TL;DR#
Audio-driven talking portrait generation has challenges like fixed 3D keypoints that limit facial detail, & traditional networks struggle with audio-keypoint causality, resulting in low pose diversity. Also, image-based methods have identity distortion with high cost. To solve it, this paper introduces KDTalker to combine unsupervised implicit 3D keypoints with a spatiotemporal diffusion model, adapting facial information for diverse poses and fine details. A custom spatiotemporal attention ensures accurate lip sync and enhances efficiency.
KDTalker uses implicit 3D deformation keypoints and transformation parameters from audio, capturing lip, expressions, and head poses. Transformed keypoints are used for facial rendering. Unlike 3DMM, KDTalker’s keypoints adapt to facial densities, enhancing expressiveness. Experiments show KDTalker’s SOTA lip sync, pose diversity, & efficiency, offering a new appraoch in virtual reality & digital human creation.
Key Takeaways#
Why does it matter?#
This work advances audio-driven talking portrait generation by offering a more efficient and accurate method that enhances both realism and diversity. It presents a new approach for future research, particularly in improving identity preservation and expressiveness.
Visual Insights#
🔼 KDTalker is a novel framework for generating high-fidelity talking videos from a single image and audio. It uses a keypoint-based spatiotemporal diffusion model that produces realistic and temporally consistent animations. The model combines implicit 3D keypoints to model diverse head poses and fine facial details, enhancing both pose diversity and expression detail, with a custom designed spatiotemporal attention mechanism for accurate lip synchronization.
read the caption
Figure 1: The proposed KDTalker: A keypoint-based spatiotemporal diffusion framework that generates synchronized, high-fidelity talking videos from audio and a single image, enhancing pose diversity and expression detail with realistic, temporally consistent animations.
Method | Venue | Lip Synchronization | Head Motion | Video Quality | Inference Time | |||
LSE-C | LSE-D | Diversity | FID | CPBD | CSIM | FPS | ||
Real Video | - | 8.243 | 6.929 | 0.639 | 0.000 | 0.353 | 1.000 | 25 |
SadTalker [5] | CVPR’23 | 7.121 | 7.813 | 0.494 | 12.701 | 0.280 | 0.929 | 21.277 |
Real3DPortrait [6] | ICLR’24 | 7.004 | 7.804 | 0.118 | 17.428 | 0.239 | 0.937 | 3.092 |
AniTalker [10] | MM’24 | 7.107 | 8.077 | 0.374 | 10.244 | 0.305 | 0.893 | 14.045 |
AniPortrait [11] | ArXiv’24 | 3.438 | 10.946 | 0.353 | 14.480 | 0.401 | 0.928 | 1.555 |
Ours | - | 7.326 | 7.548 | 0.760 | 9.756 | 0.277 | 0.949 | 21.678 |
🔼 This table presents a quantitative comparison of the proposed KDTalker model against other state-of-the-art methods for audio-driven talking portrait generation, using the HDTF dataset. It evaluates performance across several key metrics: lip synchronization accuracy (LSE-C and LSE-D), head motion diversity, video quality (FID and CPBD), identity preservation (CSIM), and inference speed (FPS). Higher LSE-C and lower LSE-D values indicate better lip synchronization. Higher diversity scores reflect more varied head poses. Lower FID scores denote higher visual realism. Higher CPBD scores indicate better image clarity. Higher CSIM scores mean better identity preservation. Higher FPS values mean faster generation. The table allows for a comprehensive comparison of the different models based on these objective metrics.
read the caption
Table 1: Quantitative comparison with the state-of-the-art methods on HDTF dataset.
In-depth insights#
Pose Diversity#
Pose diversity in audio-driven talking head generation is crucial for realism. Early methods lacked it, producing stiff animations. Recent work balances lip sync with natural head movements, but challenges remain. Keypoint-based methods, while preserving identity, can struggle with subtle motions and fixed keypoints. Image-based methods offer diverse details but face identity distortion and computational costs. Achieving a balance between accurate lip sync, diverse head poses, and efficient generation is key. The use of unsupervised keypoints and spatiotemporal diffusion models shows promise in enhancing pose variation while maintaining synchronization and computational feasibility. Explicit control over head poses is essential for greater flexibility. User studies and quantitative metrics are needed to assess the effectiveness of different approaches in achieving diverse and natural head movements. The ideal solution should generate temporally coherent motions that align with the audio input without sacrificing realism or computational efficiency. Pose diversity and keypoint adaptation are a good match.
KDTalker Design#
KDTalker, as a novel approach in audio-driven talking portrait generation, likely represents a significant advancement by combining implicit keypoint representations with spatiotemporal diffusion models. A well-designed KDTalker architecture should address the limitations of existing methods by ensuring accurate lip synchronization, diverse and realistic head pose variations, and efficient processing. The system design would involve extracting relevant features from both audio and a reference image, mapping audio cues to keypoint movements, and using a diffusion process to generate realistic facial expressions over time. The spatiotemporal component would need to capture dependencies between frames, allowing for coherent movements and avoiding jerky transitions. Effective attention mechanisms would ensure audio cues align with facial expressions and head poses. The design choices might involve the selection of a diffusion model architecture, the type of keypoint representation, and the integration of pre-trained models. The system’s efficiency and accuracy would be critical design goals.
Implicit KeyPts#
The concept of implicit keypoints in audio-driven talking portrait generation represents a significant advancement. Instead of relying on fixed, predefined landmarks, implicit keypoints dynamically adapt to facial feature densities, enabling more nuanced motion capture. This adaptability is crucial for capturing subtle expressions like eye movements and frowning, which are often missed by methods using traditional 3D Morphable Models. Furthermore, the unsupervised nature of learning these keypoints eliminates the need for manual annotation and enhances the model’s ability to generalize across diverse faces. By integrating these keypoints with spatiotemporal diffusion models, researchers can generate highly realistic and expressive talking portraits, addressing the limitations of earlier approaches that often resulted in stiff, unnatural animations due to limited head pose diversity and expression detail. The dynamic adaptation enables flexible motion capture via diffusion modeling, greatly improving the final output.
Spatio-Temporal#
Spatio-temporal modeling acknowledges that data has both spatial and temporal dimensions, necessitating methods that capture dependencies across both. In research, especially involving dynamic scenes or processes, this is crucial. Methods may involve analyzing how phenomena evolve over time and space, such as movement patterns or changes in an environment. Effective spatio-temporal analysis often uses techniques like time-series analysis combined with spatial statistics or specialized models. Such as recurrent neural networks. Addressing challenges like computational complexity and the need for rich, high-quality data is paramount for useful insights. Ultimately, the goal is to understand not just what is happening, but where and when, for a more holistic view.
Limited Occlusion#
Addressing limited occlusion in the context of audio-driven talking portraits poses a significant challenge. Occlusion hinders accurate keypoint detection and tracking, especially around crucial areas like the mouth and eyes. Methods must be robust to these visual disruptions, perhaps by employing contextual reasoning or inferential filling of missing information. A potential solution lies in using advanced generative models that can realistically hallucinate occluded facial regions based on audio cues and prior facial knowledge. Furthermore, robust training strategies using data augmentation with simulated occlusions can improve the model’s resilience. Alternatively, incorporating multi-modal information (e.g., depth data) could aid in disambiguating occluded regions. Ultimately, a system that effectively handles partial occlusions would significantly enhance the realism and robustness of talking portrait generation.
More visual insights#
More on figures
🔼 This figure shows a comparison of different talking portrait generation methods across three metrics: inference time (frames per second), head pose diversity, and lip synchronization accuracy (measured by Lip Sync Error Distance, or LSE-D). Each method is represented by a point on the graph, with its horizontal position determined by inference time and its vertical position by head pose diversity. The size of the point corresponds to the LSE-D score; smaller points indicate better lip synchronization. This visualization allows for a direct comparison of the trade-offs between speed, diversity of head movements, and the accuracy of lip movements in relation to the audio.
read the caption
Figure 2: Inference Time vs Head Diversity &\&& LSE-D. The value of LSE-D (Lip Sync Error Distance), a metric quantifying the alignment between lip movements and audio, is represented by the size of the circle. A smaller circle indicates a lower LSE-D value, reflecting better lip sync performance.
🔼 The figure shows a detailed overview of the KDTalker framework architecture. It illustrates the process of generating talking portrait videos using a combination of audio and image inputs. The input audio is processed by an encoder to extract audio features, while the reference image undergoes extraction of motion and appearance features. These features are then integrated with a noise sequence in the ‘Reference-Guided Priors’ module, which provides input to the core ‘Keypoint-based Spatiotemporal Diffusion’ module. This module predicts implicit 3D keypoints and transformation parameters (scaling, rotation, and translation), which are then used in the ‘Face Render’ module to generate the final talking head video.
read the caption
Figure 3: Overview of the proposed KDTalker for talking portrait synthesis.
🔼 The Reference-Guided Priors module combines motion information from two key reference frames with a noise sequence to guide the diffusion model. The canonical keypoints extracted from the reference image are used as input for the first frame, with zero-padding applied to other parameters. This provides prior information on facial structure, ensuring that canonical keypoints serve as a foundation for subsequent frames. The expression deformation aligns with the canonical keypoints (xe), while other parameters are initialized with zero-padding. These priors are then integrated with a noise sequence to create the input for the diffusion model. By initializing the process with reference frames, the model conditions the noise sequence on structured motion information. This enables temporally coherent motion generation aligned with the original facial structure and adapting to audio-driven dynamics.
read the caption
Figure 4: Reference-Guided Priors.
🔼 This figure illustrates the architecture of the Spatiotemporal-Aware Attention Network, a key component of the KDTalker framework. The network takes as input the timestep (which indicates the stage of the denoising process), audio features, and the output from the ResNet blocks. These inputs are processed through several modules including a Time MLP for temporal embedding, rotary embedding, and a softmax attention mechanism. The final output is a set of refined motion parameters that incorporate both spatial and temporal consistency.
read the caption
Figure 5: Spatiotemporal-Aware Attention Network.
🔼 Figure 6 presents a qualitative comparison of talking head videos generated by different state-of-the-art methods and the proposed method (KDTalker) using the HDTF dataset. For each method, several frames from a generated video are shown alongside the corresponding ground truth frames. This visual comparison allows for a direct assessment of the realism, accuracy of lip synchronization, and overall quality of the generated videos. The figure highlights KDTalker’s ability to generate high-fidelity animations that closely match the natural head movements and lip synchronization of the ground truth videos.
read the caption
Figure 6: Qualitative comparison with the state-of-the-art methods on HDTF dataset.
🔼 Figure 7 presents a qualitative comparison of head motion diversity between several existing audio-driven talking portrait generation methods and the proposed KDTalker approach. It uses motion heatmaps to visualize the diversity of head movements generated by each method, including SadTalker, Real3DPortrait, AniTalker, AniPortrait, and the proposed method. The heatmaps provide a visual representation of the range and variation of head poses generated, allowing for a direct comparison of the dynamic head motion generated by each method. The ground truth (GT) heatmap is also shown for reference.
read the caption
Figure 7: Qualitative comparison of head motion diversity between existing methods and our proposed approach.
More on tables
Method | Lip | Head | Overall |
---|---|---|---|
Sync. | Diversity | Naturalness | |
SadTalker [5] | 14.5% | 22.8% | 15.8% |
Real3DPortrait [6] | 3.8% | 0.5% | 0.0% |
AniTalker [10] | 39.0% | 13.0% | 38.5% |
AniPortrait [11] | 2.0% | 2.0% | 0.0% |
Ours | 40.7% | 61.7% | 45.7% |
🔼 This table presents the results of a user study comparing the performance of different talking portrait generation methods across three key dimensions: lip synchronization, head motion diversity, and overall naturalness. The study involved 20 participants who evaluated 20 sample videos, selecting the best method for each sample based on each criterion. The percentages shown indicate the proportion of participants who rated each method as the best in each dimension.
read the caption
Table 2: User study showing the percentage of participants who selected a method as the best across three evaluation dimensions.
