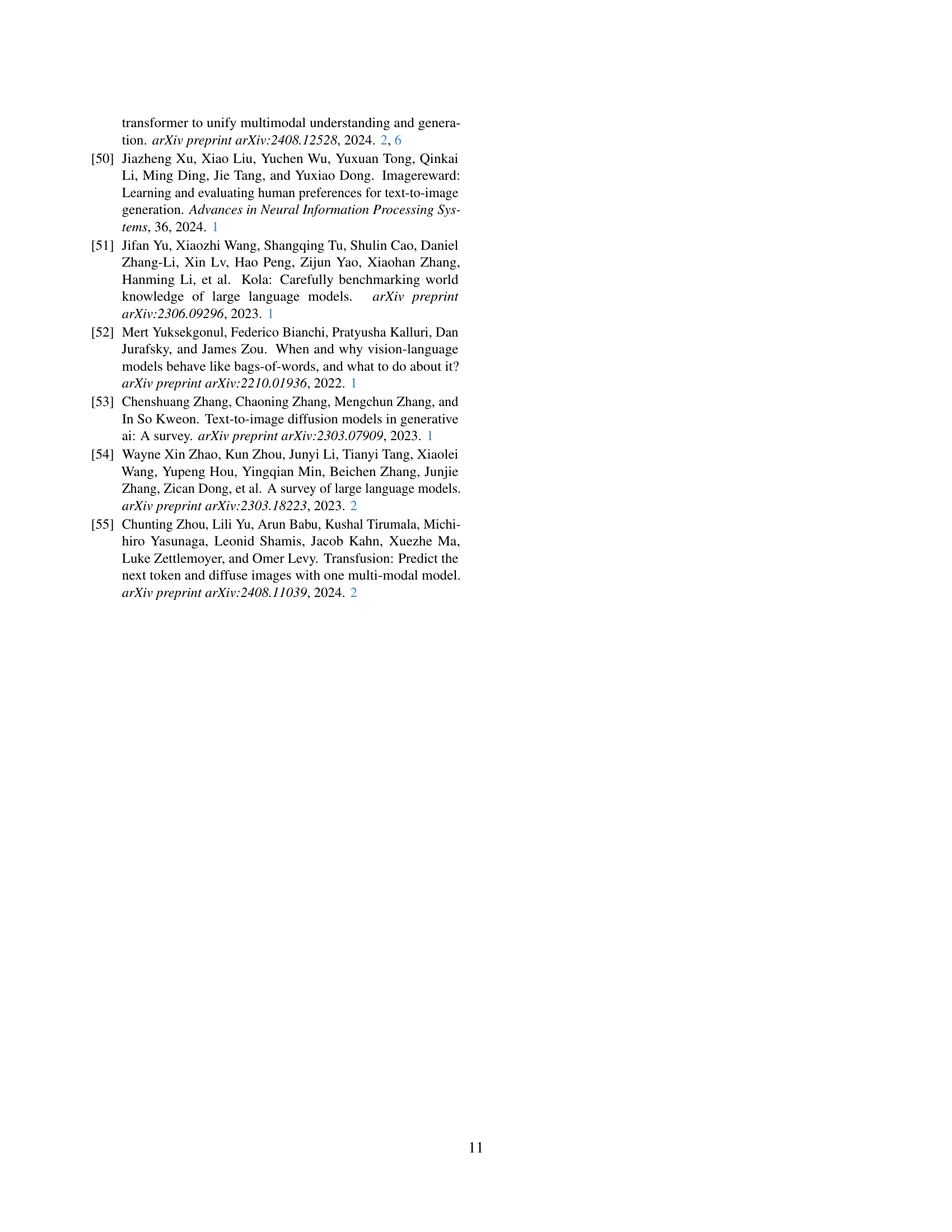
