TL;DR#
Relational video customization, creating personalized videos showing specific relations between subjects, is key to understanding visual content. Current methods struggle with complex relational videos where accurate relational modeling and generalization across subjects are needed. They often focus on irrelevant details rather than meaningful interactions.
This paper proposes a new method that personalizes relations through exemplar videos. It uses relational decoupling learning to separate relations from appearances and relational dynamics enhancement to prioritize relational dynamics. Experiments show it outperforms existing methods.
Key Takeaways#
Why does it matter?#
This paper introduces a novel method for relational video customization, a crucial area for understanding complex visual content. It addresses limitations in existing methods by decoupling relations from appearances, modeling relational dynamics accurately and enhancing generalization. This work can inspire new research directions in personalized video generation and content creation.
Visual Insights#
🔼 This figure showcases the results of the DreamRelation model. Four examples are displayed, each demonstrating a different human-like interaction between two animals (hugging, punching, cheering, shaking hands). The key takeaway is that given a few example videos of a specific relationship, DreamRelation can not only generate new videos exhibiting that same relationship but also extrapolate the relationship to different animal pairs and settings, showcasing the model’s ability to customize and generalize relational interactions.
read the caption
Figure 1: Relational video customization results of DreamRelation. Given a few exemplar videos, our method can customize specific relations and generalize them to novel domains, where animals mimic human interactions.
Method |
| CLIP-T |
| FVD | ||||
---|---|---|---|---|---|---|---|---|
Mochi (base model) [69] | 0.26230.04 | 0.3237 | 0.9888 | 2047.37 | ||||
Direct LoRA finetuning | 0.32580.05 | 0.2966 | 0.9945 | 2229.08 | ||||
ReVersion [33] | 0.26900.01 | 0.3013 | 0.9921 | 2682.69 | ||||
MotionInversion [74] | 0.31510.03 | 0.3217 | 0.9855 | 2084.51 | ||||
DreamRelation | 0.44520.01 | 0.3248 | 0.9954 | 2079.87 |
🔼 This table presents a quantitative comparison of DreamRelation against several baseline methods for relational video customization. The metrics used are Relation Accuracy (assessed using a Vision-Language Model), CLIP-T (measuring text-video alignment), Temporal Consistency (evaluating video coherence), and FVD (assessing video quality). The results demonstrate DreamRelation’s superior performance in accurately generating videos that reflect the intended relations and avoid issues like appearance leakage seen in other methods.
read the caption
Table 1: Quantitative comparison results.
In-depth insights#
Relation LoRA Triplet#
The Relation LoRA Triplet seems to be a core component for customizing complex relations. It decomposes relational patterns from videos into subject appearances and relations. The method uses a composite LoRA set that includes Relation LoRAs and Subject LoRAs. Relation LoRAs capture relational information, and Subject LoRAs capture appearance information. The use of a LoRA triplet helps to disentangle the relation and the appearance, which helps the model to be more robust.
MM-DiT Analysis#
Analyzing MM-DiT, a powerful vision transformer, reveals crucial insights for video customization. Key features, like query, key, and value matrices in attention mechanisms, play distinct roles. Value features capture rich appearance and relational information, yet are intertwined. In contrast, query and key features exhibit abstract, similar patterns, differing from value features. Subspace similarity analysis confirms query and key matrices share more information, independent of the value matrix. These observations motivate the decoupling of appearance and relation, enabling targeted customization strategies. This analysis guides the design of relation LoRA triplets, enhancing the model’s ability to disentangle and generalize relations effectively.
Dynamics Enhance.#
Enhancing the temporal aspect of relational videos is a crucial step to ensure realism. This module prioritizes modeling the temporal dynamics inherent in relations while minimizing reliance on appearances, thus boosting generalization. They introduce a space-time relational contrastive loss, pulling together features from videos depicting similar relations across frames. A memory bank stores both positive and negative samples enhancing contrastive learning. The design choices here aim to capture the subtle nuances of interaction over time, beyond static appearance.
Hybrid Mask Train.#
Hybrid mask training is a technique designed to improve the decoupling of relational and appearance information within generated videos. The masks guide the model’s attention, focusing it on specific regions. This helps the model learn relationships while minimizing interference from subject appearances. The goal is to achieve better generalization and customization in relational video generation. Masks also enables LoRA training to be more efficient.
Novel Relation VLM#
While the paper doesn’t explicitly discuss a “Novel Relation VLM,” we can infer potential contributions by considering the broader context of relational understanding and Vision-Language Models (VLMs). A novel approach could involve enhancing VLMs to better capture and reason about relationships between entities in visual scenes. This might entail developing specialized architectures or training strategies that emphasize relational reasoning. Another key aspect is improving the alignment between textual descriptions of relations and their visual representations. This could involve contrastive learning techniques that encourage the model to associate similar relations with similar visual patterns, irrespective of subject appearance. Additionally, a novel VLM might incorporate explicit relational knowledge from external sources, such as knowledge graphs, to guide its understanding of complex interactions. The core is better relational modeling to enhance generalizability, and it can be achieved via relational decoupling and relation dynamics enhancement.
More visual insights#
More on figures
🔼 Figure 2 presents a comparison of video generation results between a state-of-the-art text-to-video model (Mochi) and the proposed DreamRelation model. Both models received the same prompt: ‘A bear is hugging with a tiger.’ (a) shows Mochi’s output, which demonstrates a failure to generate the unconventional interaction of a bear and tiger hugging; instead, it produced a more typical or expected interaction. (b) illustrates DreamRelation’s result, showcasing the model’s ability to generate the specific, uncommon relation requested, adapting it even to new subjects not seen during training. This highlights DreamRelation’s superior capacity for relational video customization.
read the caption
Figure 2: (a) General Video DiT models like Mochi [69] often struggle to generate unconventional or counter-intuitive interactions, even with detailed descriptions. (b) Our method can customize a specific relation to generate videos on new subjects.
🔼 The figure visualizes the average of value feature maps across all layers and frames of the Mochi model. The value feature is a key component in the attention mechanism, representing information about the objects and their relationships. By averaging these features, the figure highlights the consistent patterns that the Mochi model detects during relation processing. The analysis reveals that relations involve complex interactions in terms of spatial arrangement, positional layout changes, and intricate temporal dynamics. These combined factors present significant challenges when developing methods for relational video customization.
read the caption
Figure 3: Averaged value feature across all layers and frames in Mochi. We identify that the relations encompass intricate spatial arrangements, layout variations, and nuanced temporal dynamics, presenting challenges in relational video customization.
🔼 DreamRelation processes relational video customization in two stages: Relational Decoupling Learning and Relational Dynamics Enhancement. The first stage uses Relation LoRAs and Subject LoRAs within a ‘relation LoRA triplet’ to separate relational information from subject appearances. A hybrid mask training strategy ensures the LoRAs focus on the correct aspects. The second stage uses a space-time relational contrastive loss. This loss function brings together relational dynamics features from different frames of similar videos and pushes away unrelated appearance features from single frames. During inference, Subject LoRAs are omitted to improve generalization and reduce unwanted appearance influence.
read the caption
Figure 4: Overall framework of DreamRelation. Our method decomposes relational video customization into two concurrent processes. (1) In Relational Decoupling Learning, Relation LoRAs in relation LoRA triplet capture relational information, while Subject LoRAs focus on subject appearances. This decoupling process is guided by hybrid mask training strategy based on their corresponding masks. (2) In Relational Dynamics Enhancement, the proposed space-time relational contrastive loss pulls relational dynamics features (anchor and positive features) from pairwise differences closer, while pushing them away from appearance features (negative features) of single-frame outputs. During inference, subject LoRAs are excluded to prevent introducing undesired appearances and enhance generalization.
🔼 Figure 5 analyzes the query, key, and value features within the Multimodal Diffusion Transformer (MM-DiT) model’s attention mechanism. Part (a) visualizes these features across various video examples, demonstrating that value features capture rich appearance information, often intertwined with relational information, while query and key features display similar patterns distinct from value features. Part (b) quantifies this observation by performing singular value decomposition on the query, key, and value matrices of each MM-DiT block. It then calculates the subspace similarity between these matrices, revealing that query and key matrices share substantial common information, yet remain independent of the value matrix.
read the caption
Figure 5: Features and subspace similarity analysis of MM-DiT. (a) Value features across different videos encapsulate rich appearance information, and relational information often intertwines with these appearance cues. Meanwhile, query and key features exhibit similar patterns that differ from those of value features. (b) We perform singular value decomposition on the query, key, and value matrices of each MM-DiT block and compute the similarity of the subspaces spanned by their top-k left singular vectors, indicating query and key matrices share more common information while remaining independent of the value matrix.
🔼 Figure 6 presents a qualitative comparison of DreamRelation against several baseline methods for relational video customization. The figure showcases example videos generated by each method for two different relations: ‘shaking hands’ and ‘high-fiving’. By comparing the results, we observe that DreamRelation excels at accurately representing the intended relationship between the subjects, while effectively reducing unwanted appearance artifacts and background distractions present in the videos generated by other methods. This visual comparison highlights DreamRelation’s superiority in capturing the nuanced details of the specified relations, showcasing the effectiveness of its relational decoupling and dynamics enhancement strategies.
read the caption
Figure 6: Qualitative comparison results. Our method outperforms all baselines in precisely capturing the intended relation and mitigating appearance and background leakage.
🔼 Figure 7 presents a two-part analysis of the DreamRelation model’s performance. (a) shows attention maps, visualizations that highlight where the model focuses its attention. The visualization demonstrates that DreamRelation concentrates its attention on the relevant areas depicting the specified relationship between subjects, rather than being distracted by irrelevant details or background elements. (b) shows the results of a user study comparing DreamRelation to other methods. The bar chart indicates that users overwhelmingly favored DreamRelation across all evaluation criteria (Relation Alignment, Text Alignment, and Overall Quality). This shows that not only does the model focus on the correct areas but also generates videos of superior quality and alignment with user expectations.
read the caption
Figure 7: (a) Our method focuses on the desired relational region. (b) Our method is most preferred by users across all aspects.
🔼 This ablation study visually demonstrates the individual contributions of each component of the DreamRelation model. It shows the results of removing, one at a time, the hybrid mask training strategy (HMT), the space-time relational contrastive loss (RCL), the Relation LoRAs, the Subject LoRAs, and the FFN LoRAs. By comparing these results to the complete model, the impact of each component on the model’s performance can be assessed. Each row shows the performance of a modified model on several evaluation metrics.
read the caption
Figure 8: Qualitative ablation study on each component.