Method | Lip Synchronization | Head Motion | |
---|---|---|---|
LSE-C | LSE-D | Diversity | |
w/o Reference | 6.656 | 8.203 | 0.791 |
w/o Attention | 1.360 | 11.777 | 2.292 |
w/o RoPE | 7.225 | 7.663 | 0.706 |
Ours Full | 7.326 | 7.548 | 0.760 |
🔼 This table presents the results of an ablation study conducted to evaluate the impact of different components of the proposed KDTalker model on its performance. It shows quantitative metrics (LSE-C, LSE-D, and Diversity) to assess the effect of removing key components, such as the reference keypoints (xc), the spatiotemporal attention mechanism, and the Rotary Positional Embedding (ROPE) used for temporal modeling. The results highlight the contribution of each component to lip synchronization accuracy, head motion diversity, and overall model performance.
read the caption
Table 3: Ablation study for main components in proposed method.
Method | Lip Synchronization | Head Motion | |
---|---|---|---|
LSE-C | LSE-D | Diversity | |
VAE [13] | 0.665 | 13.879 | 0.846 |
GAN [14] | - | - | - |
VAE [13] + GAN [14] | 1.232 | 13.284 | 0.876 |
Diffusion (Ours) | 7.326 | 7.548 | 0.760 |
🔼 This table presents a comparison of the proposed KDTalker model with variations using different generative approaches (VAE, GAN, and a combined VAE-GAN) in place of the diffusion model. It quantifies the performance of each model