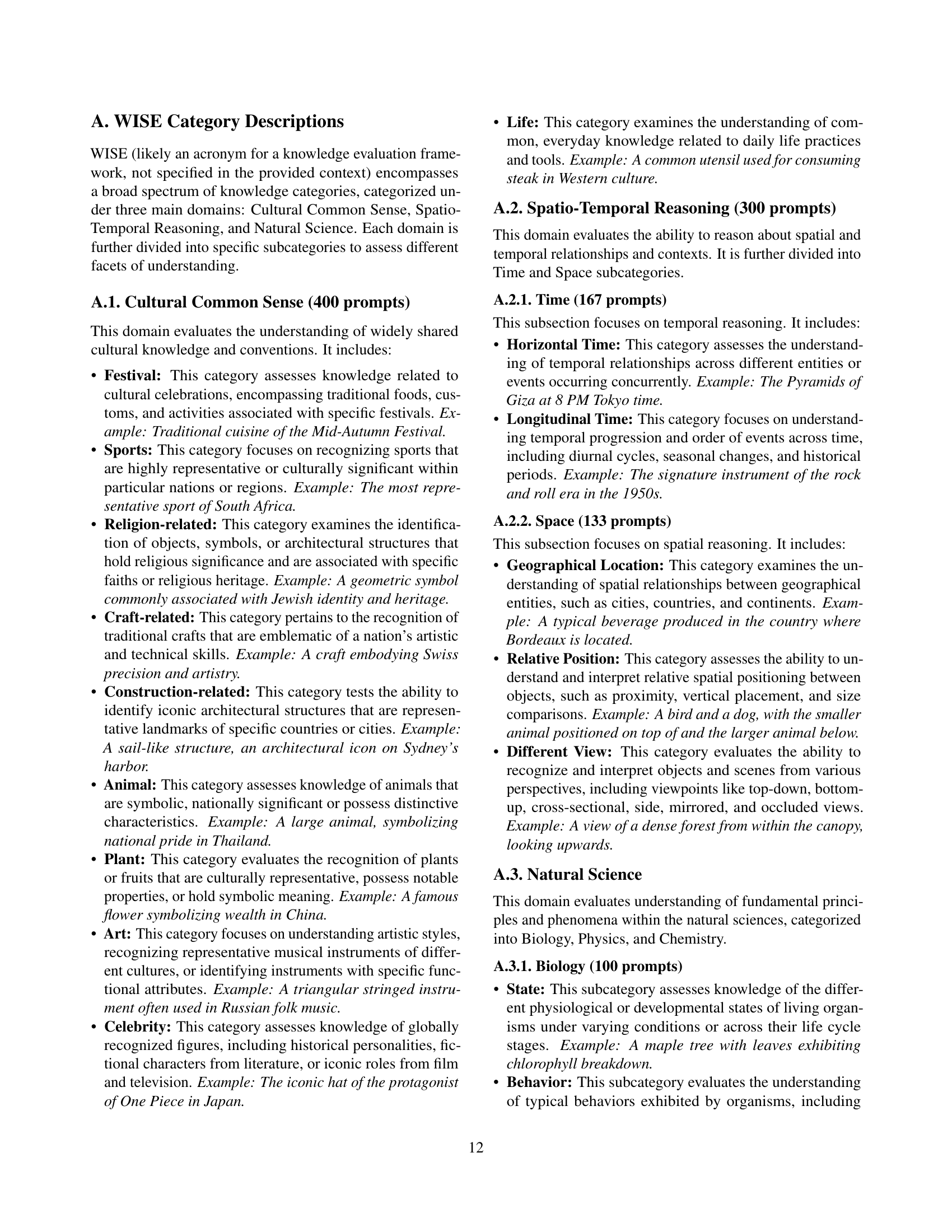