🔼 This figure displays several example results from the DreamRelation model, showcasing its ability to generate videos depicting various relational interactions between animals. Each row presents a specific relation (e.g., cheering, high-five, hugging, etc.) along with corresponding example videos generated by the model. The videos show different animals performing actions that represent human-like interactions, highlighting the model’s capacity to generalize and apply relations across novel domains and animal species. The caption encourages viewers to zoom in for better detail visualization.
read the caption
Figure 9: More qualitative results of DreamRelation (1/2). Please zoom in for a better view.
More on tables
Relation |
Accuracy |
🔼 This table presents the results of ablation experiments conducted on the DreamRelation model. It shows the impact of removing key components: the hybrid mask training strategy (HMT), the space-time relational contrastive loss (RCL), and each type of LoRA (Relation LoRA, Subject LoRAs, FFN LoRA). The results demonstrate that each of these components is crucial for the model’s overall performance, and removing any one significantly degrades its ability to accurately generate relational videos. The metrics used likely include measures of relation accuracy, visual-textual alignment, and video quality.
read the caption
Table 2: Ablation studies on effects of hybrid mask training strategy (HMT), space-time relational contrastive loss (RCL), and each type of LoRA. Removing any of the above components significantly reduces the overall performance.
Temporal |
Consistency |
🔼 This table presents an ablation study analyzing the impact of placing Relation LoRAs (Low-Rank Adaptation) in different positions within the MM-DiT (Multi-Modal Diffusion Transformer) architecture. The study investigates how placing the Relation LoRAs in the query (Q), key (K), and value (V) matrices of the attention mechanism, as well as their