TL;DR#
Recent advances in Large Reasoning Models (LRMs) are opening brand new possibility for Machine Translation (MT), transforming traditional paradigms. This reframes translation as a dynamic reasoning task requiring understanding of context, culture, and linguistic nuances. The paper identifies foundational shifts such as contextual coherence, cultural intentionality, and self-reflection. Unlike traditional NMT systems, LRMs excel in zero-shot and few-shot translation scenarios. They show remarkable versatility in style transfer, summarization, and question answering opening avenues for MT research.
LRMs tackled stylized and document-level translation by showcasing empirical examples that demonstrate their superiority in translation. It identifies interesting phenomenons including auto-pivot translation and critical challenges like over-localisation and inference efficiency. The research suggests that LRMs can redefine translation systems as multilingual cognitive agents, capable of reasoning about meaning beyond the text. This shift broadens the scope of translation problems in a much wider context.
Key Takeaways#
Why does it matter?#
This paper is important for researchers as it redefines Machine Translation (MT) with Large Reasoning Models, highlighting their ability to understand context and cultural nuances. It opens new research avenues in areas like stylized and multi-modal translation.
Visual Insights#
πΌ This figure illustrates the promising applications of Large Reasoning Models (LRMs) in machine translation (MT). It showcases how LRMs address traditional MT challenges (stylized translation, document translation, multimodal