TL;DR#
Existing 4D reconstruction methods are limited by their inability to handle wide-range spatial movements and the lack of suitable datasets for evaluation. Current benchmarks mainly focus on actions performed in place within limited scenarios, highlighting the need for datasets that include more complex spatial dynamics. Additionally, existing methods struggle with deformation fields for dynamics estimation, resulting in poor quality when dealing with wide-range movements.
This paper introduces WideRange4D, a novel 4D reconstruction benchmark designed to address these limitations. It features rich 4D scene data with large spatial variations, enabling a more comprehensive evaluation of 4D generation methods. The paper also presents Progress4D, a new 4D reconstruction method that divides the generation process into high-quality 3D scene reconstruction and progressive fitting of 4D dynamics, achieving stable and high-quality results in complex scenes.
Key Takeaways#
Why does it matter?#
This paper introduces a new benchmark for 4D reconstruction, which can encourage researchers to develop more robust and generalized 4D reconstruction methods. It also introduces a novel method to address the limitations of existing 4D reconstruction techniques, which can serve as a solid foundation for future research in this field.
Visual Insights#
🔼 This figure visualizes a comparison of 4D scene reconstruction results between the proposed method, Progress4D, and an existing state-of-the-art method, 4DGS, on the newly introduced WideRange4D benchmark. The comparison highlights the challenges faced by existing methods in generating high-quality 4D scenes that feature wide-range object movements. Progress4D demonstrates a significant improvement in generating visually consistent and accurate 4D reconstructions, validating its effectiveness.
read the caption
Figure 1: Visualization of the 4D scenes generated by our Progress4D and 4DGS [32] on the WideRange4D. Existing 4D reconstruction methods struggle to generate 4D scenes with wide-range movements, highlighting the need for our proposed new benchmark WideRange4D, and the high-quality 4D scenes produced by Progress4D validate the effectiveness of our method.
Method | L1 | PSNR | SSIM | LPIPS |
---|---|---|---|---|
Dreamscene4D | 0.0168 | 21.33 | 0.75 | 0.30 |
SC4D | 0.0165 | 21.72 | 0.77 | 0.29 |
4DGS | 0.0155 | 24.65 | 0.82 | 0.25 |
ST-4DGS | 0.0153 | 26.35 | 0.84 | 0.24 |
Ours | 0.0145 | 28.86 | 0.87 | 0.22 |
🔼 This table presents a quantitative comparison of different 4D reconstruction methods. The comparison is based on four metrics: L1 loss (lower is better, representing reconstruction error), PSNR (higher is better, representing peak signal-to-noise ratio), SSIM (higher is better, representing structural similarity), and LPIPS (lower is better, representing perceptual similarity). The best performing method for each metric is shown in bold. The methods compared include DreamScene4D, SC4D, 4DGS, ST-4DGS, and the authors’ proposed method, Progress4D.
read the caption
Table 1: Quantitative Comparison of 4D Reconstruction Methods (Best in Bold)
In-depth insights#
Spatial 4D needs#
While ‘Spatial 4D needs’ isn’t explicitly addressed, several implicit needs arise from the paper. Primarily, there’s a need for datasets that capture wide-range spatial movements, moving beyond in-place actions. Existing datasets limit the evaluation of 4D reconstruction methods in complex scenarios. Therefore, the main need is to create high-quality 4D scene reconstruction and maintaining stability, especially in complex 4D scenes. Overcoming deformation field limitations and wide-range variations is the fundamental need. Further, advanced algorithms for generating stable and rational 4D scenes that integrate with current SOTA methods is a practical need.
Progress4D: SOTA#
While the provided text doesn’t explicitly have a “Progress4D: SOTA” heading, the content strongly suggests Progress4D achieves state-of-the-art (SOTA) performance. The paper introduces Progress4D as a novel method for 4D reconstruction, specifically designed to overcome limitations of existing methods in handling wide-range movements and complex scenes. Experimental results on the newly proposed WideRange4D benchmark demonstrate that Progress4D outperforms existing state-of-the-art 4D reconstruction methods. This claim is supported by both quantitative metrics (L1, PSNR, SSIM, LPIPS) and qualitative comparisons. The method’s success is attributed to its two-stage approach: high-quality 3D scene initialization followed by progressive fitting of 4D dynamics. Ablation studies further validate the importance of key components, such as the timestep alignment loss, in achieving stable and high-quality results. Therefore, based on the presented evidence, Progress4D is positioned as a significant advancement in 4D reconstruction and demonstrates SOTA performance.
New: WideRange4D#
The introduction of ‘WideRange4D’ marks a significant advancement in 4D reconstruction. Existing datasets often lack the complexity of real-world scenarios, particularly in capturing wide-range spatial movements. ‘WideRange4D’ directly addresses this limitation by providing a benchmark with diverse scenes and dynamic object motions. This new dataset enables a more comprehensive evaluation of 4D reconstruction methods, pushing the boundaries beyond localized actions. By including varied environments and complex movements, ‘WideRange4D’ presents a more realistic and challenging testbed, ultimately fostering innovation in the field.
Accurate init.#
The accurate initialization is important for 4D scene generation. The initialization is the first step, it greatly impacts the scene quality. A blurred foreground object makes it harder to achieve high-quality 4D synthesis. Hence, the object should be clean to make it easier for the next generation process. It is easier to init a high-quality 3D scene if the test cases in 4D reconstruction benchmarks involve in-place movement. It is hard to init a high-quality 3D scene with a wide-range of movements, the existing reconstruction methods struggle to initialize a high-quality 3D scene. The high-quality 3D reconstruction of all objects in the 4D scene should be performed in their stationary state to ensure high-quality 4D scene reconstruction.
Future: Flow?#
Considering potential future directions in the context of 4D reconstruction, exploring the integration of flow-based methods presents a compelling avenue. Flow-based techniques, particularly flow matching, could offer enhanced efficiency in capturing the dynamic evolution of scenes. The explicit modeling of temporal coherence inherent in flow-based approaches might lead to more stable and accurate reconstruction, addressing current limitations in handling large displacements and complex deformations. The inherent ability of flow matching to define straight flows with velocity consistency would be beneficial. However, challenges remain. The high dimensionality and intricate nature of 4D data necessitate careful design of the flow architecture. Moreover, effective handling of occlusions and discontinuities in the temporal domain would be crucial for robust performance. Integrating flow matching could lead to significant advancements in 4D reconstruction.
More visual insights#
More on figures
🔼 Figure 3 shows examples from the WideRange4D dataset, demonstrating its diversity and complexity. It showcases various real-world and virtual scenes under different weather conditions (sunny, rainy, sandstorm). The scenes feature objects with a range of movement scales (long, medium, short), and different motion complexities (complex, slow, repetitive). This visualization highlights the breadth of WideRange4D’s scope and its ability to challenge existing 4D reconstruction methods.
read the caption
Figure 2: Exhibition of Testing Examples in WideRange4D.
🔼 Figure 3 presents a statistical analysis of the WideRange4D dataset, broken down into three subfigures. Figure 3(a) shows the distribution of location types (City, Road, Cartoon) and weather conditions (Sunny, Rainy, Sandstorm) within the dataset. Figure 3(b) displays the distribution of object categories, categorized by their size (Small, Medium, Large). Figure 3(c) illustrates the breakdown of motion types (In-place, Short-range, Medium-range, Long-range), categorized by their movement range.
read the caption
Figure 3: Statistical Distribution of WideRange4D.
🔼 This figure showcases various foreground objects included in the WideRange4D dataset. It visually demonstrates the diversity of objects in terms of size, shape, type (animals, humans, stylized objects), and style (realistic, cartoonish). The figure highlights the richness and variety of objects present in the dataset, which is crucial for evaluating the robustness and generalization capabilities of 4D reconstruction methods.
read the caption
Figure 4: Exhibition of foreground objects
🔼 This figure illustrates the pipeline for constructing the WideRange4D dataset. It begins with the integration of diverse 3D assets (humanoid models, animals, stylized characters) and the generation of corresponding motion data using techniques like animation retargeting and procedural path creation. Various environmental elements are then added, such as weather conditions (sunny, rainy, sandstorm), and virtual or real-world settings (cities, roads, cartoons). Finally, multiple cameras are virtually positioned to capture the scenes from different viewpoints, resulting in a multi-view video sequence dataset ideal for 4D reconstruction tasks.
read the caption
Figure 5: WideRange4D Construction Pipeline.