combination affects the model’s performance on relational video customization. The performance is measured using Relation Accuracy, CLIP-T (CLIP text similarity), Temporal Consistency, and FVD (Fréchet Video Distance), providing a comprehensive assessment of the different configurations.
read the caption
Table 3: Ablation study of Relation LoRA position.
Method |
| CLIP-T |
| FVD | ||||
---|---|---|---|---|---|---|---|---|
w/o HMT | 0.35740.02 | 0.3244 | 0.9938 | 2248.52 | ||||
w/o RCL | 0.34160.03 | 0.3185 | 0.9953 | 2136.95 | ||||
w/o Relation LoRAs | 0.36260.02 | 0.3035 | 0.9950 | 2318.49 | ||||
w/o Subject LoRAs | 0.37690.04 | 0.3147 | 0.9949 | 2408.59 | ||||
w/o FFN LoRAs | 0.40210.03 | 0.3241 | 0.9914 | 2369.98 | ||||
ours | 0.44520.01 | 0.3248 | 0.9954 | 2079.87 |
🔼 This table presents the ablation study results of adding the space-time relational contrastive loss (RCL) to the MotionInversion method. It shows the impact of incorporating RCL on the relation accuracy, CLIP-T (text alignment), temporal consistency, and FVD (video quality) metrics. The goal is to demonstrate how RCL enhances relational dynamics learning, improving the overall performance of the motion customization method in terms of precise relation modeling and video quality.
read the caption
Table 4: Effects of space-time relational contrastive loss on motion customization method (MotionInversion).
Relation |
Accuracy |
🔼 This table presents the results of an ablation study investigating the effect of different values for the loss weight (λ1) in the space-time relational contrastive loss (RCL) component of the DreamRelation model. The study varied λ1 and measured the impact on four key metrics: Relation Accuracy, CLIP-T (text alignment score), Temporal Consistency, and Fréchet Video Distance (FVD). The goal was to find the optimal balance between contrastive learning (which emphasizes relational dynamics) and reconstruction loss, to achieve the best overall performance on relational video generation.