translation) while also highlighting new opportunities and challenges enabled by LRMs. Specifically, it emphasizes the significant shift from simple text conversion to a more dynamic reasoning task that includes contextual coherence, cultural intentionality, and self-reflection. The figure uses DeepSeek R1 as a representative example of such LRMs. The new challenges include self-reflection and auto-pivoting.
read the caption
Figure 1: Promsing directions for MT using LRMs (e.g., DeepSeek R1), including some foundational and classical MT scenarios such as stylized translation, new challenges with LRMs like self-reflection, and some new challenges for LRMs.
Method | Lexical | Contextless | Contextual | |||
---|---|---|---|---|---|---|
COMET | BLEURT | COMET | BLEURT | COMET | BLEURT | |
DeepSeek-R1 | 84.3 | 73.9 | 84.7 | 73.9 | 84.0 | 73.3 |
DeepSeek-V3 | 84.7 | 74.2 | 84.4 | 74.1 | 84.1 | 73.2 |
QwQ-32B | 84.1 | 73.0 | 84.0 | 72.8 | 84.1 | 72.9 |
GPT-4o | 84.8 | 74.1 | 84.6 | 73.7 | 85.0 | 74.9 |
πΌ This table presents the results of an experiment evaluating the performance of four different large language models (LLMs) on a commonsense translation task using the CommonMT benchmark dataset [He et al., 2020]. The models are assessed using two automated metrics: COMET (for evaluating the overall quality and coherence of the translation) and BLEURT (for assessing the fluency and grammatical correctness). The table shows the scores for each model across three conditions: lexical (evaluating translation quality based on individual words), contextless (evaluating translation quality without considering surrounding context), and contextual (evaluating