TL;DR#
Unified multimodal models (UMMs) show promise in computer vision, but face-specific research lags behind, especially in fine-grained details and generation. Current face methods often treat understanding and generation separately, limiting their effectiveness. To address this, a first UMM tailored specifically for face understanding and generation is introduced. The proposed model aims to train specifically for fine-grained face understanding and generation.
In response to the above issue, the paper proposes UniFace, trained on UniF²ace-130K, a new dataset with detailed image-text pairs and VQAs spanning facial attributes. A dual discrete diffusion (D3Diff) loss bridges masked generative models and score-based diffusion, improving face synthesis. The results of extensive experiments demonstrate that UniFace outperforms existing UMMs and generative models and achieves superior performance across understanding and generation tasks.
Key Takeaways#
Why does it matter?#
This paper introduces UniFace and UniF2ace-130K, which represents a significant advancement in face understanding and generation. The research offers researchers a robust platform for investigating multimodal learning, prompting further investigation into specialized UMMs and refined facial analysis techniques.
Visual Insights#
🔼 UniFace is a novel unified multimodal model designed for both fine-grained face understanding and generation. It excels at tasks like visual question answering (VQA), where it can accurately describe detailed facial features from an image, and face image captioning, generating rich, descriptive captions. Furthermore, it enables text-to-face image generation, producing high-quality images from textual descriptions with accurate fine-grained details. The figure showcases examples of its capabilities in each of these areas, demonstrating the model’s ability to capture subtle facial attributes and nuances.
read the caption
Figure 1: UniF2superscriptF2\textbf{F}^{2}F start_POSTSUPERSCRIPT 2 end_POSTSUPERSCRIPTace is the first unified multimodal model specifically designed for face understanding and generation, encompassing tasks such as visual question answering, face image captioning and text-to-face image generation. The generated responses and images demonstrate UniF2superscriptF2\textbf{F}^{2}F start_POSTSUPERSCRIPT 2 end_POSTSUPERSCRIPTace’s significant potential in capturing fine-grained face attributes.
Type | Model | Method | # Params | VQAscore-CF5 | VQAscore-LV | FID | VLM-score |
Gen. Only | LlamaGen [53] | AR | 0.8B | 0.746 | 0.551 | 183.466 | 49.773 |
DALL-E 3 [3] | AR | - | 0.845 | 0.644 | 106.477 | 50.122 | |
SD3 [14] | Diff | 2B | 0.903 | 0.671 | 93.471 | 75.944 | |
SDXL [43] | Diff | 2.6B | 0.876 | 0.660 | 123.095 | 72.764 | |
Und. and Gen. | TokenFlow [44] | AR | 7B | 0.871 | 0.664 | 98.194 | 73.177 |
OmniFlow [23] | Diff | 3.4B | 0.798 | 0.585 | 180.933 | 24.96 | |
JanusFlow [36] | AR + Diff | 1.3B | 0.881 | 0.653 | 72.825 | 61.593 | |
Show-o [67] | AR + Diff | 1.3B | 0.855 | 0.650 | 142.557 | 75.618 | |
Uniace(Ours) | AR + Diff | 1.8B | 0.894 | 0.679 | 66.005 | 88.049 |
🔼 Table 1 compares the performance of UniFace, a novel unified multimodal model for fine-grained face understanding and generation, against other state-of-the-art (SOTA) models. It evaluates both generative capabilities (using metrics such as VQAscore-CF5, VQAscore-LV, FID, and VLM-score) and the model’s parameter size. The results demonstrate that UniFace achieves the best overall performance among models with similar parameter counts and performs comparably to, or even better than, significantly larger models. Bold values indicate the absolute best performance, and underlined values highlight the best performance within either the ‘Generation Only’ or ‘Understanding and Generation’ model categories. Larger model sizes are indicated in red.
read the caption
Table 1: Comparing the generative capability of UniF2superscriptF2\textbf{F}^{2}F start_POSTSUPERSCRIPT 2 end_POSTSUPERSCRIPTace with other generative and unified multimodal models, UniF2superscriptF2\textbf{F}^{2}F start_POSTSUPERSCRIPT 2 end_POSTSUPERSCRIPTace achieves state-of-the-art performance for models of the same parameter size and delivers comparable or superior results against larger models. Bold indicates the best performance overall, while underlined denotes the best among Und. and Gen. types. We use red to highlight the larger model size than ours.
In-depth insights#
UniFace: Face UMM#
UniFace: A Unified Multimodal Model (UMM) for Faces represents a significant stride towards holistic face understanding and generation. The model’s core strength lies in its ability to bridge the gap between understanding intricate facial details and generating high-fidelity face images from text. It underscores the growing importance of UMMS in AI, extending their utility to the nuanced realm of facial analysis. This approach streamlines different tasks, enhancing flexibility and performance, moving beyond traditional task-specific models. By unifying understanding and generation, UniFace captures complex interdependencies between facial attributes and textual descriptions, enabling more context-aware and human-like interactions. Its design likely incorporates mechanisms for fine-grained representation learning and cross-modal alignment, which is crucial for accurately interpreting and synthesizing facial features from diverse data sources. Potential architectures may include transformers or attention mechanisms. UniFace not only advances the field but also establishes a robust platform for future research, offering a unified framework for exploring the interplay between facial attributes and multimodal inputs.
MoE for fine detail#
Mixture of Experts (MoE) is crucial for capturing the intricate details in a task, particularly in scenarios that require fine-grained understanding. The MoE architecture is beneficial to model subtle nuances, allowing different experts to specialize in distinct features. Token/Sequence-level MoEs enable the model to adaptively handle diverse attributes by dynamically selecting relevant experts. This results in efficient representation learning for both understanding and generation tasks. Through selective information processing, MoE aids in capturing minute facial nuances, improving overall model performance. By concentrating on essential details, MoEs facilitate enhanced feature extraction. This specialized approach is essential for intricate tasks.
D3Diff for realism#
D3Diff, or Dual Discrete Diffusion, emerges as a pivotal training strategy meticulously crafted to enhance the synthesis of fine-grained facial details. By theoretically connecting masked generative models with score-based diffusion models, D3Diff facilitates the simultaneous optimization of evidence lower bounds (ELBOs). This is significant because typical masked generative losses rely solely on likelihood, whereas D3Diff optimizes two distinct upper bounds of maximum likelihood, leading to higher-fidelity face generation. D3Diff leverages the theory of score matching in discrete diffusion, thereby bolstering the synthesis of subtle facial attributes. This strategy ensures that the generative model is not just producing faces, but is meticulously capturing intricate details. D3Diff serves to meticulously synthesize facial details, enabling UMMs to capture more human-like nuances. It also guarantees superior performance in face generation tasks.
UniFace-130K Data#
UniFace-130K is a dataset created to address the limitations of existing facial datasets for multimodal modeling. It contains 130K facial image-text pairs with detailed captions, as well as one million VQA pairs. The dataset covers a wide range of facial attributes related to appearance, actions, and emotions. Images come from CelebV-HQ, FFHQ, and MM-CelebA-HQ datasets. A two-stage caption generation refines MLLM-generated captions with face attribute classifiers. GPT-40 then generates diverse VQAs based on these captions. This ensures fine-grained descriptions and enhanced VQAs. The dataset aims to advance facial image understanding and generation, providing a solid foundation for training and evaluating multimodal models.
AGI face research#
AGI face research represents a pivotal frontier in artificial intelligence, aiming to develop systems capable of understanding and generating realistic, nuanced facial representations. This involves not only creating high-fidelity images but also enabling AI to interpret and respond to facial cues like expressions and micro-expressions. The challenge lies in capturing the complexity of human faces and behaviors, which requires advanced multimodal models that integrate visual and textual data. Progress in this area can revolutionize human-computer interaction, enabling more intuitive and empathetic AI systems. Overcoming limitations in existing models, creating specialized datasets, and innovating in network architectures are key to advancing AGI in face-related tasks.
More visual insights#
More on figures
🔼 This figure illustrates the creation of the UniF2ace-130K dataset. The left panel details a three-step pipeline: Step 1 involves collecting high-quality facial images; Step 2 uses GPT-40 and a fine-grained face attribute classification model to generate detailed captions, correcting and enhancing initial captions; and Step 3 creates visual question-answering (VQA) pairs using GPT-4. The right panel showcases examples demonstrating how this process refines GPT-40 generated captions, resulting in more accurate, comprehensive descriptions that include corrected attributes (e.g., gender), enhanced details (e.g., bags under eyes), and inferred information (e.g., a person is talking and seems slightly tired).
read the caption
Figure 2: Pipeline and examples of UniF2superscriptF2\textbf{F}^{2}F start_POSTSUPERSCRIPT 2 end_POSTSUPERSCRIPTace-130K construction. Left: A three-stage pipeline for building UniF2superscriptF2\textbf{F}^{2}F start_POSTSUPERSCRIPT 2 end_POSTSUPERSCRIPTace-130K. Step-1: High-quality face images are collected. Step-2: Detailed captions are generated by GPT-4o with a face attribute model trained to classify fine-grained appearance, action, and emotion. Step-3: Question-answering pairs are created. These stages collectively refine GPT-4o-generated captions and produce fine-grained descriptions for VQAs generation. Right: A representative example showcasing UniF2superscriptF2\textbf{F}^{2}F start_POSTSUPERSCRIPT 2 end_POSTSUPERSCRIPTace-130K’s ability to correct (e.g., gender), enhance (e.g., bags under eyes), and reason (e.g., talking, slight tiredness) in GPT-4o-generated captions.
🔼 UniFace, a unified multimodal model, processes both text and image inputs to perform Text-to-Image (T2I) and Multimodal Understanding (MMU) tasks. Text is tokenized, and images are encoded using VQGAN. These are combined into a single sequence. A noise scheduler then masks a portion of the image tokens. The masked tokens are fed through a Transformer network containing Mixture-of-Experts (MoE) layers. The MoEs are divided into generation and understanding groups. The first group of MoEs operates at the token level, using both shared and routed experts, while the second group operates at the sequence level and incorporates domain-specific features. This hierarchical architecture allows for fine-grained processing of facial features. The model’s training objective combines a D3Diff loss (based on the noise scheduler’s output pt(xt|x0)) and a text autoregressive loss.
read the caption
Figure 3: Our UniF2superscriptF2\textbf{F}^{2}F start_POSTSUPERSCRIPT 2 end_POSTSUPERSCRIPTace architecture integrates Text-to-Image (T2I) and Multimodal Understanding (MMU) tasks. Text inputs are encoded via a tokenizer, while input images are processed through a VQGAN encoder, merging into a unified token sequence. A noise scheduler masks a subset of image tokens, which are then processed by a Transformer with Mixture-of-Experts (MoE) layers. These MoE layers are grouped for generation and understanding tasks, with the first operating at the token level using shared and routed experts, and the second incorporating domain-specific features at the sequence level. This hierarchical design enables fine-grained facial feature processing. The noise scheduler outputs pt(𝐱t|𝐱0)subscript𝑝𝑡conditionalsubscript𝐱𝑡subscript𝐱0p_{t}(\mathbf{x}_{t}|\mathbf{x}_{0})italic_p start_POSTSUBSCRIPT italic_t end_POSTSUBSCRIPT ( bold_x start_POSTSUBSCRIPT italic_t end_POSTSUBSCRIPT | bold_x start_POSTSUBSCRIPT 0 end_POSTSUBSCRIPT ) for D3Diff loss computation, combined with text autoregressive loss to form the training objective.