variation across lip synchronization accuracy (LSE-C and LSE-D) and head motion diversity, showing the superiority of the diffusion-based model used in the original KDTalker architecture.
read the caption
Table 4: Comparison of our model with different generators
Method | Points | Lip Synchronization | Video Quality | |||
---|---|---|---|---|---|---|
LSE-C | LSE-D | FID | CPBD | CSIM | ||
F.V. [24] | 15 | 5.579 | 9.228 | 8.625 | 0.307 | 0.940 |
L.P. [28] | 21 | 7.326 | 7.548 | 9.756 | 0.277 | 0.949 |
🔼 This table presents an ablation study comparing two face rendering methods: Face-vid2vid (F.V.) and LivePortrait (L.P.), used in the proposed KDTalker model. It evaluates their performance on lip synchronization accuracy (LSE-C, LSE-D), and overall video quality (FID, CPBD, CSIM). The number of keypoints used by each method is also listed, highlighting the impact of keypoint representation on the final results.
read the caption
Table 5: Ablation of Face Render. F.V. denotes Face-vid2vid, while L.P. stands for LivePortrait, which is used in our method.
Step | Lip Synchronization | Video Quality | |||
---|---|---|---|---|---|
LSE-C | LSE-D | FID | CPBD | CSIM | |
1 | 0.817 | 12.630 | 19.058 | 0.262 | 0.823 |
5 | 7.455 | 7.424 | 10.226 | 0.277 | 0.949 |
10 | 7.448 | 7.436 | 9.939 | 0.278 | 0.948 |
20 | 7.394 | 7.501 | 9.797 | 0.277 | 0.945 |