translation quality by considering the surrounding context). This experiment aims to compare the translation abilities of the models with varying degrees of reasoning capabilities.
read the caption
Table 1: Result of commonsense translation performance on commonMT [He etΒ al., 2020].
Full paper#
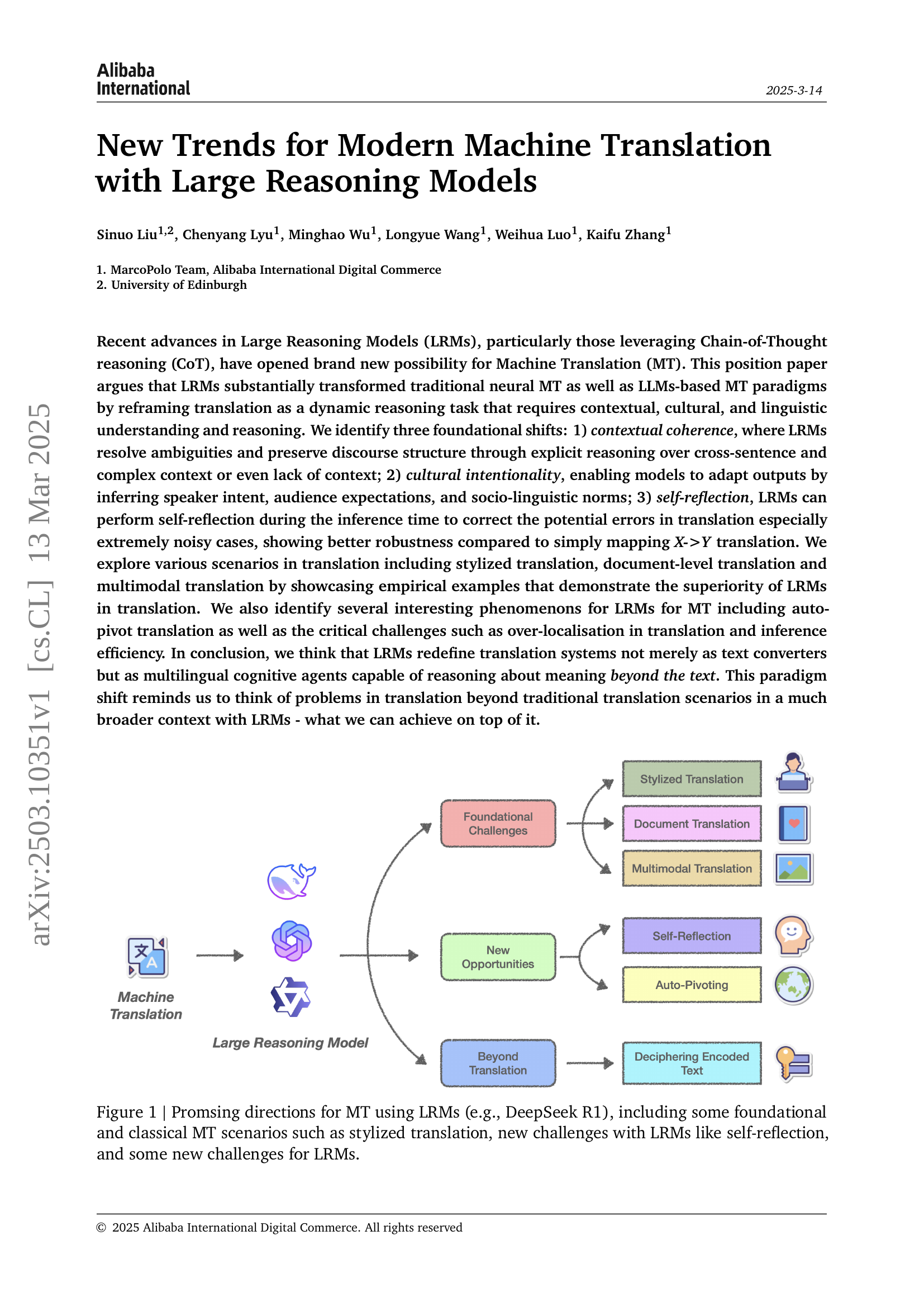
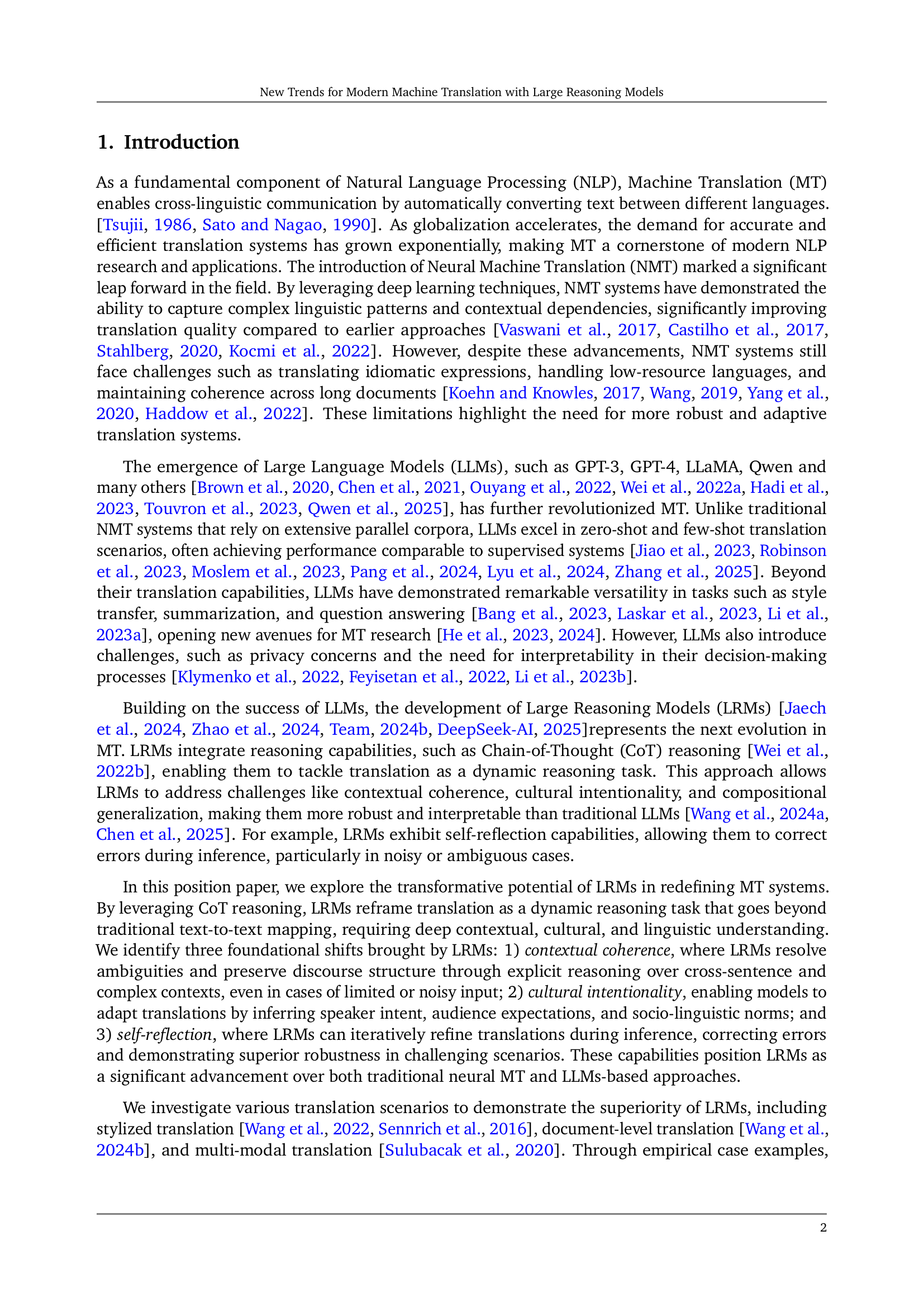
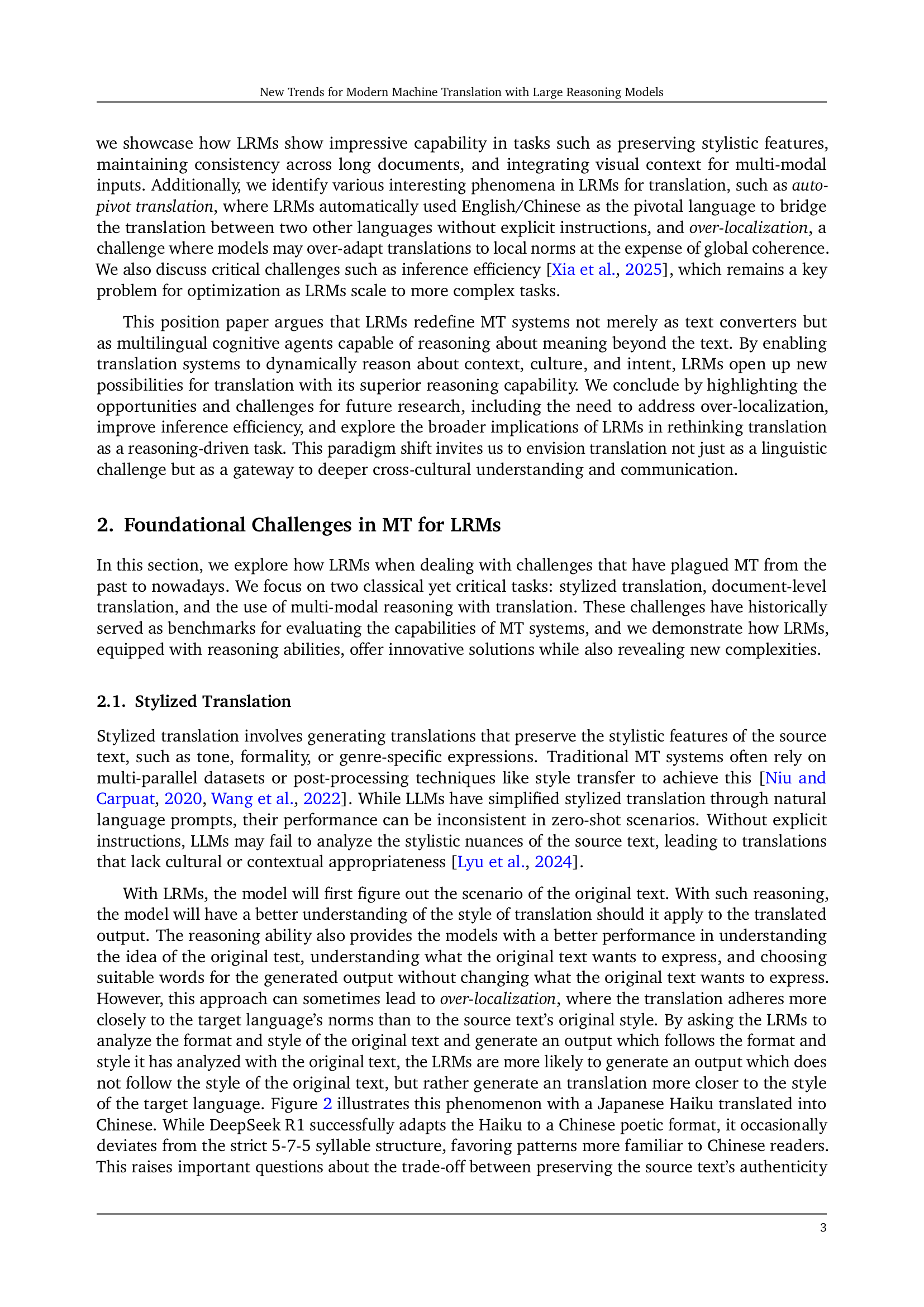
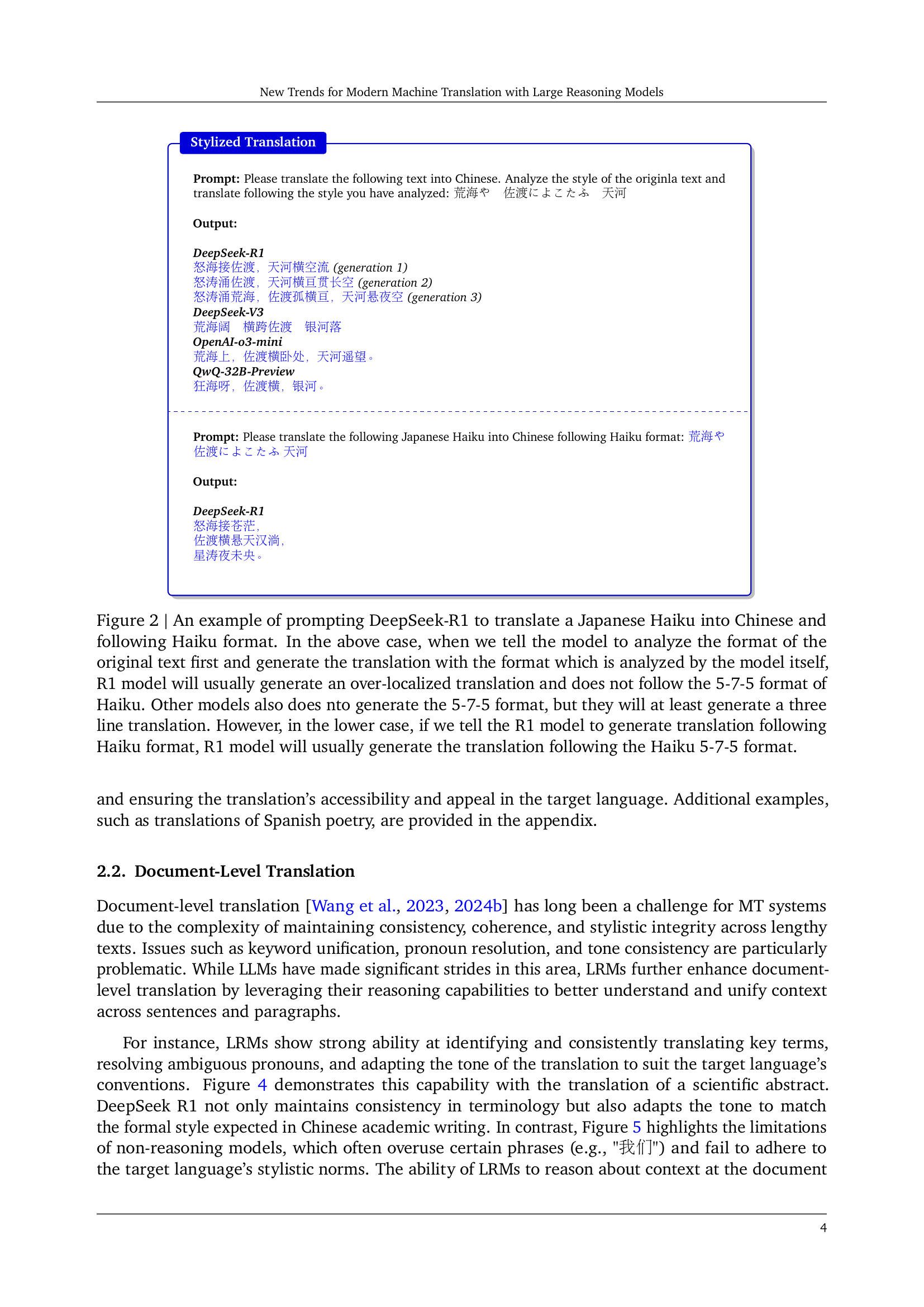
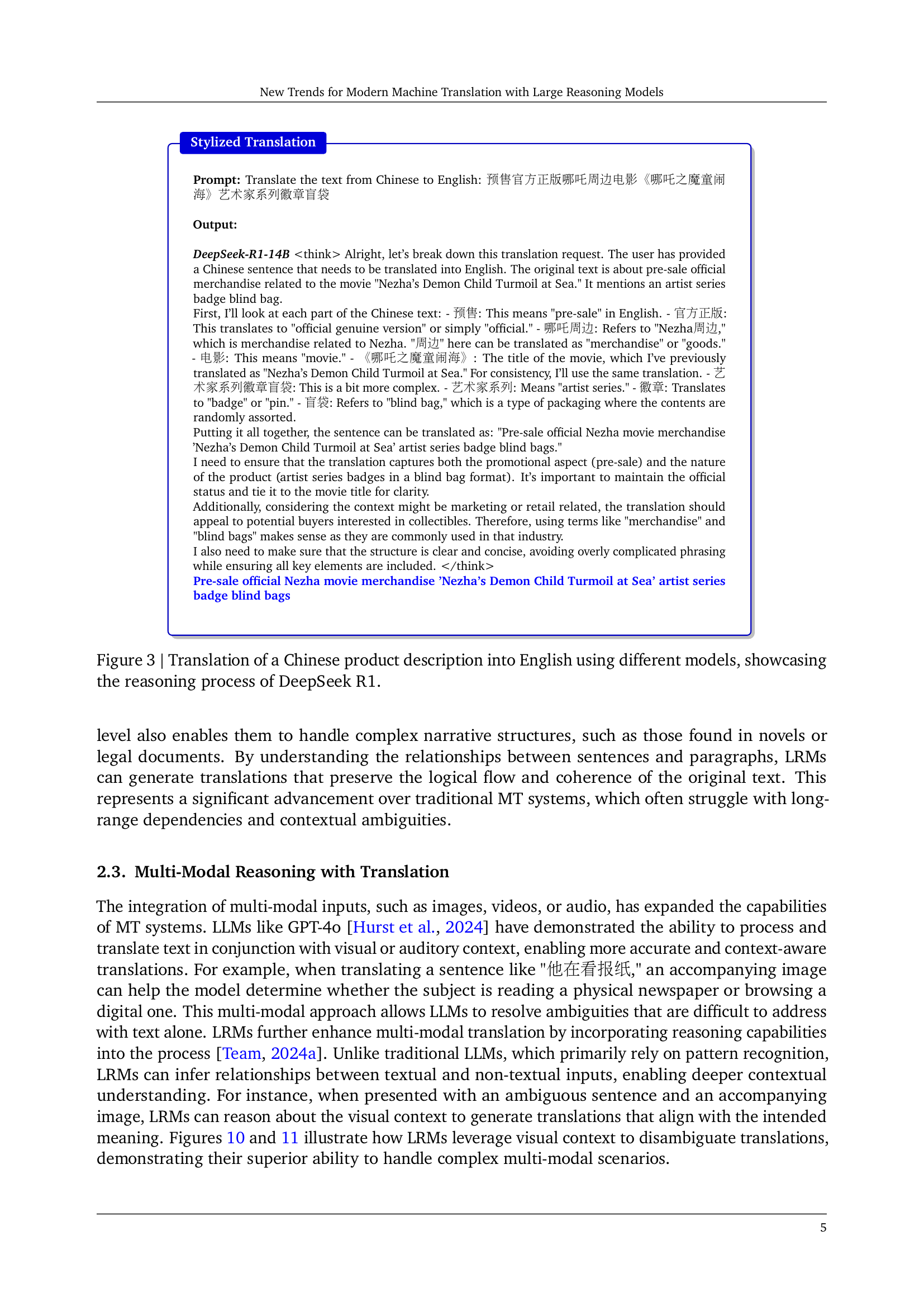
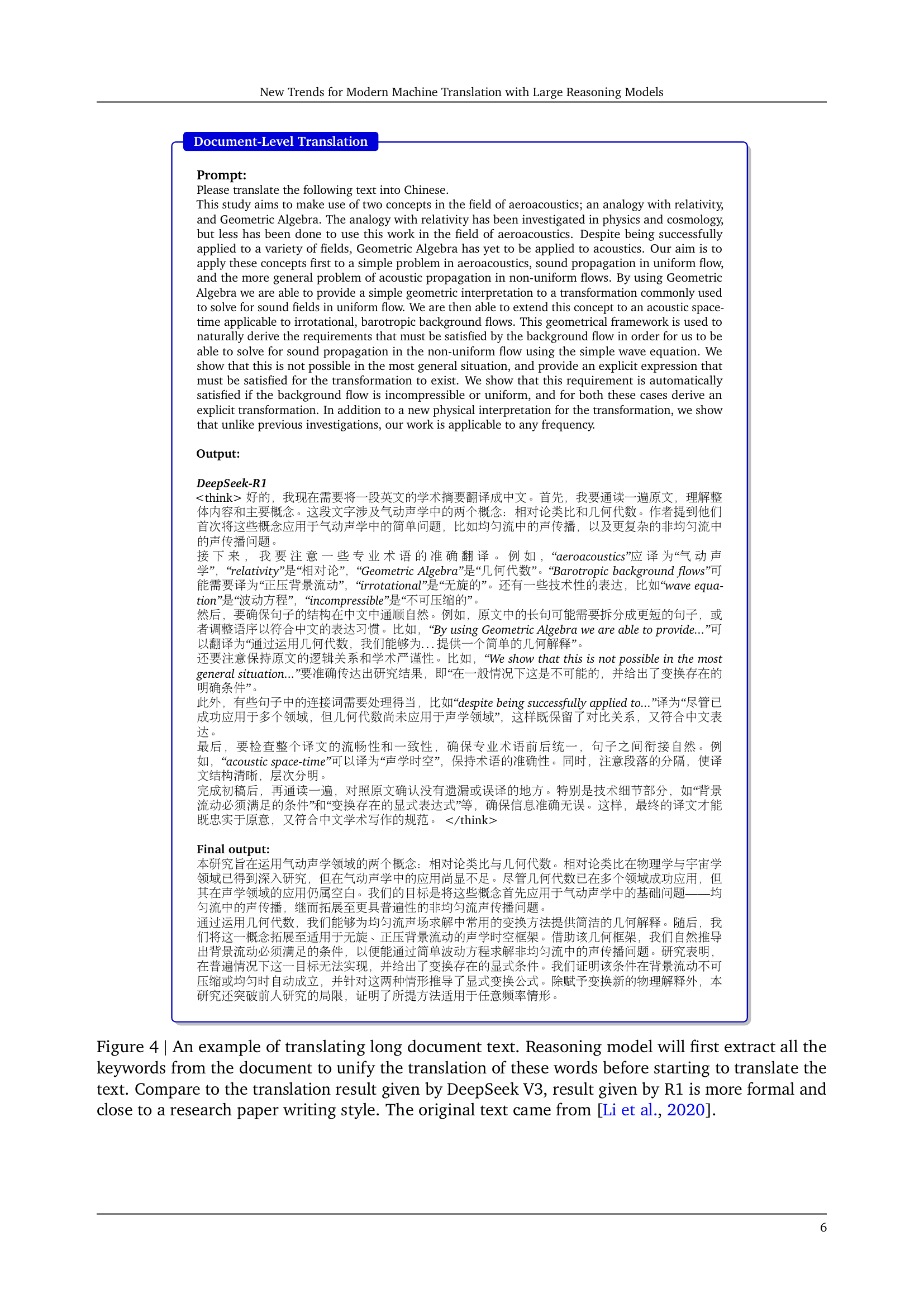