🔼 Figure 4 presents a comparison of face image generation results from five different models: SDXL, TokenFlow, OmniFlow, Show-o, and UniFace. The goal is to showcase UniFace’s superior ability to generate high-fidelity images that accurately reflect the details specified in text prompts. Each model is given the same prompt, and the resulting images are displayed alongside the prompt used to generate them. By comparing the generated images, one can observe that UniFace produces images with significantly more detail and accuracy in capturing fine-grained attributes (such as hair color, clothing, and facial features) compared to the other models. The highlighted attributes in the figure emphasize UniFace’s strength in this area.
read the caption
Figure 4: Comparative analysis of face images generation quality across SDXL [43], TokenFlow [44], OmniFlow [23], Show-o [67], and UniF2superscriptF2\textbf{F}^{2}F start_POSTSUPERSCRIPT 2 end_POSTSUPERSCRIPTace. Our proposed UniF2superscriptF2\textbf{F}^{2}F start_POSTSUPERSCRIPT 2 end_POSTSUPERSCRIPTace effectively captures more detailed information from prompts. We highlight fine-grained attributes.
🔼 This figure visualizes the activation frequencies of the Mixture-of-Experts (MoE) layers within the UniFace model. It’s broken down into token-level and sequence-level MoE activations for both understanding and generation tasks. The size of the circles in the heatmaps directly corresponds to the frequency of activation for each expert within each layer. Larger circles represent more frequent activation. The left column shows the understanding tasks (image-to-text), while the right column shows the generation tasks (text-to-image). This allows for a visual comparison of expert usage patterns between the two main tasks and across different layers of the network.
read the caption
Figure 5: Activation frequency of Token-Level and Sequence-Level MoE in different layers. The left column corresponds to understanding tasks, while the right column corresponds to generation tasks. Larger circles indicate experts that are activated more frequently.
🔼 Figure 6 presents a comparison of visual question answering (VQA) performance between UniFace and other state-of-the-art models. UniFace excels at generating detailed and accurate answers to complex questions about facial features. The figure demonstrates UniFace’s ability to capture and express subtle nuances of facial attributes such as hairstyles, earrings, or expressions, offering a superior level of detail and accuracy compared to alternative approaches. GPT-40 scores are also included to provide a benchmark against a powerful large language model.
read the caption
Figure 6: Comparison of visual question-answering results and GPT-4o-based scores.
🔼 This figure shows a comparison of face images generated by different models: SDXL, TokenFlow, OmniFlow, Show-o, and UniFace (the authors’ model). For each model, several example images are displayed alongside a textual description of the generated face. This visual comparison highlights the differences in image quality, detail, and adherence to the prompt across various models. UniFace demonstrates a higher level of detail and accuracy in capturing fine-grained features compared to other models.
read the caption
Figure 1: More comparison of generated face images with other models.
🔼 This figure showcases additional examples of faces generated by the UniFace model. It demonstrates the model’s ability to produce diverse and high-quality images of faces with varying attributes and characteristics.
read the caption
Figure 2: More face images generated by UniF2superscriptF2\textbf{F}^{2}F start_POSTSUPERSCRIPT 2 end_POSTSUPERSCRIPTace
🔼 Figure 3 showcases a comparison of captioning results from various models, specifically highlighting fine-grained facial attributes. The figure directly compares the captions generated by different models against a ground truth. Blue highlights indicate correctly identified fine-grained attributes, while red highlights indicate errors or omissions. This allows for a visual assessment of each model’s capability in capturing nuanced facial details and the accuracy of their descriptions. Each model’s caption is presented alongside a numerical score reflecting its performance. This visual representation aids in understanding the relative strengths and weaknesses of each model concerning fine-grained detail in facial attribute identification and description.
read the caption
Figure 3: Comparison of captioning results and DeepSeeek-v3-based scores. We highlight fine-grained attributes with blue and errors in answers with red.
🔼 Figure 4 details the prompts used to create the UniF2ace-130K dataset. The process involved three steps. First, a prompt was given to GPT-40 to generate initial captions describing facial attributes. If the image had existing captions (as in the MM-CelebA-HQ dataset), those were incorporated into the prompt. Second, another prompt was used to refine those captions using additional fine-grained attributes. Third, a prompt was given to GPT-4 to generate visual question-answering (VQA) pairs based on the refined captions, aiming for a detailed understanding of facial attributes.
read the caption
Figure 4: Prompts for building dataset. The first and second prompts are to GPT-4o, while the last prompt is to GPT-4. In the first prompt, the content in “[]” is used only when the image data includes built-in captions, such as in MM-CelebA-HQ dataset.
More on tables
Type | Model | Method | # Params | Desc-GPT | Conv-GPT | Desc-DS | Conv-DS |
Und. Only | VILA1.5 [28] | AR | 3B | 4.76 | 5.20 | 6.56 | 6.54 |
Qwen2-VL [59] | AR | 7B | 5.16 | 6.27 | 5.50 | 6.86 | |
LLaVA-v1.5 [31] | AR | 7B | 4.28 | 5.48 | 4.84 | 6.20 | |
InternVL2.5 [7] | AR | 8B | 5.62 | 5.89 | 6.30 | 6.55 | |
Und. and Gen. | TokenFlow [44] | AR | 7B | 5.02 | 5.80 | 5.82 | 6.39 |
OmniFlow [23] | Diff | 3.4B | 1.62 | - | 1.90 | - | |
JanusFlow [36] | AR + Diff | 1.3B | 4.88 | 6.06 | 5.42 | 6.77 | |
Show-o [67] | AR + Diff | 1.3B | 3.88 | 4.17 | 5.24 | 4.90 | |
Uniace(Ours) | AR + Diff | 1.8B | 6.02 | 6.53 | 7.38 | 7.29 |
🔼 Table 2 presents a comparison of UniFace’s performance on face understanding tasks against several state-of-the-art multimodal models, including both advanced models focusing solely on understanding and unified multimodal models capable of both understanding and generation. The metrics used assess the model’s ability to extract and analyze detailed facial features from images. UniFace significantly outperforms all other models across all evaluation metrics, showcasing its superior capabilities in fine-grained face understanding.
read the caption
Table 2: Evaluation on face understanding tasks compared with advanced multimodal models and unified multimodal models. Our UniF2superscriptF2\textbf{F}^{2}F start_POSTSUPERSCRIPT 2 end_POSTSUPERSCRIPTace achieves the highest scores across all metrics, demonstrating superior ability to extract and analyze features from face images.
Loss Type | Weight | VQAscore-CF5 | VQAscore-LV | FID | VLM-score |
D3Diff | 0.1 | 0.887 | 0.673 | 68.903 | 86.378 |
0.01 | 0.894 | 0.679 | 66.005 | 88.049 | |
0.001 | 0.884 | 0.668 | 72.736 | 89.220 | |
Only Mask | 0 | 0.879 | 0.661 | 77.463 | 85.993 |
Only Score | 0.01 | 0.886 | 0.670 | 69.694 | 87.951 |
🔼 This table presents an ablation study comparing the performance of the UniFace model trained with different loss functions. The primary loss function used is D3Diff (Dual Discrete Diffusion), which combines elements of score-matching and masked generative losses. The table shows the results when training with only the score matching loss, only the masked generative loss, and varying the weighting coefficient (α) in the D3Diff loss. Metrics evaluated include VQAscore-CF5, VQAscore-LV, FID, and VLM-score. The best overall results (bold) are achieved with α = 0.01 in the D3Diff loss function, indicating the effectiveness of the dual loss approach. The second-best results (underlined) provide further context for the relative performance of alternative loss functions.
read the caption
Table 3: Performance comparison with different loss. Considering all metrics, the optimal result is achieved with α=0.01𝛼0.01\alpha=0.01italic_α = 0.01 in D3Diff. Bold: Best performance. Underlined: Second best performance.
Token MoE | Sequence MoE | Generation | Understanding | |||
VQAscore | FID | VLM-score | Desc | Conv | ||
✗ | ✗ | 0.878 | 72.877 | 84.432 | 4.988 | 6.031 |
✓ | ✗ | 0.887 | 67.415 | 87.917 | 5.678 | 6.495 |
✗ | ✓ | 0.889 | 69.312 | 86.790 | 5.864 | 6.247 |
✓ | ✓ | 0.894 | 66.005 | 88.049 | 6.023 | 6.532 |
🔼 This table presents an ablation study evaluating the impact of token-level and sequence-level Mixture-of-Experts (MoEs) on the UniFace model’s performance. It shows the results of experiments where each type of MoE was removed individually to measure their contribution to overall performance. The results demonstrate that both token-level and sequence-level MoEs significantly improve the model’s performance across multiple metrics.
read the caption
Table 4: Performance impact of token-level and sequence-level MoE in UniF2superscriptF2\textbf{F}^{2}F start_POSTSUPERSCRIPT 2 end_POSTSUPERSCRIPTace through ablation study. Both MoEs contribute significant performance improvements.