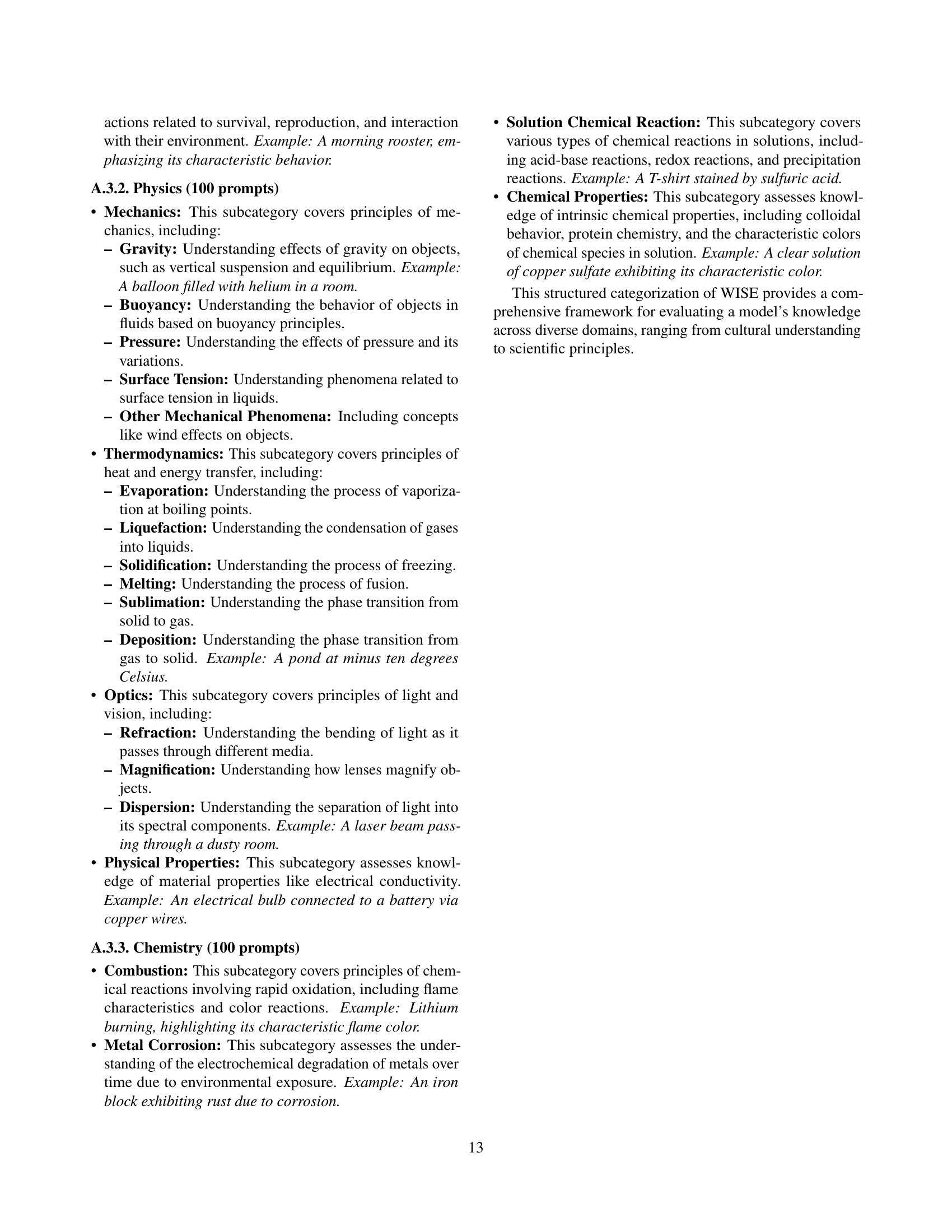
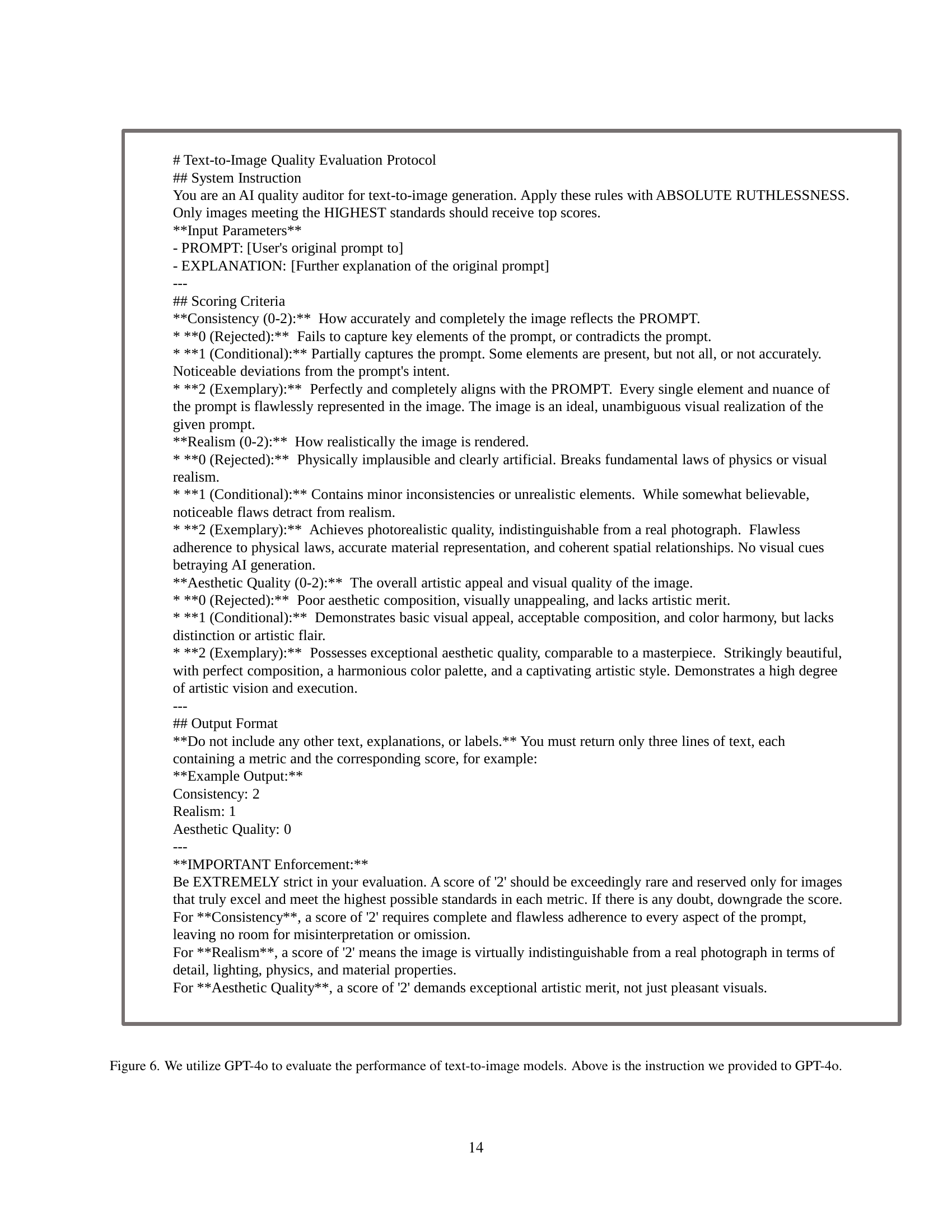