50 | 7.326 | 7.548 | 9.756 | 0.277 | 0.949 |
100 | 7.264 | 7.614 | 9.987 | 0.277 | 0.948 |
200 | 7.221 | 7.633 | 9.901 | 0.277 | 0.948 |
🔼 This table presents the results of an ablation study on the number of inference steps used in the KDTalker model. It shows how varying the number of steps impacts lip synchronization accuracy (LSE-C and LSE-D) and the overall quality of the generated video (FID, CPBD, and CSIM). The goal is to find the optimal balance between accuracy, quality, and computational efficiency.
read the caption
Table 6: Ablation of inference step
Frame Number | Lip Synchronization | Head Motion | |
---|---|---|---|
LSE-C | LSE-D | Diversity | |
8 | 6.875 | 7.928 | 0.673 |
16 | 6.912 | 7.904 | 0.687 |
32 | 7.256 | 7.636 | 0.686 |
64 | 7.326 | 7.548 | 0.760 |
🔼 This table presents the results of an ablation study on the impact of varying the number of frames used in generating talking head videos. It shows how the lip synchronization accuracy (LSE-C, LSE-D), and head motion diversity change as the number of frames increases from 8 to 64. The goal is to determine the optimal number of frames to balance video quality and computational efficiency.
read the caption
Table 7: Ablation of frame number.
Full paper#
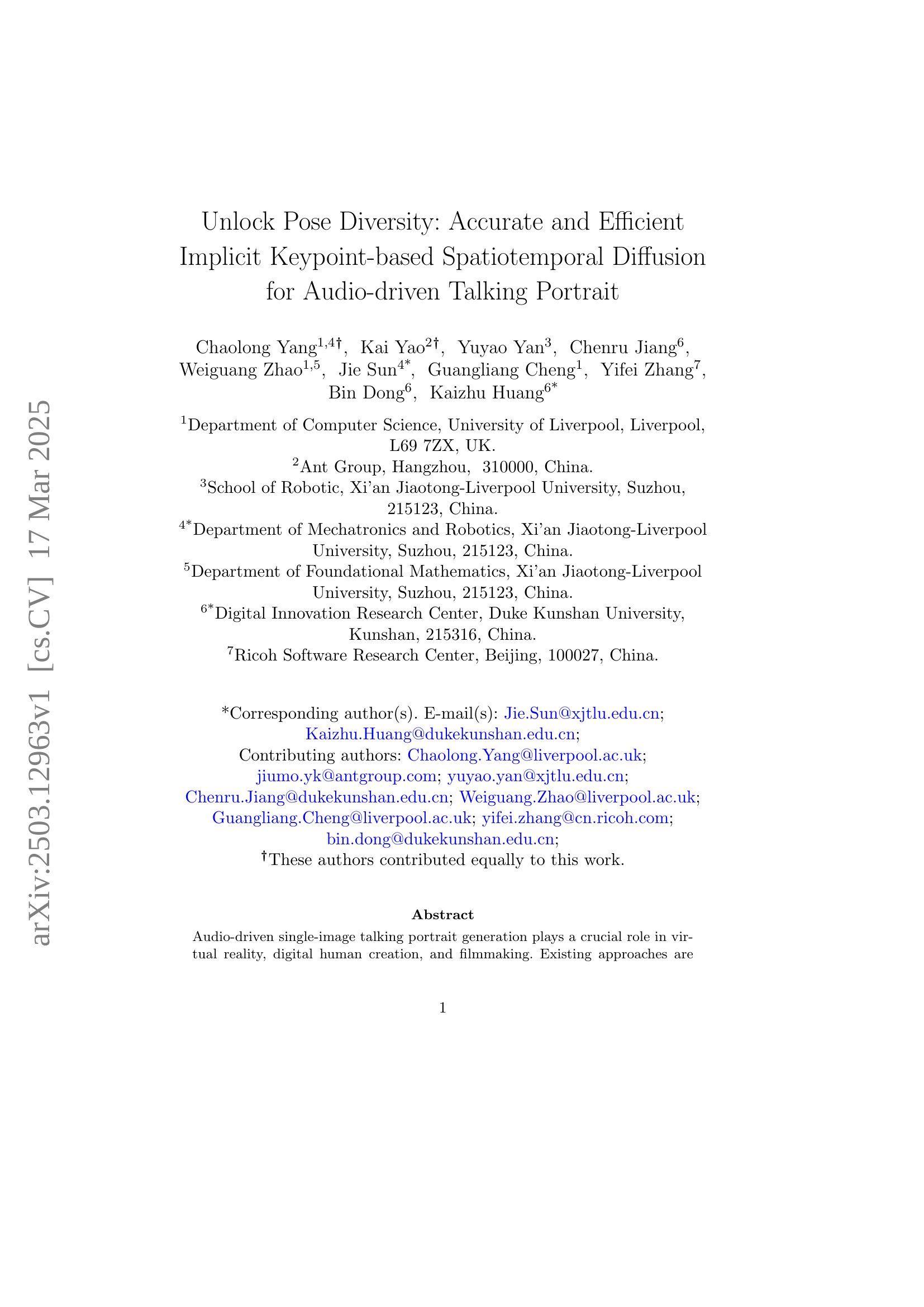
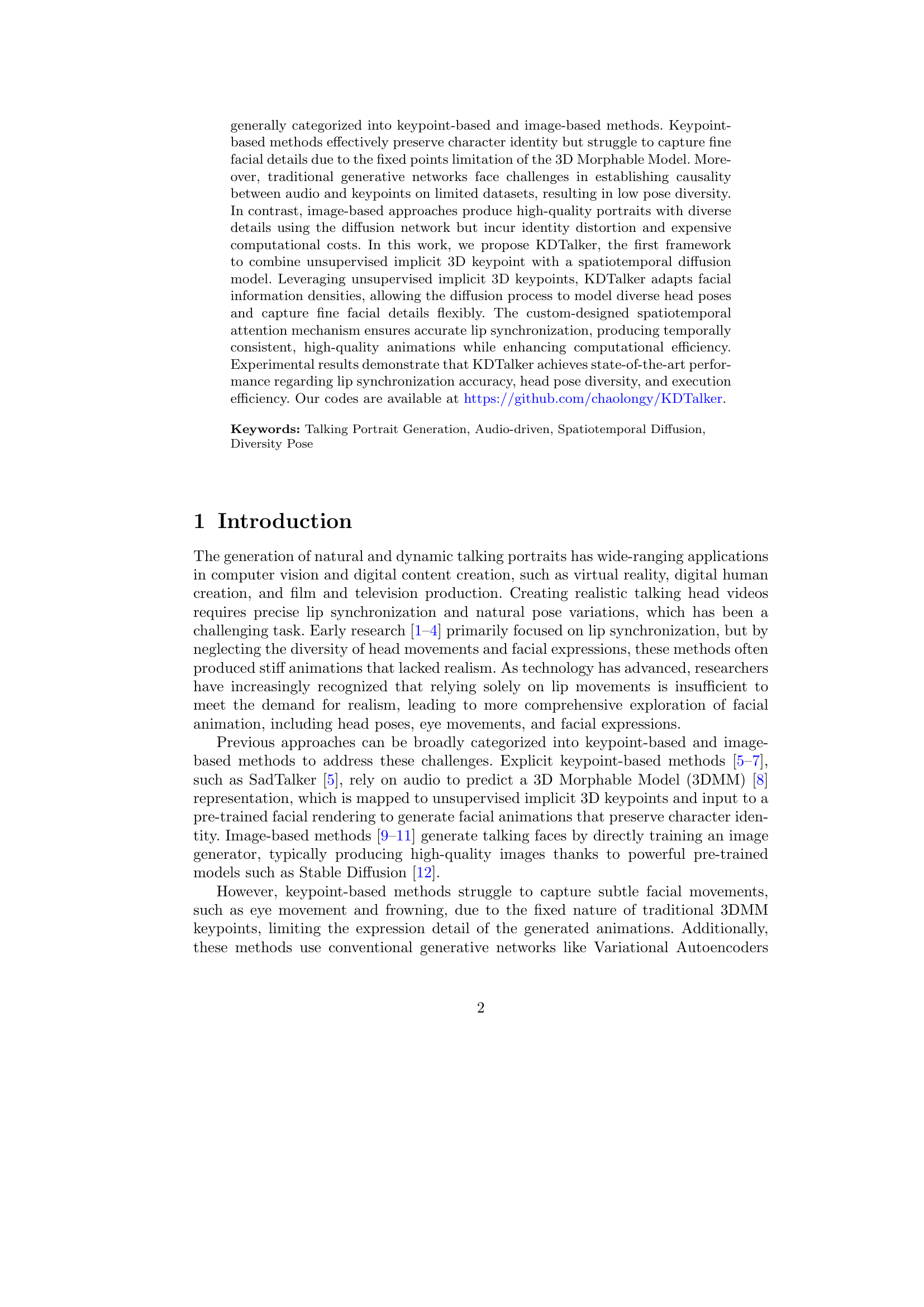
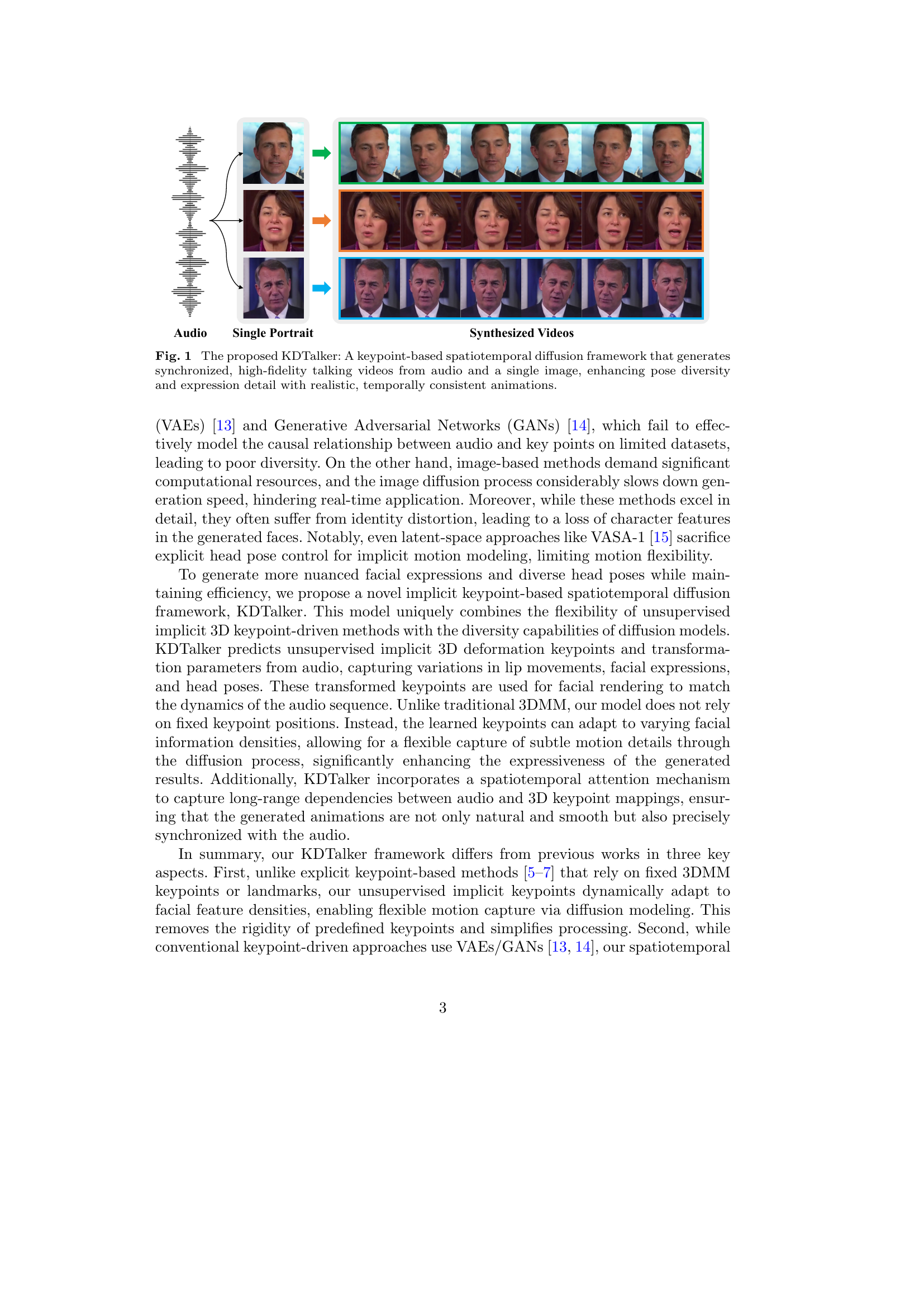
