TL;DR#
Synthetic videos primarily replicate real-world scenarios, leaving impossible, counterfactual and anti-reality video concepts underexplored. This paper tries to answers: Can today’s video generation models effectively create impossible video content? Are today’s video understanding models good enough for understanding impossible videos? The authors introduce a benchmark IPV-BENCH designed to evaluate and foster progress in video understanding and generation.
To address the questions, the authors built a comprehensive taxonomy, encompassing 4 domains, 14 categories and constructed a prompt suite to evaluate video generation models, challenging their prompt following and creativity capabilities. They also curate a video benchmark to assess Video-LLMs on their ability of understanding impossible videos, which requires reasoning on temporal dynamics and world knowledge. This shows limitations and insights for future directions.
Key Takeaways#
Why does it matter?#
This paper introduces a novel and practical benchmark for evaluating video understanding and generation models. By focusing on impossible videos, the authors challenge models to move beyond memorization and demonstrate genuine reasoning. This work also highlight the limitations of existing models and inspire future research.
Visual Insights#
🔼 This figure showcases several examples of impossible videos generated by various AI models. Each example shows a short video clip alongside a description of the impossible event depicted, categorized by the type of impossible law or principle that is violated (e.g., physical, biological, geographical, social). This demonstrates the diversity and range of impossible video scenarios the authors explore in their work, highlighting how these scenarios defy common sense and the established laws of physics and nature.
read the caption
Figure 1: Impossible Video Examples with Impossible Type and Explanation.
Benchmark | Tasks | Video Data | Text Data | ||||||
AIGC Detection | Video Understanding | Video Generation | Real World Videos | Generated Videos | Impossible Videos | Text Prompts | Text Descriptions | Impossible Text | |
GenVideo (Chen et al., 2024a) | ✓ | ✗ | ✗ | ✓ | ✓ | ✗ | ✓ | ✗ | ✗ |
GenVidBench (Ni et al., 2025) | ✓ | ✗ | ✗ | ✓ | ✓ | ✗ | ✓ | ✗ | ✗ |
LOKI (Ye et al., 2024) | ✓ | ✓ | ✗ | ✓ | ✓ | ✗ | ✗ | ✓ | ✗ |
VBench (Huang et al., 2024) | ✗ | ✗ | ✓ | ✗ | ✗ | ✗ | ✓ | ✗ | ✗ |
VideoPhy (Bansal et al., 2024) | ✗ | ✗ | ✓ | ✗ | ✗ | ✗ | ✓ | ✗ | ✗ |
PhyGenBench (Meng et al., 2024a) | ✗ | ✗ | ✓ | ✗ | ✗ | ✗ | ✓ | ✗ | ✗ |
SEED-Bench (Li et al., 2023) | ✗ | ✓ | ✗ | ✓ | ✗ | ✗ | ✗ | ✓ | ✗ |
Video-Bench (Ning et al., 2023) | ✗ | ✓ | ✗ | ✓ | ✗ | ✗ | ✗ | ✓ | ✗ |
MV-Bench (Li et al., 2024) | ✗ | ✓ | ✗ | ✓ | ✗ | ✗ | ✗ | ✓ | ✗ |
TempCompass (Liu et al., 2024d) | ✗ | ✓ | ✗ | ✓ | ✗ | ✗ | ✗ | ✓ | ✗ |
IPV-Bench (Ours) | ✓ | ✓ | ✓ | ✓ | ✓ | ✓ | ✓ | ✓ | ✓ |
🔼 This table compares the IPV-Bench benchmark with other existing video benchmarks. It shows a feature comparison across several key aspects, such as the types of tasks supported (video understanding, generation, etc.), the types of video data used (real-world, generated, impossible videos), the availability of text data (prompts, descriptions, etc.), and the overall focus of each benchmark.
read the caption
Table 1: Comparison of IPV-Bench and Existing Benchmarks.
In-depth insights#
Impossibility AI#
Impossibility AI explores the realm of artificially generated scenarios that defy the known laws of nature, social norms, or common sense. This emerging field presents challenges and opportunities for both AI video generation and understanding. For generation, it pushes models beyond simple replication towards demonstrating true creativity and flexible prompt adherence, needing to “break the rules” while staying contextually relevant. For understanding, it requires more advanced reasoning about temporal dynamics and real-world knowledge, going beyond mere object recognition to discern what is fundamentally impossible, thereby enabling sophisticated error detection and model robustness. The creation and analysis of such impossible videos could significantly advance AI’s comprehension of the world, moving from pattern matching to deeper causal understanding. The field also touches on broader implications around AI safety and ethical considerations as models become capable of generating increasingly deceptive or misleading content.