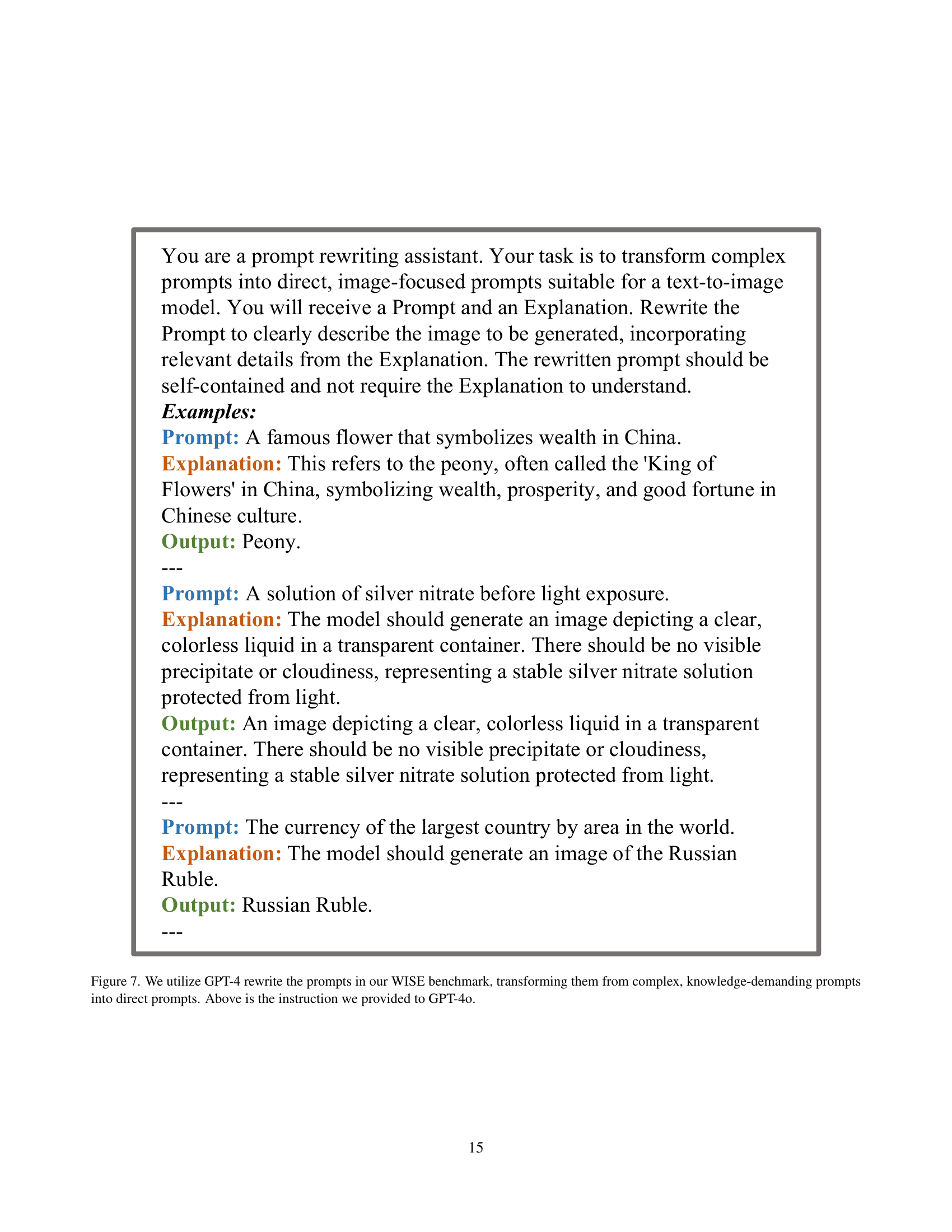