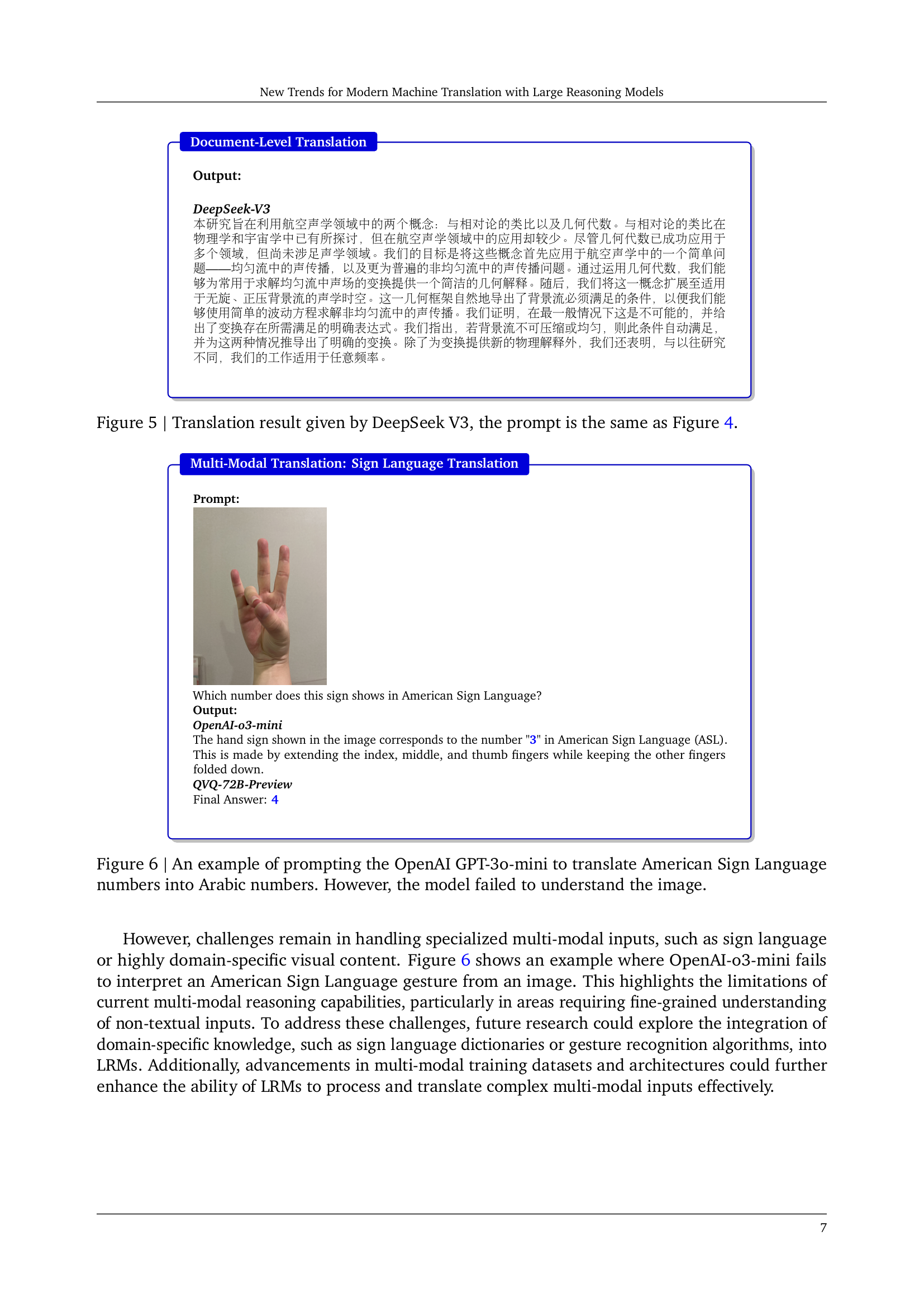
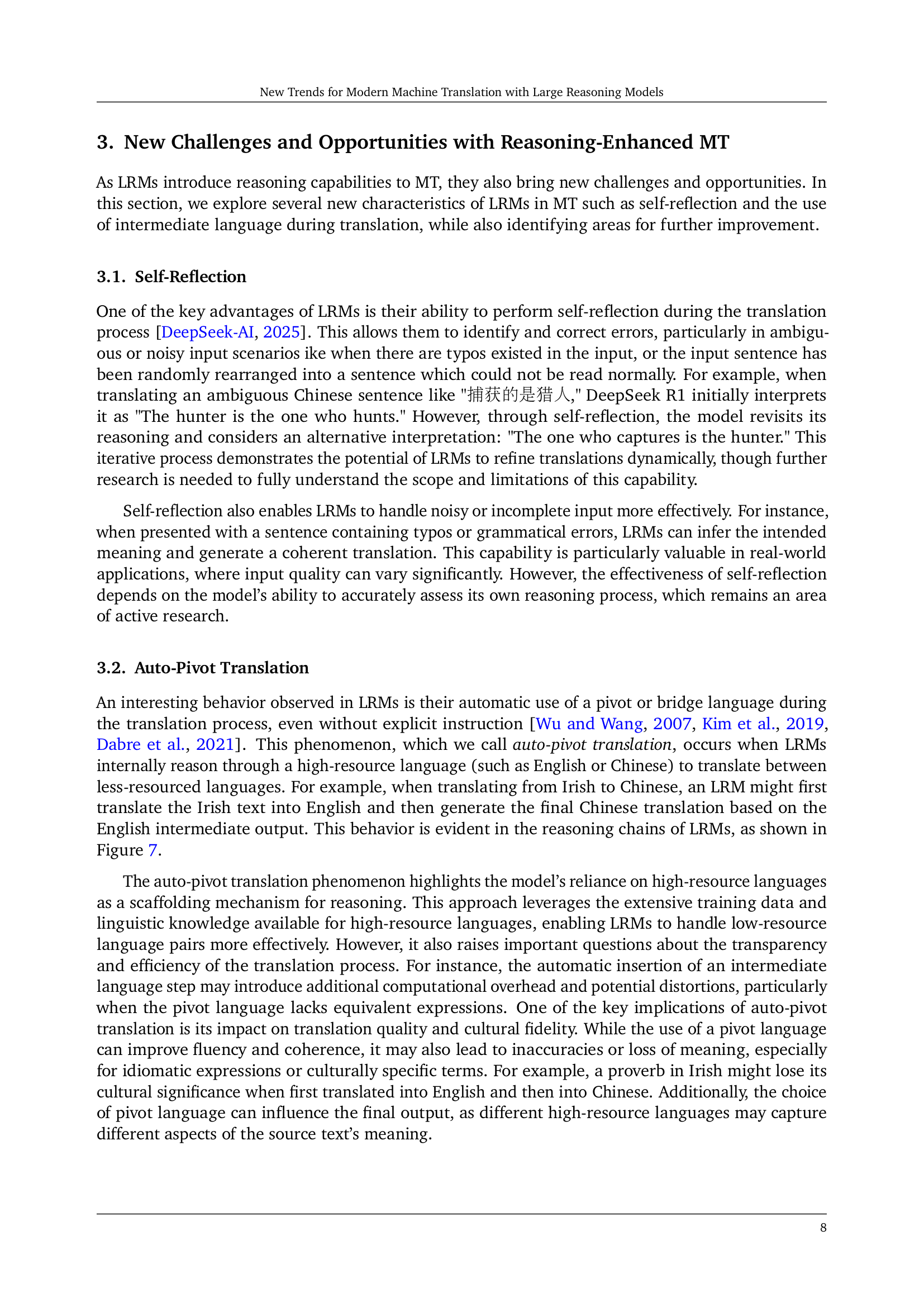
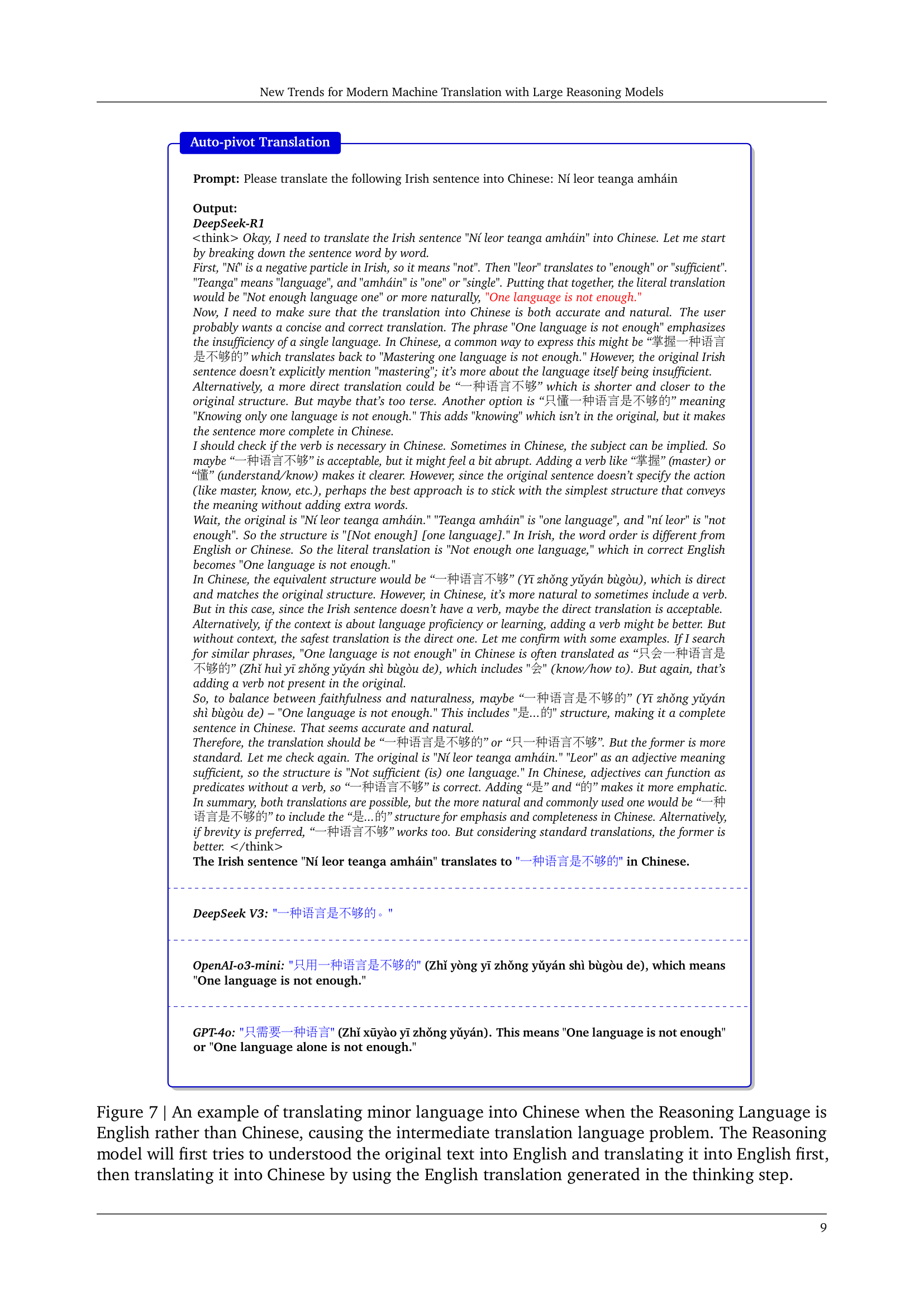
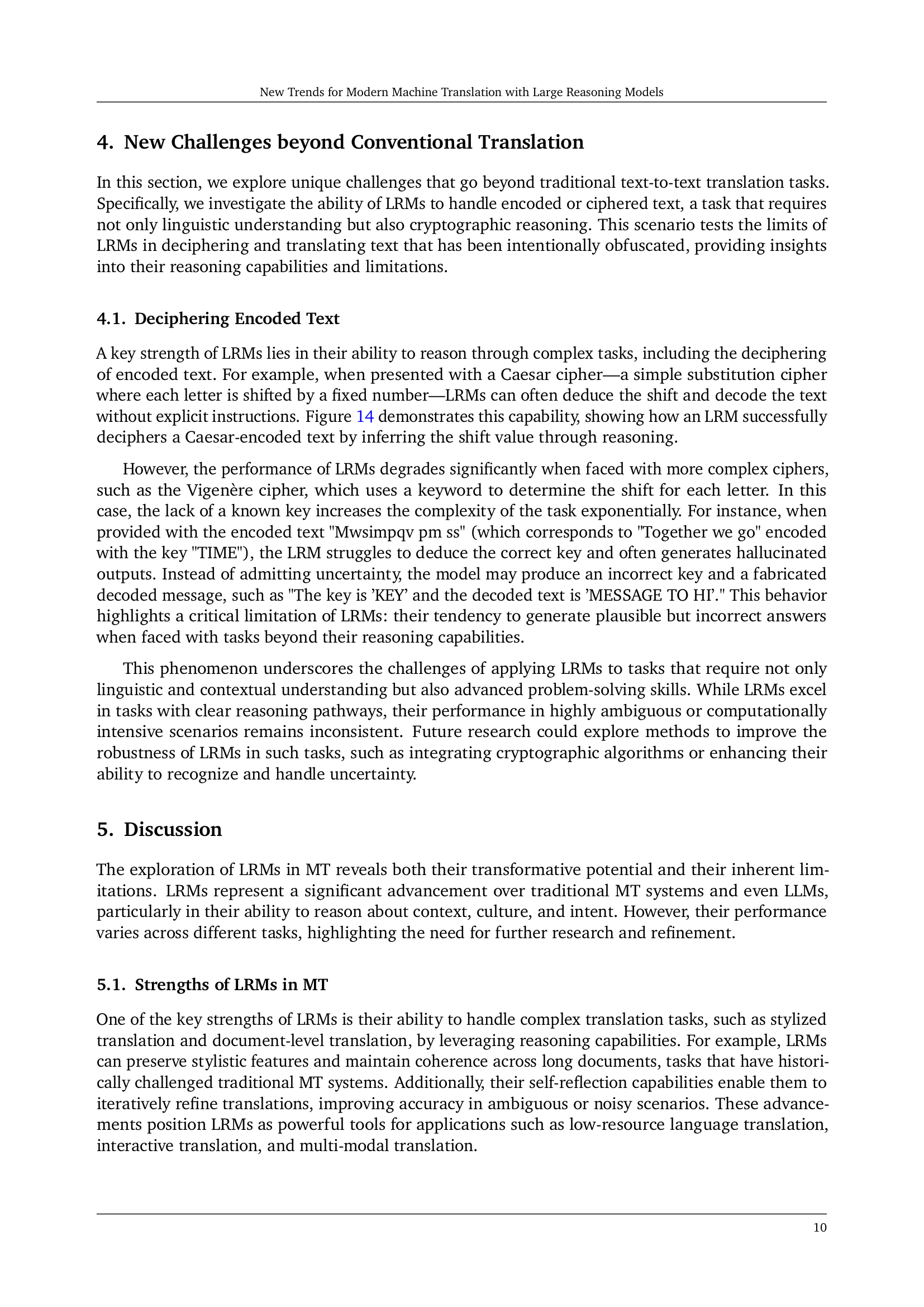
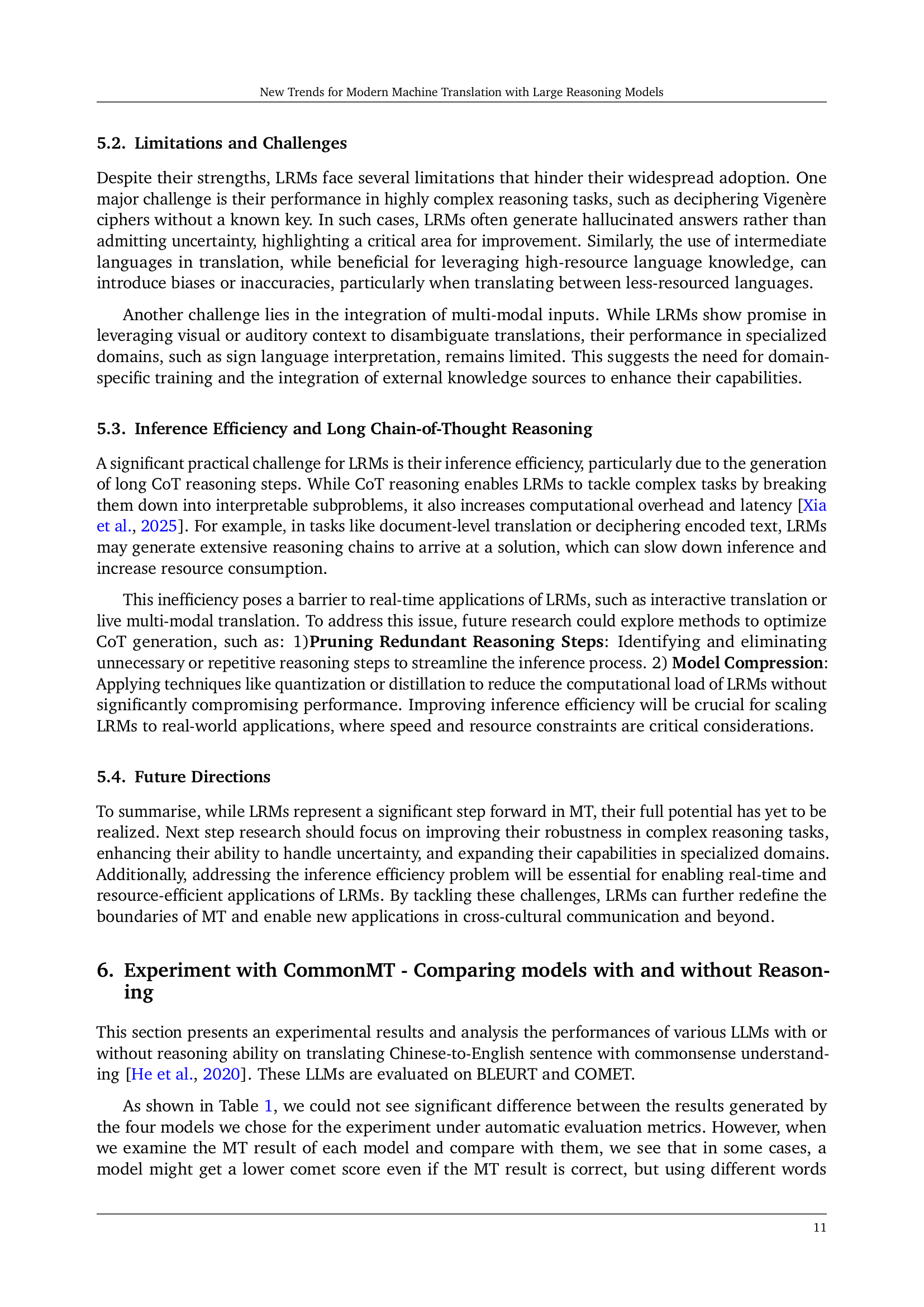
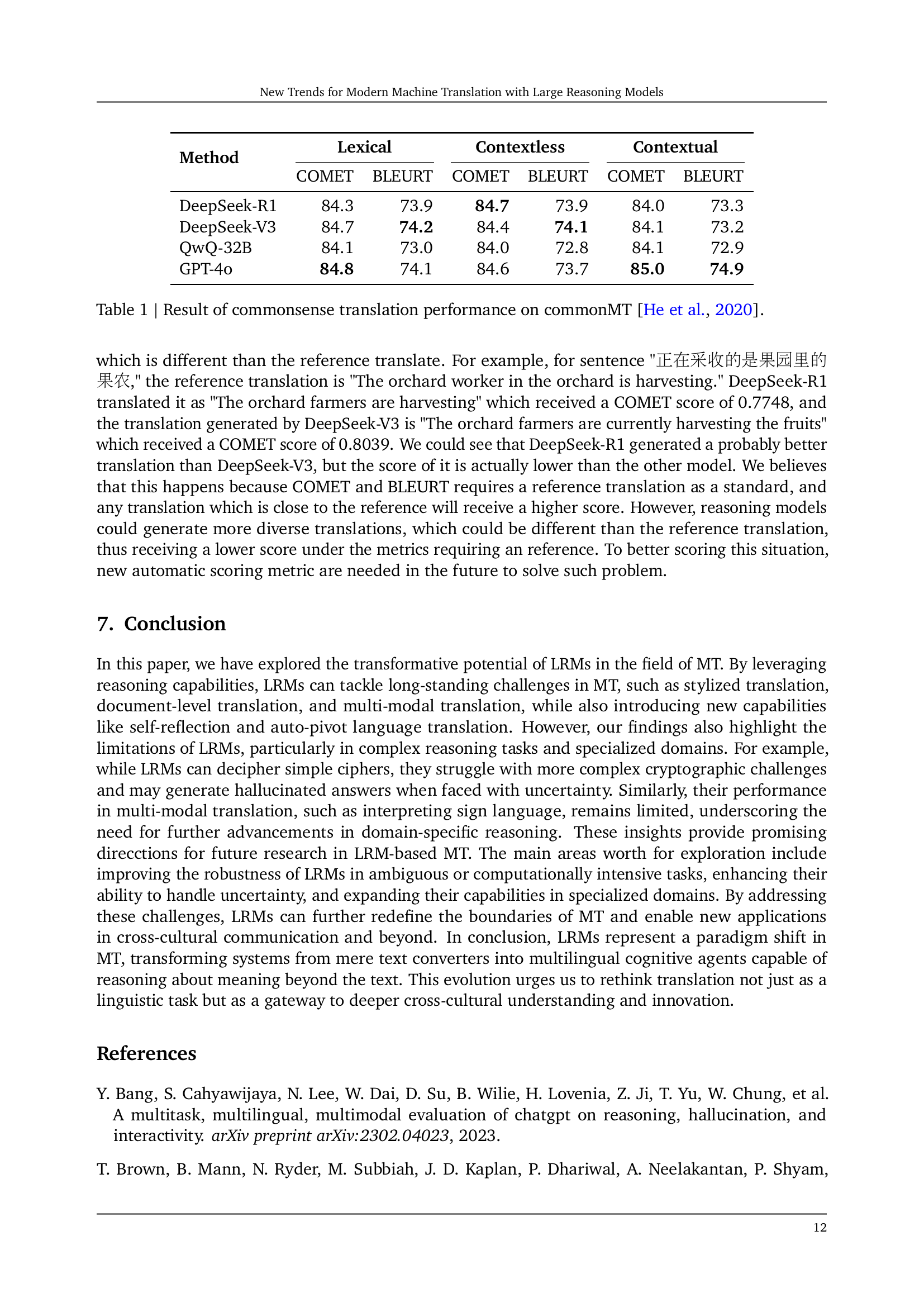
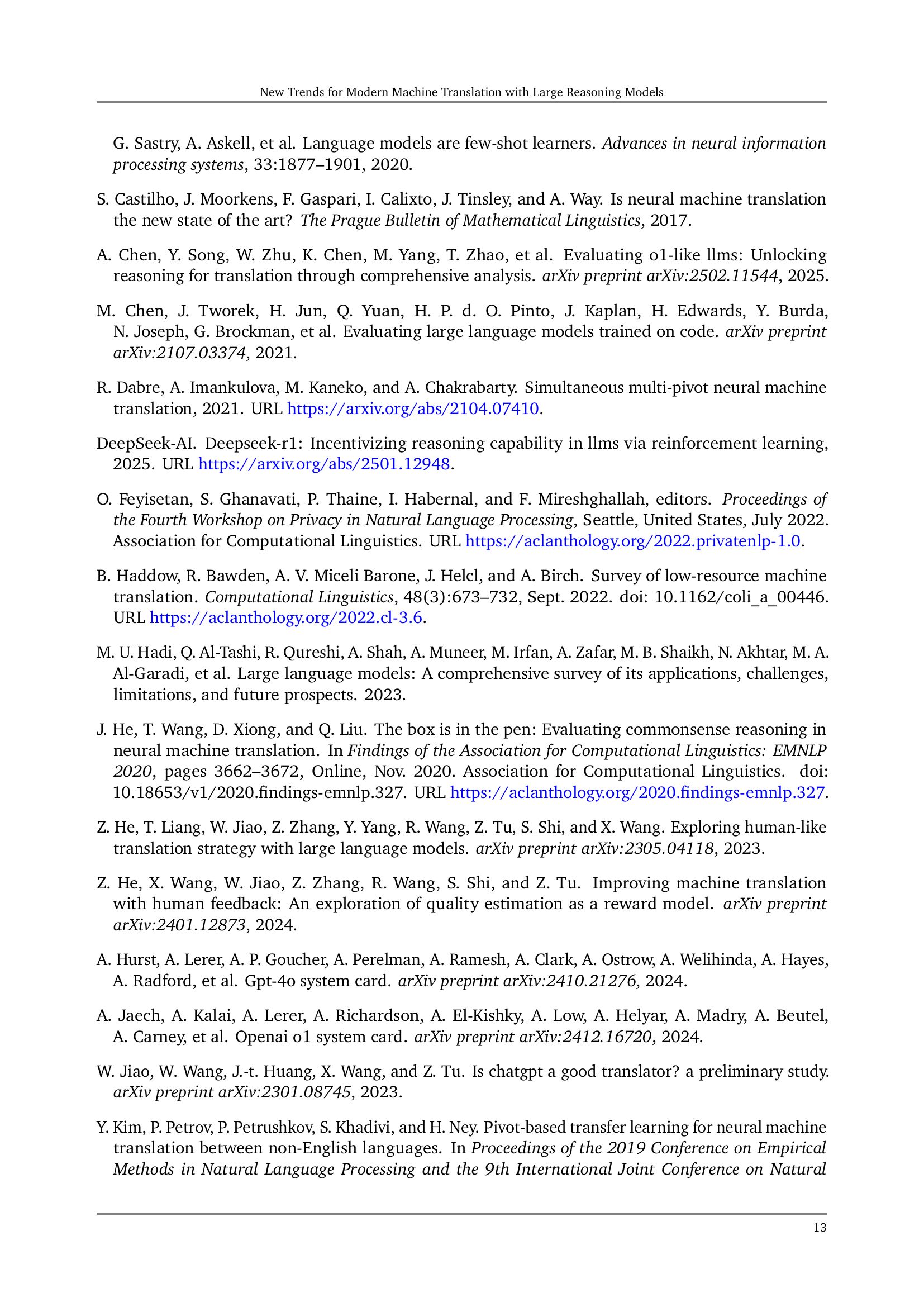
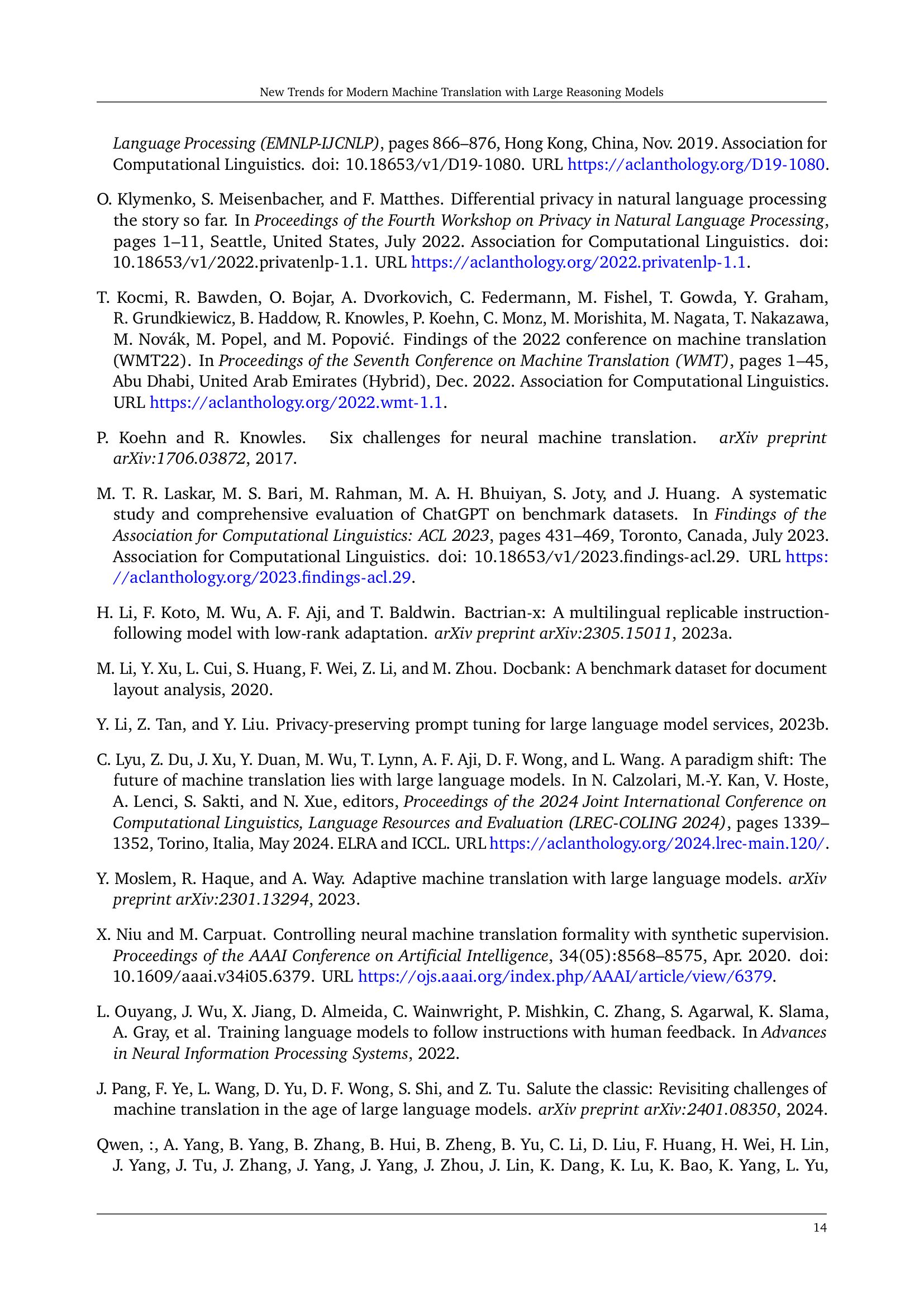
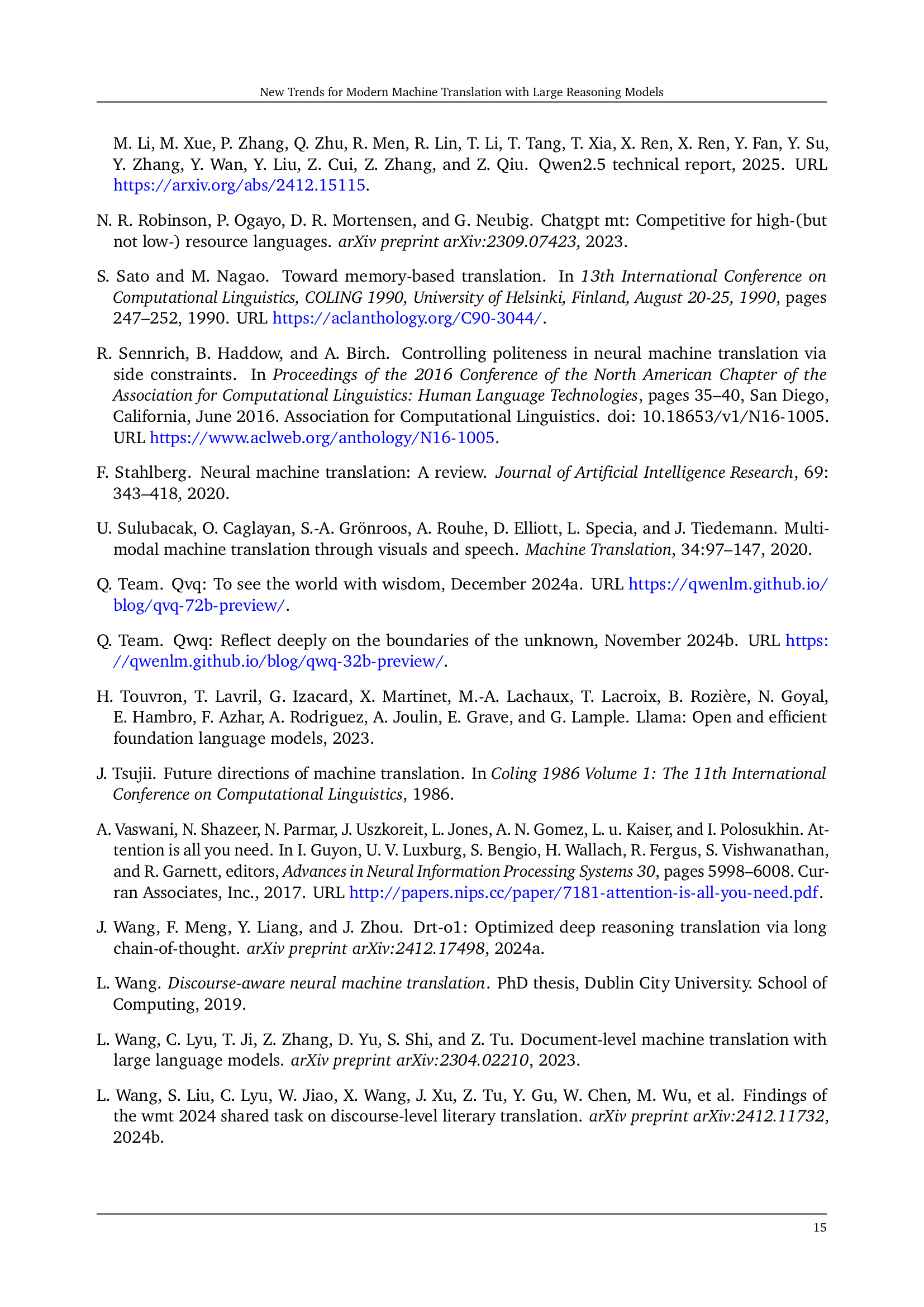
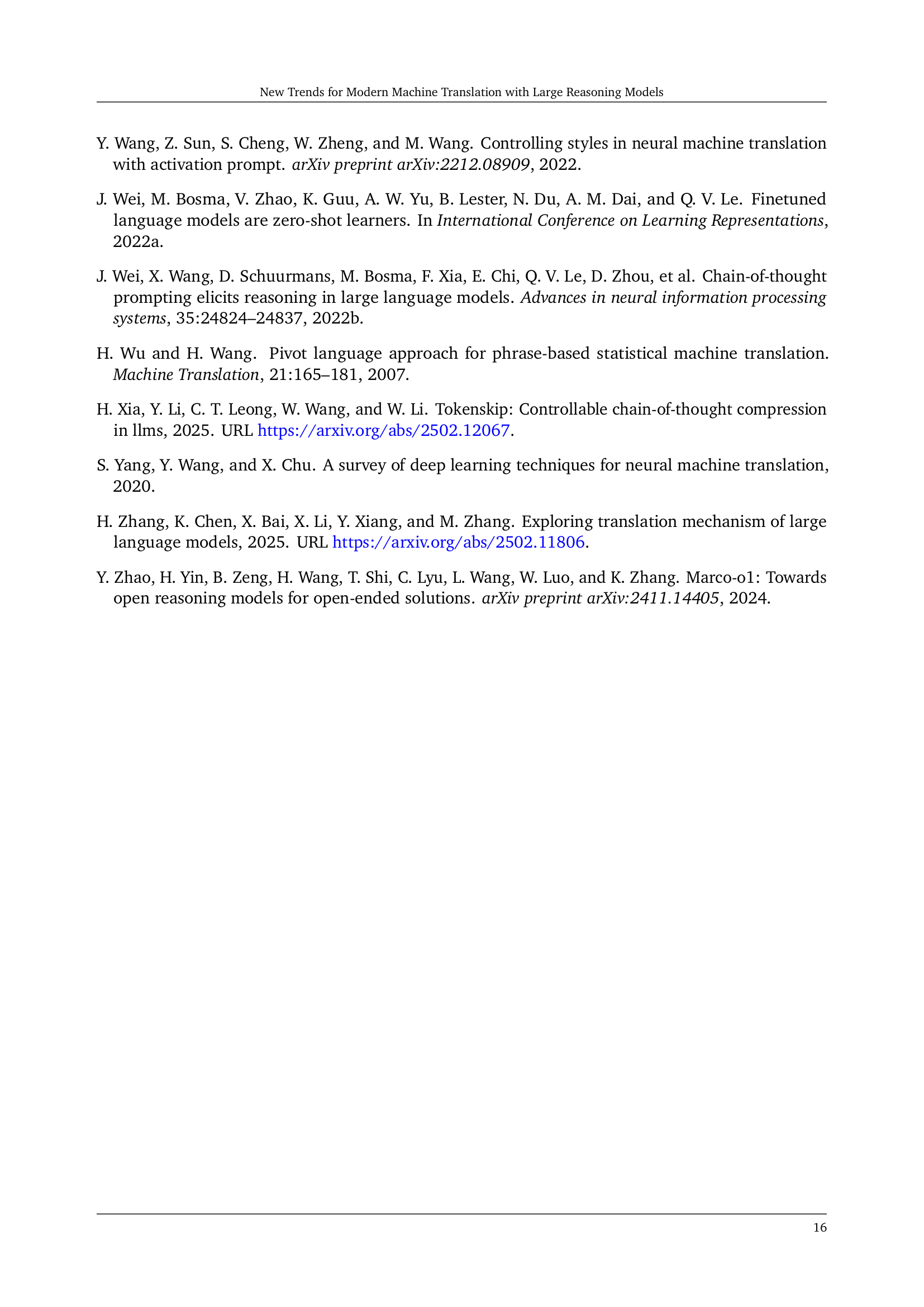
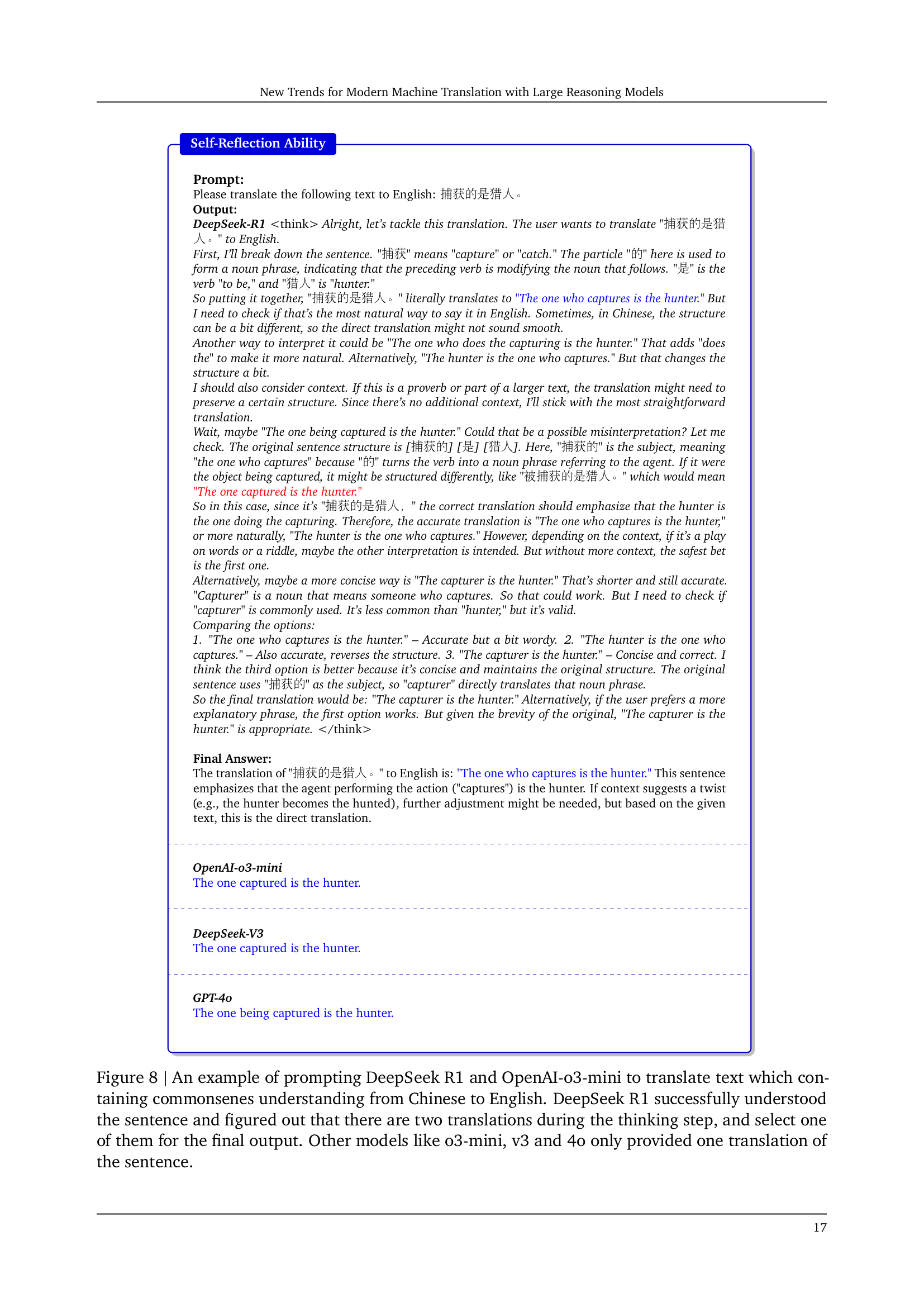
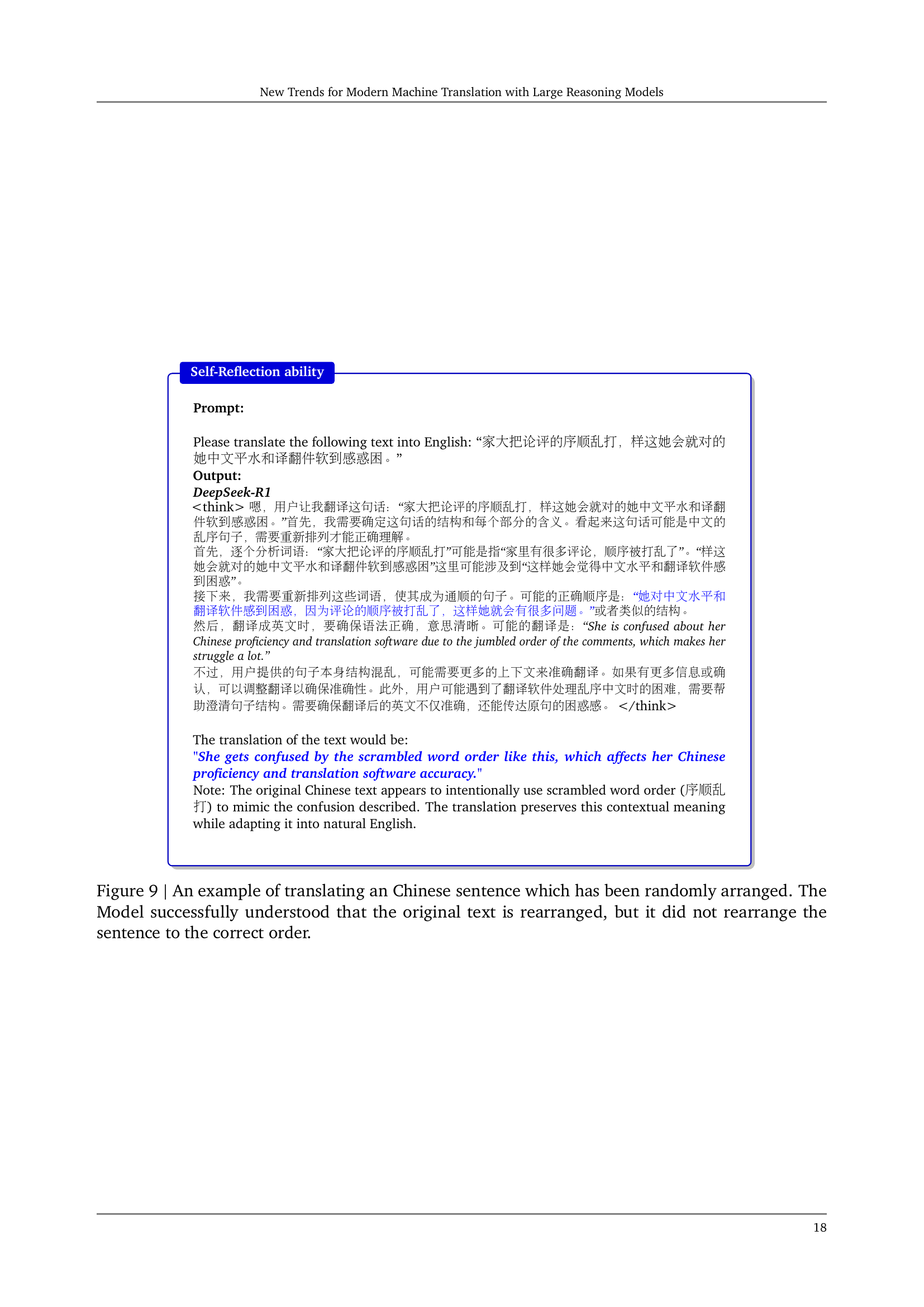
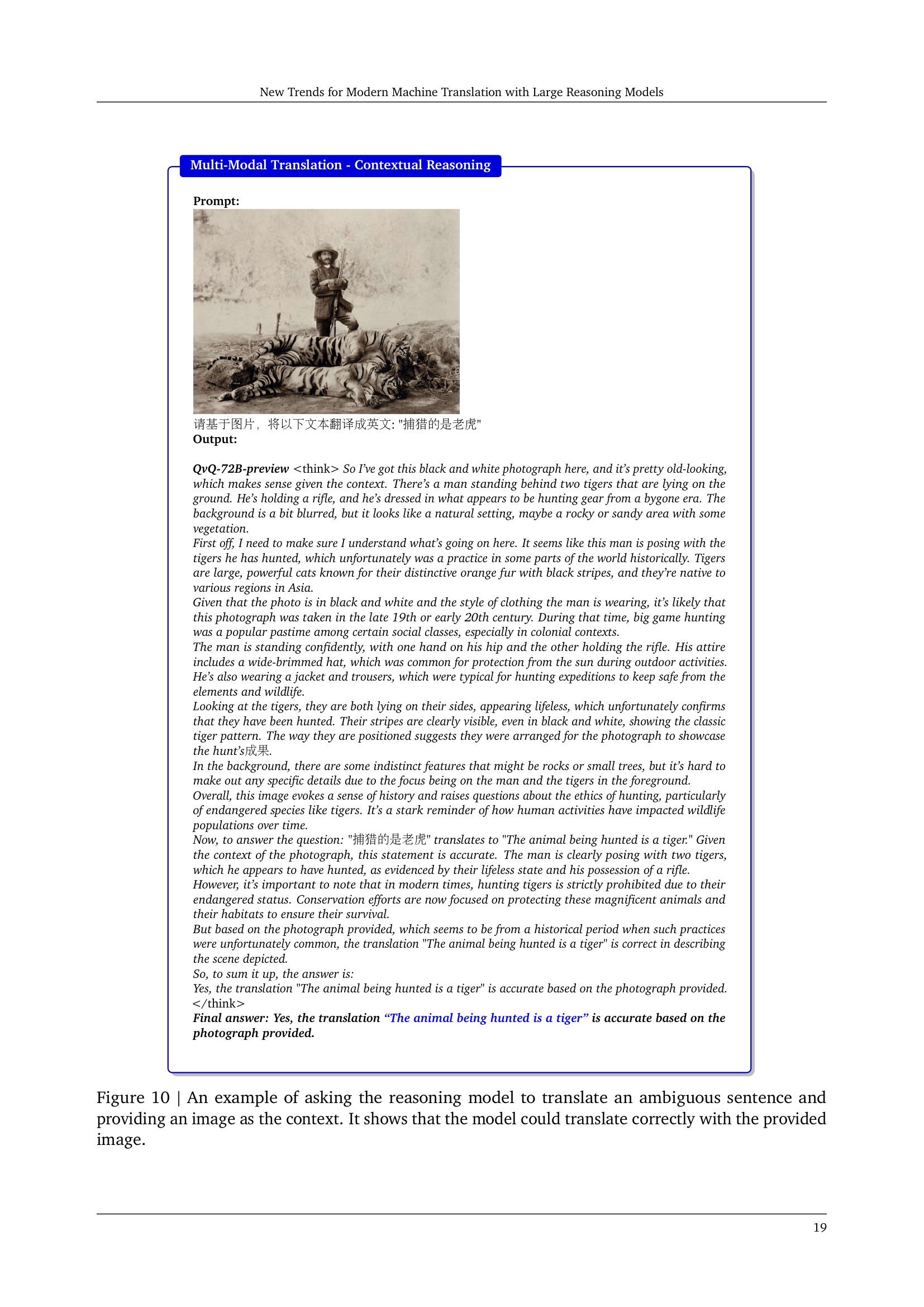
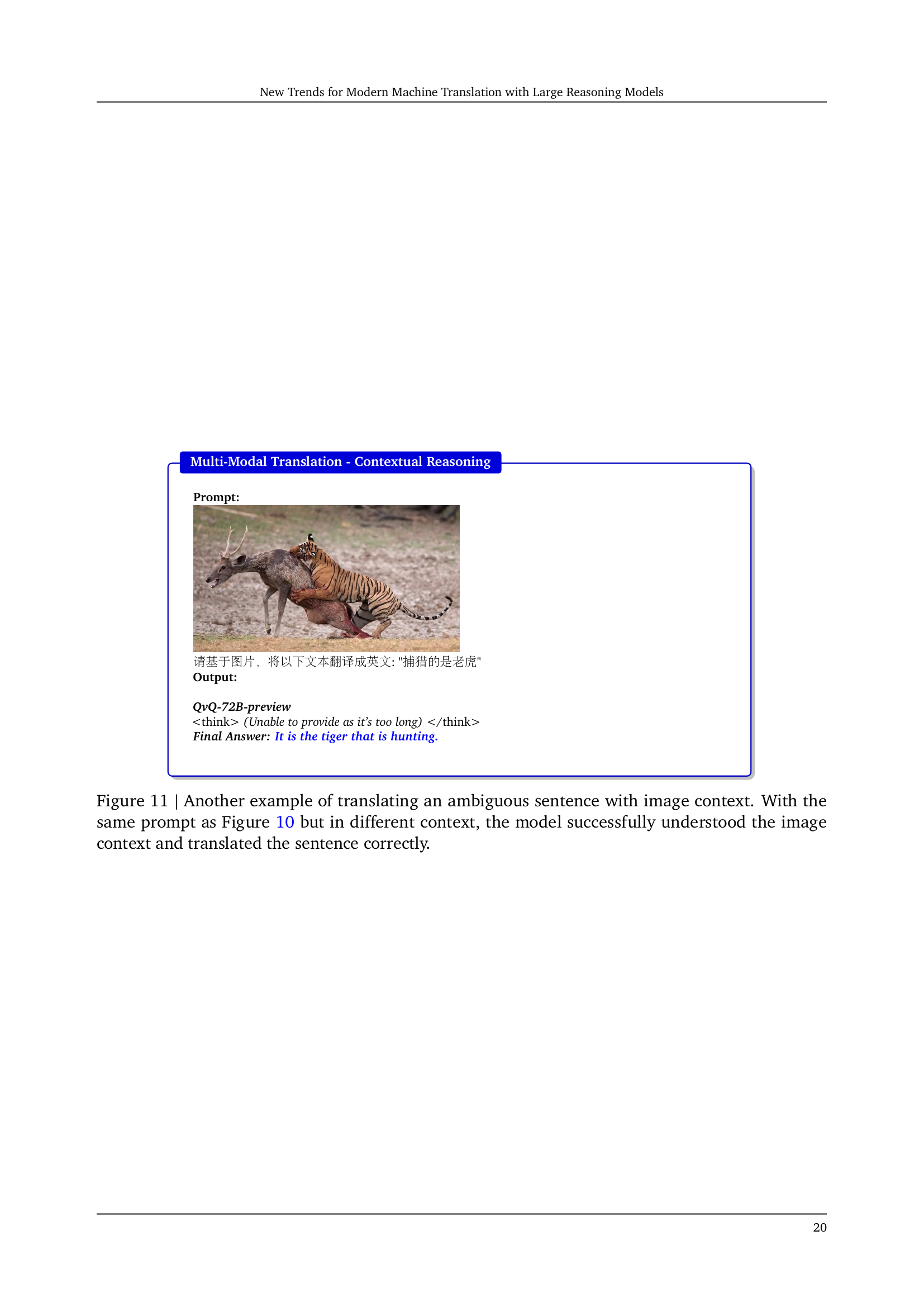