Full paper#
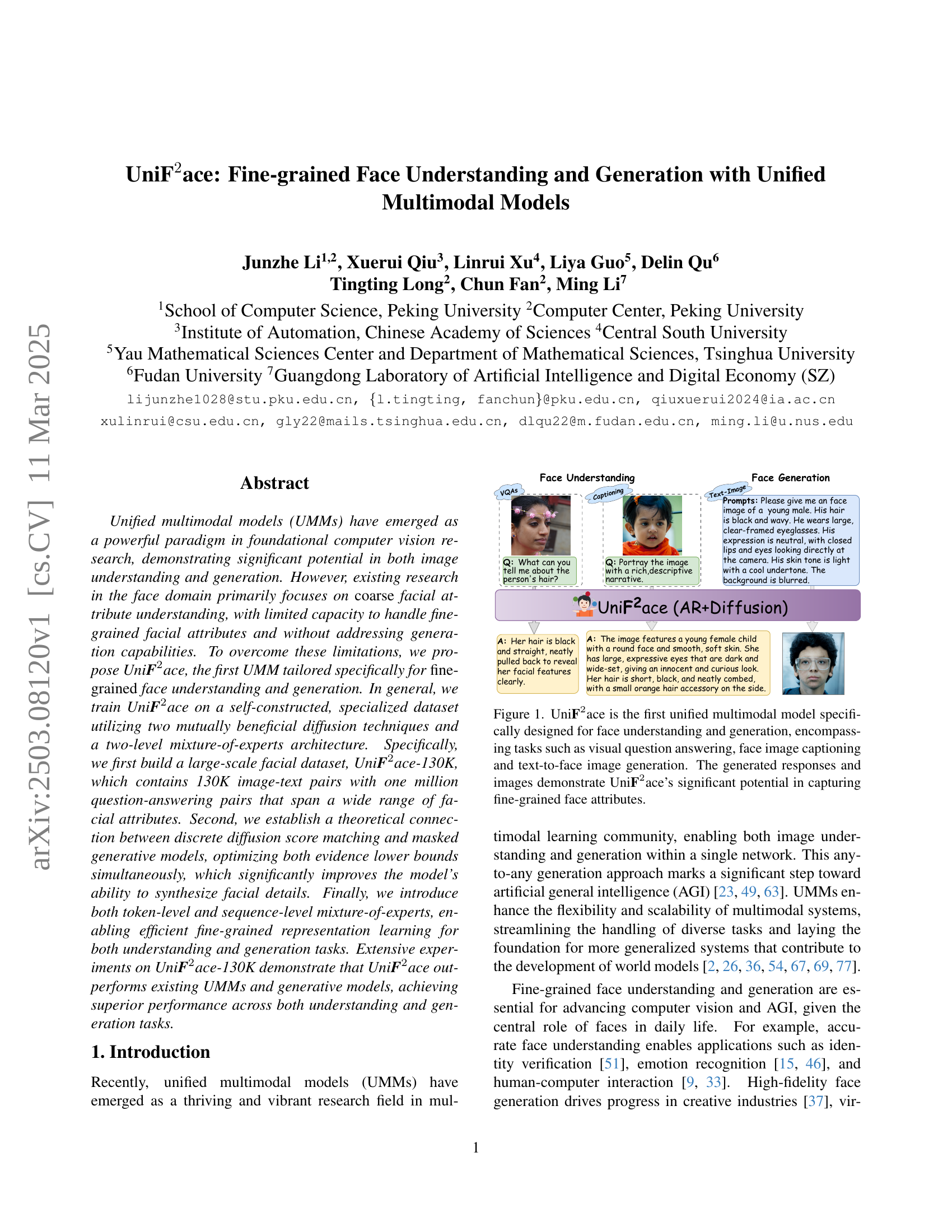
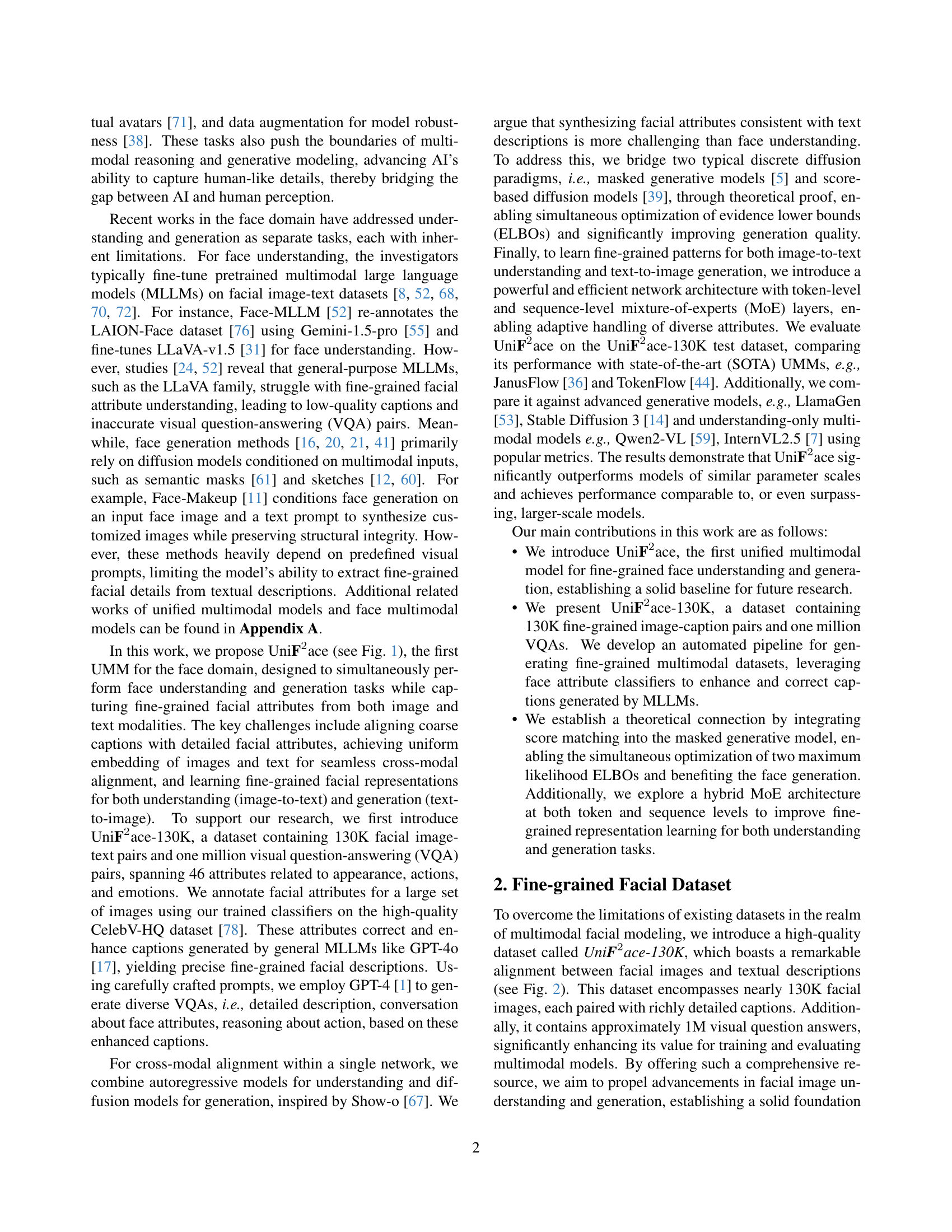
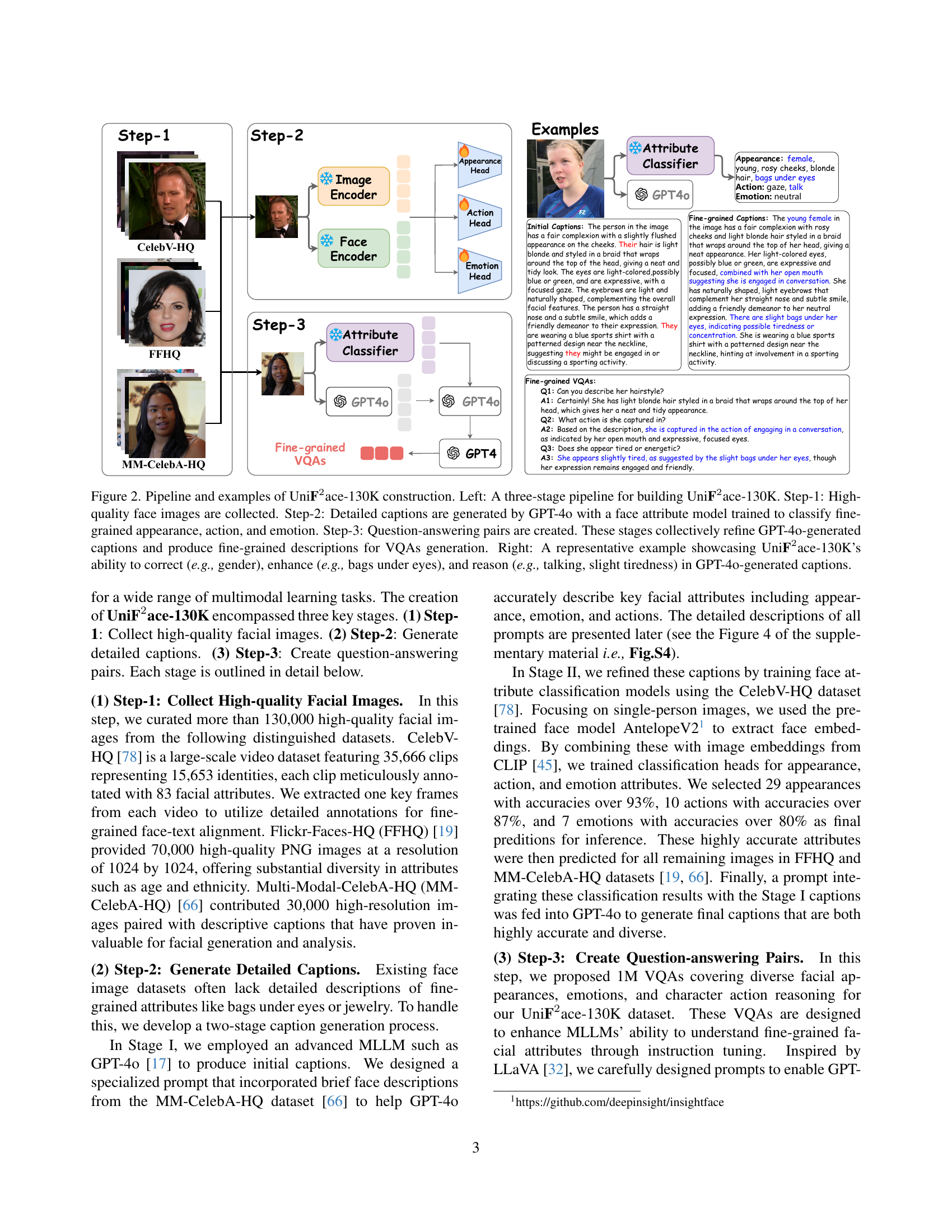