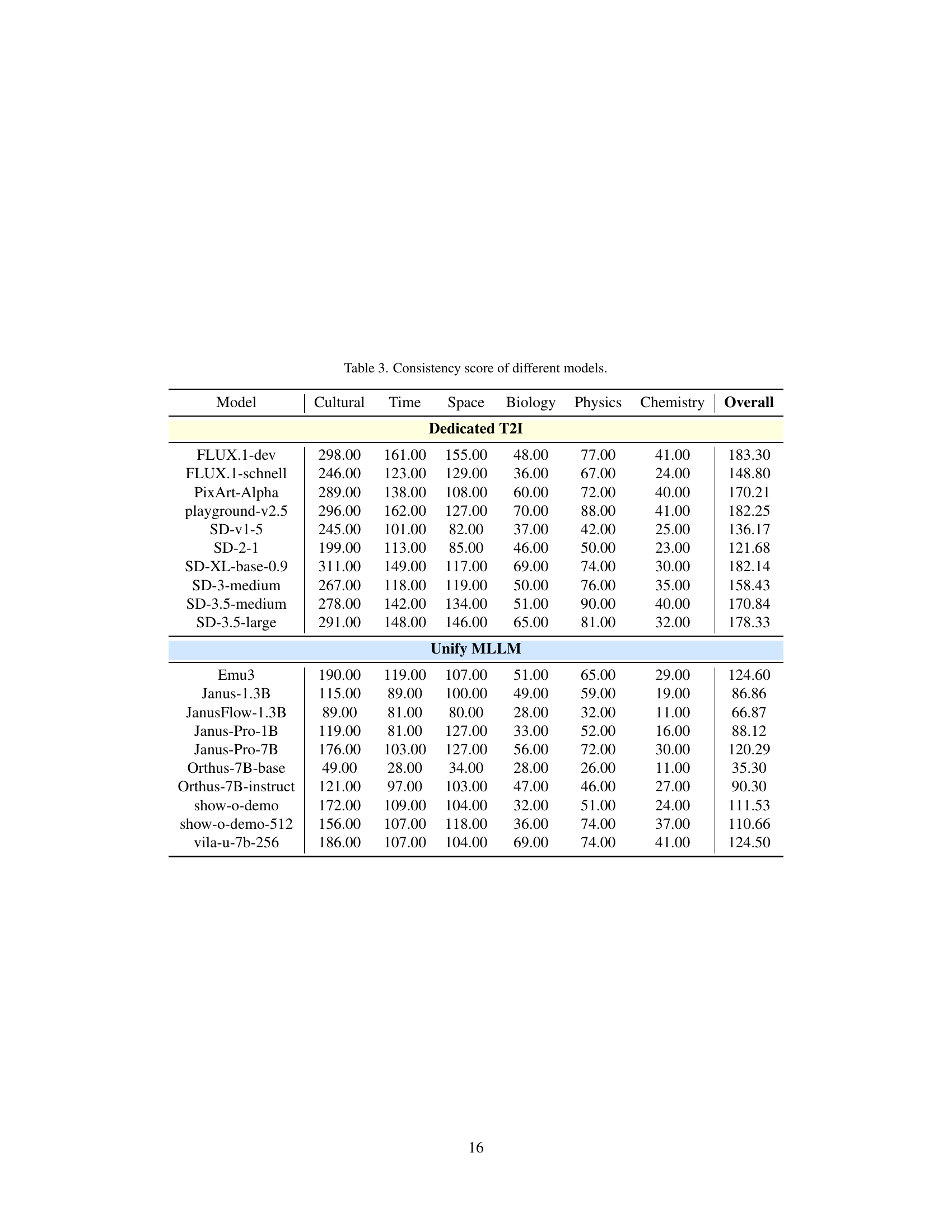