read the caption
Table 5: Ablation study of the loss weight λ1subscript𝜆1\lambda_{1}italic_λ start_POSTSUBSCRIPT 1 end_POSTSUBSCRIPT.
Temporal |
Consistency |
🔼 This ablation study investigates the impact of varying the mask weight (λm) during training on the performance of the DreamRelation model. The table shows how changes in λm affect the relation accuracy, CLIP-T score, temporal consistency, and Fréchet video distance (FVD) which are metrics to evaluate the generated videos. Different λm values were tested to determine the optimal weight that balances the focus on the designated regions while avoiding excessive emphasis on specific areas.
read the caption
Table 6: Ablation study of the mask weight λmsubscript𝜆𝑚\lambda_{m}italic_λ start_POSTSUBSCRIPT italic_m end_POSTSUBSCRIPT.
|
|
| CLIP-T |
| FVD | ||||||||
---|---|---|---|---|---|---|---|---|---|---|---|---|---|
V | Q, K | 0.34440.02 | 0.3225 | 0.9953 | 2233.48 | ||||||||
Q | K, V | 0.39210.03 | 0.3301 | 0.9951 | 2284.65 | ||||||||
K, V | Q | 0.39370.04 | 0.3196 | 0.9954 | 2180.27 | ||||||||
Q, K | V | 0.44520.01 | 0.3248 | 0.9954 | 2079.87 |
🔼 This ablation study investigates the impact of varying the number of positive and negative samples used in the space-time relational contrastive loss. The goal is to understand how changing the balance of positive (similar relational dynamics) and negative (dissimilar, or appearance-based) samples affects the model’s performance on relational video generation. The table shows the results of experiments with different combinations of positive and negative samples, evaluating relation accuracy, CLIP-T score (text-image alignment), temporal consistency, and Fréchet Video Distance (FVD, video quality).
read the caption
Table 7: Ablation study of the number of positive and negative samples.
Relation |
LoRA |