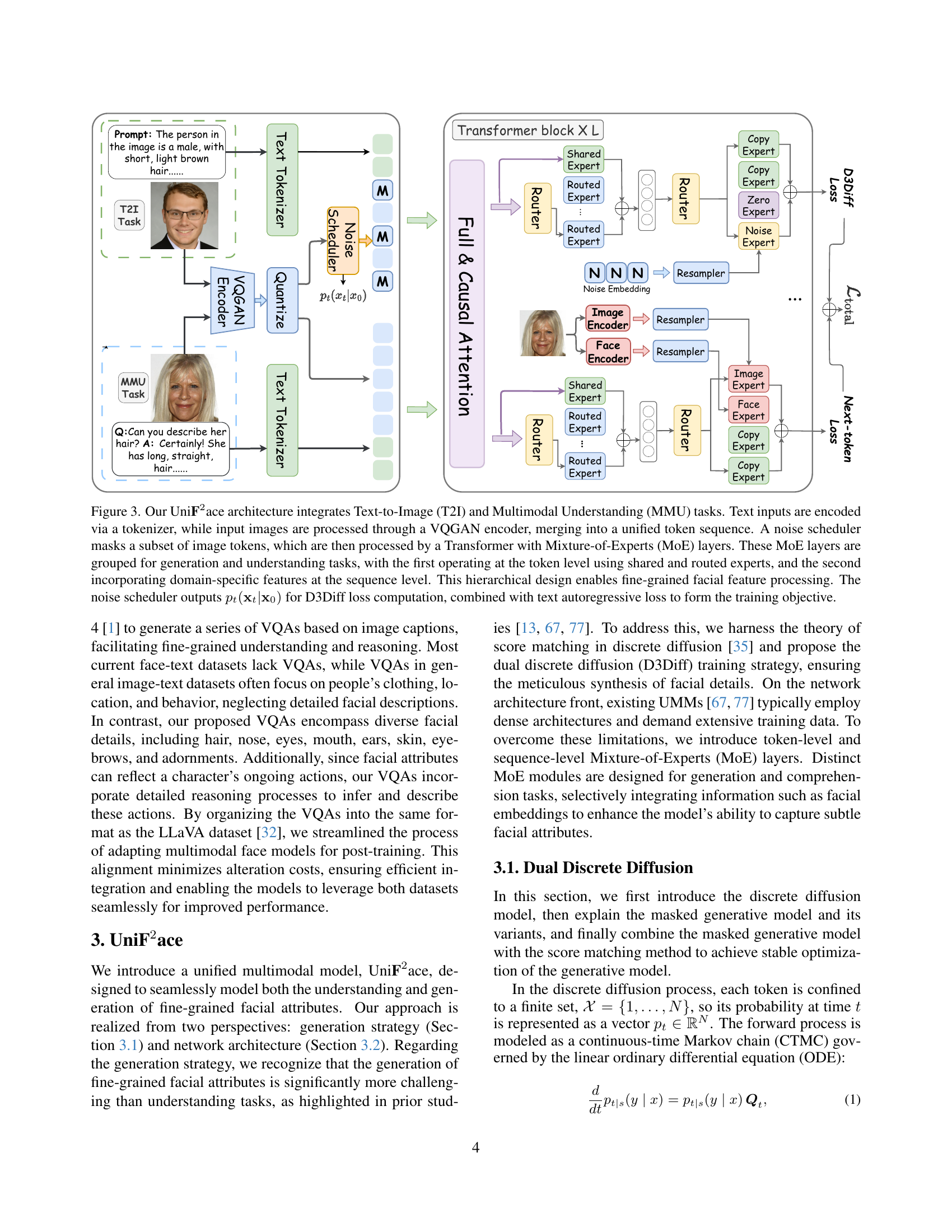
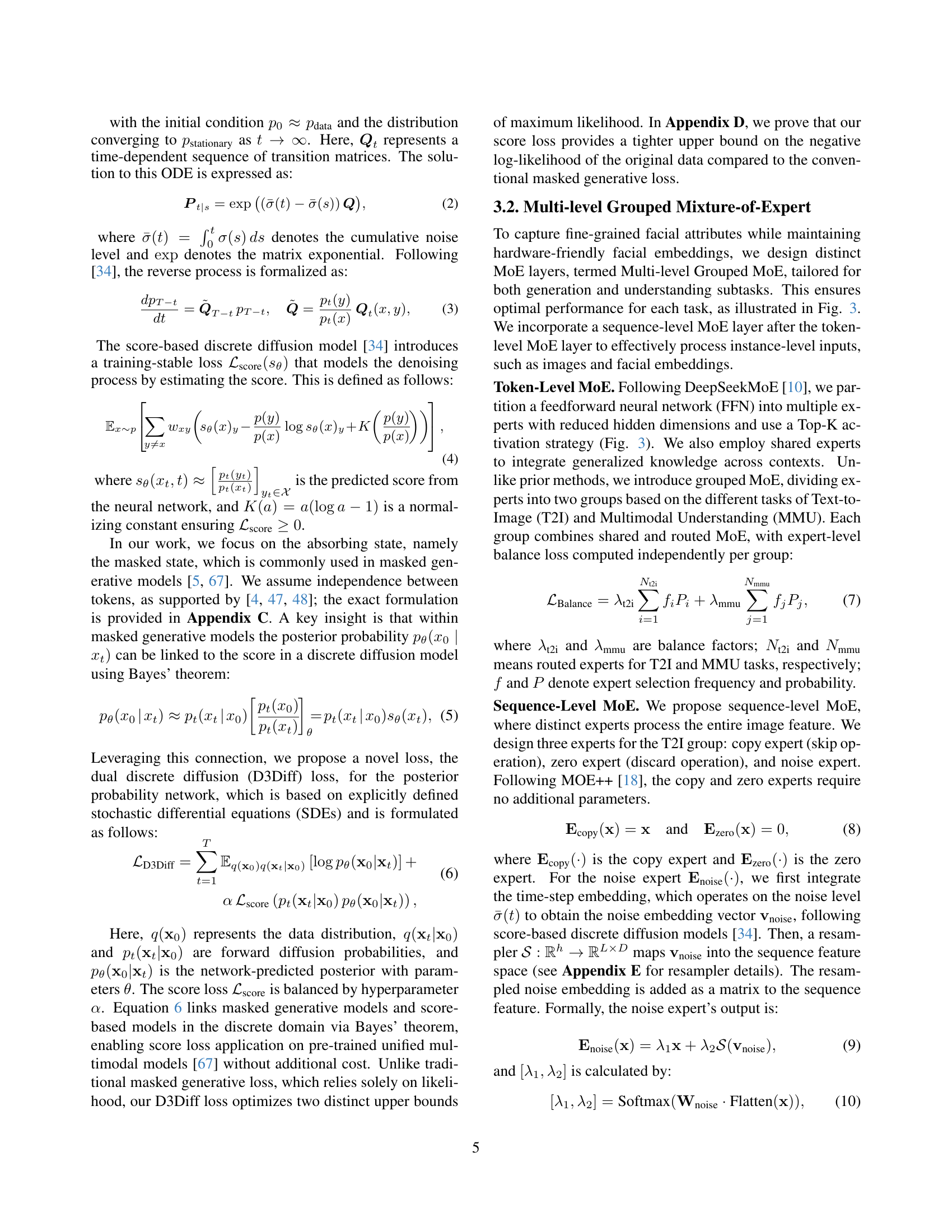
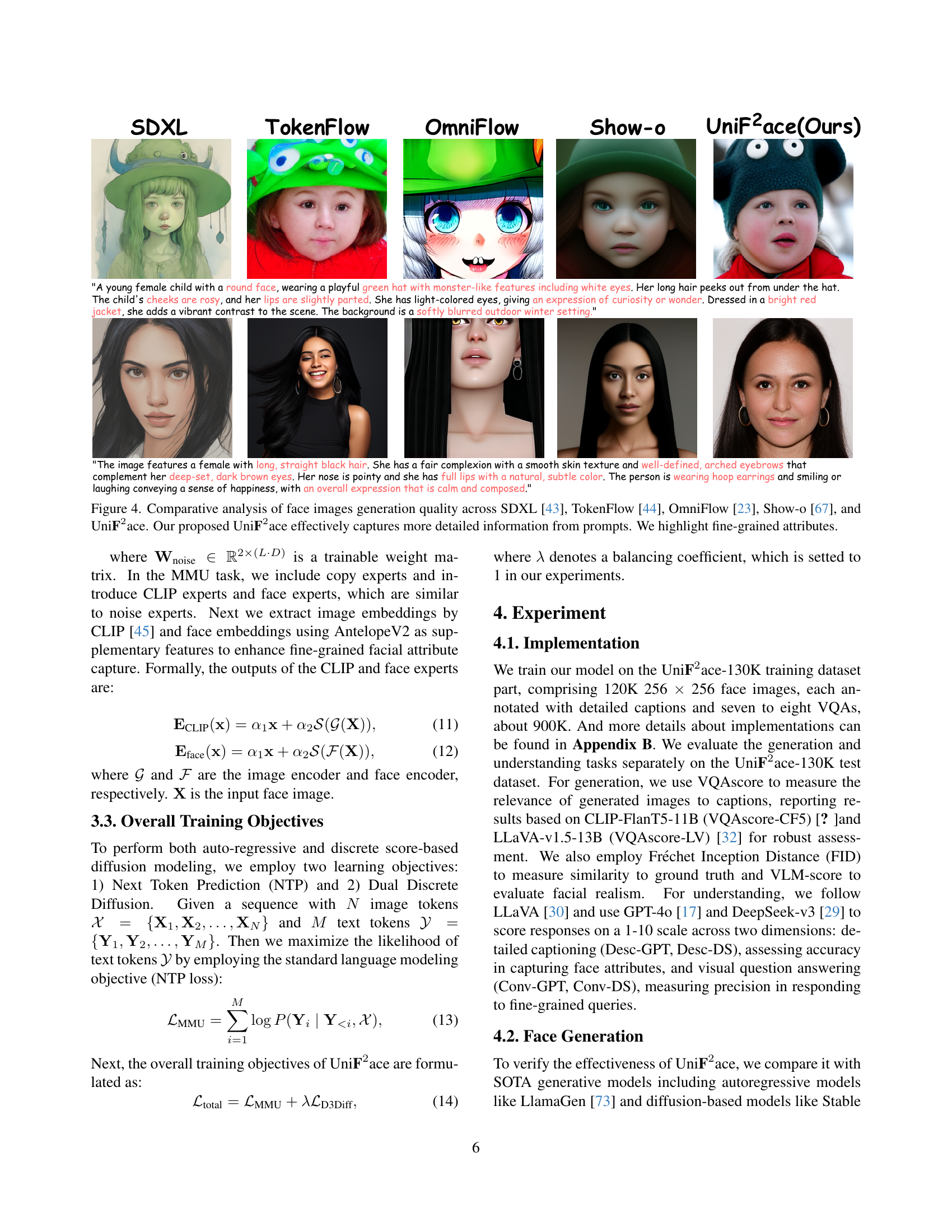