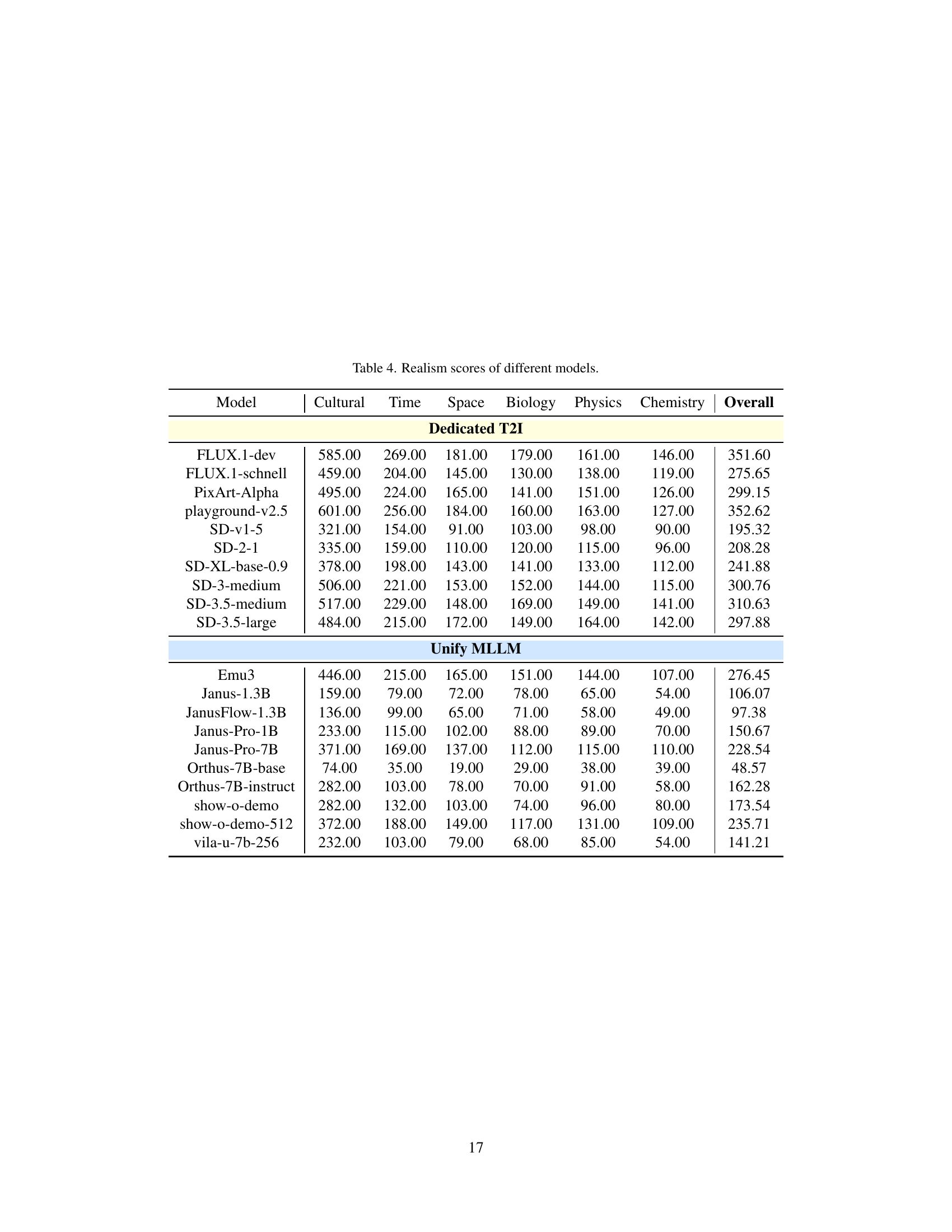