🔼 This figure compares the initialization of a 4D scene using two different methods: an existing method (4DGS) and the authors’ proposed method (Progress4D). When dealing with wide-range movements of objects within a 4D scene, existing methods often produce blurred or poorly defined foreground objects in the initial 3D reconstruction. This makes it extremely difficult to generate a high-quality 4D reconstruction in later processing steps. In contrast, the new method’s initialization shows clearer and more well-defined objects, even in scenes with significant movement.
read the caption
Figure 6: 4D scene initial comparison. The reason why existing methods fail to generate high-quality 4D scenes is that when a 4D scene involves wide-range variations, the initialized foreground objects become so blurred that they seem to vanish completely, making it difficult to achieve high-quality 4D synthesis in subsequent generation processes.
🔼 The figure shows the framework of the Progress4D method for 4D reconstruction. It’s a two-stage process. The first stage initializes a high-fidelity 3D scene using multi-view consistency. The second stage progressively fits the 4D dynamics using an MLP to estimate deformation fields (Δμ, ΔS, ΔR) at each timestep. A timestep alignment process is used, calculating weights based on temporal similarity and a motion mask, to help maintain stability and accuracy, particularly in scenes with wide-range movements. The overall loss function includes color loss, total variation loss, and the timestep alignment loss.
read the caption
Figure 7: Framework of our Progress4D.
🔼 This figure presents a qualitative comparison of 4D scene reconstruction results between the proposed Progress4D method and other state-of-the-art methods (SC4D, DreamScene4D, 4DGS, and ST-4DGS). It uses two examples, each showing ground truth, and the results of each method. For areas where the visual difference isn’t