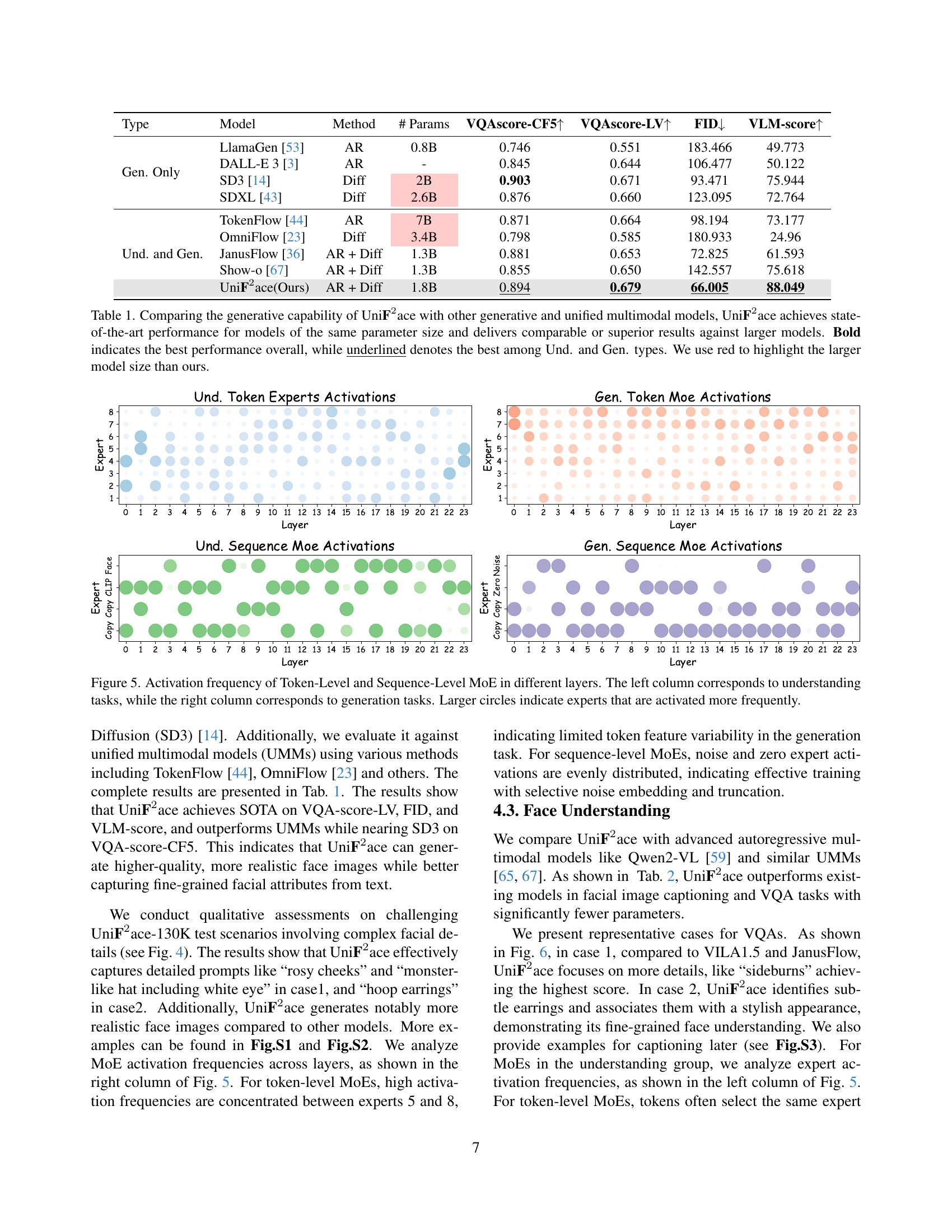
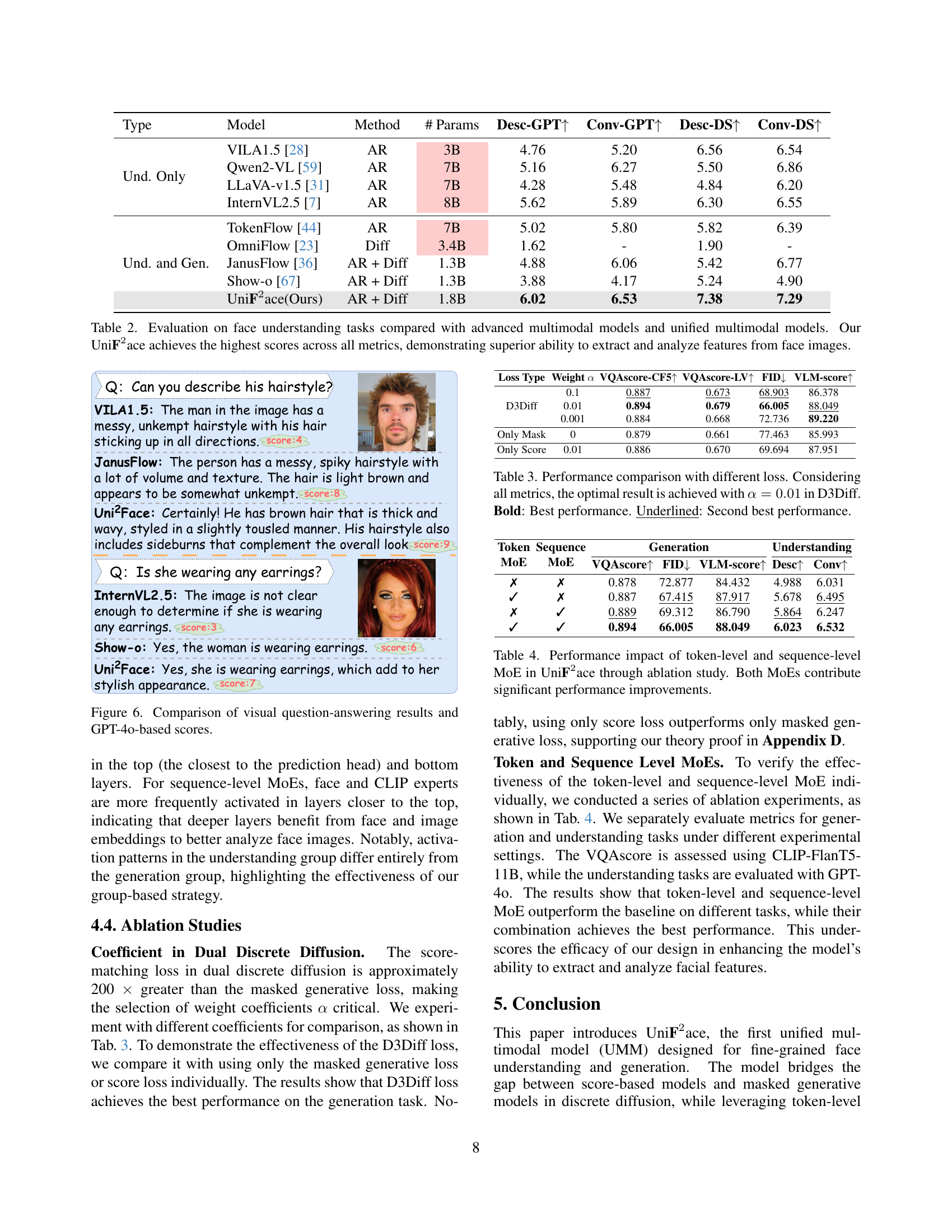
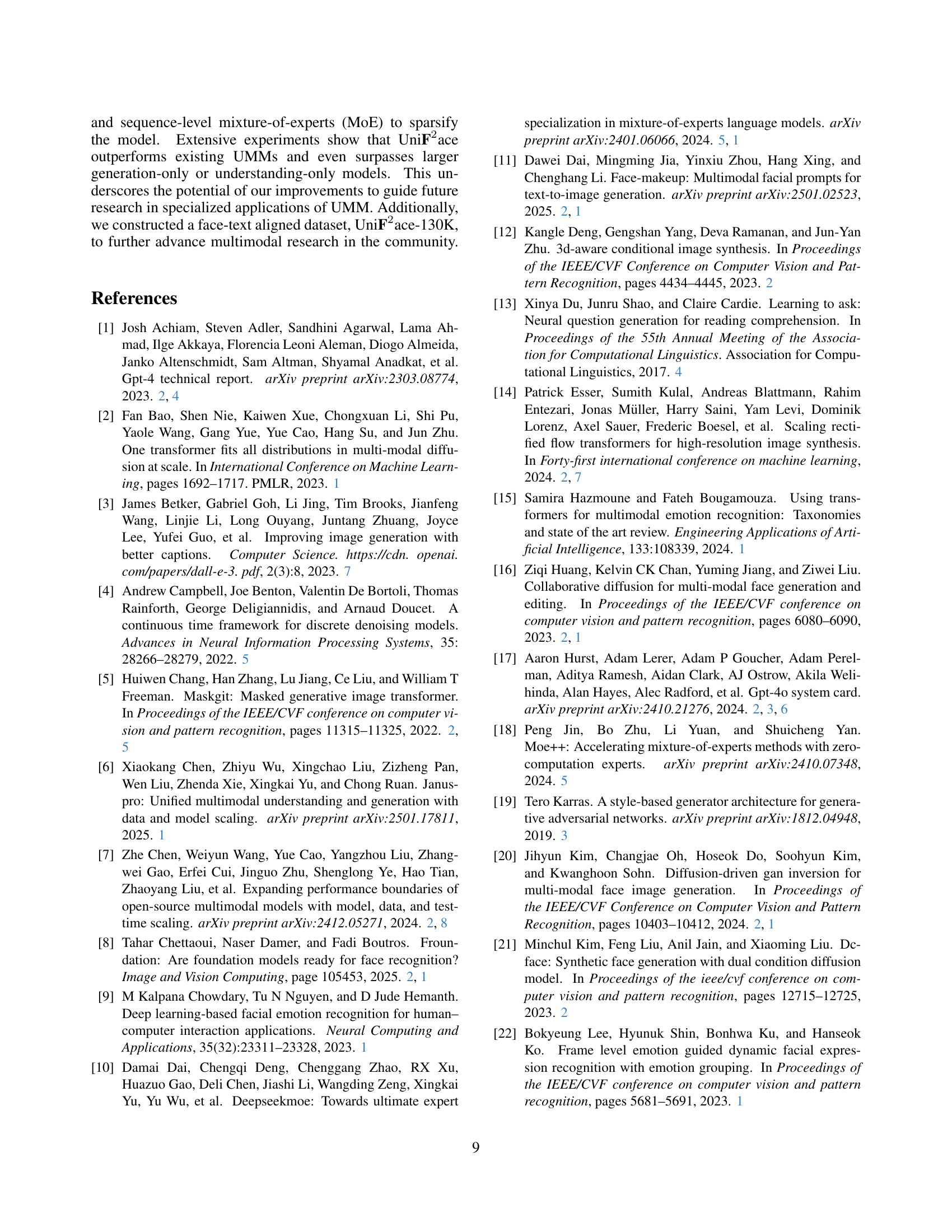
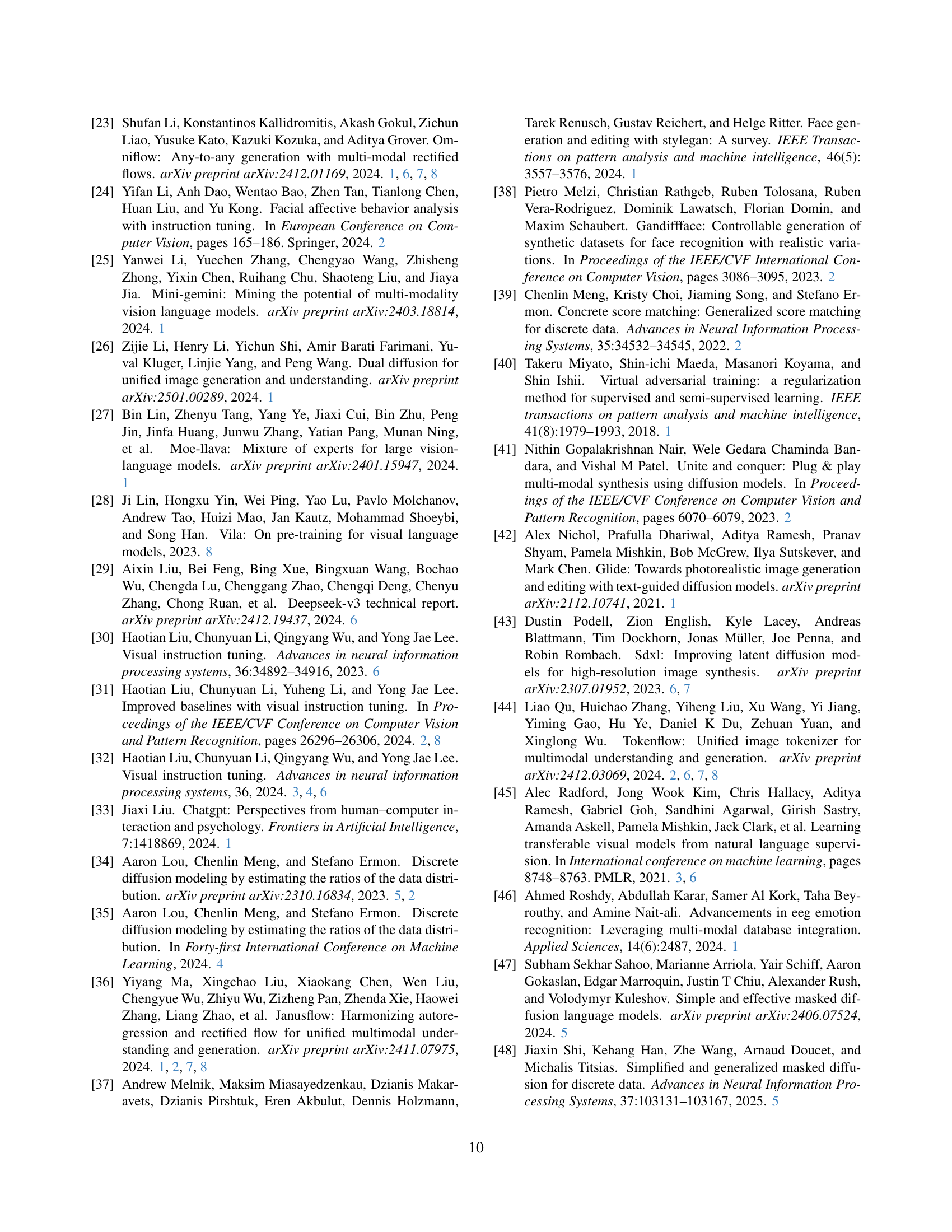