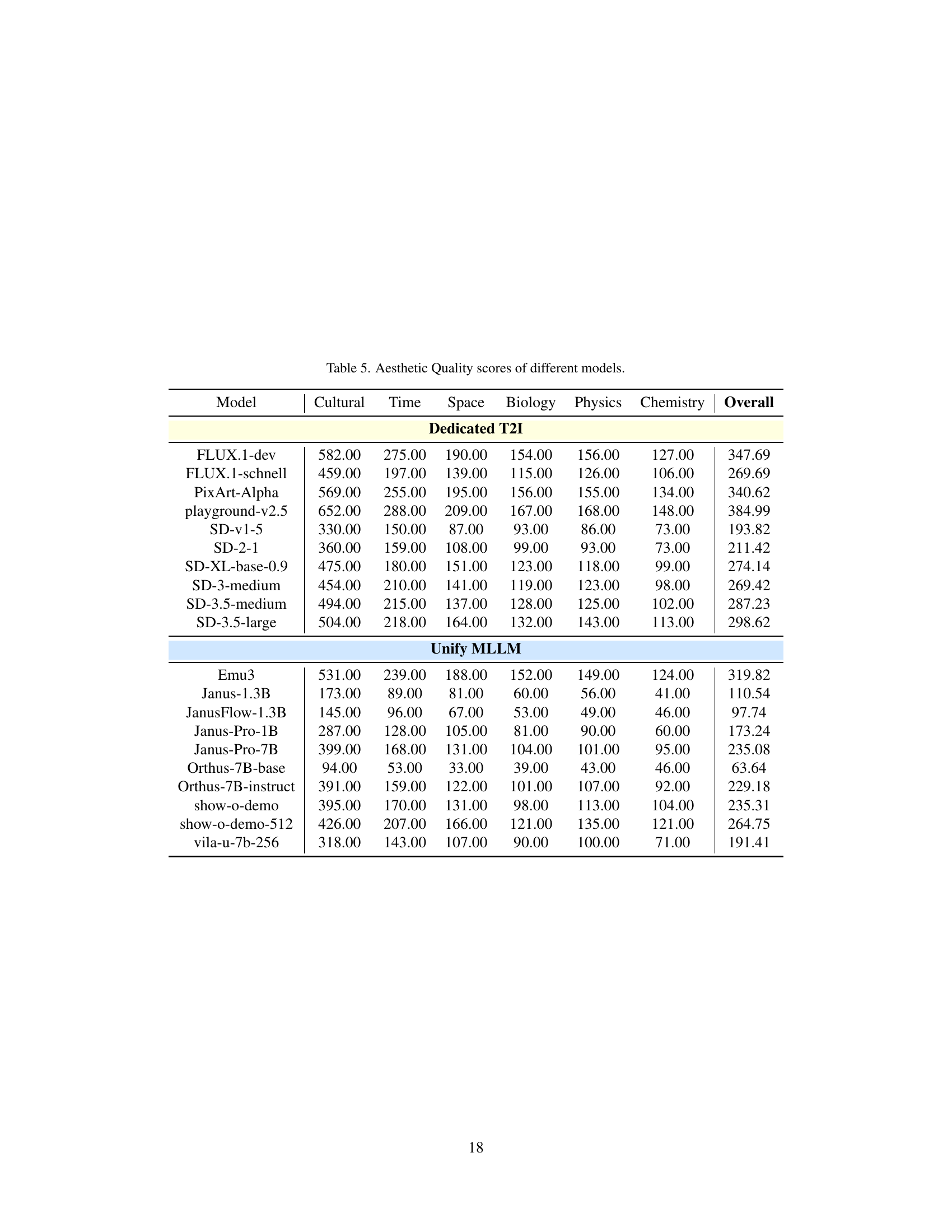
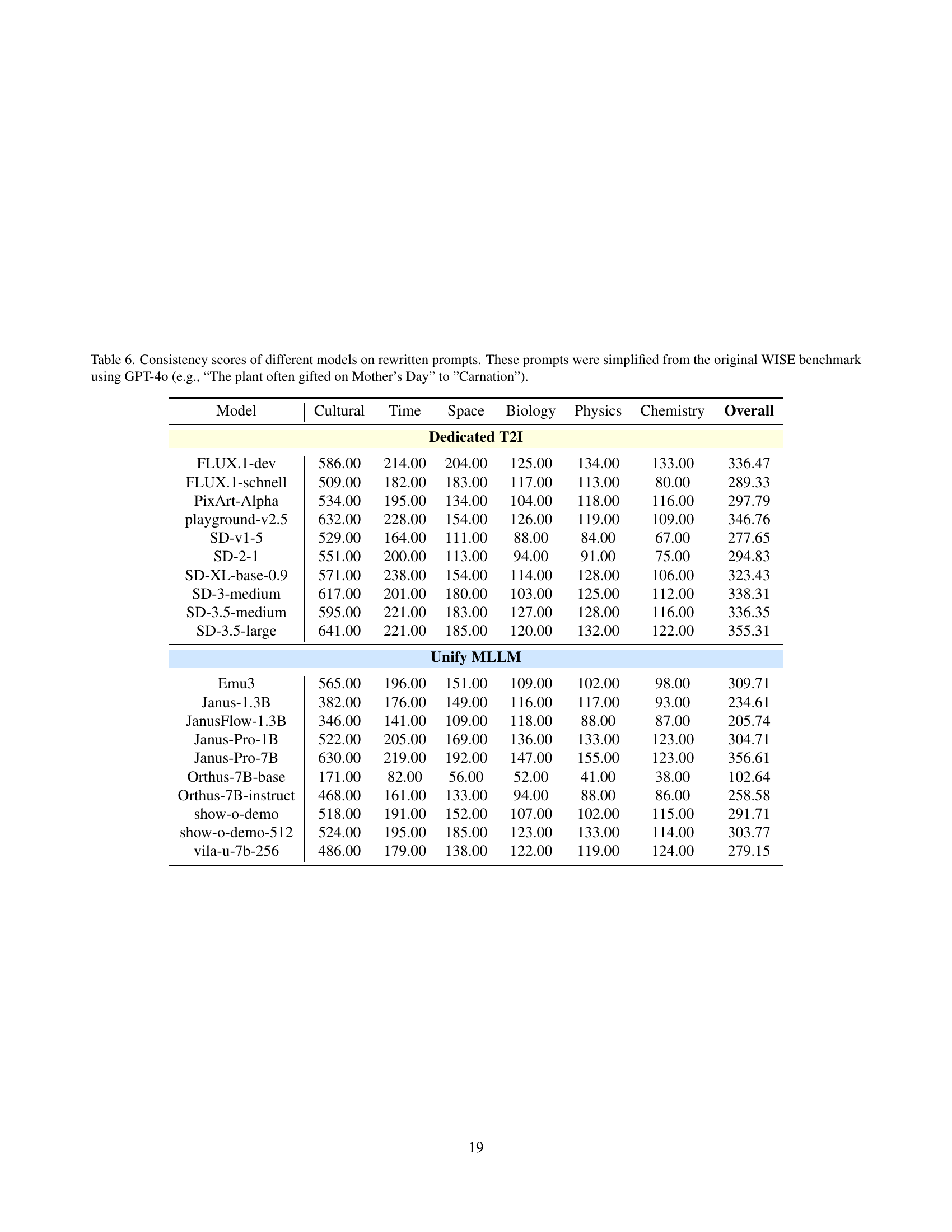