immediately clear, zoomed-in sections are provided to highlight subtle differences in detail and quality. This comparison showcases the superior performance of Progress4D in terms of generating more realistic and finely detailed 4D scenes, especially in areas with complex motion and intricate details, demonstrating the ability to capture fine-grained features and avoid artifacts.
read the caption
Figure 8: Qualitative comparison. For images that seem similar, we zoom in on the same regions in both our outputs and the comparison images to highlight the finer details of our Progress4D.
🔼 This ablation study analyzes the impact of the timestep alignment loss (Lalign) on the performance of the Progress4D method. The graph likely shows a comparison of the 4D reconstruction results with and without the Lalign component. The results would demonstrate how the inclusion of Lalign improves the quality and stability of 4D scene reconstruction, especially when dealing with complex scenes involving wide-range spatial movements. Specifically, it likely shows that without Lalign, the quality of the reconstruction degrades over time as the movement becomes more extensive. However, with Lalign, the quality remains consistently high, even for complex movements.
read the caption
Figure 9: Ablation study of timestep alignment loss.
🔼 This figure presents a qualitative comparison of 4D reconstruction results across different methods on three diverse scenes: Walk_Fox_City, Splitkick_Ancient_City, and Walkaround_Wolf_Cartoon. Each scene displays three viewpoints (VIEW I, VIEW II, VIEW III), showcasing the ground truth, 4DGS method results, and the proposed method’s results (Ours). It visually demonstrates the performance differences between the methods, particularly in handling complex motions and wide-range movements, highlighting the improvements achieved by the proposed approach in terms of visual quality and accuracy.