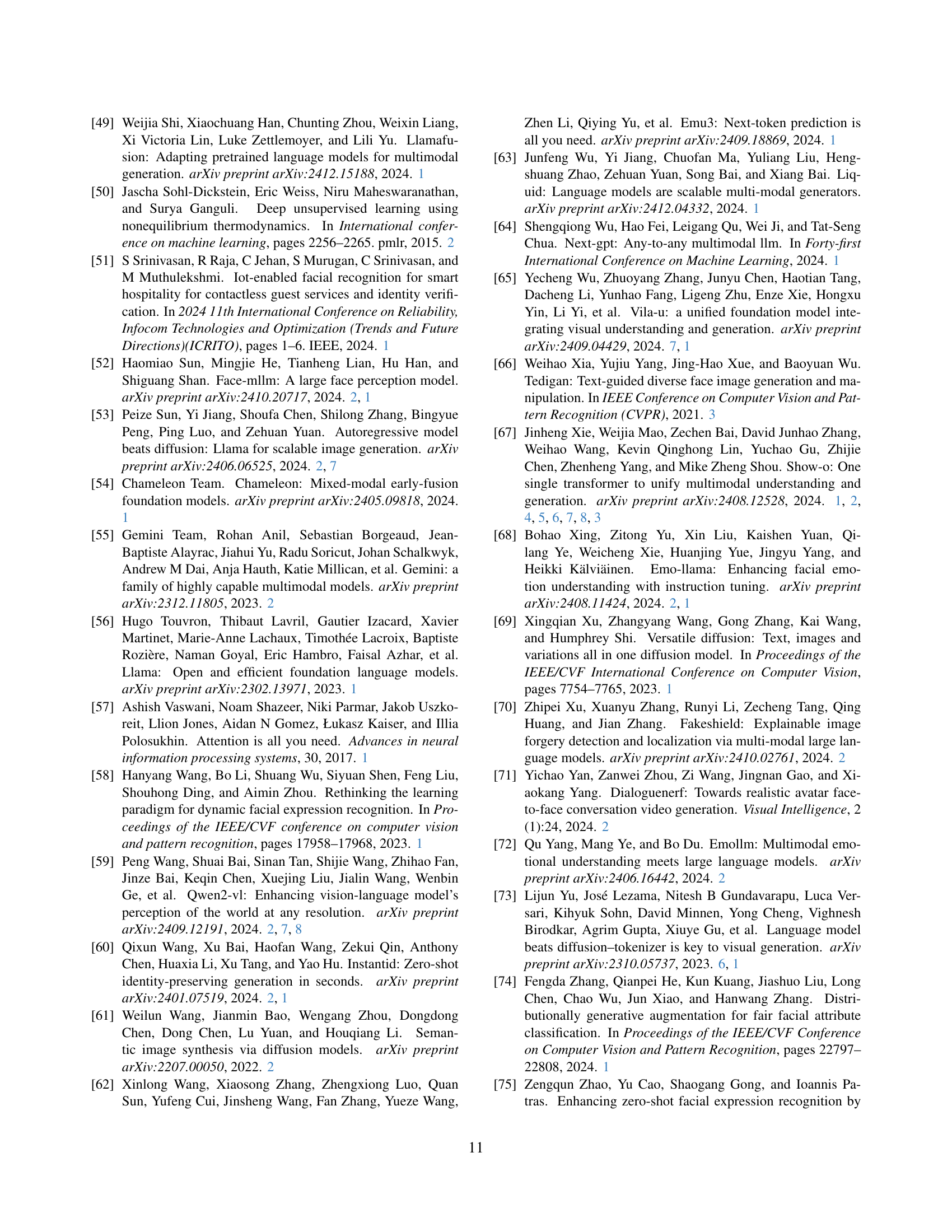
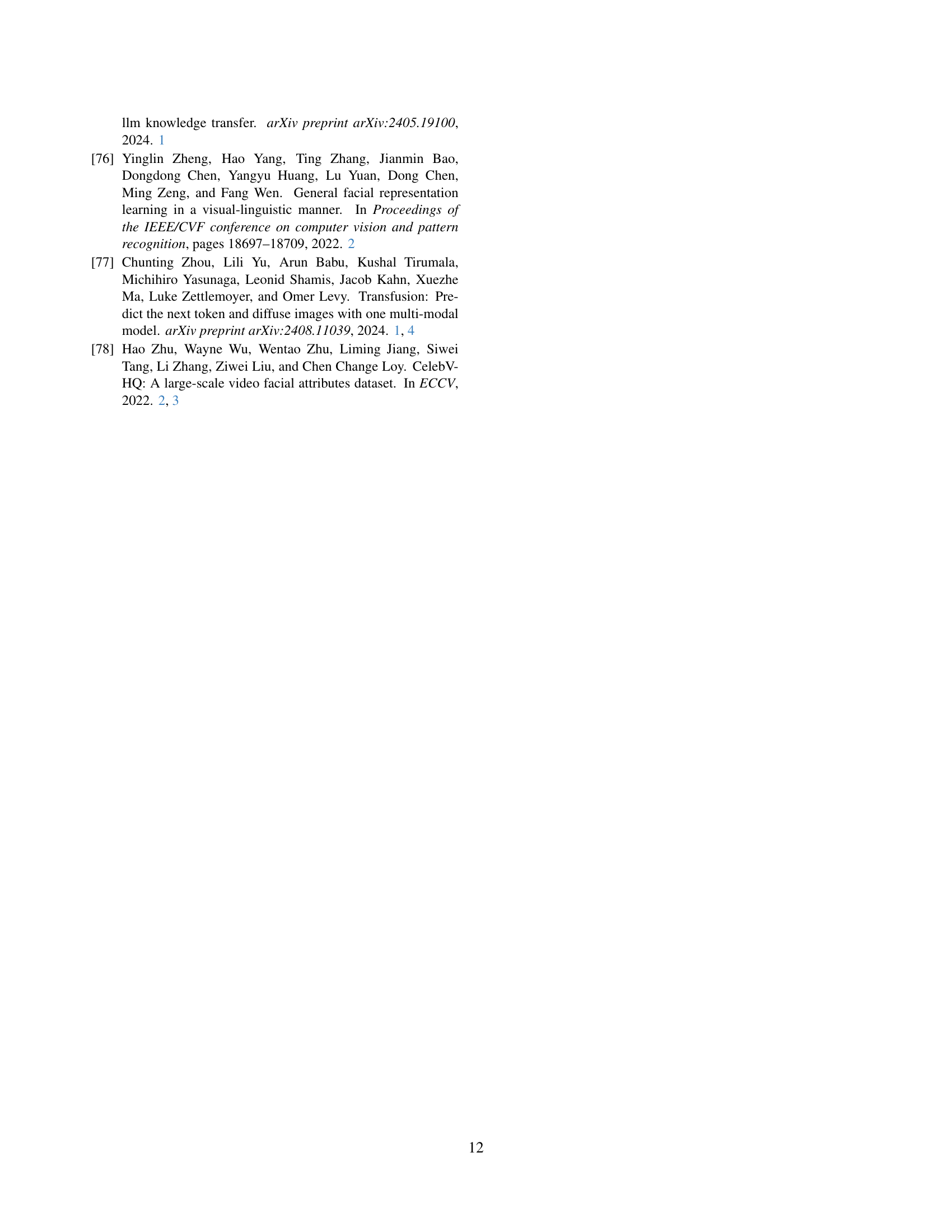
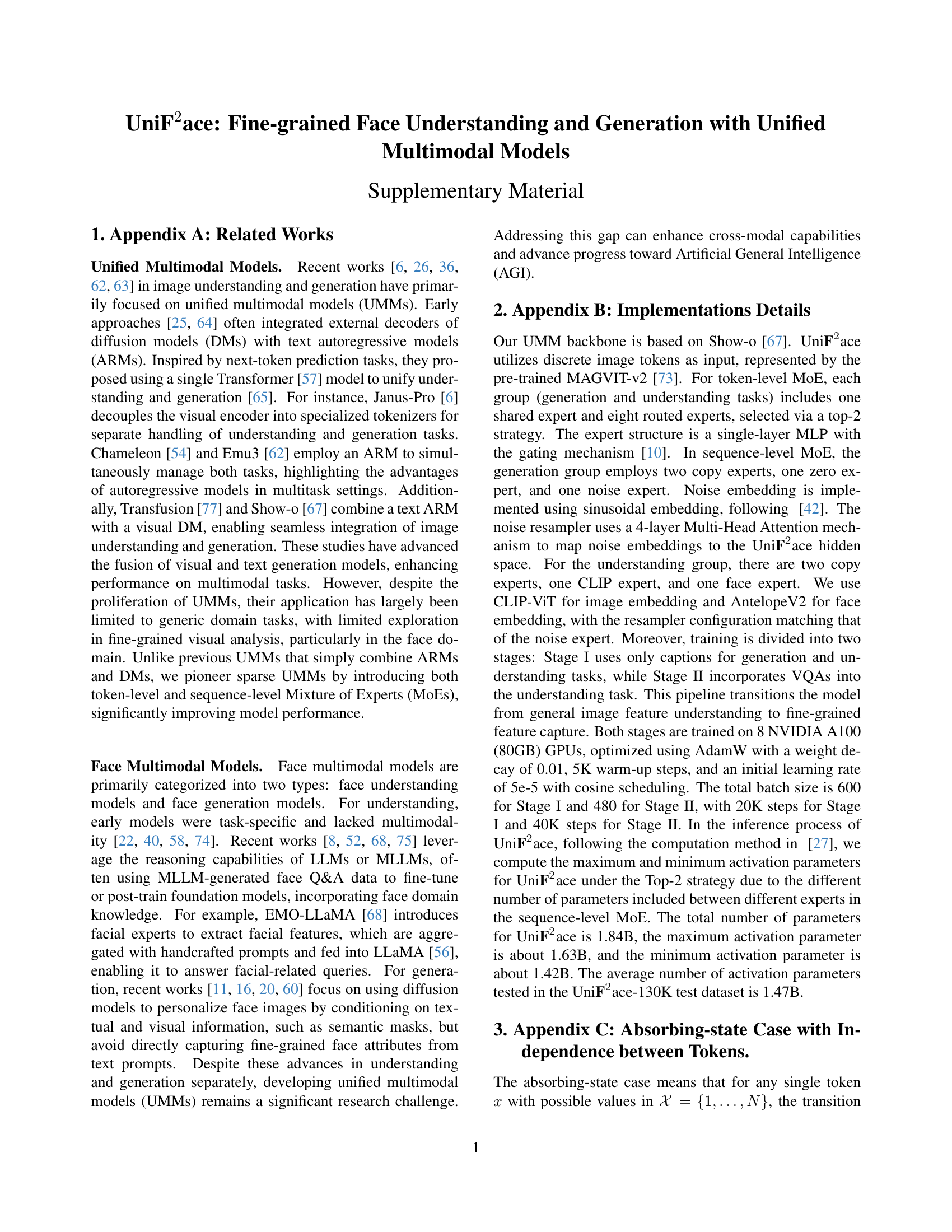