🔼 This table lists 26 different types of human interactions used in the paper’s experiments. For each interaction, a textual prompt is provided that describes the action. These prompts serve as input to the model, which then generates videos depicting those interactions.
read the caption
Table 8: The list of 26 human interactions with their textual prompts.
Full paper#
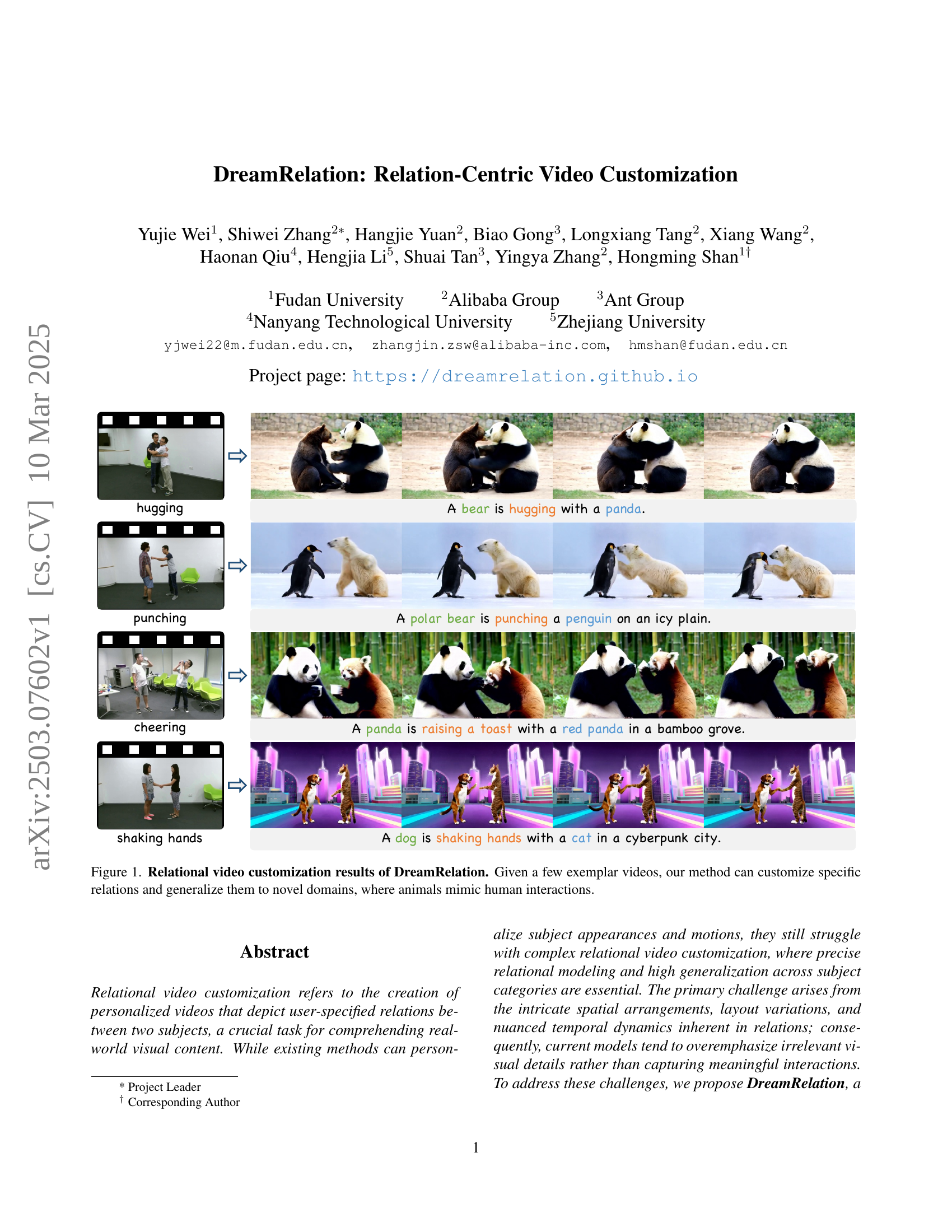
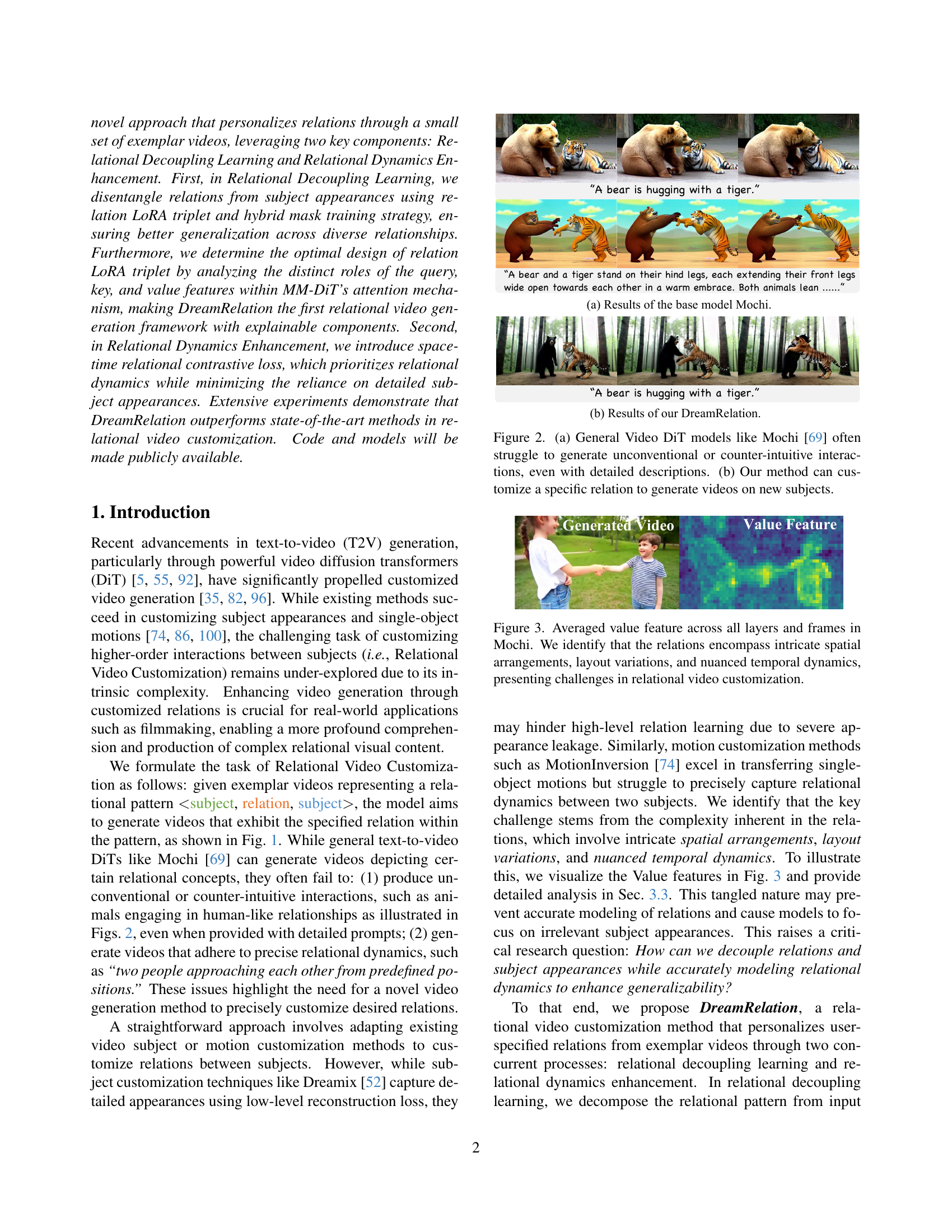
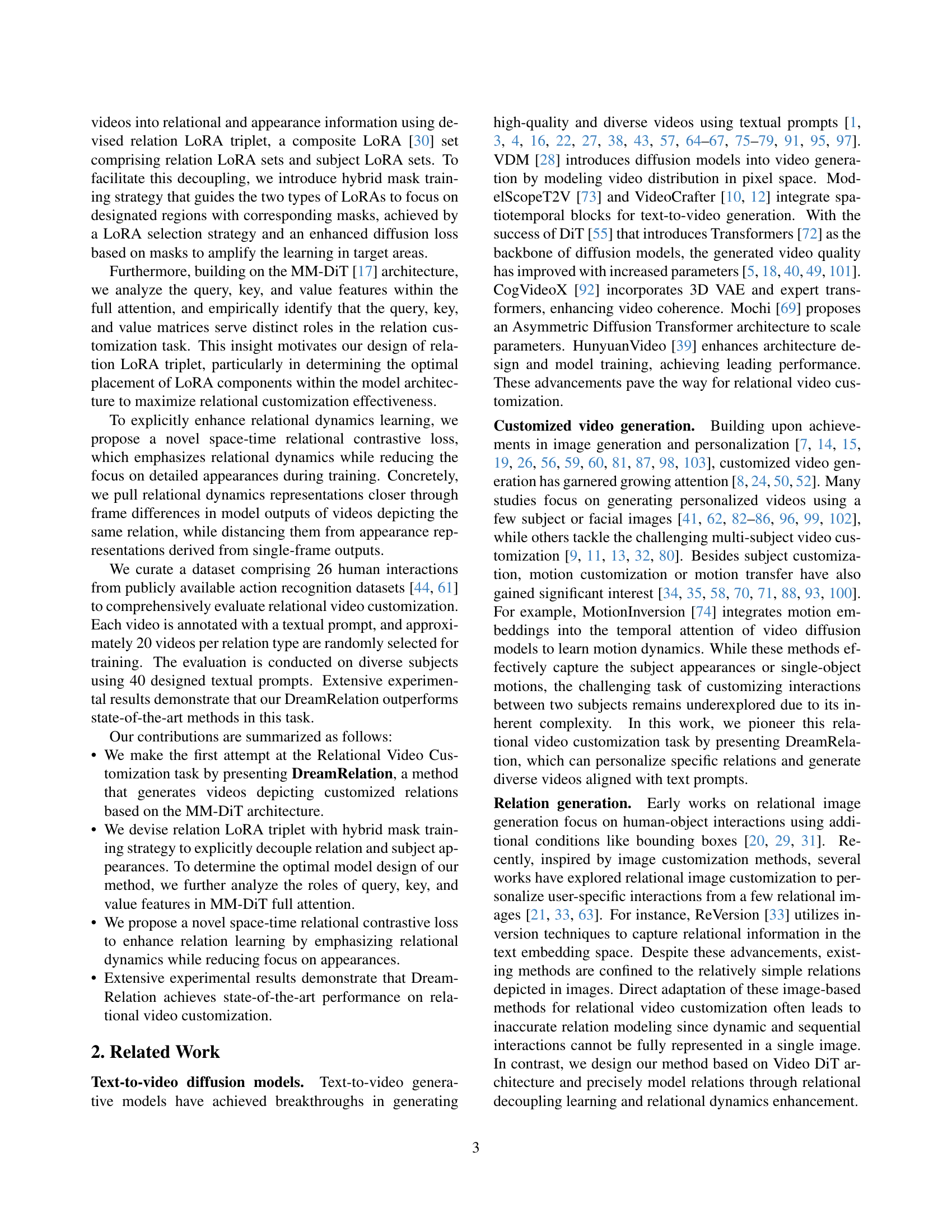
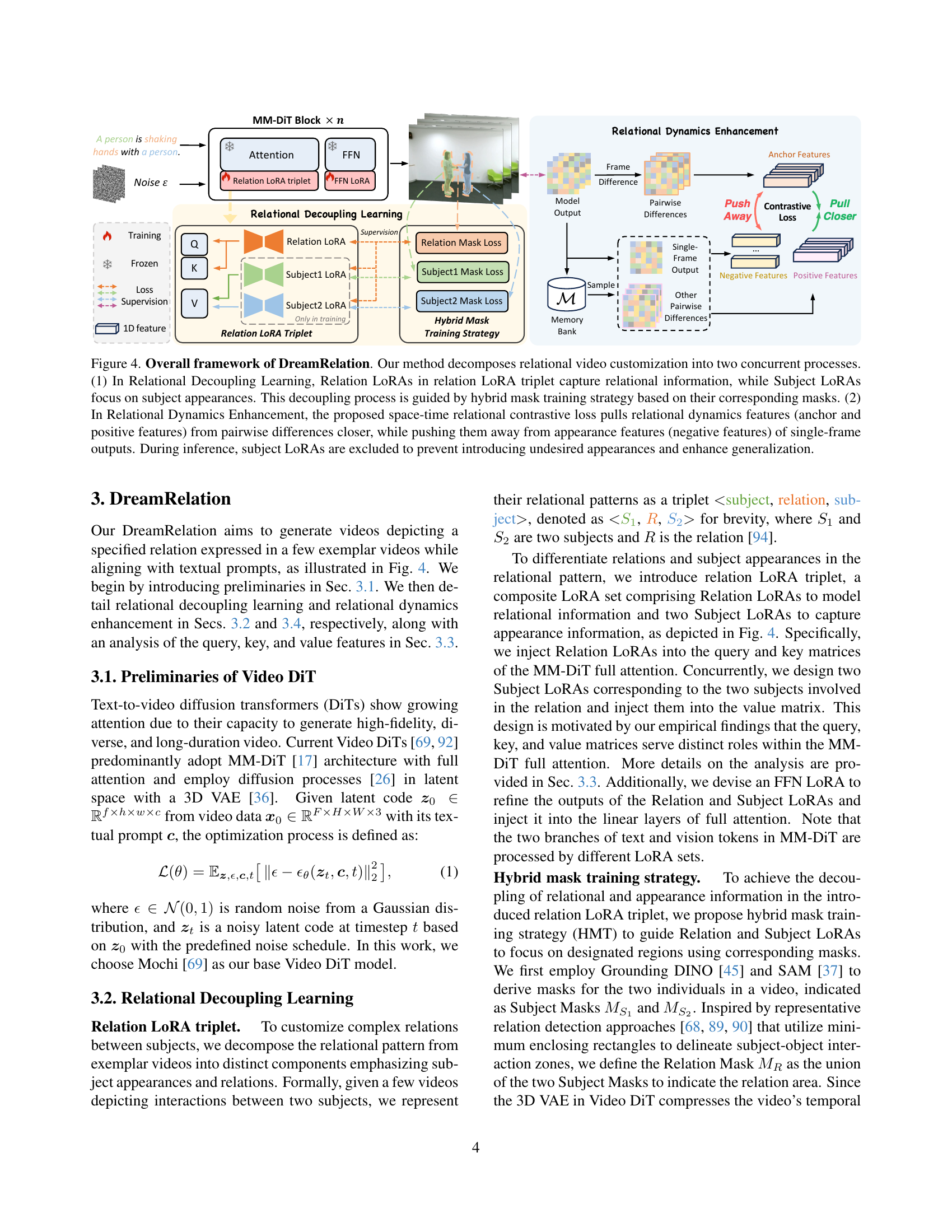
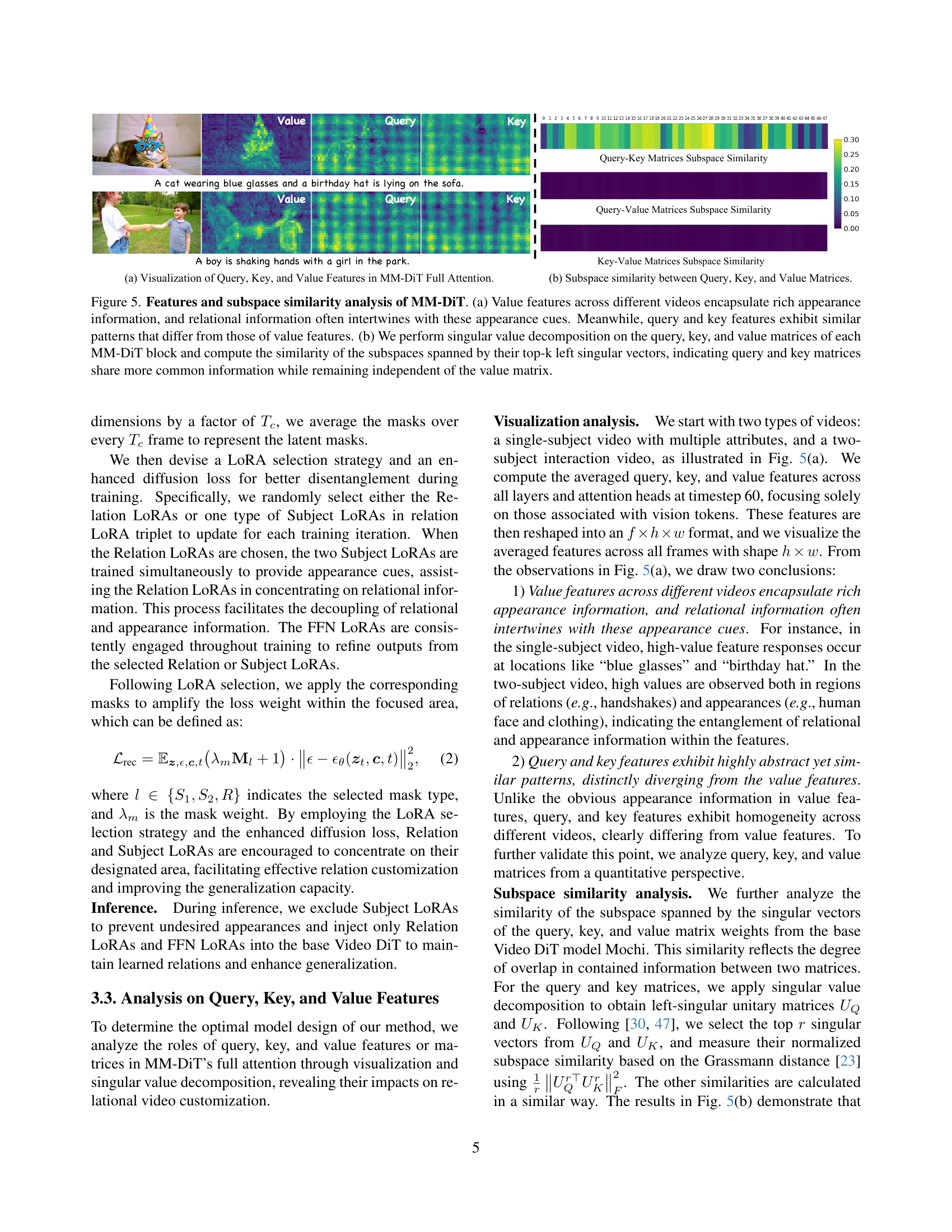
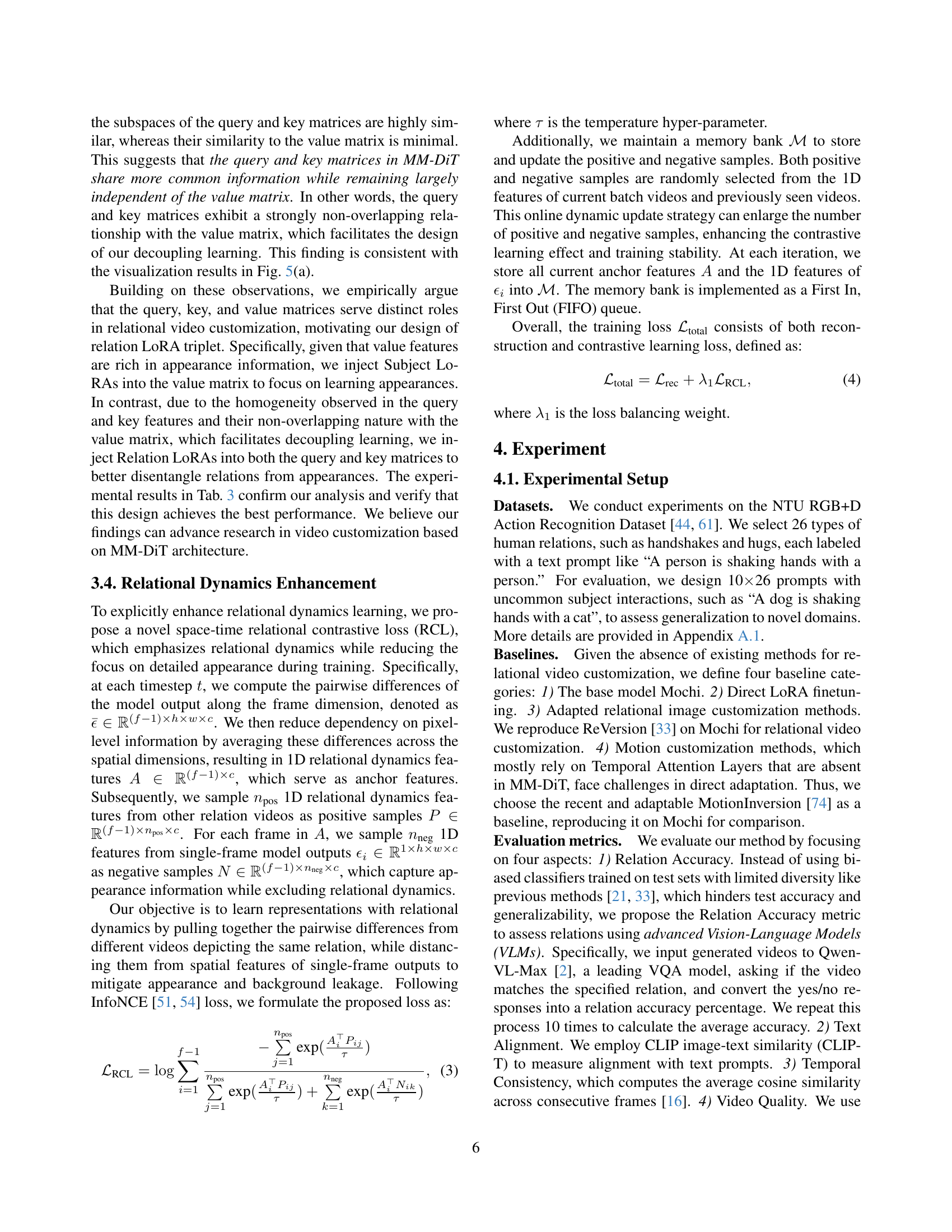
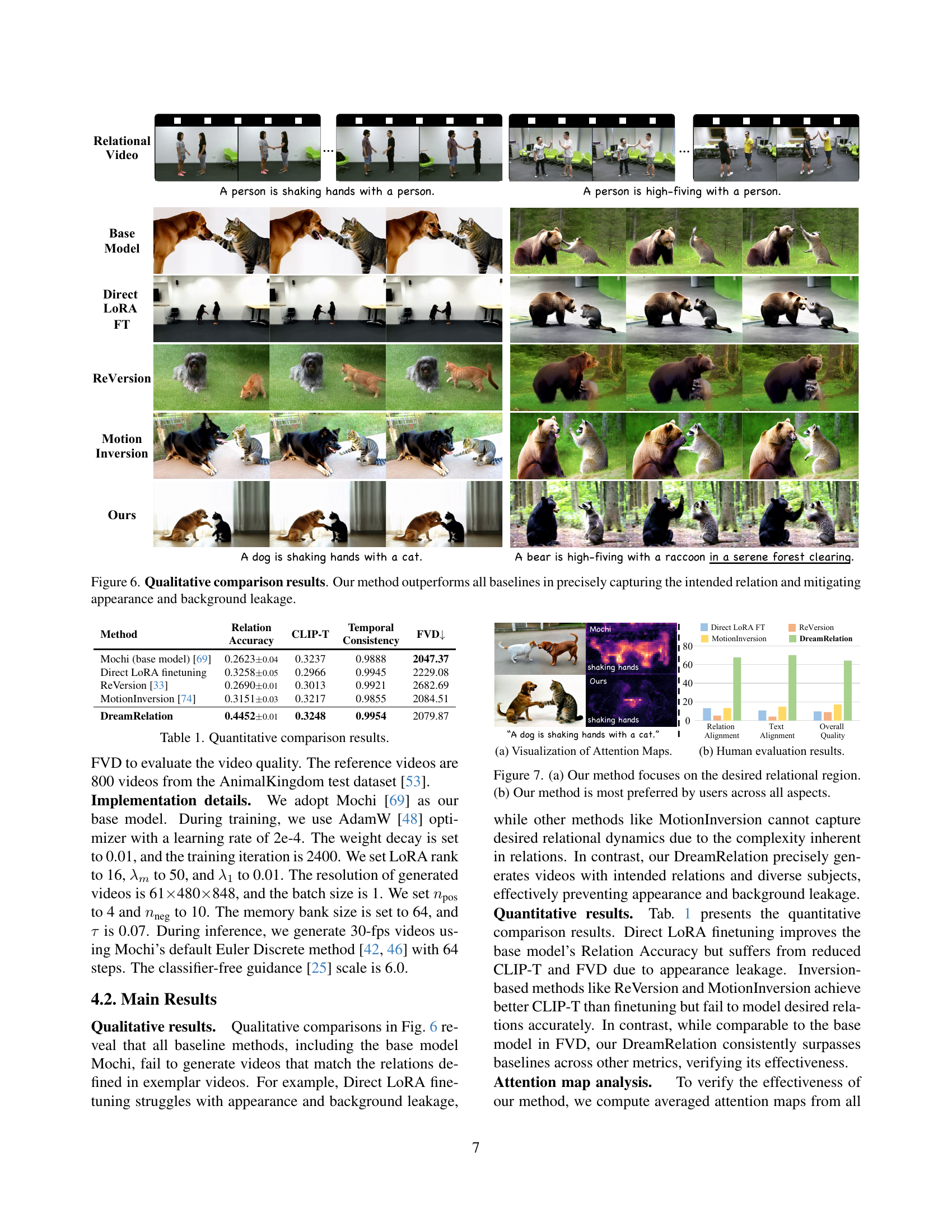
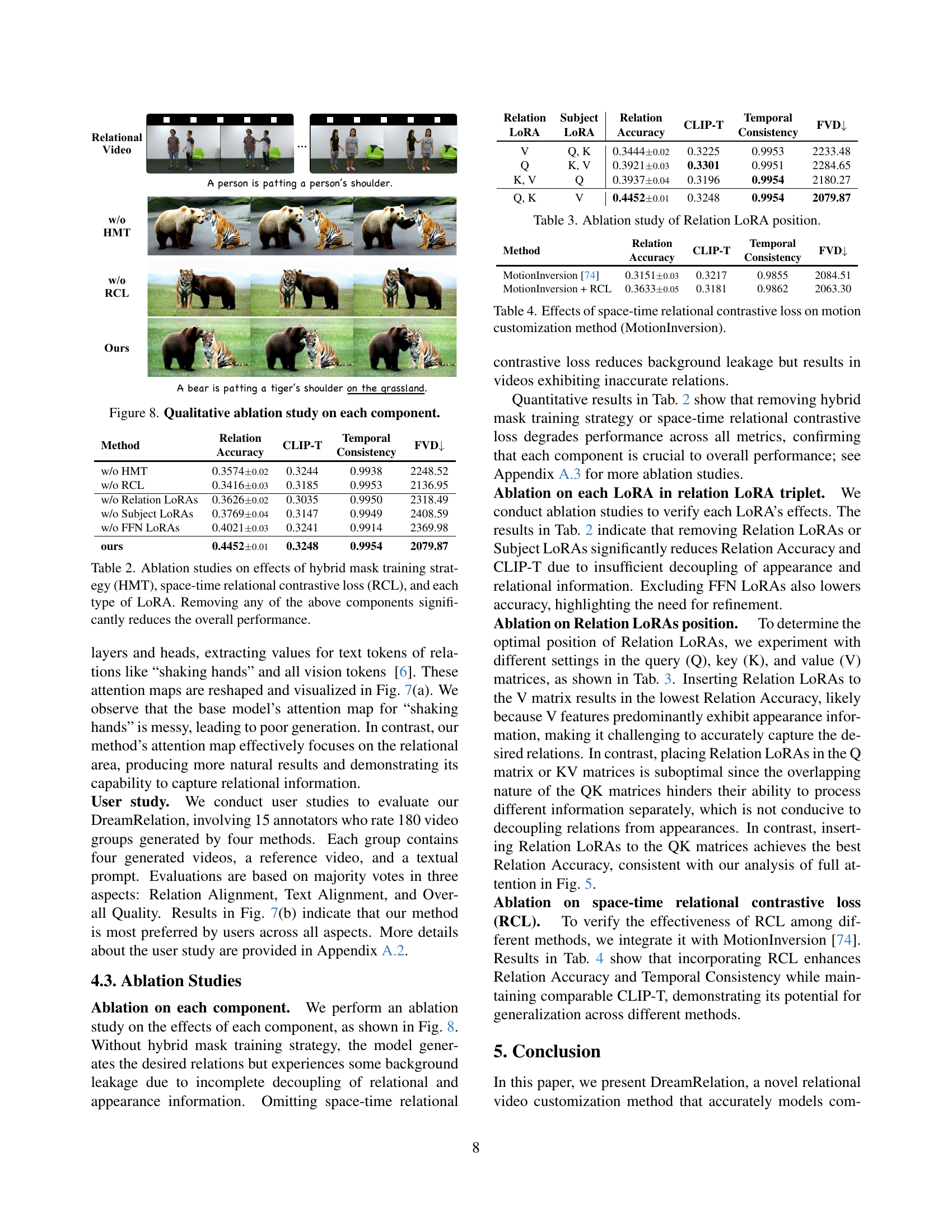
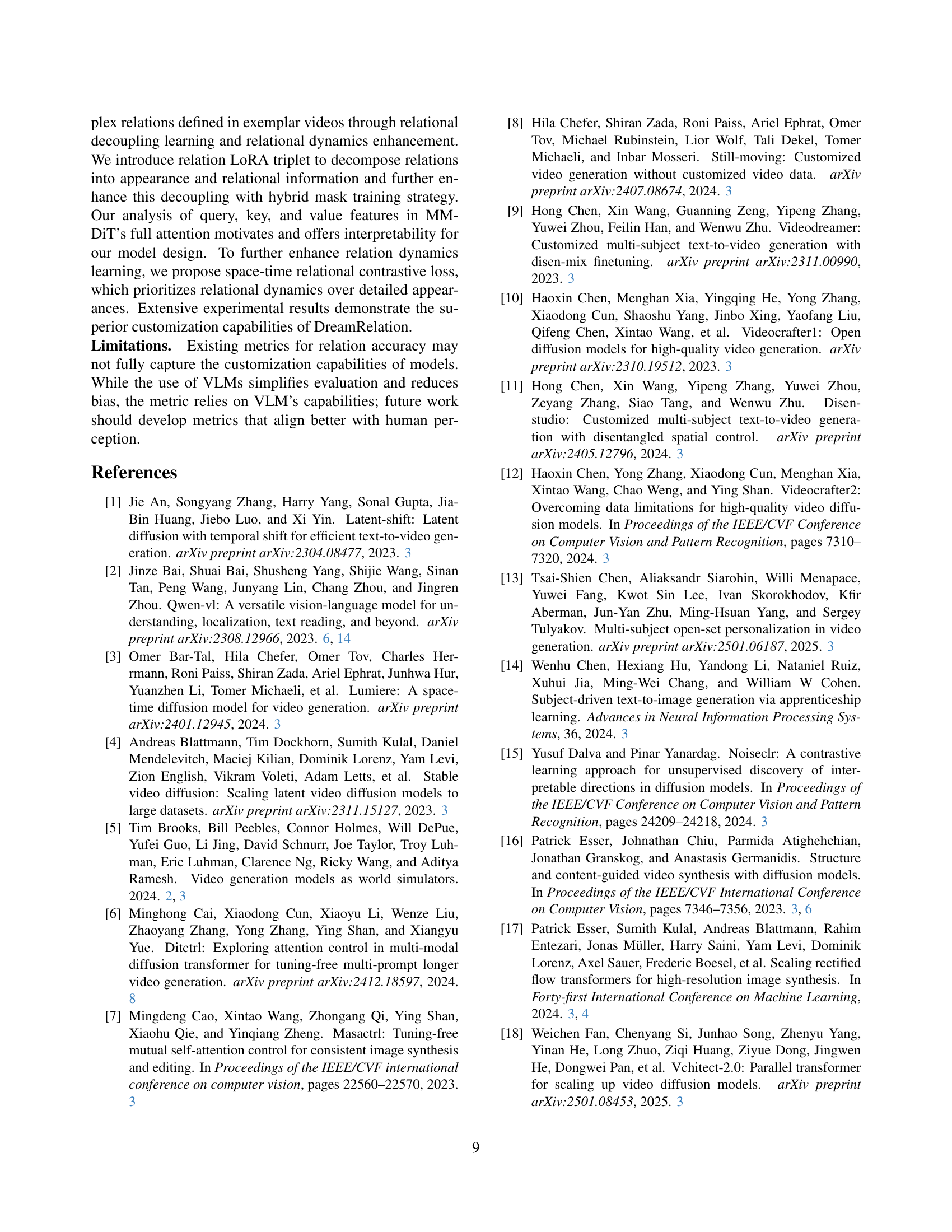
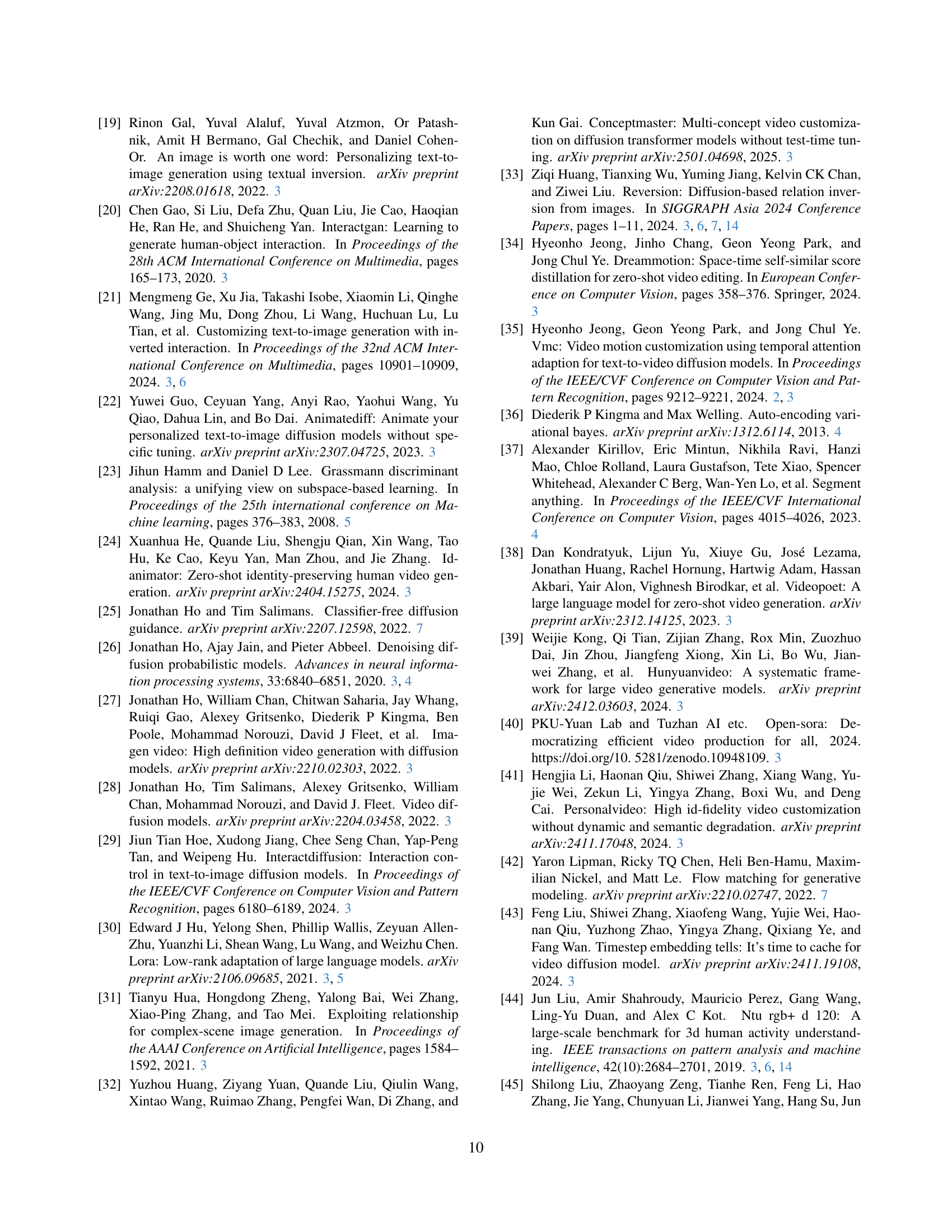
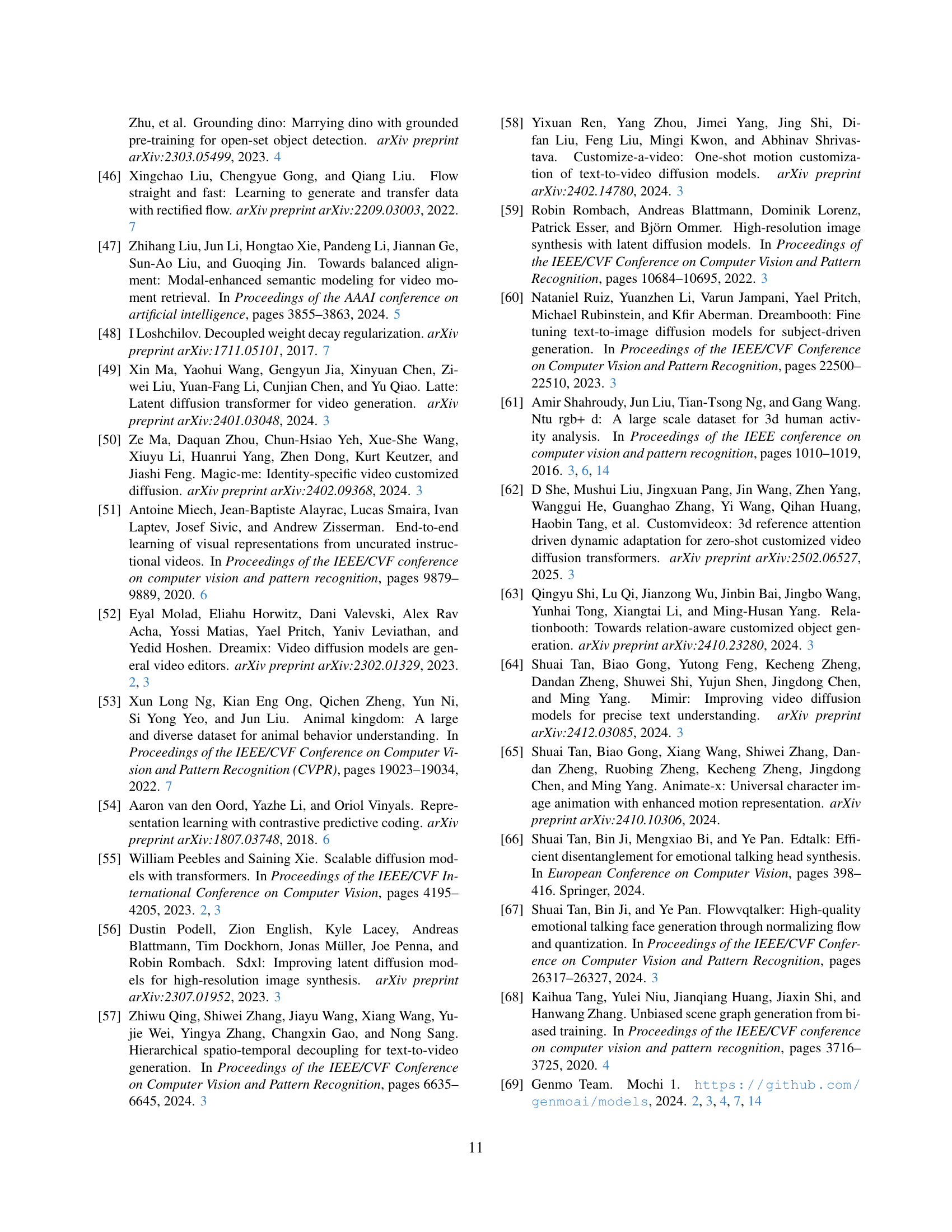
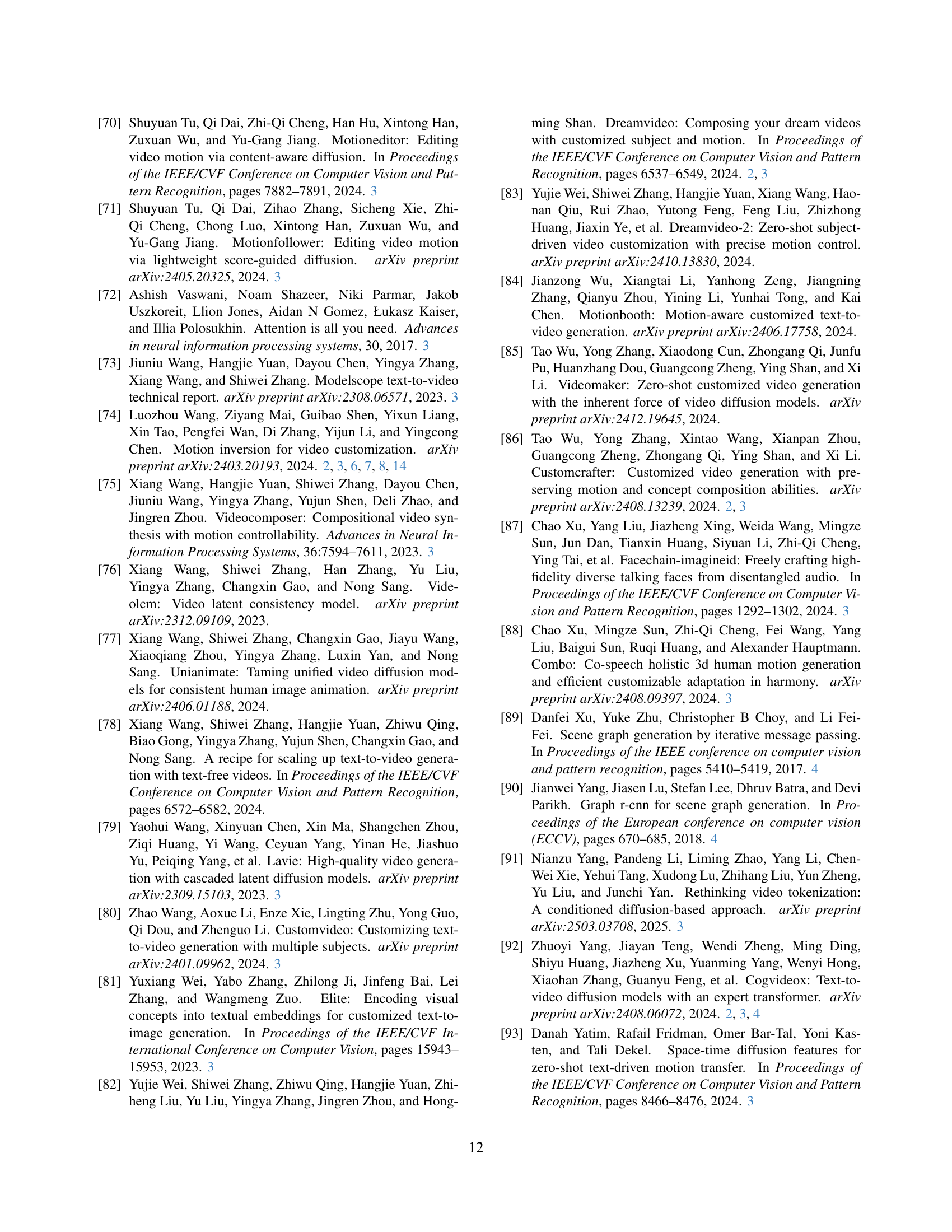
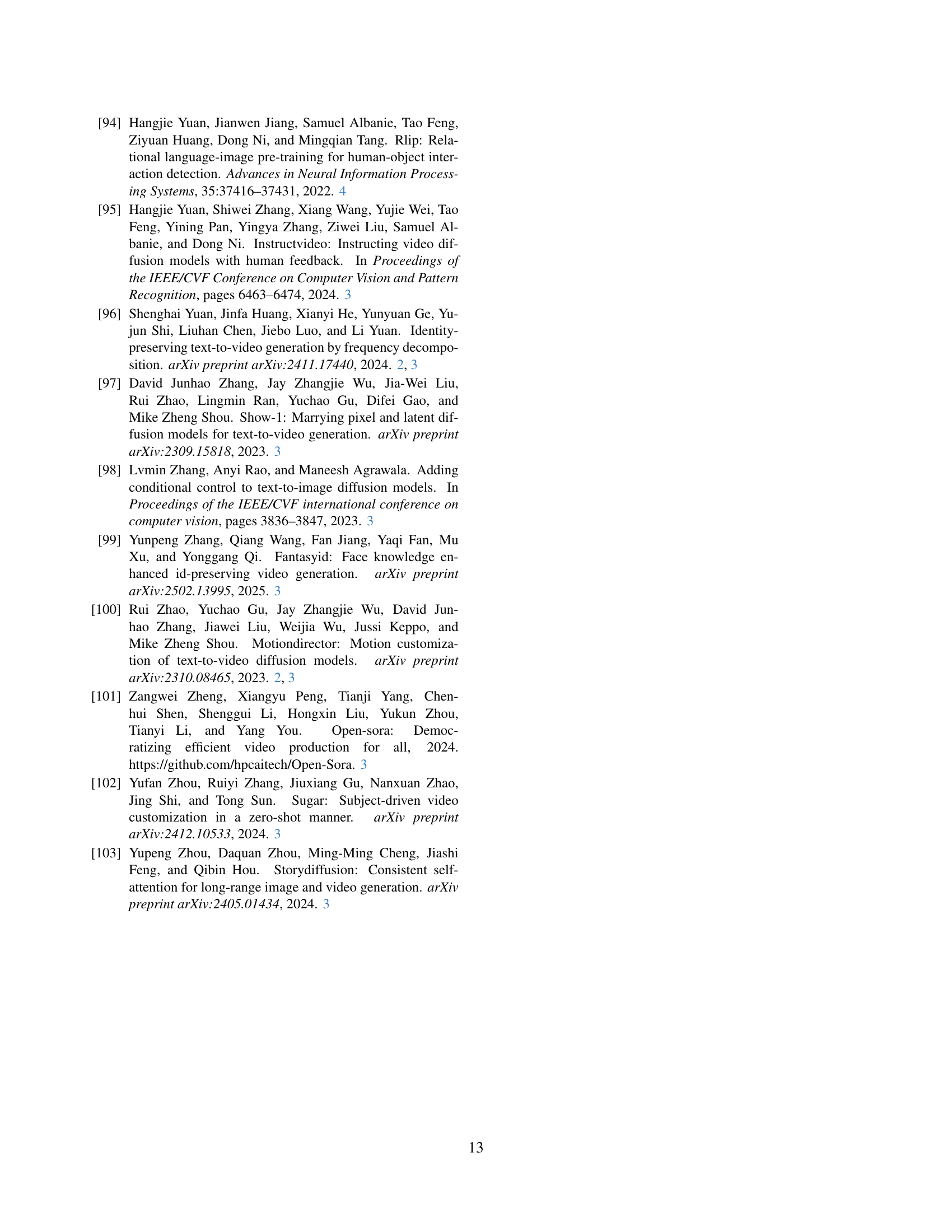
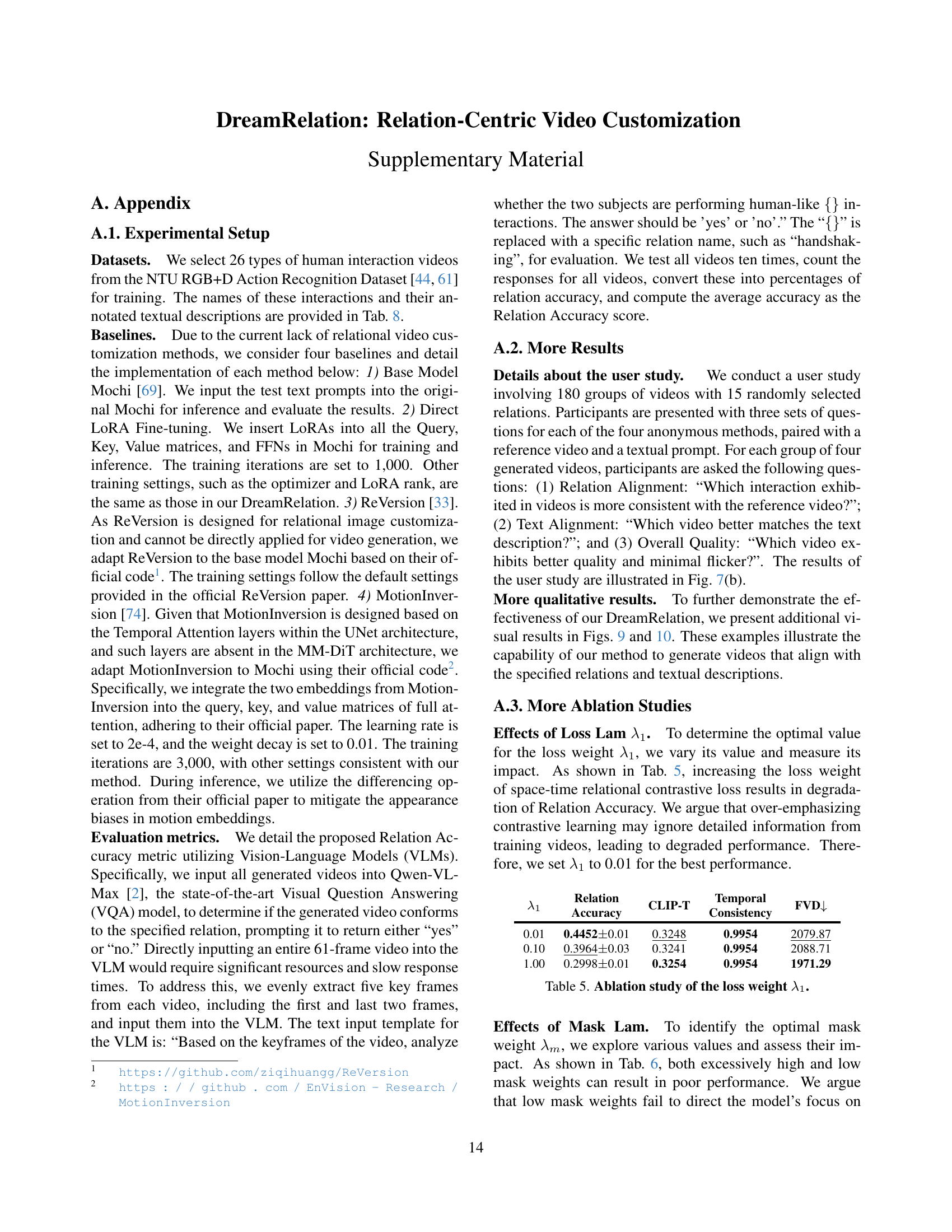
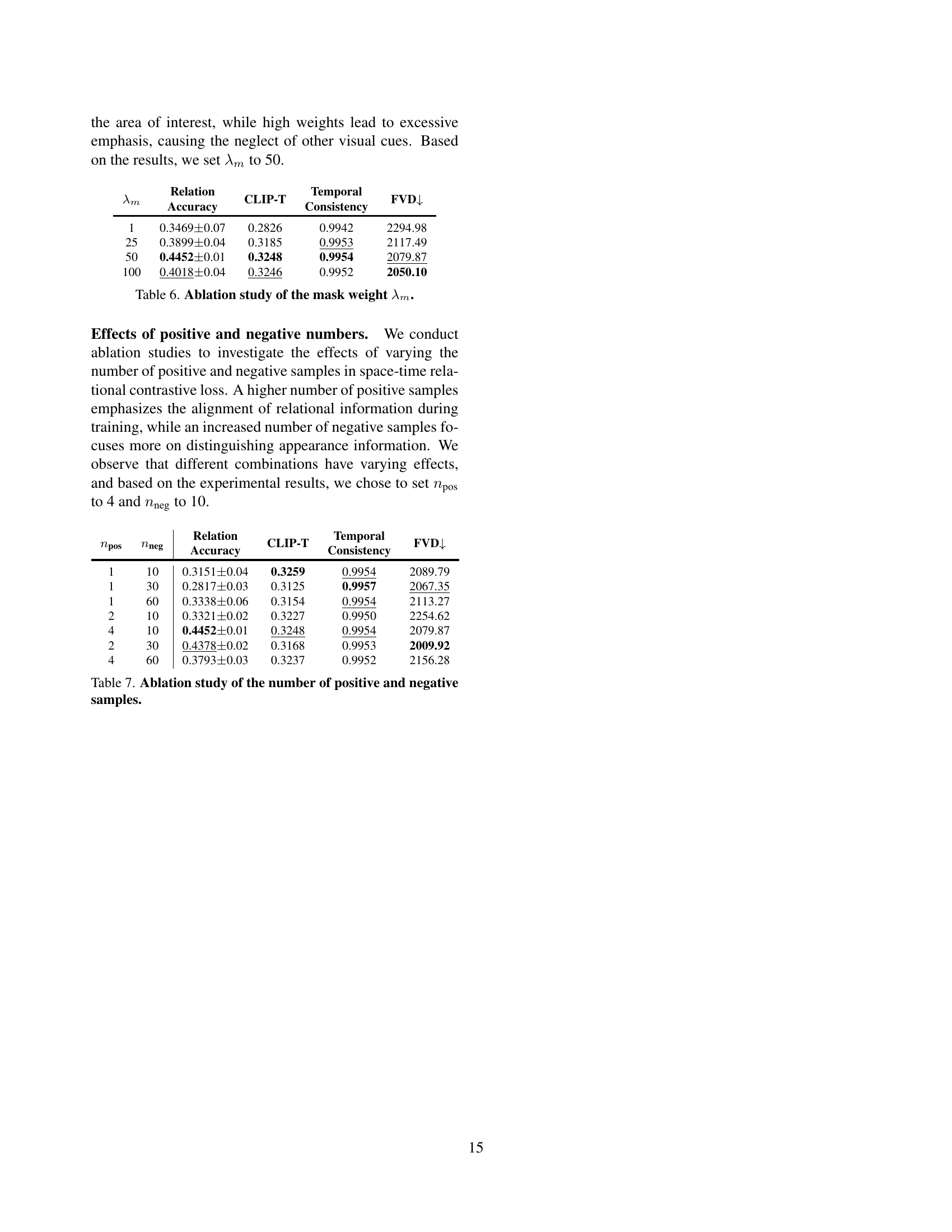
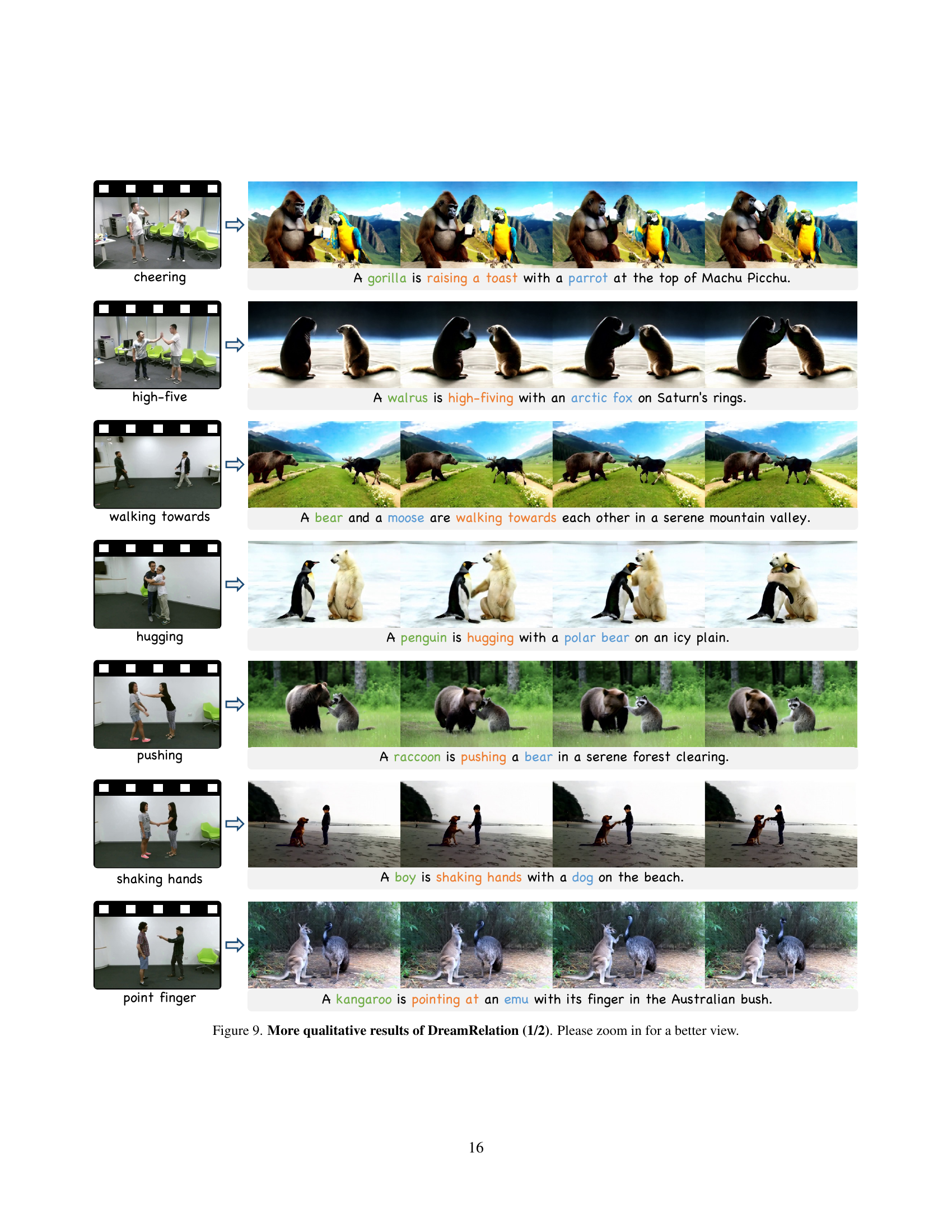
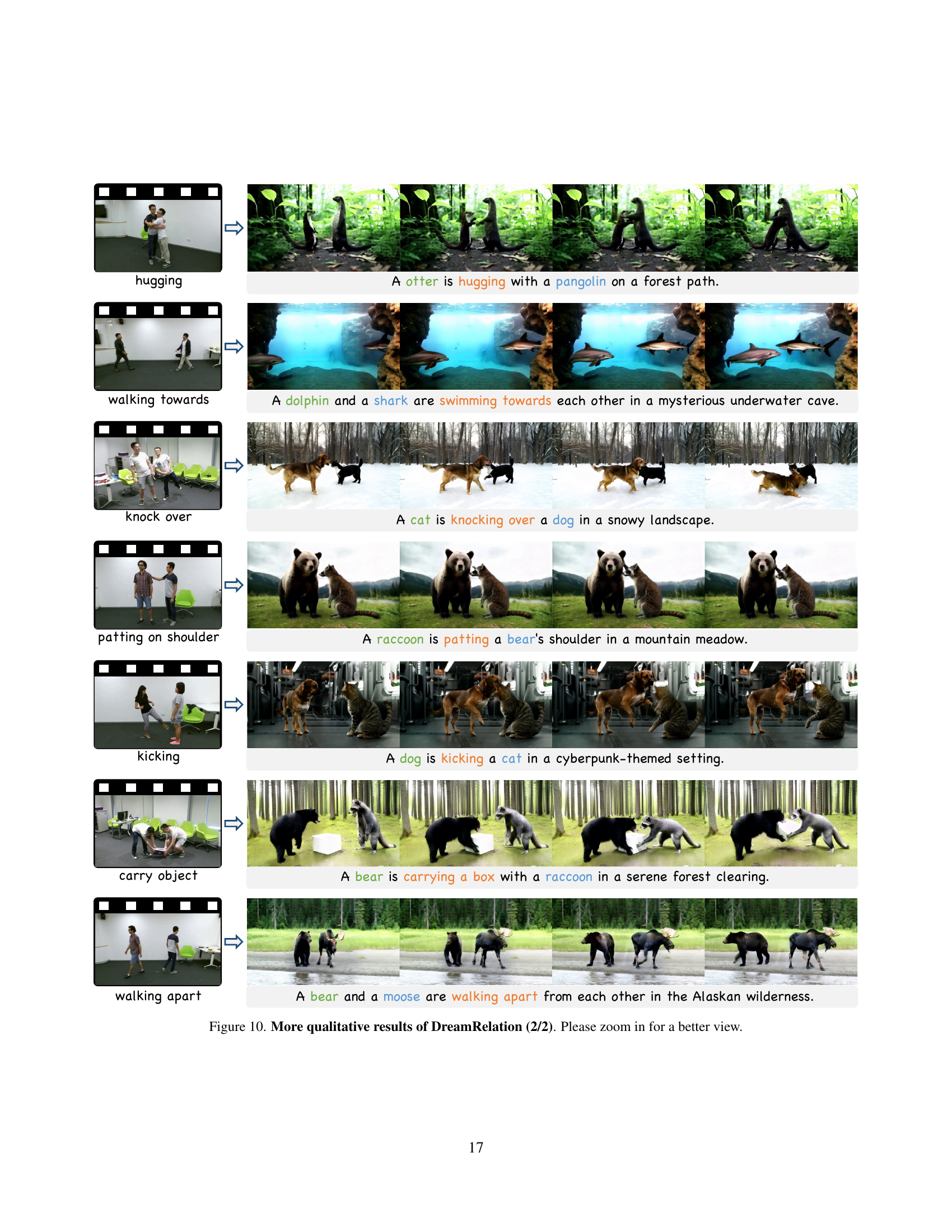
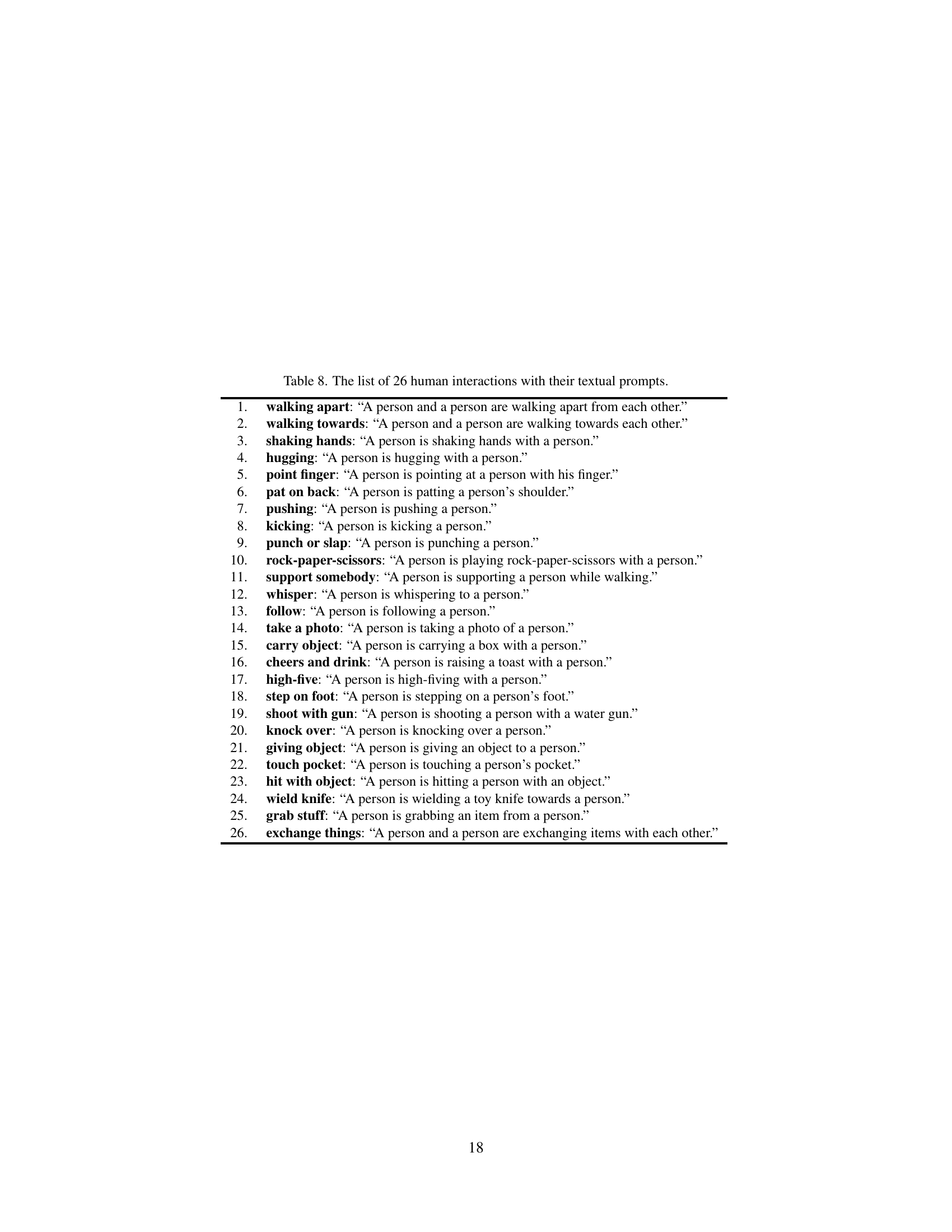