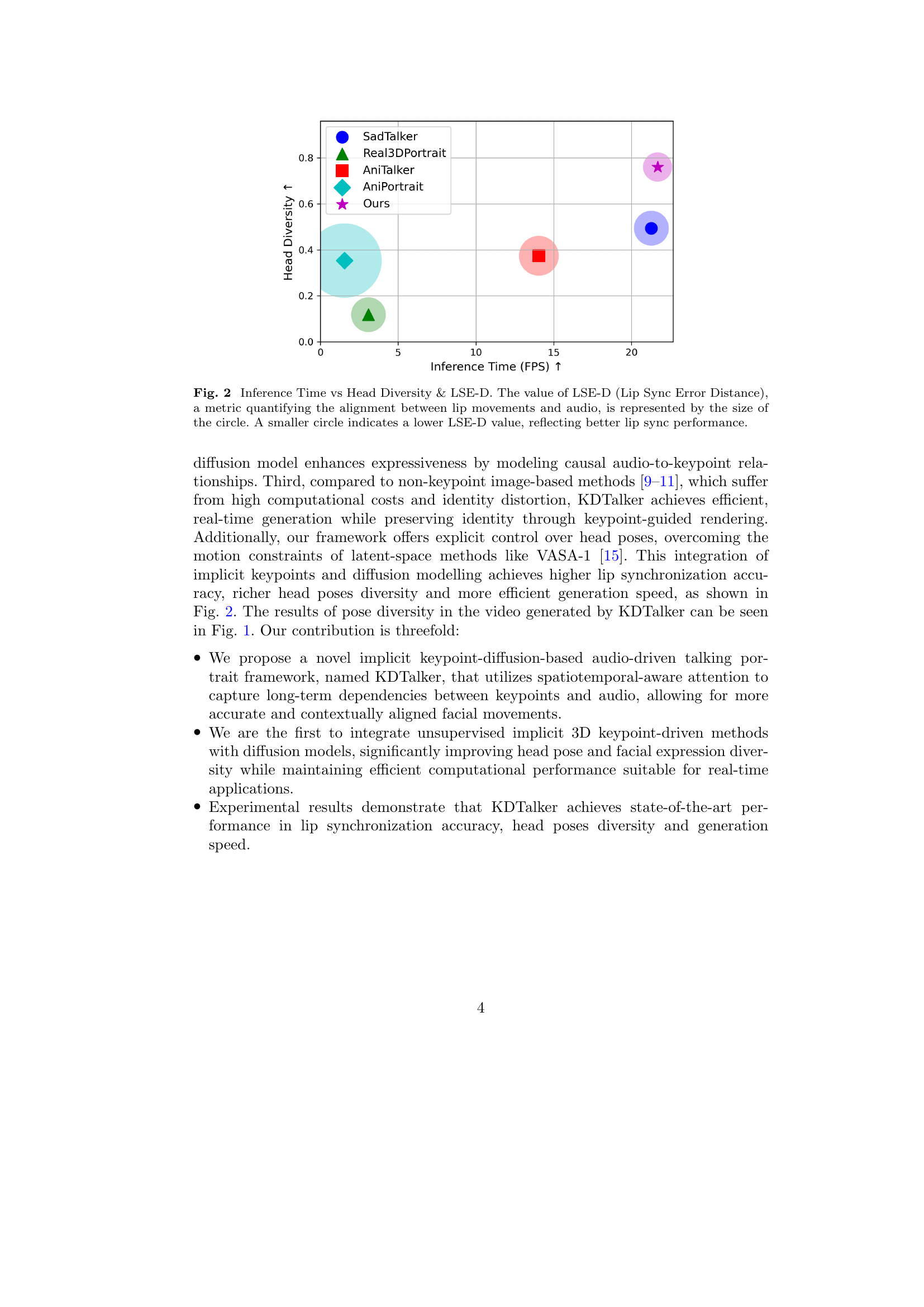
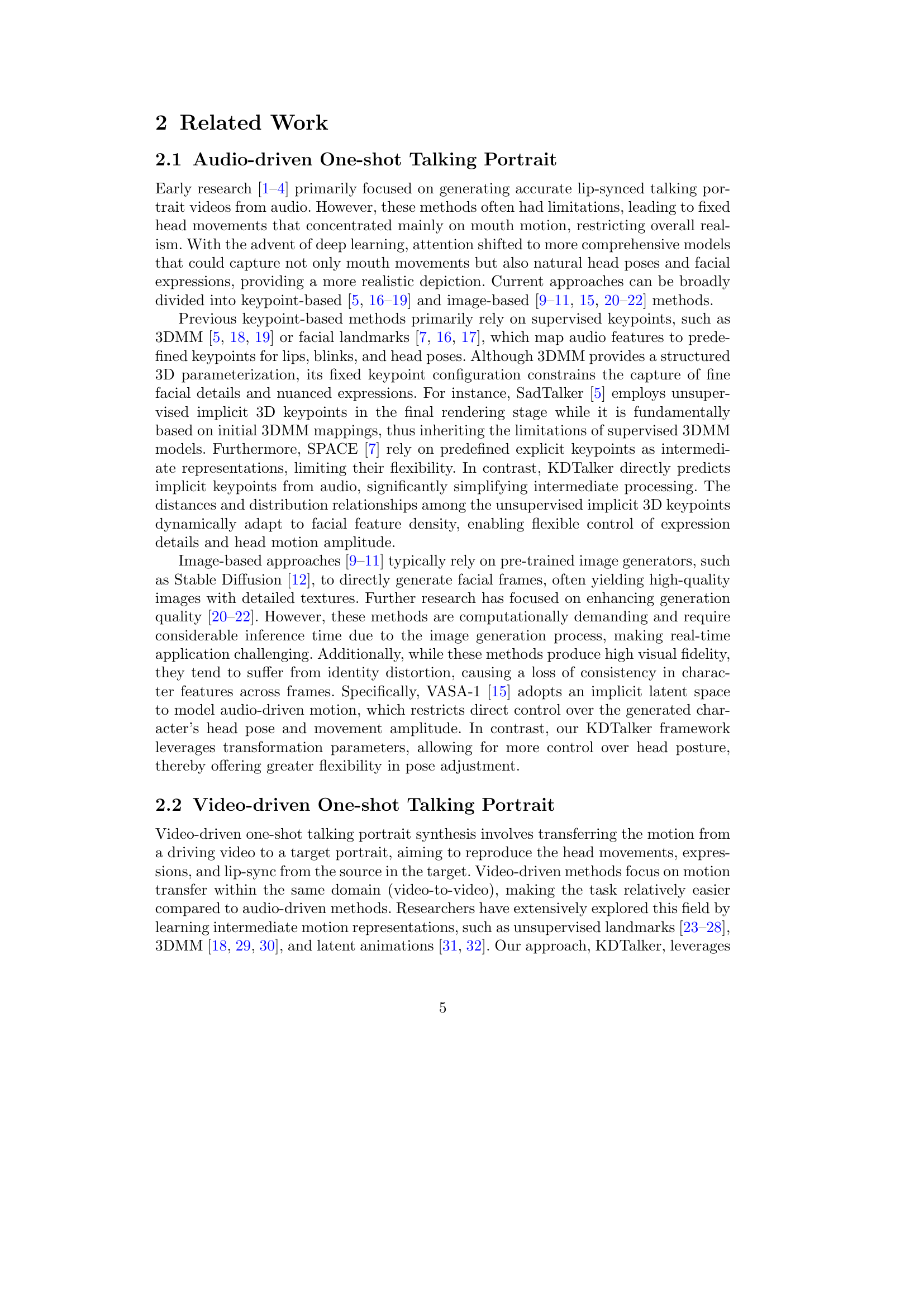
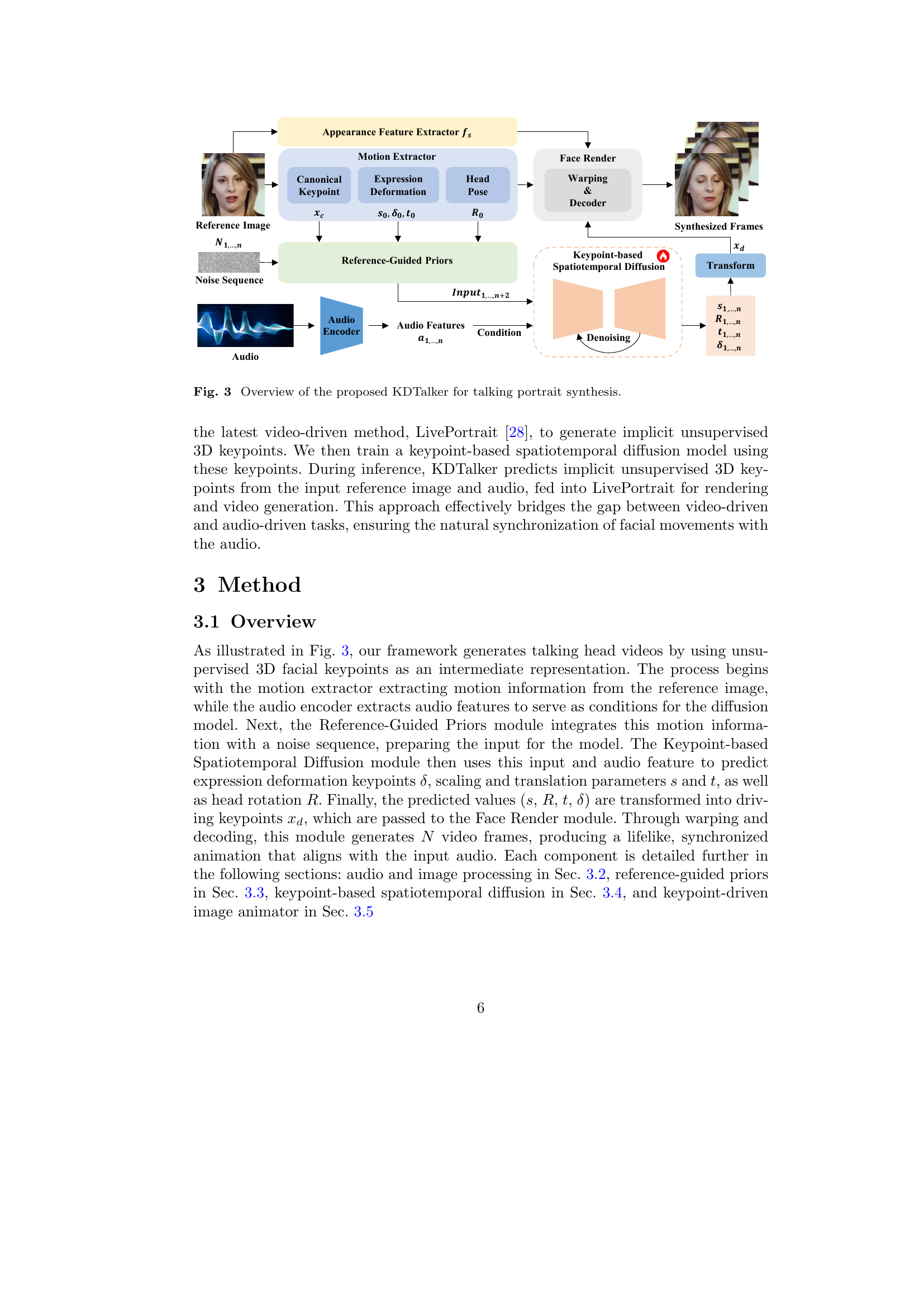
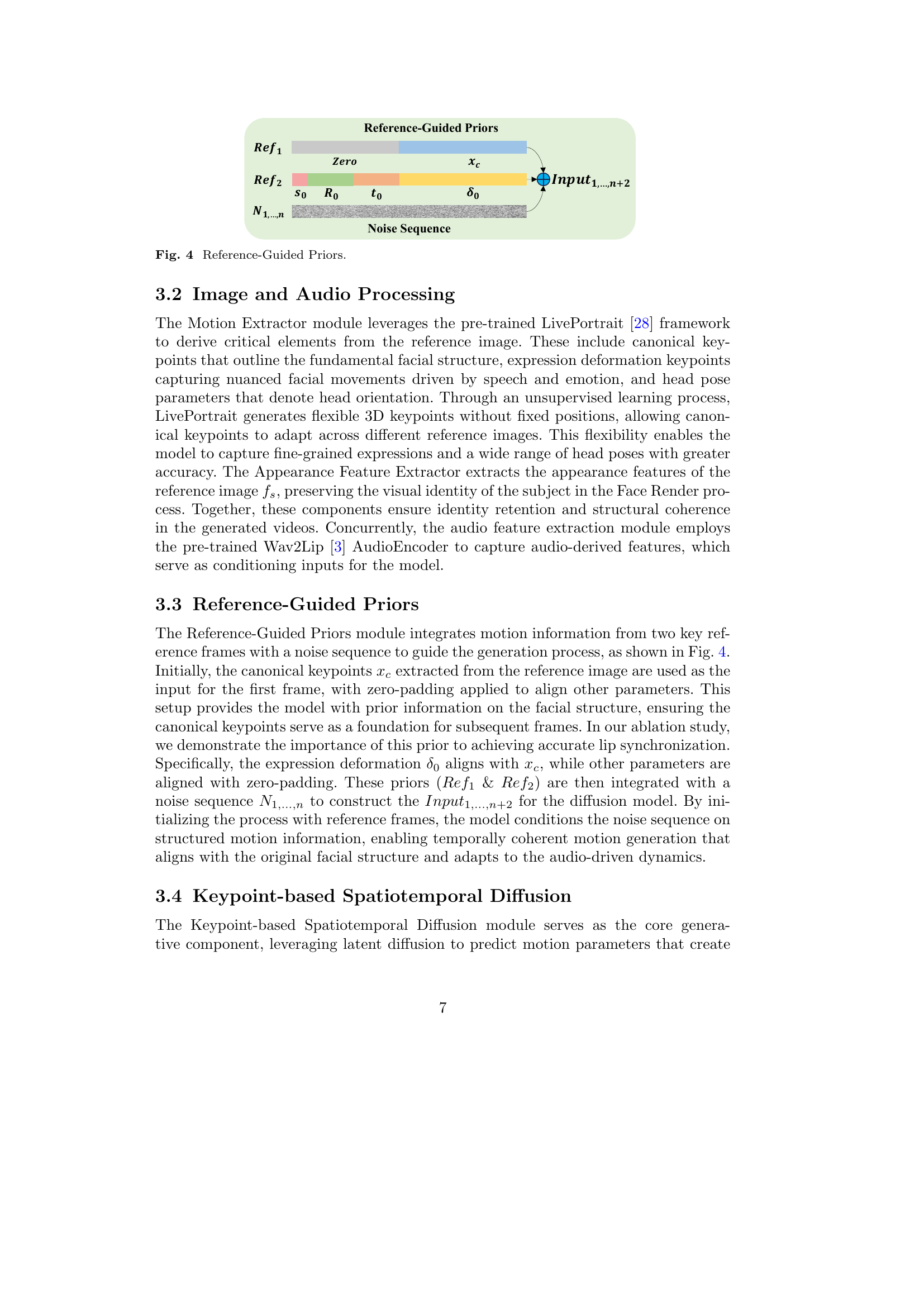
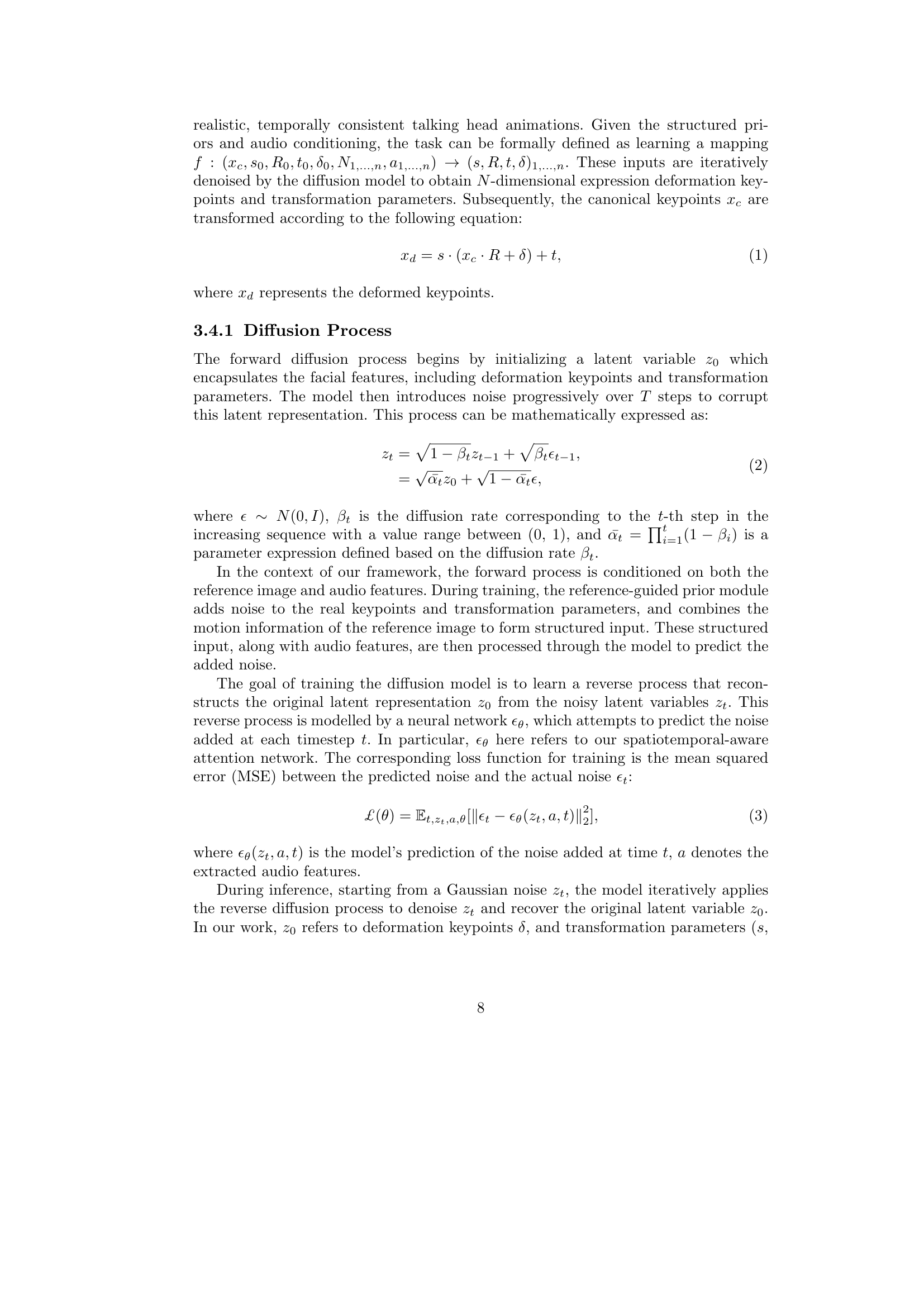
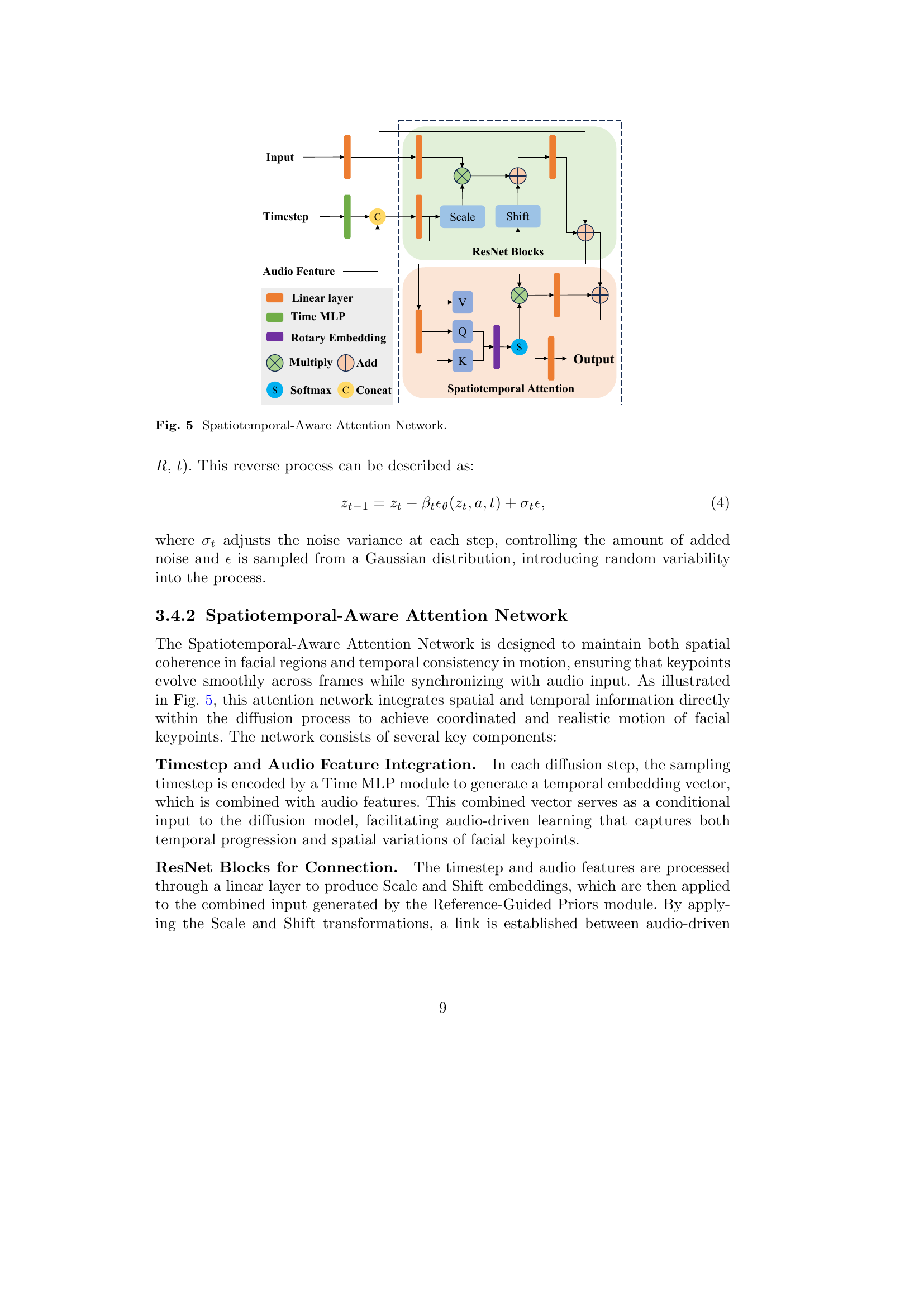
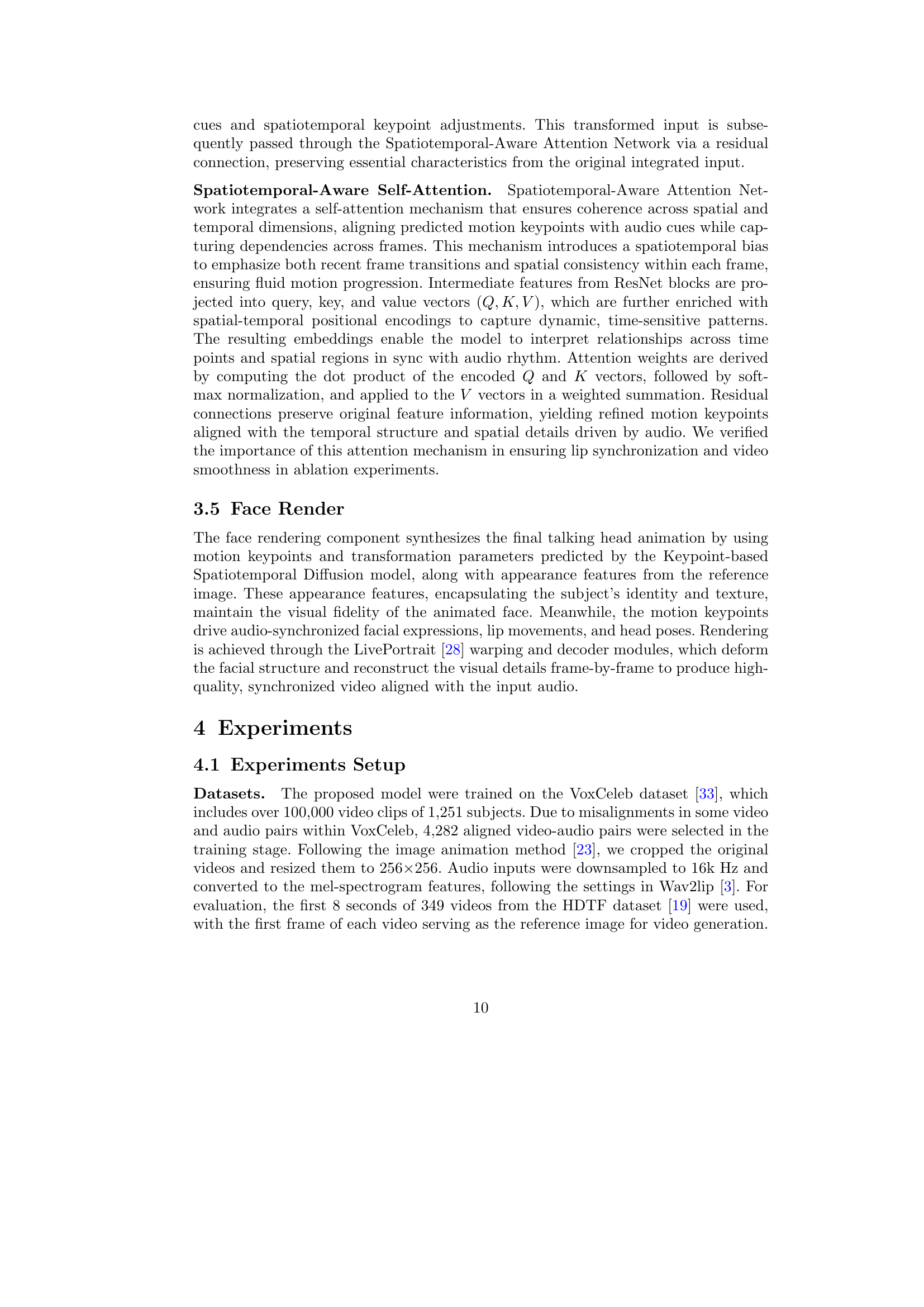
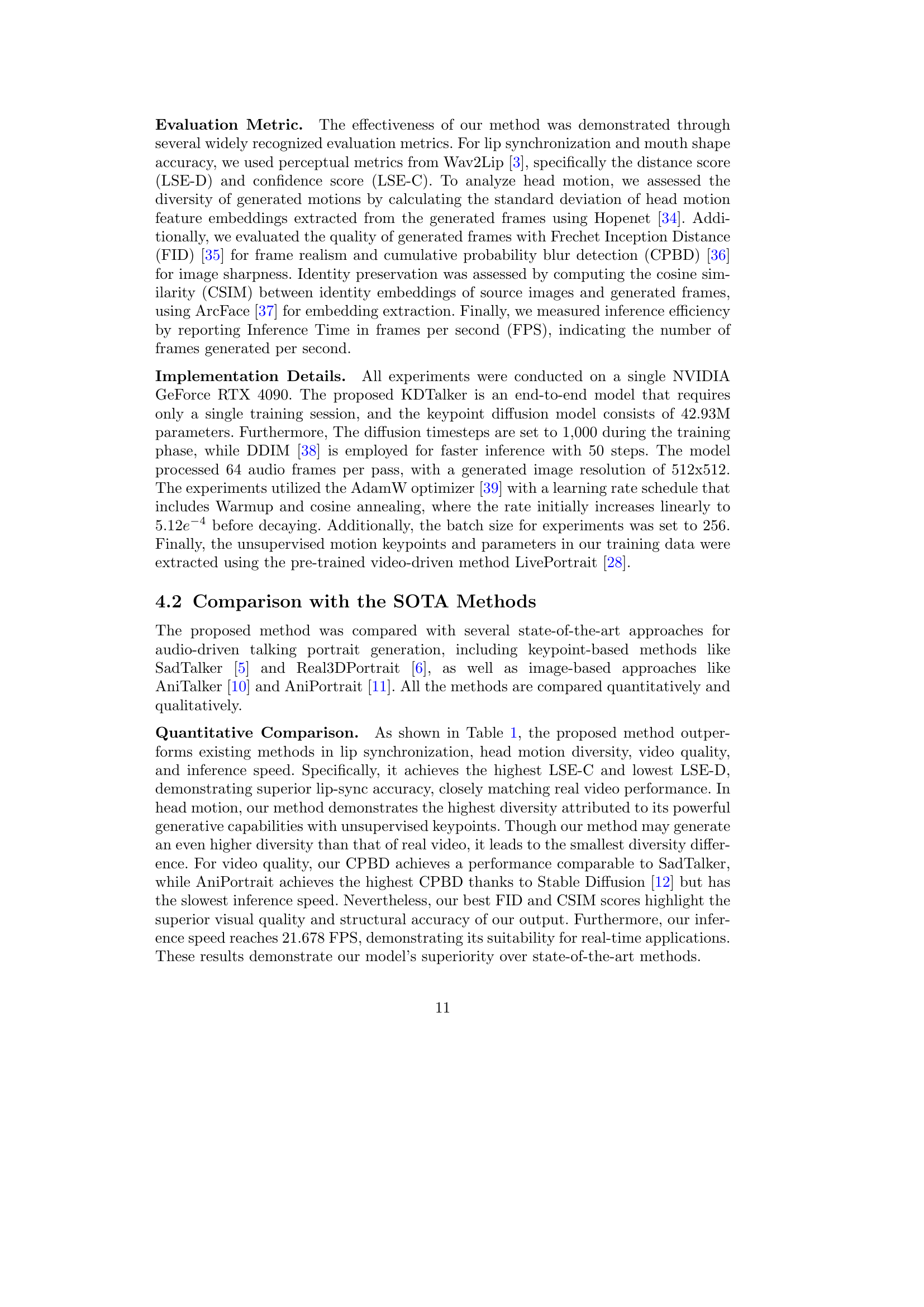
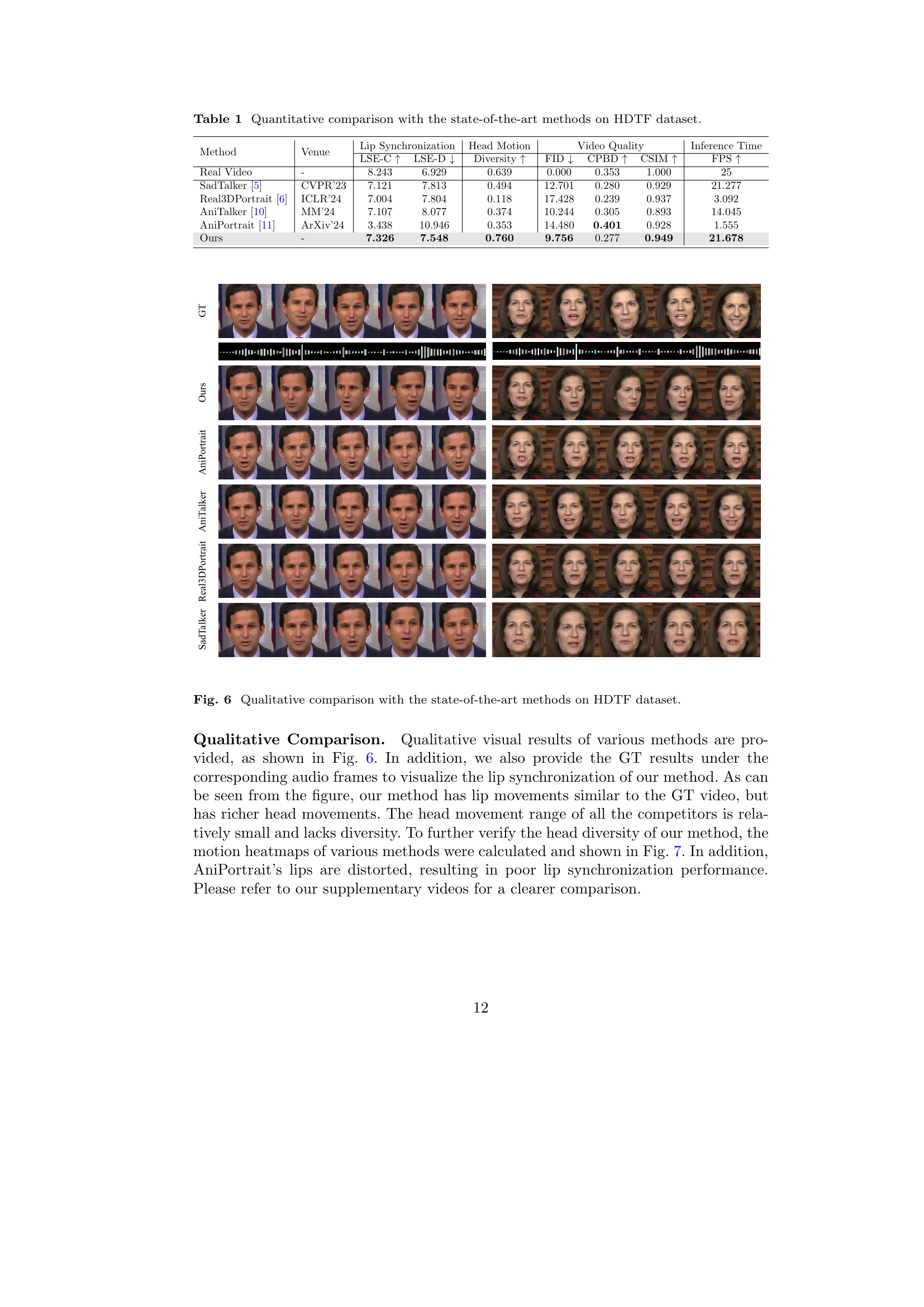
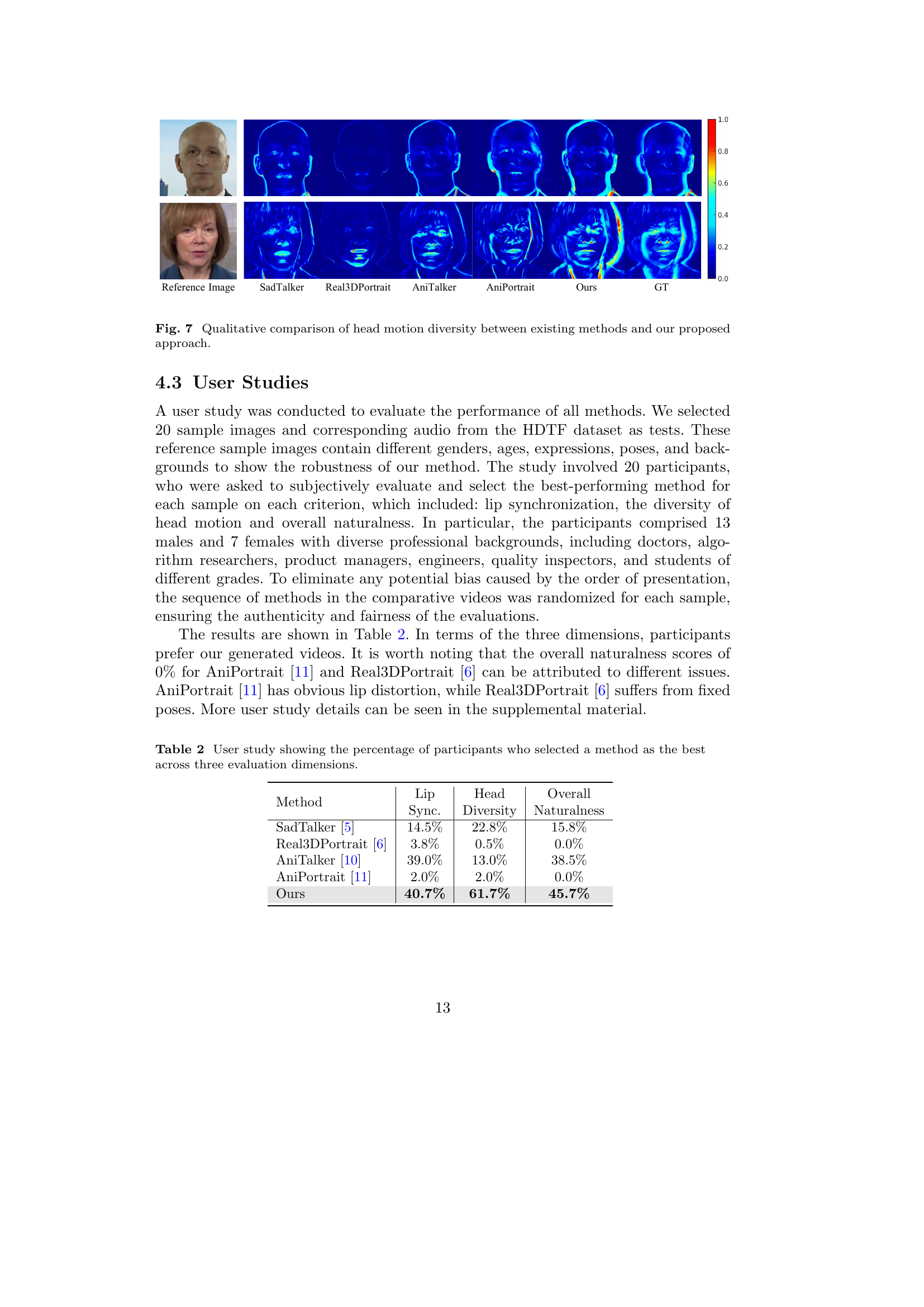
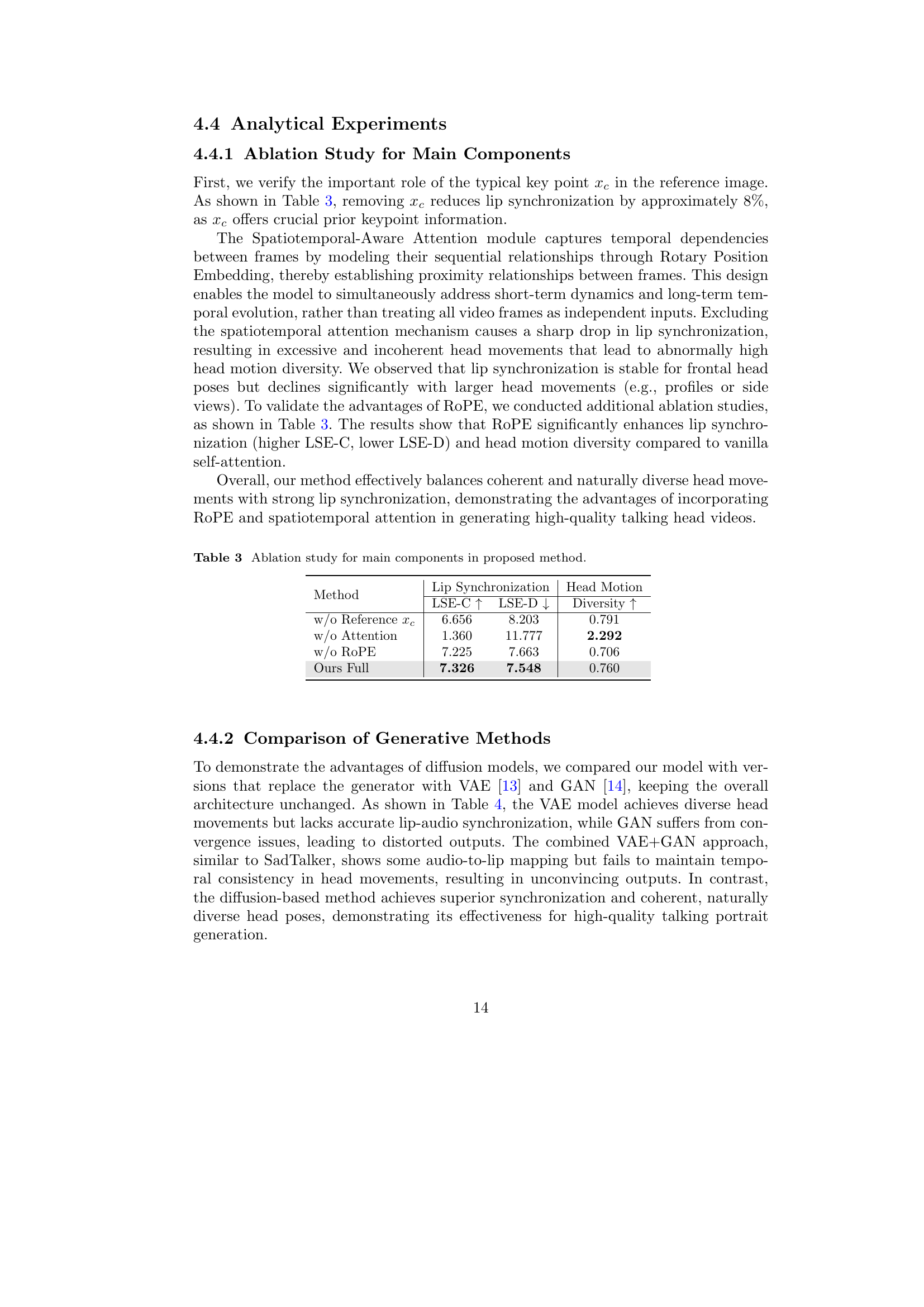
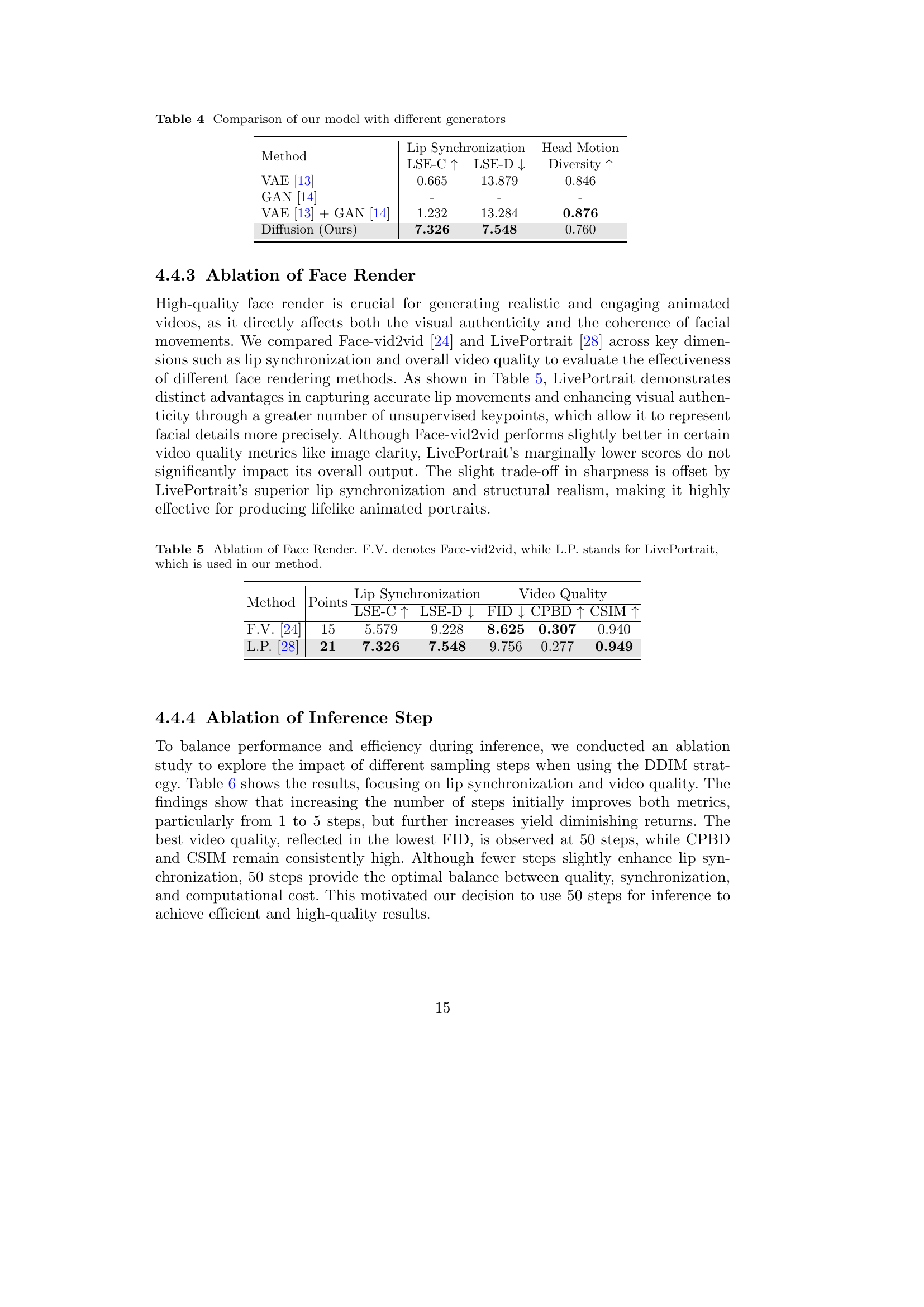
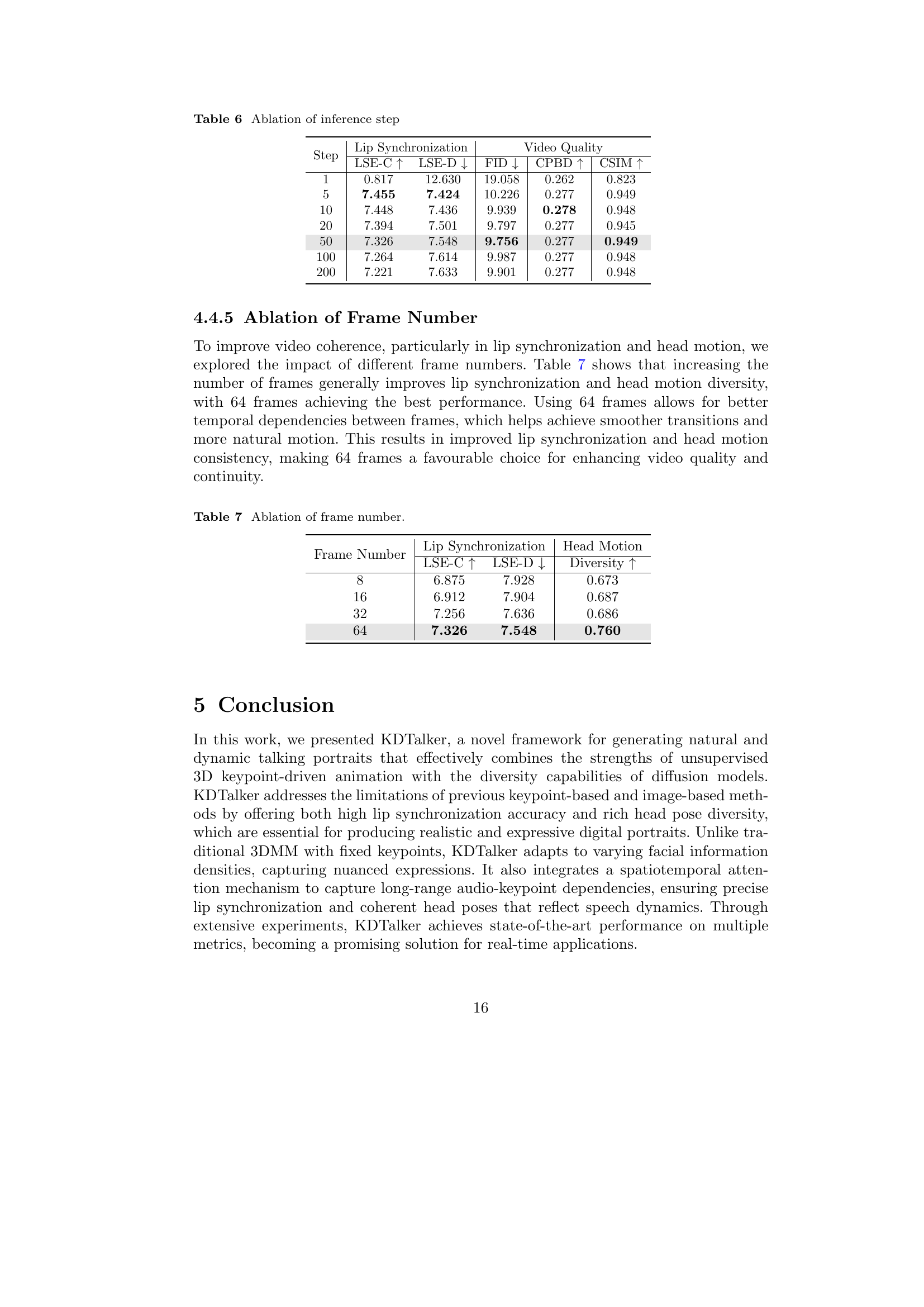
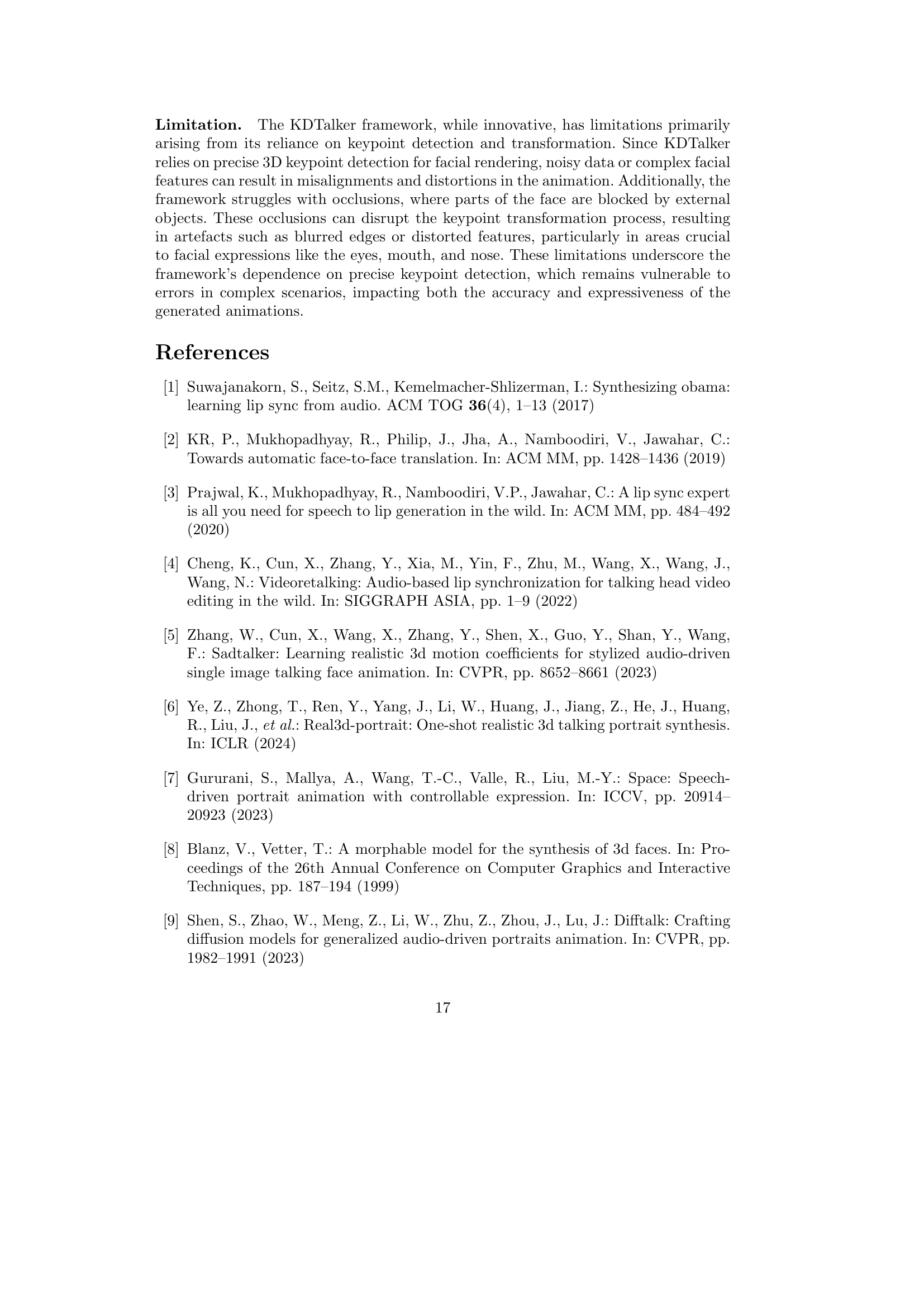
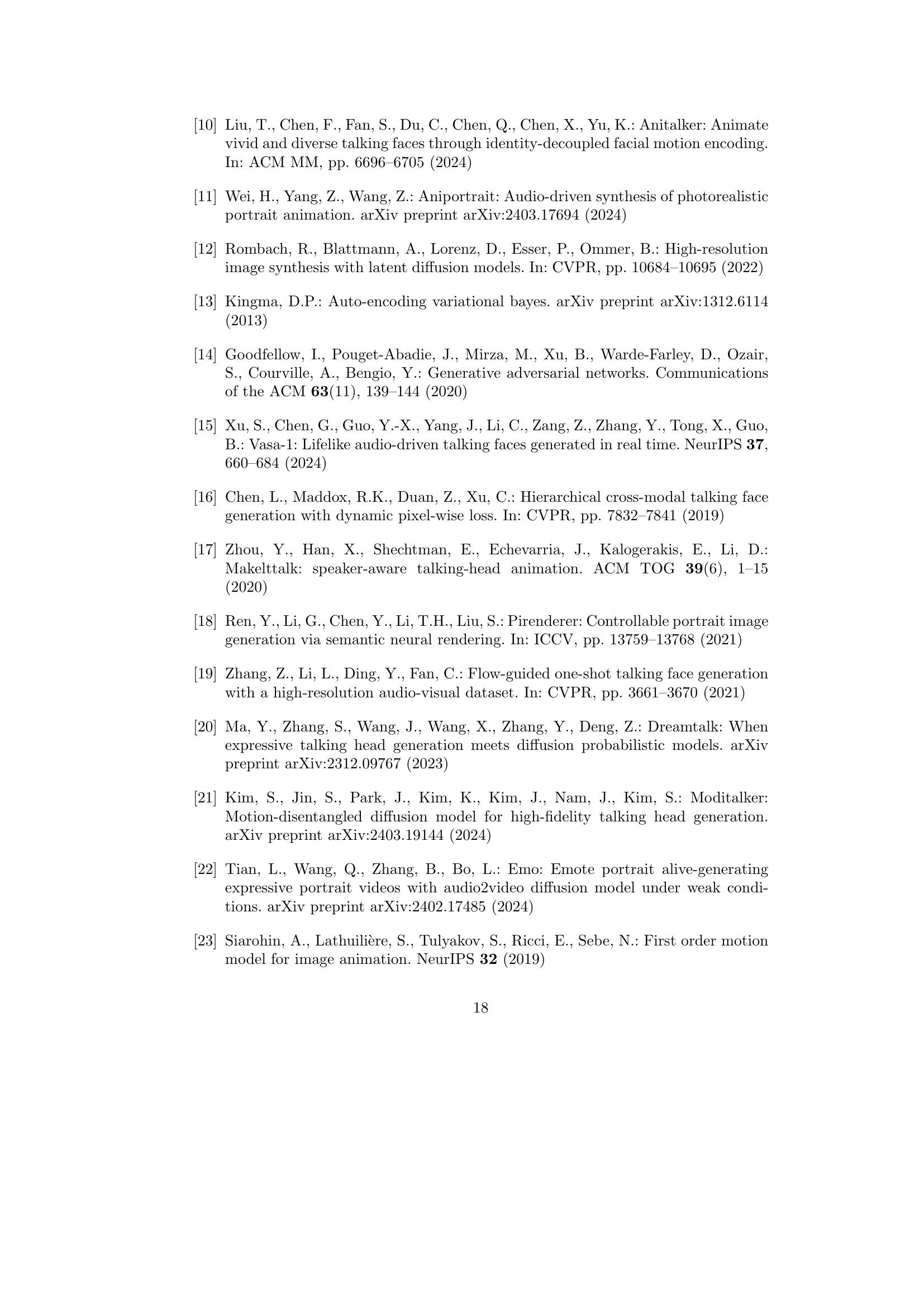
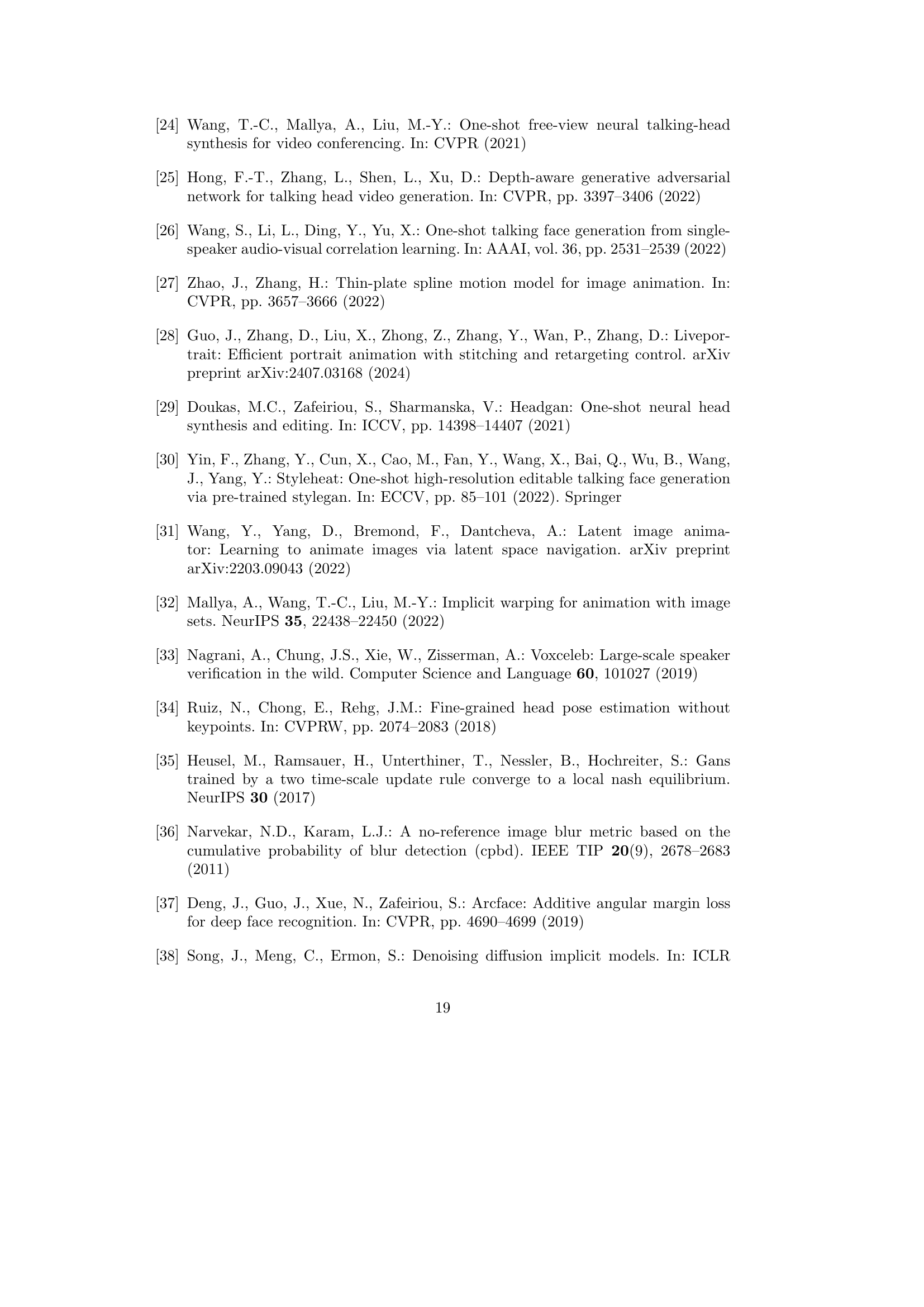
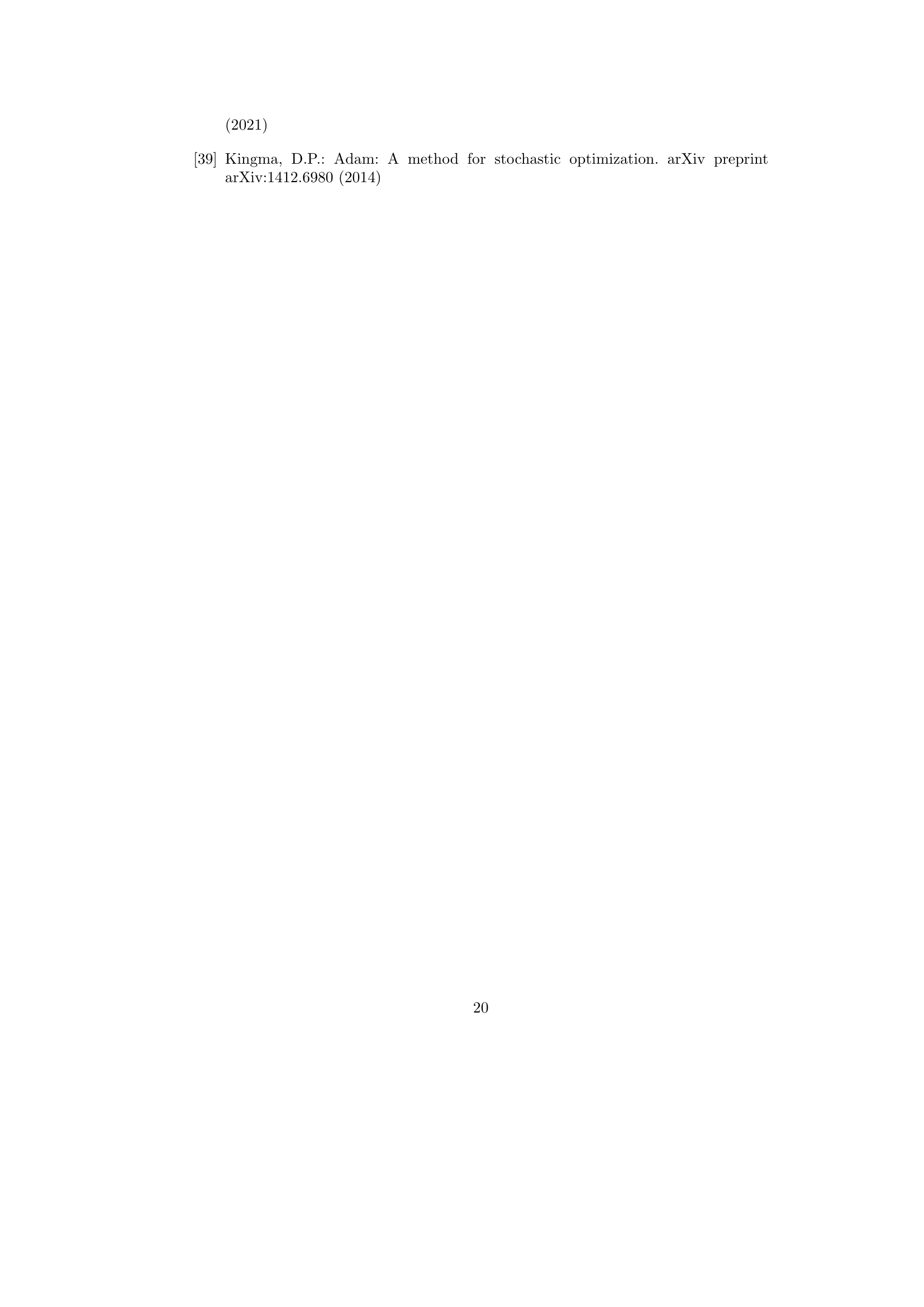