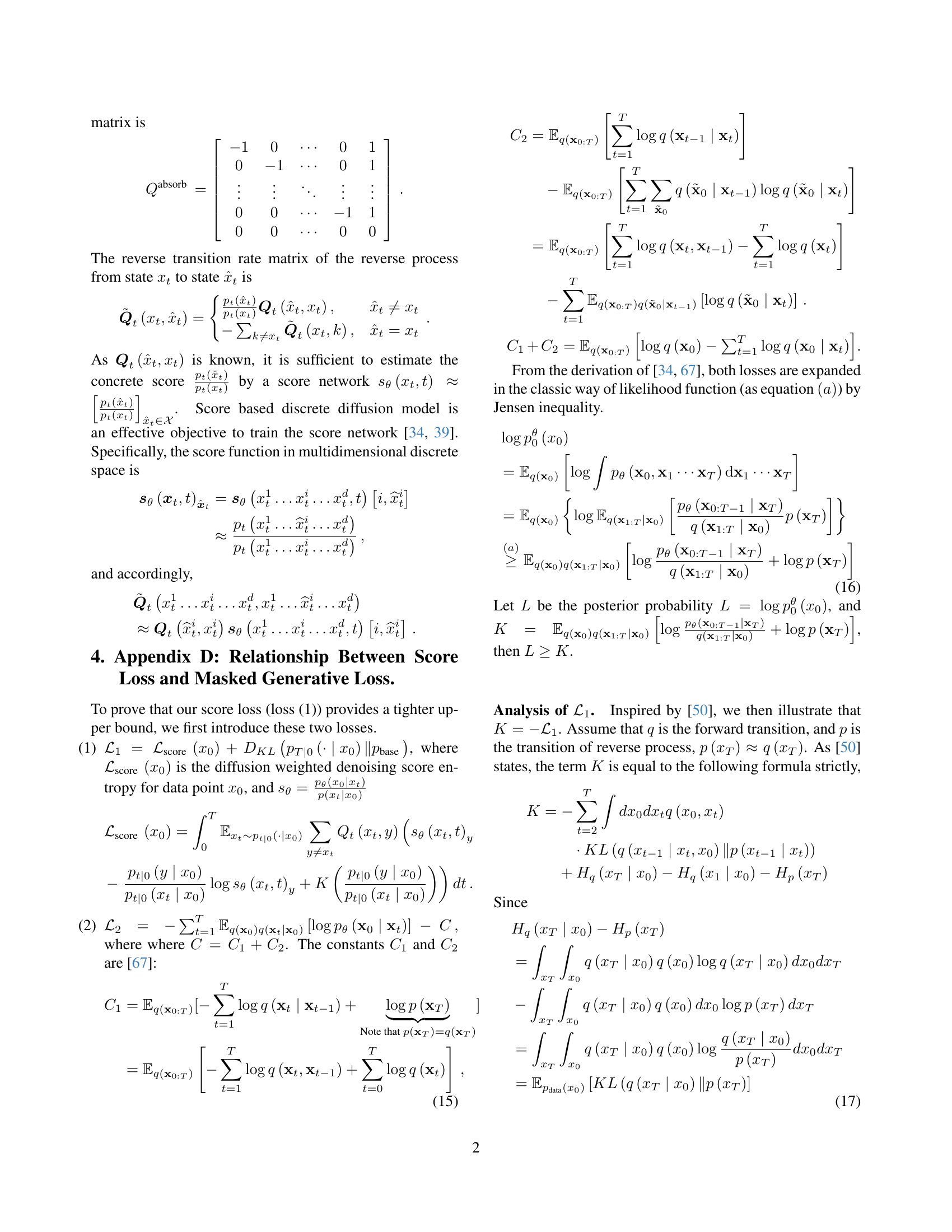
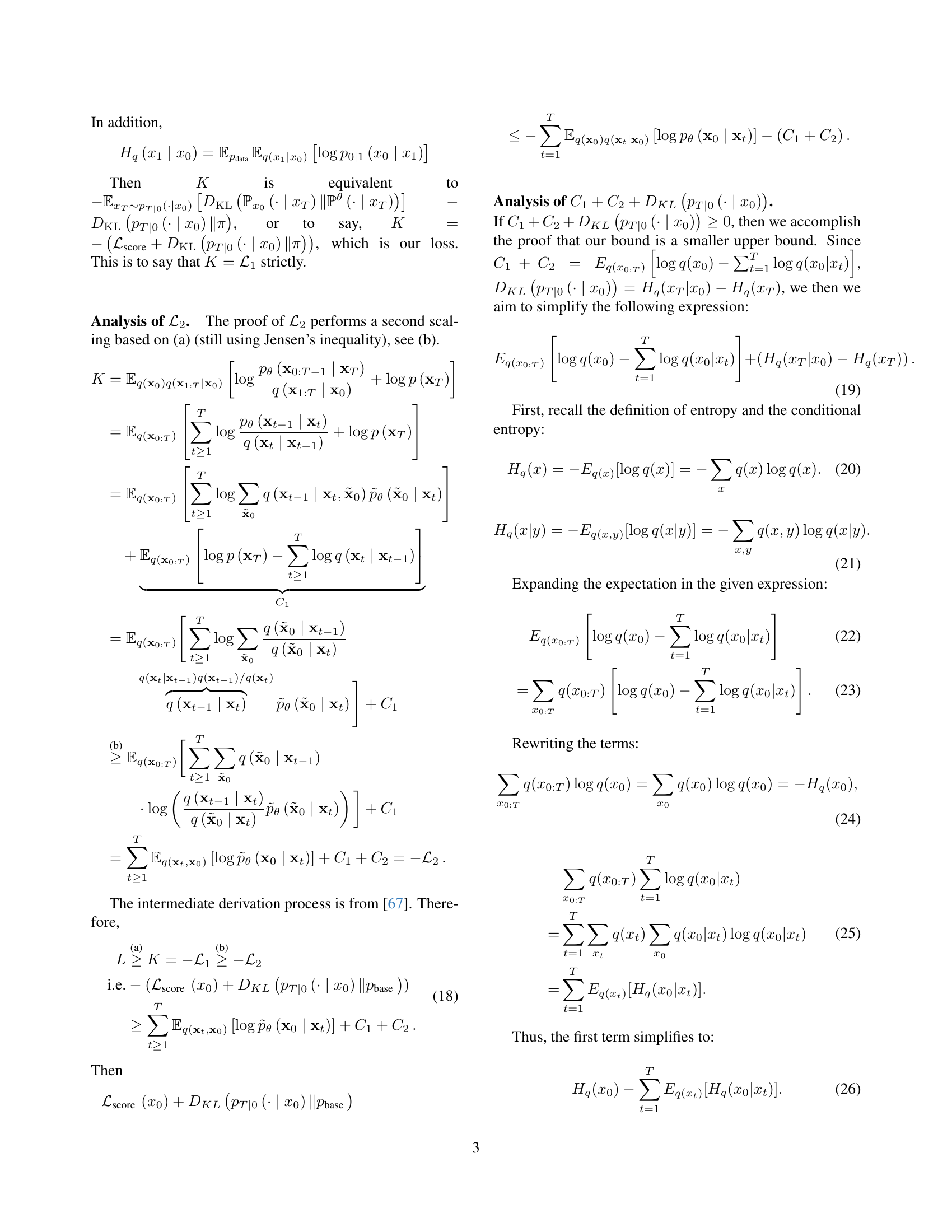
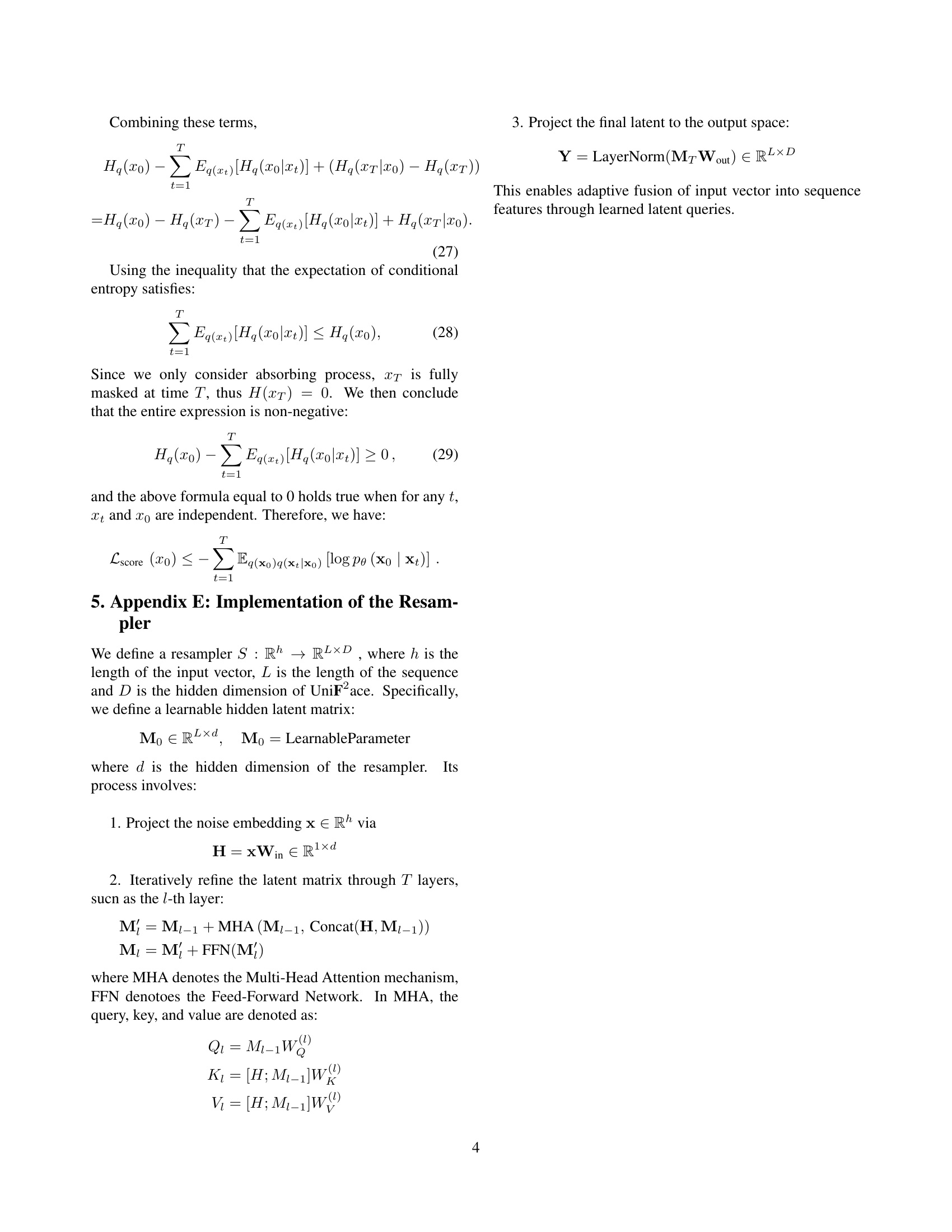
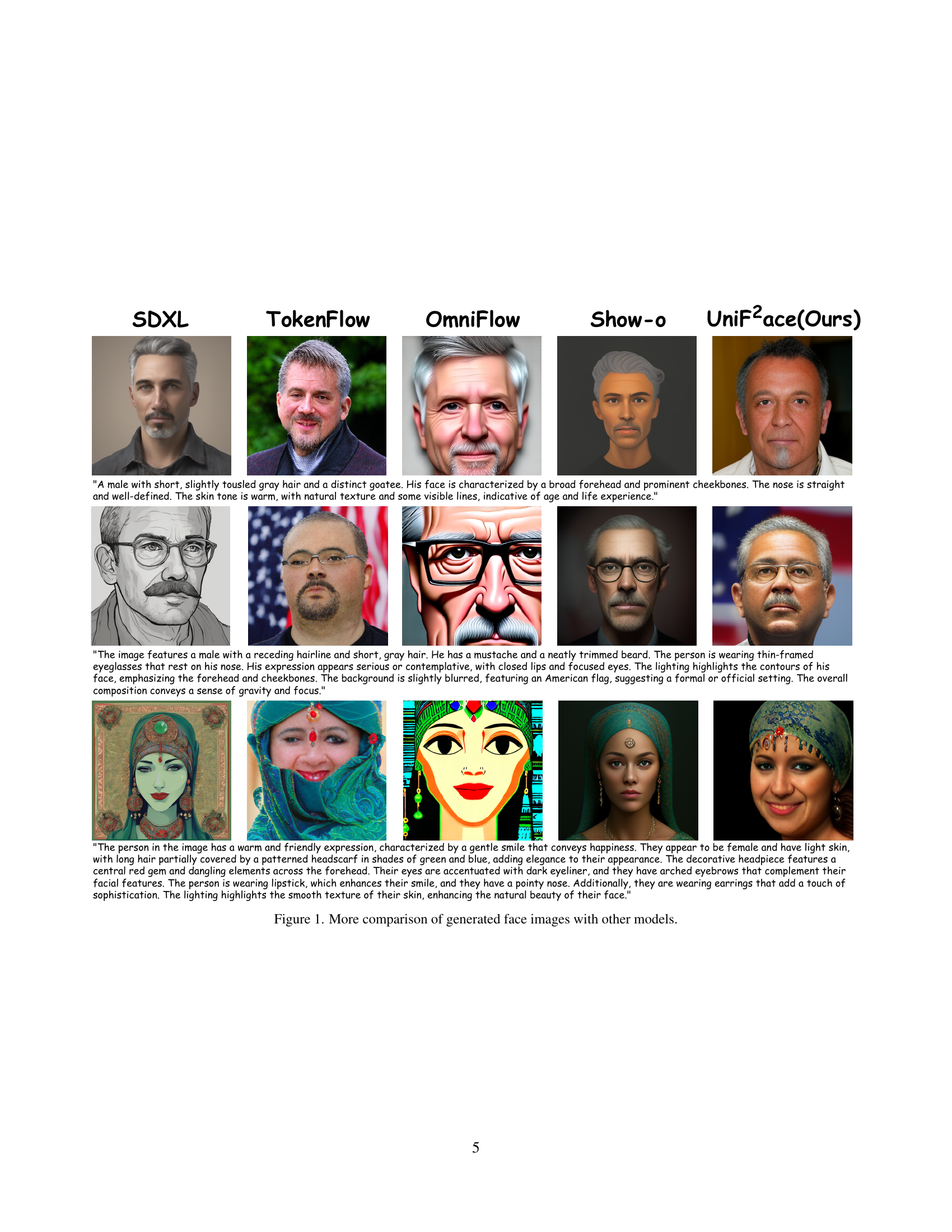