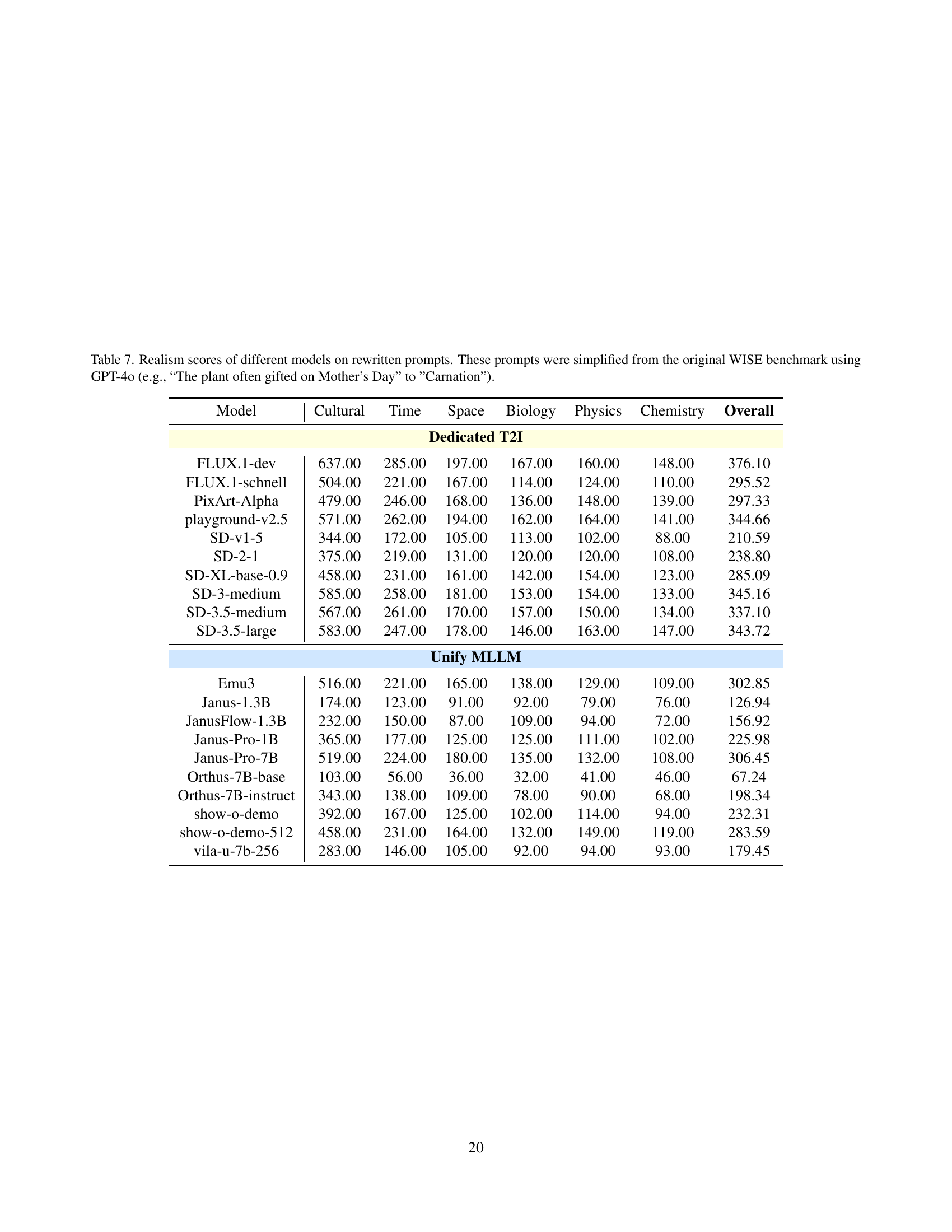