read the caption
Figure 10: Other Qualitative Comparison Results.
Full paper#
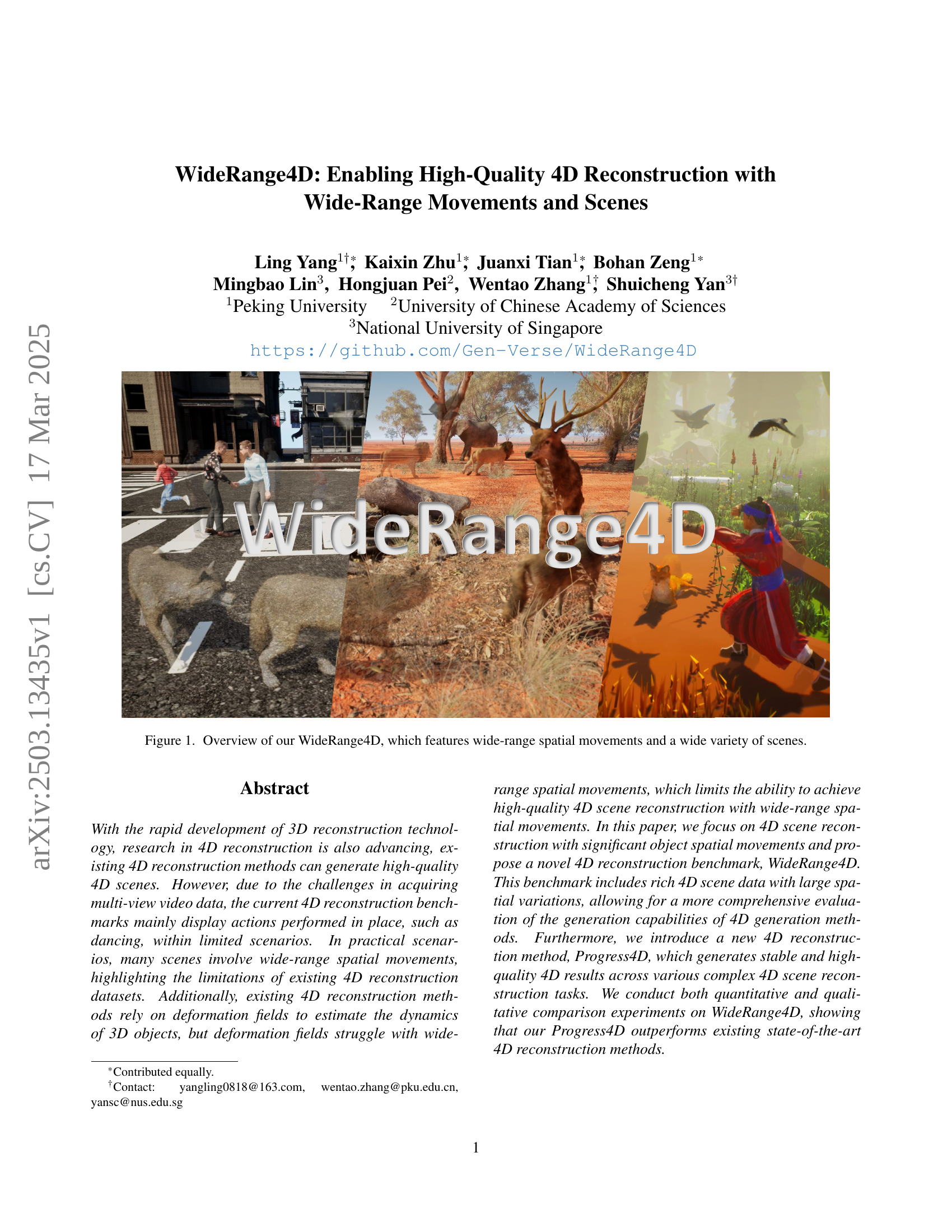
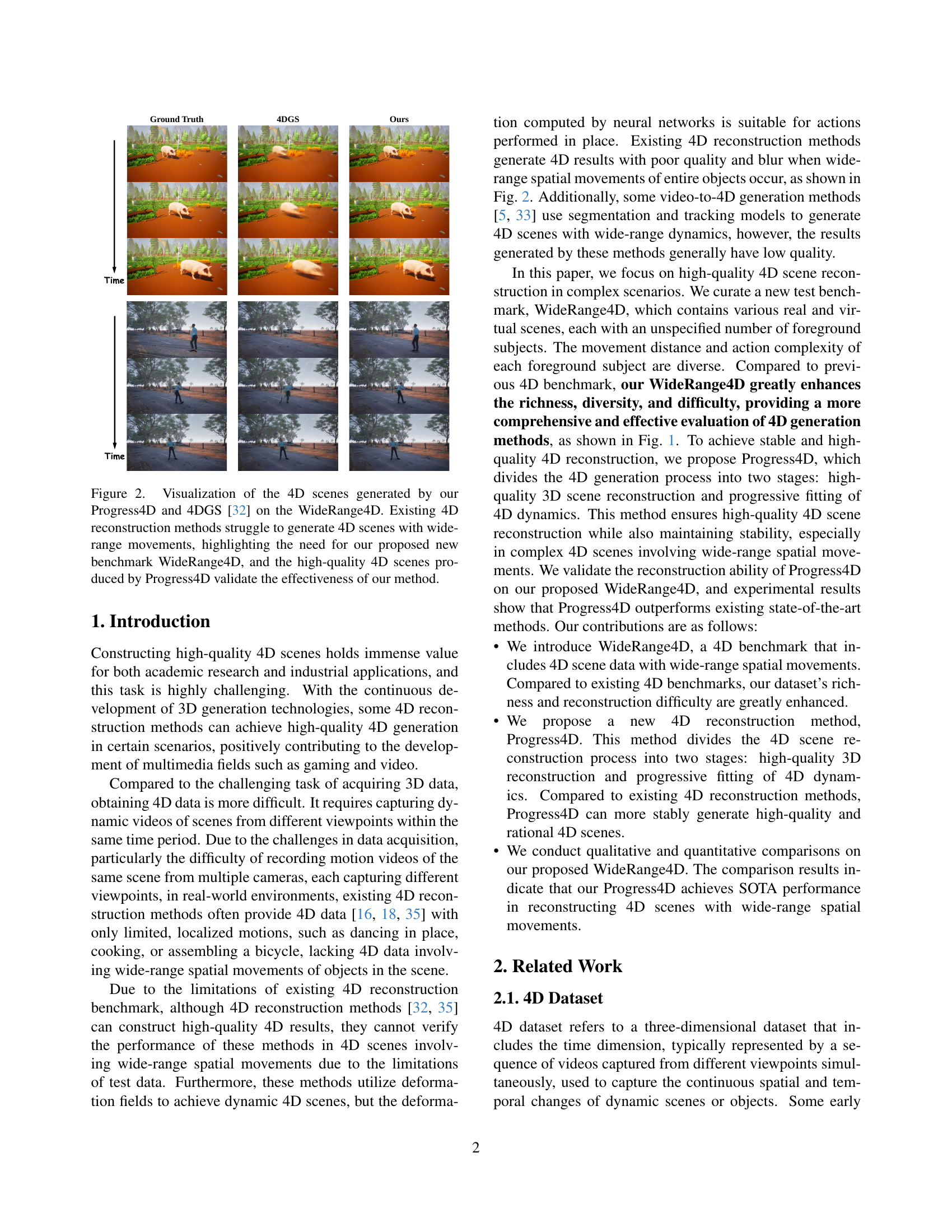
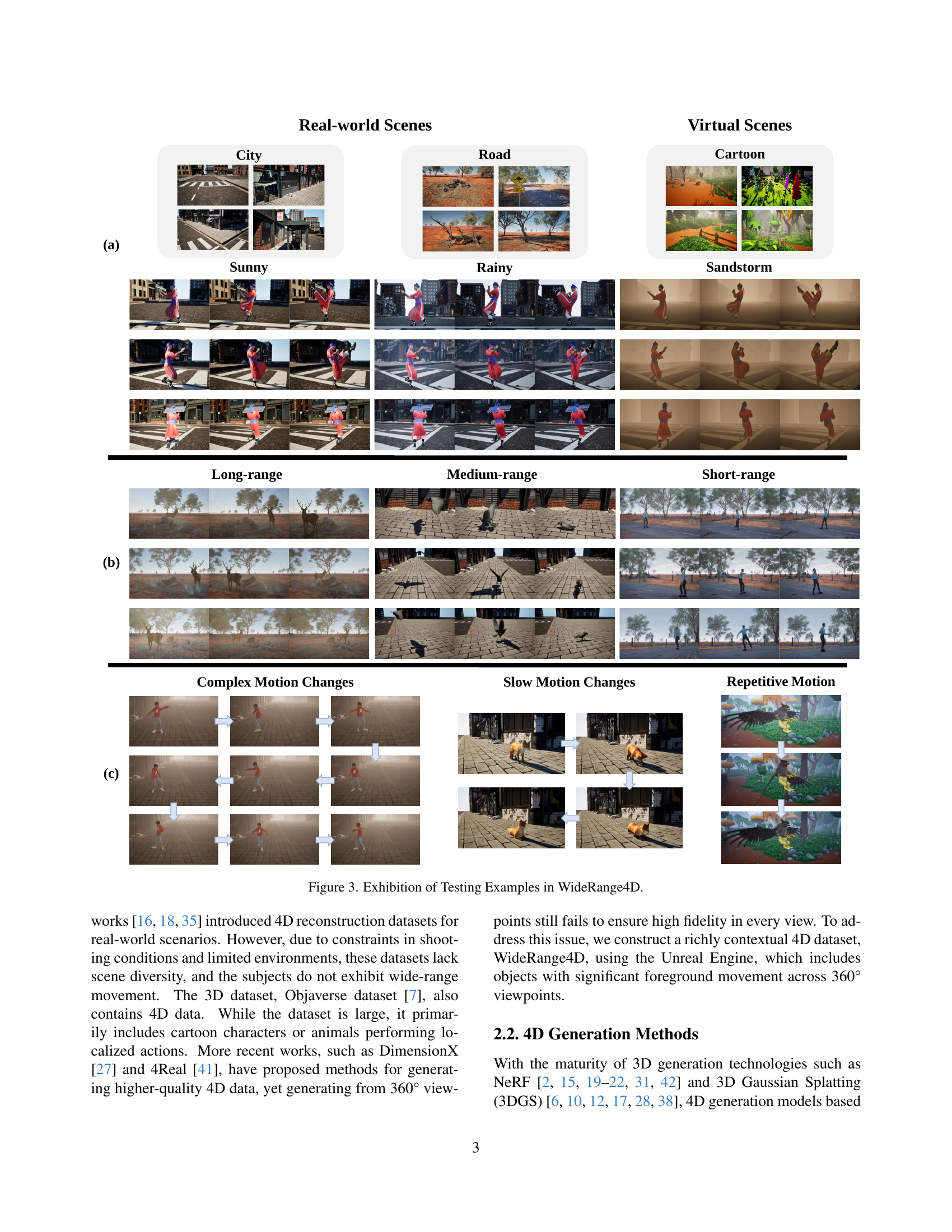
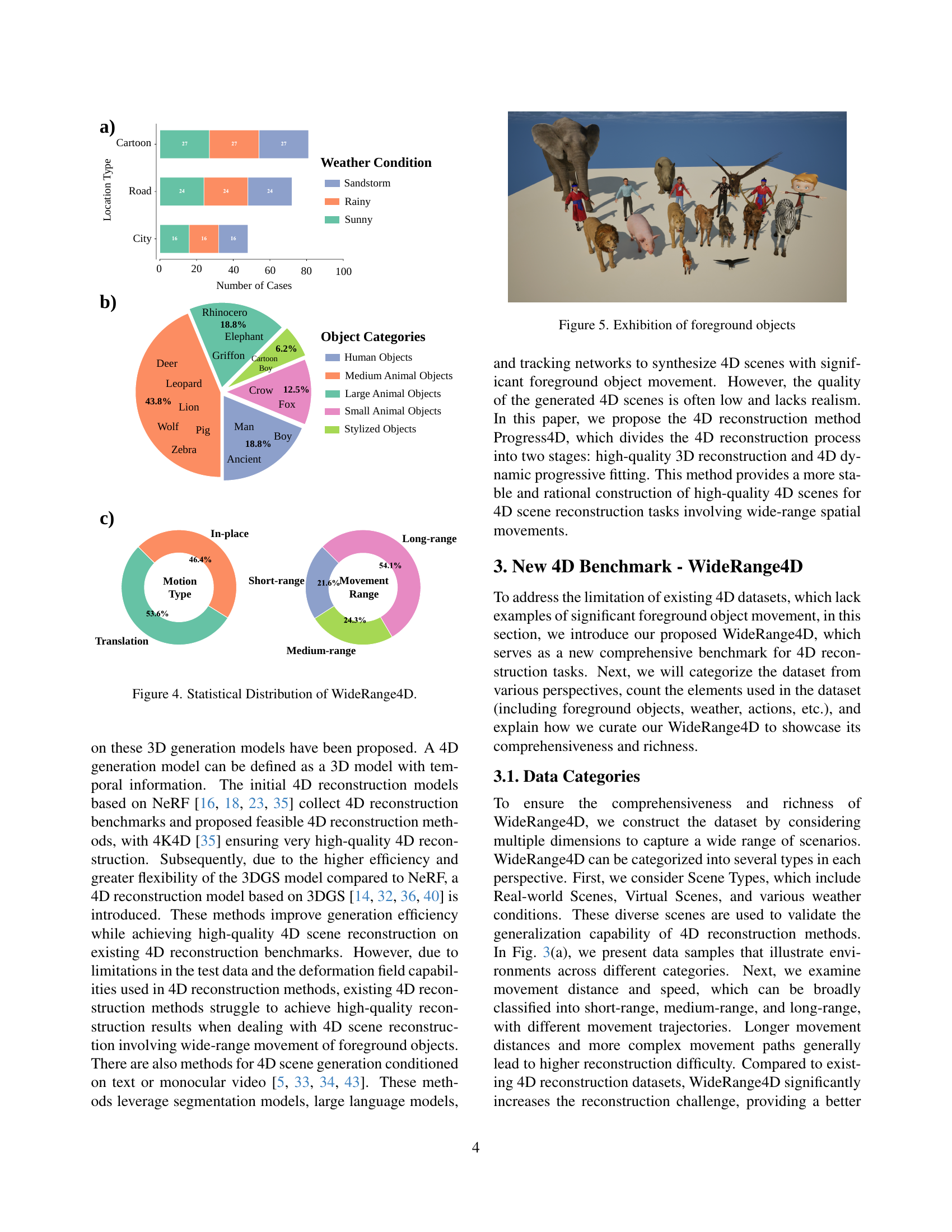
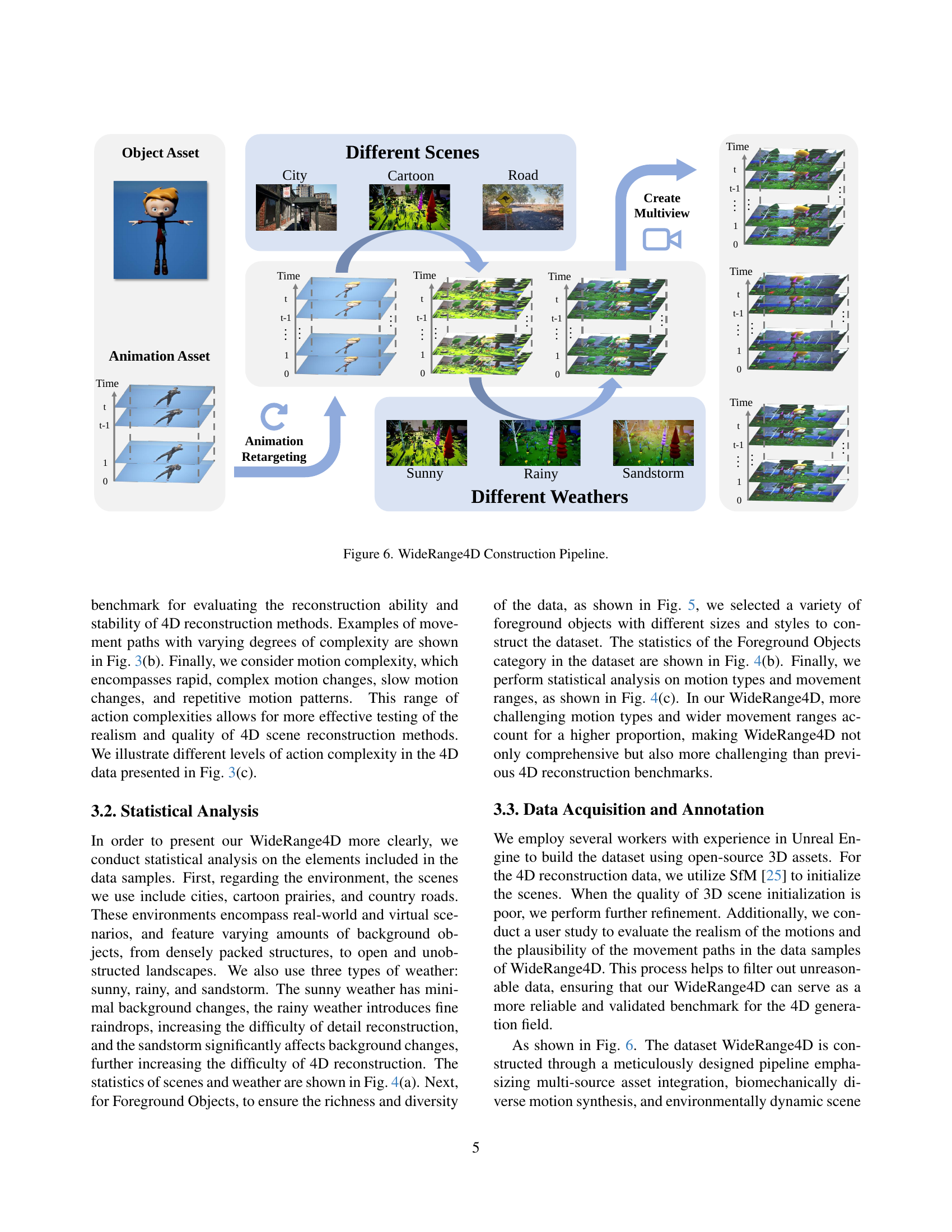
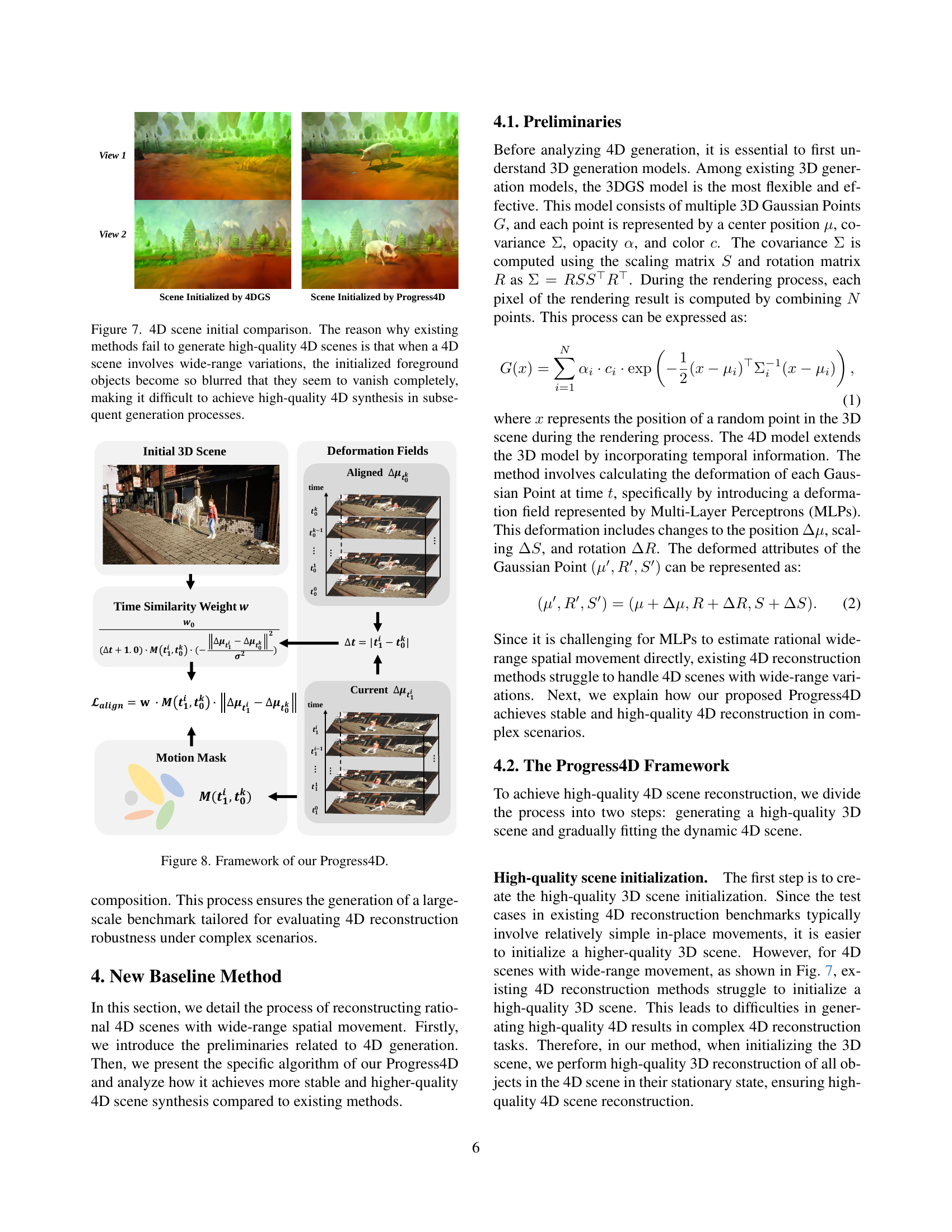
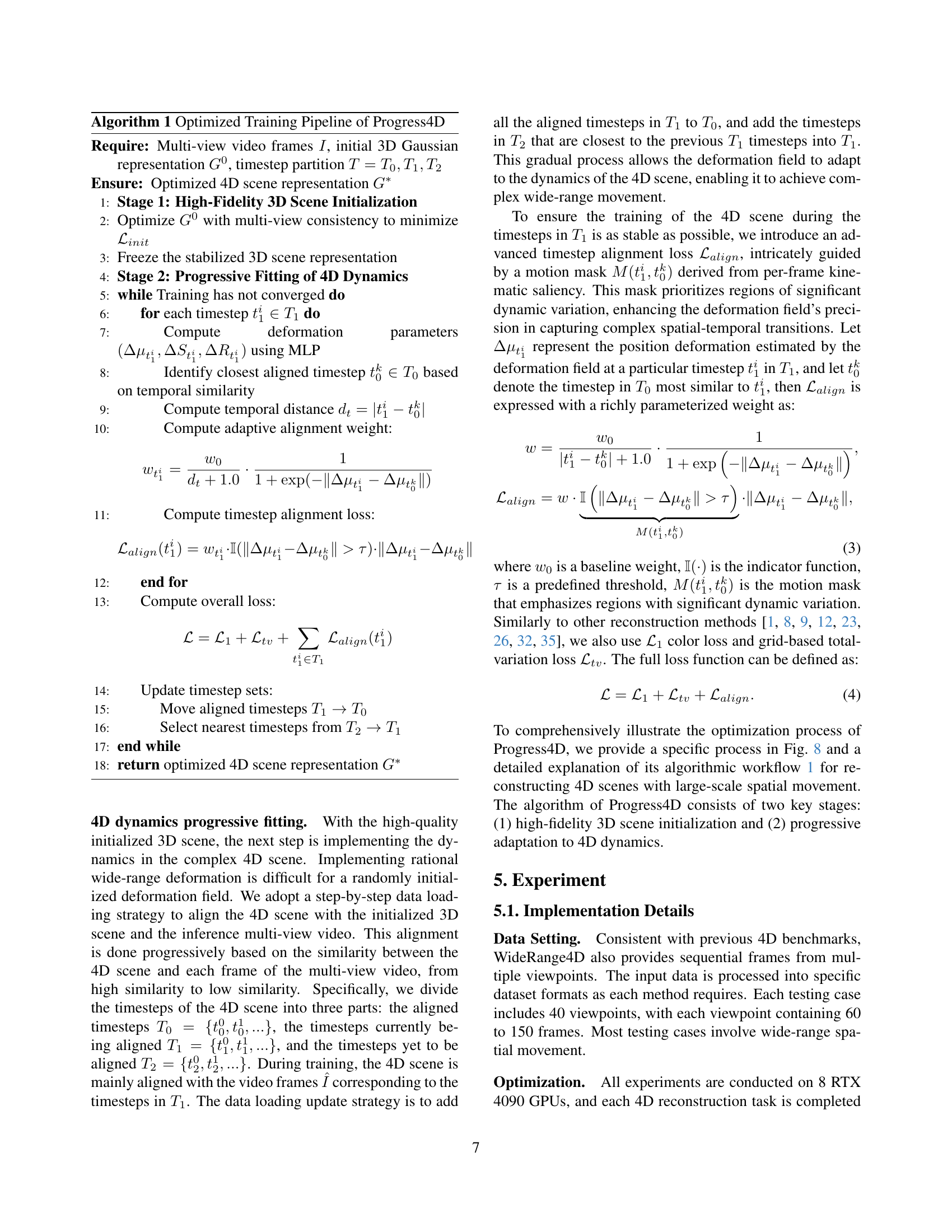
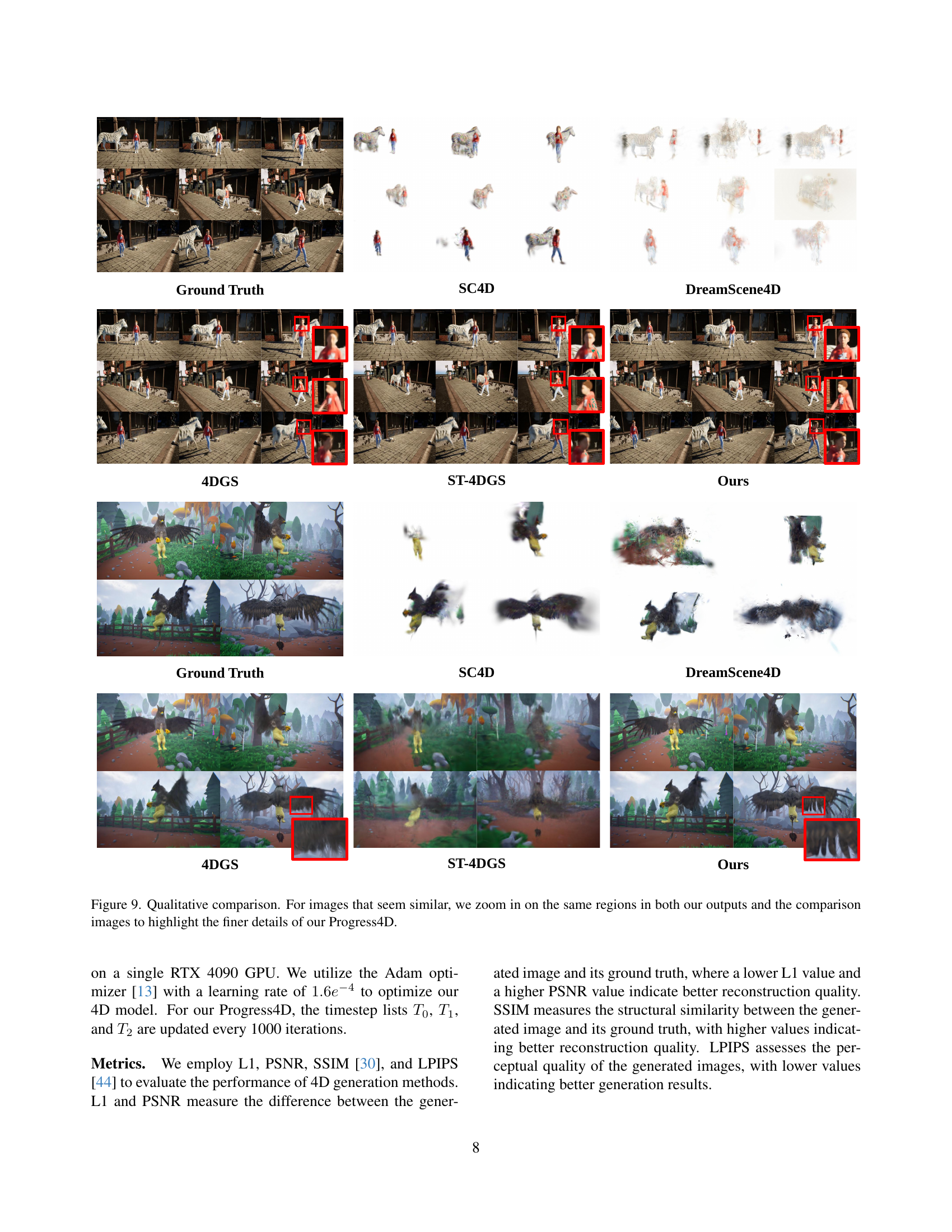
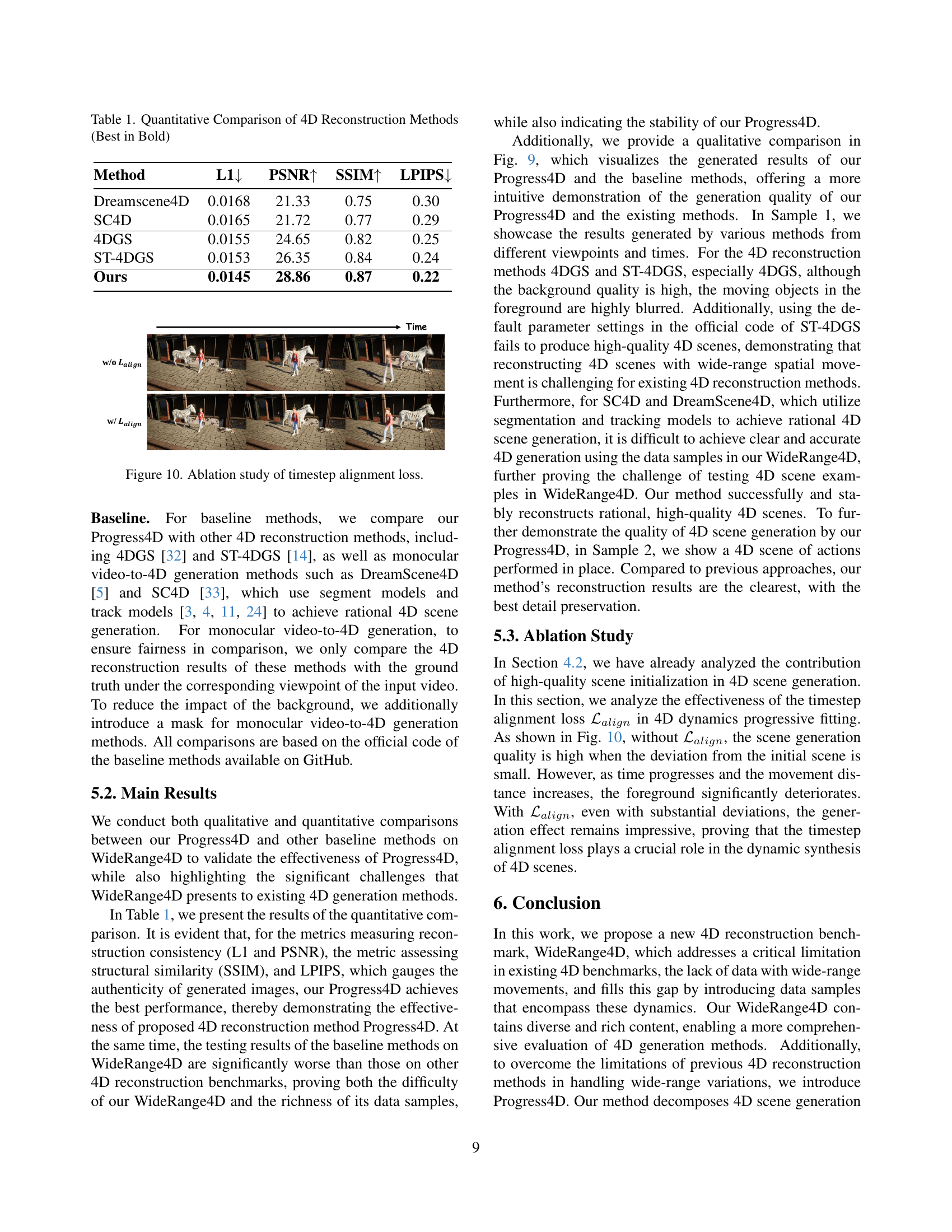
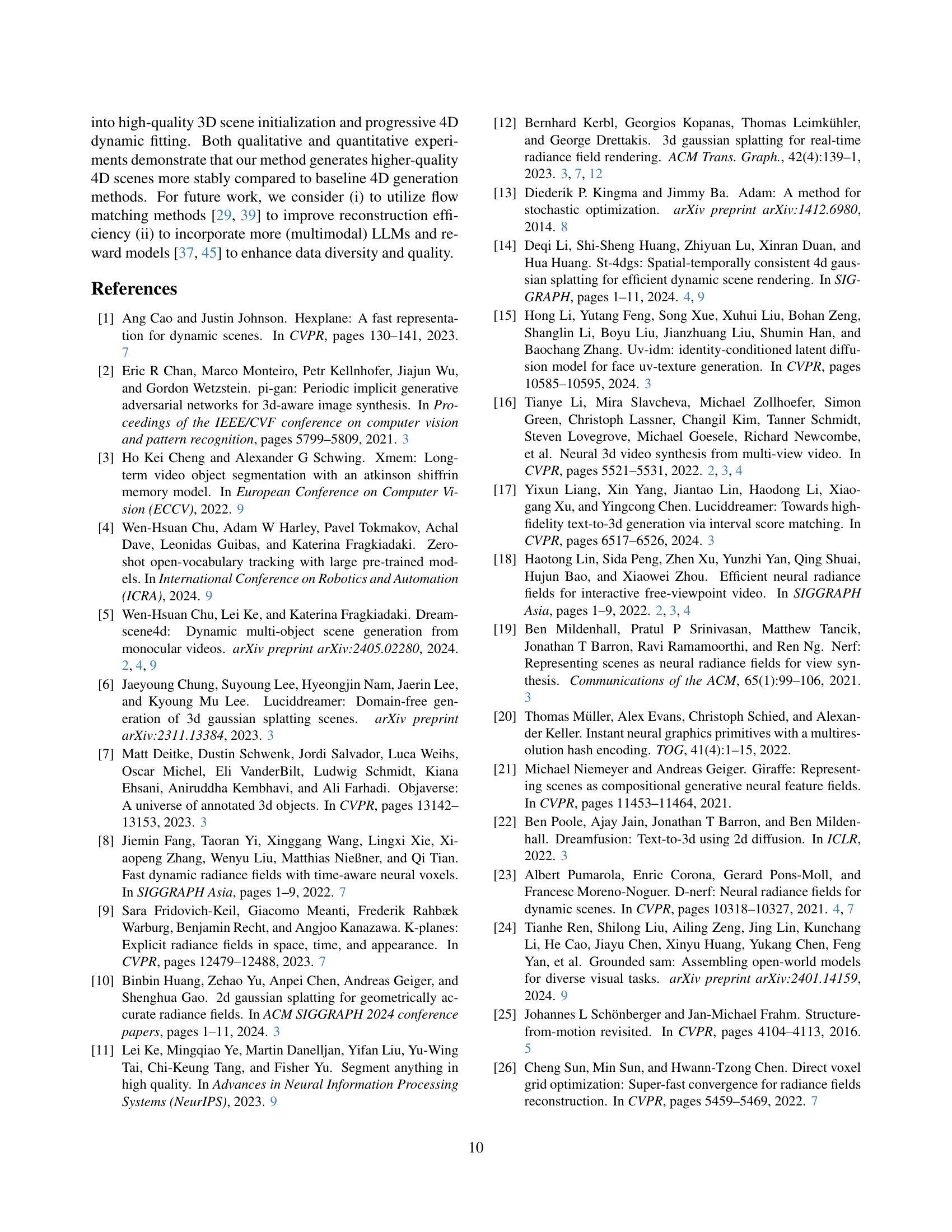
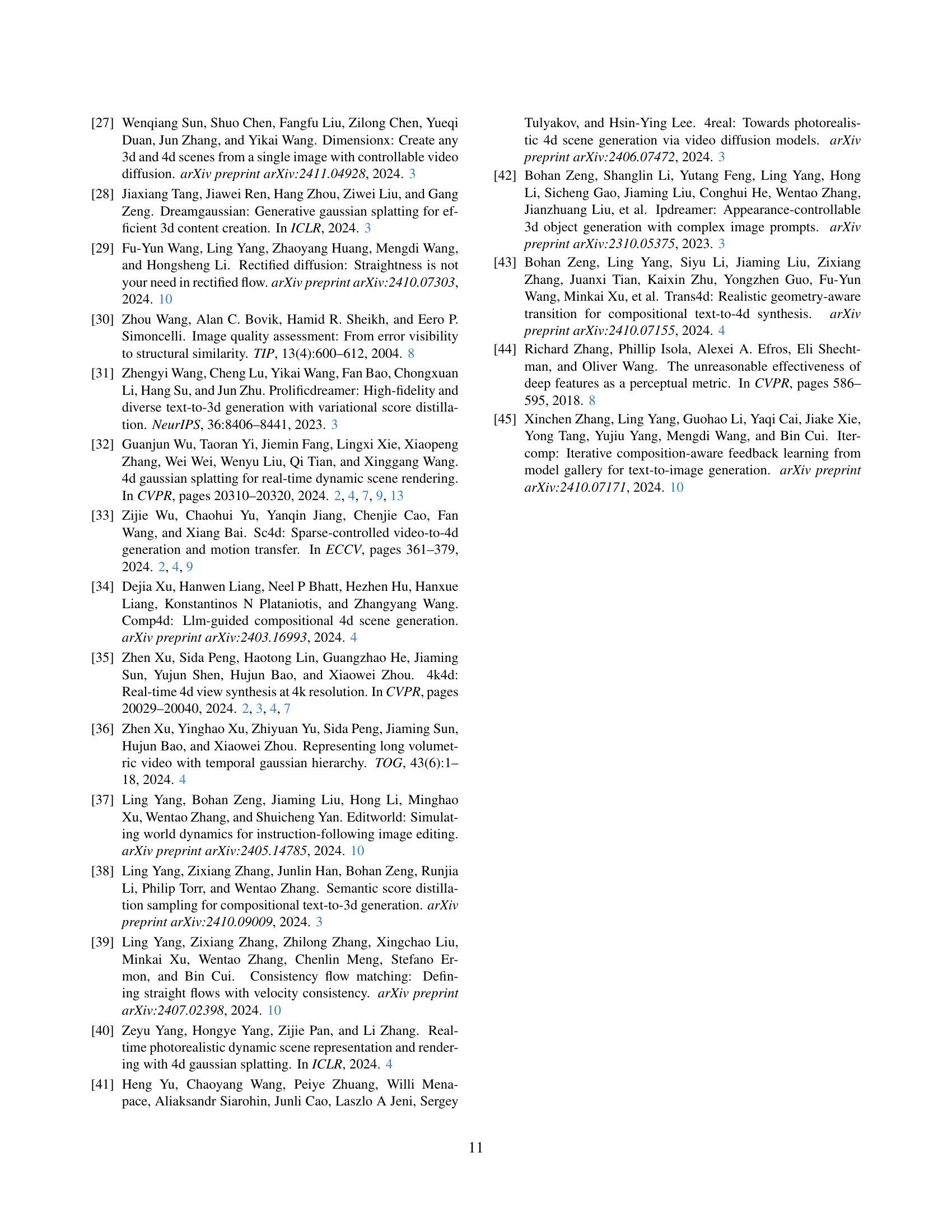
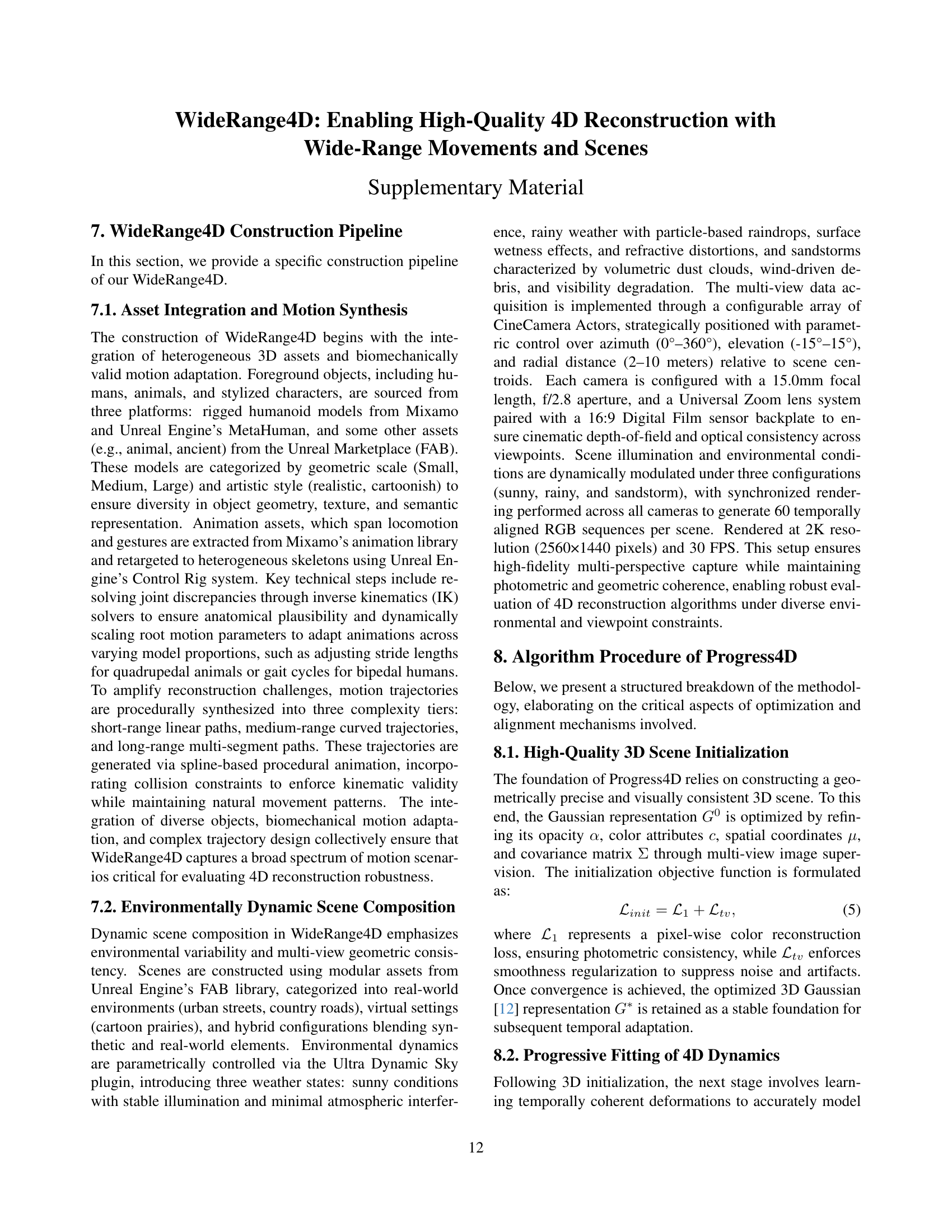
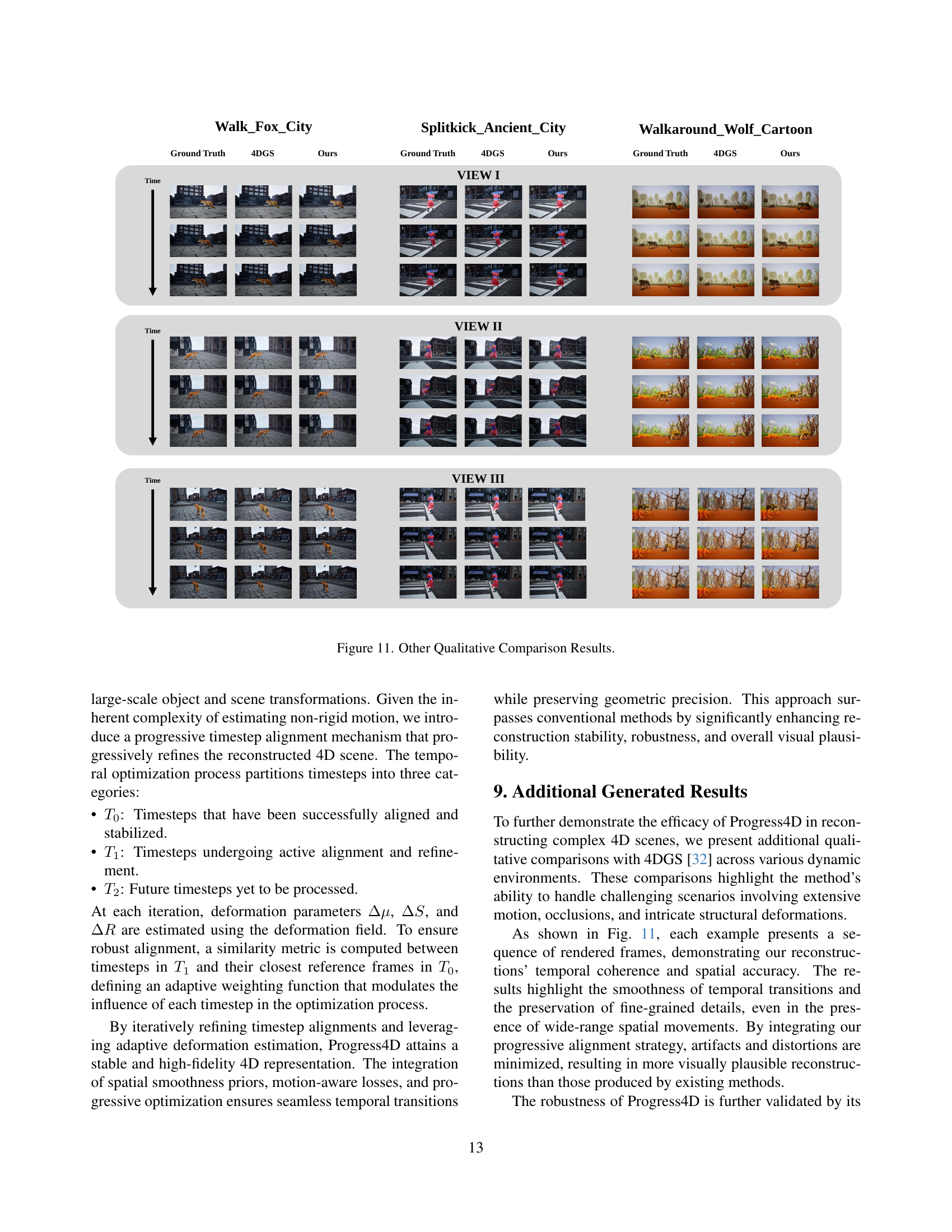
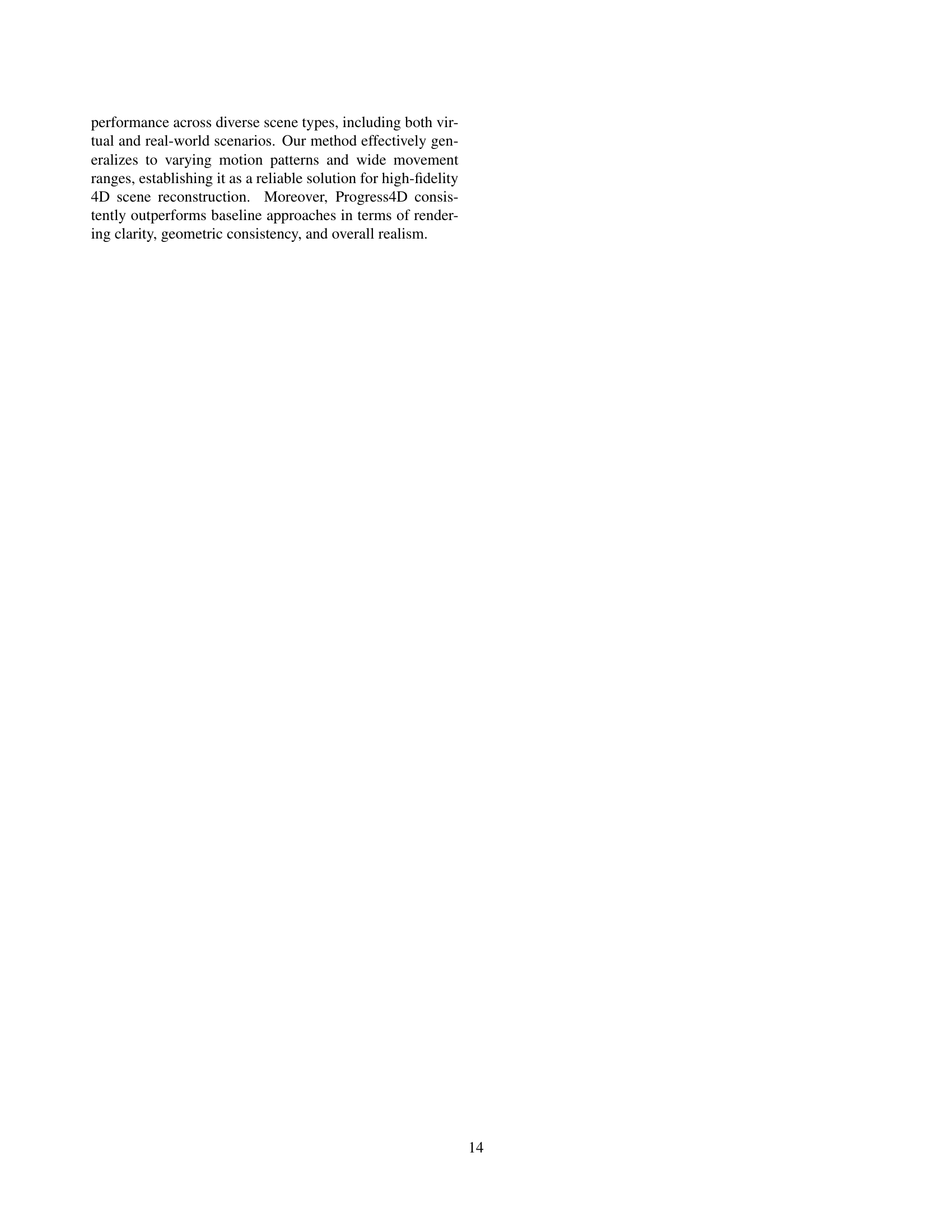