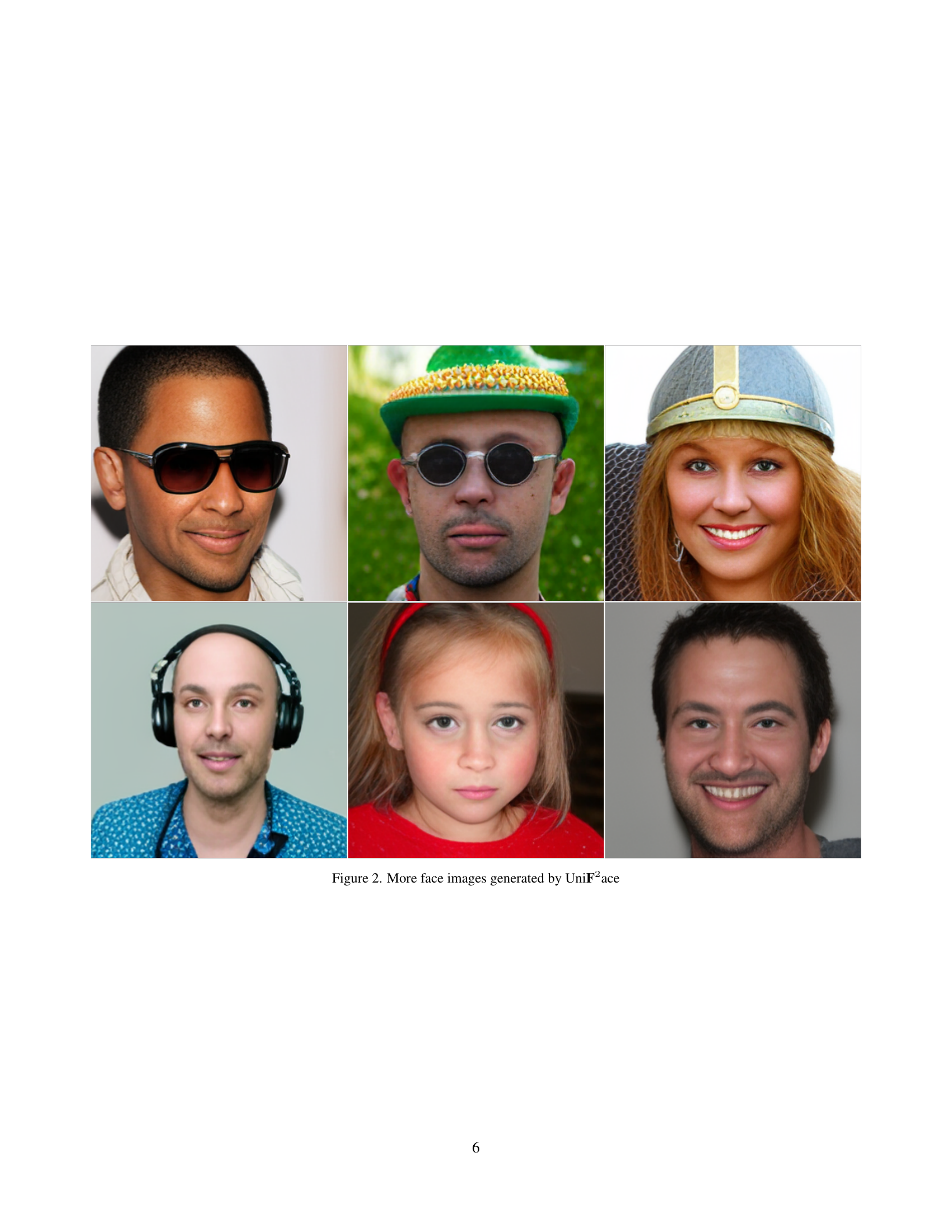
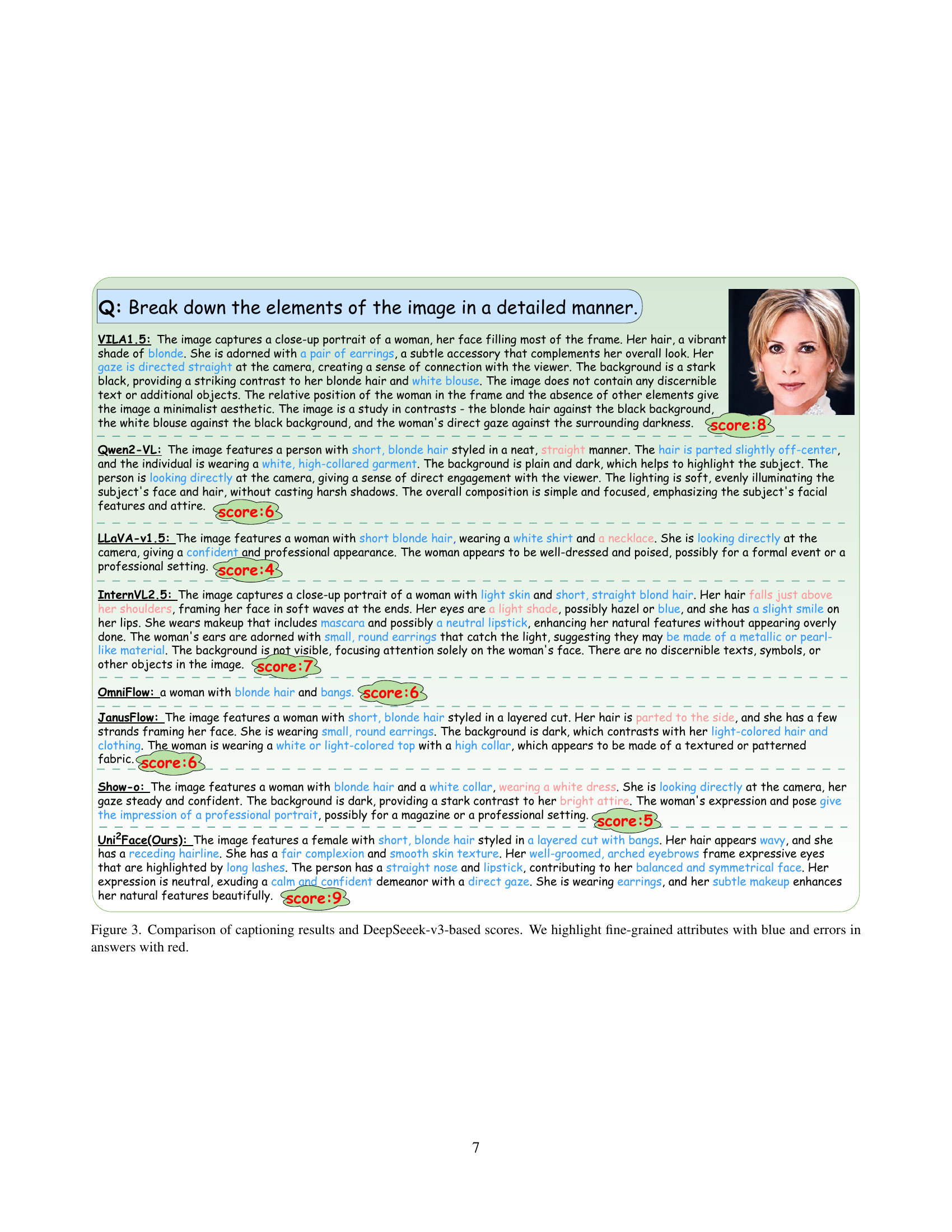
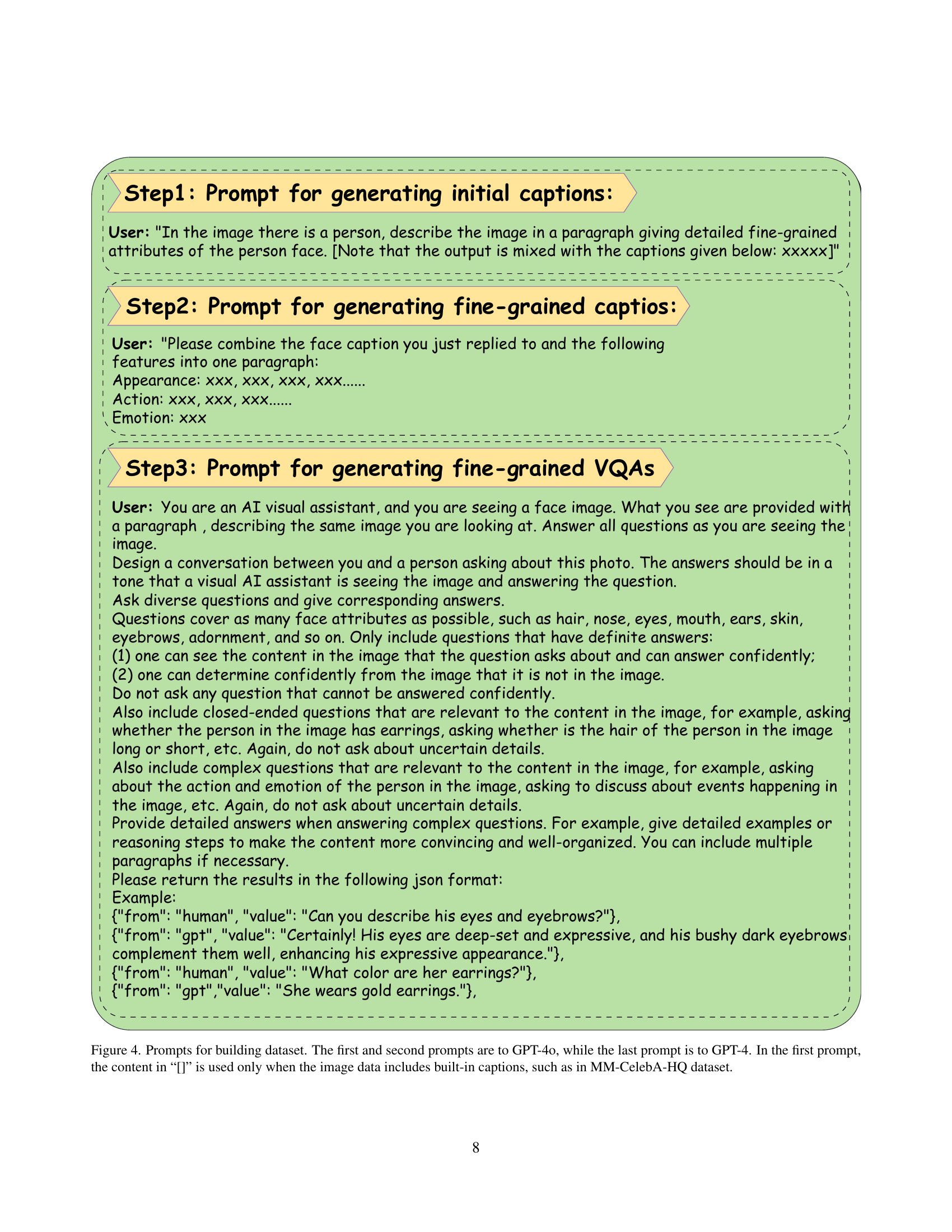