IPV-BENCH#
IPV-BENCH, as introduced in this paper, represents a novel and significant contribution to the fields of video understanding and generation. It addresses a critical gap in existing benchmarks by focusing on impossible videos, which require models to move beyond mere memorization of real-world scenarios. The construction of IPV-BENCH is underpinned by a comprehensive taxonomy, encompassing physical, biological, geographical, and social laws, allowing for the systematic categorization of impossible scenes. The benchmark includes both a text prompt suite (IPV-TXT) and a high-quality video dataset (IPV-VID), enabling thorough evaluation of video generation models’ ability to follow prompts and creativity, as well as video understanding models’ capacity to reason about temporal dynamics and world knowledge. The rigorous evaluation protocols, including tasks like multiple-choice question answering and open-ended question answering, provide valuable insights into the limitations of current models and pave the way for future research directions. Furthermore, the emphasis on temporal dynamics sets IPV-BENCH apart, challenging models to understand how impossible events unfold over time, rather than relying solely on static image analysis.
Video-LLM Limits#
Video-LLMs, while promising, likely face limitations in truly understanding impossible videos. They might struggle with temporal dynamics, failing to connect events across frames to detect violations of physics or logic. Their world knowledge, crucial for identifying anomalies like snow in Singapore, could be incomplete or biased. The models might over-rely on memorized patterns, missing subtle impossibilities that require deeper reasoning. Furthermore, current architectures may lack the sophisticated temporal modules needed to process and understand complex, time-dependent violations. Addressing these limits requires novel architectures and training strategies focused on enhancing temporal reasoning and integrating comprehensive world knowledge.
Prompt Creative#
While the paper does not explicitly have a section labeled ‘Prompt Creative’, we can infer its importance based on the context of impossible video generation. The creation of effective prompts is critical for guiding models to generate counterfactual and anti-reality scenes. These prompts must go beyond simple scene descriptions and actively encourage violations of physical, biological, geographical, or social laws. A successful ‘Prompt Creative’ strategy would involve innovative use of language to push the boundaries of model’s understanding and creative capabilities, resulting in videos that are both visually compelling and conceptually impossible. This may involve carefully wording the prompts to set up the desired counterfactual scenario, and provide guidance for the specific violation of the rules.
Reasoning Needed#
Reasoning is paramount for identifying impossible scenarios, going beyond mere object recognition. Temporal dynamics and world knowledge are crucial elements. Many video models excel in processing spatial information but struggle with temporal reasoning, especially on fast-paced or unusual events. A robust video understanding model must not only identify objects but also infer relationships and predict their evolution over time. This requires integrating prior knowledge about how objects normally behave and applying logical reasoning to detect deviations from expected norms. Furthermore, identifying impossible scenarios often necessitates considering contextual cues and drawing inferences from multiple frames. Current models often fall short in seamlessly integrating these diverse sources of information, leading to a reliance on lower level pattern matching. Ultimately, the ability to reason about impossible videos reflects a model’s capacity for high-level understanding and flexible adaptation to novel situations.
More visual insights#
More on figures
🔼 The figure illustrates the architecture of the IPV-Bench benchmark, a novel dataset designed to evaluate video generation and understanding models’ capabilities in handling impossible videos. The benchmark is built upon a comprehensive taxonomy categorizing impossible scenarios across four domains: Physical, Biological, Geographical, and Social laws. This taxonomy facilitates the creation of two key components: a diverse prompt suite (IPV-Txt) which includes various text descriptions of impossible events, and a high-quality video dataset (IPV-Vid) that visually represents these events. The integration of these components allows for a thorough evaluation of models on both their ability to generate such videos and to understand their content.
read the caption
Figure 2: Overview of the IPV-Bench Benchmark. IPV-Bench is structured with a comprehensive taxonomy, enabling the creation of a diverse prompt suite (IPV-Txt) and a high-quality video dataset (IPV-Vid). These components facilitate the evaluation of popular video generation and understanding models.
🔼 This figure shows the questionnaire used to collect impossible video scenarios for the IPV-TXT benchmark. The questionnaire guides participants to brainstorm dynamic video scenarios that are impossible or extremely difficult to achieve in the real world. It emphasizes the importance of dynamic scenes (not static), the use of common, everyday objects for creative and unexpected scenarios, and the consideration of scenarios violating social norms, expectations, or physical laws. Participants are explicitly instructed not to use large language models like ChatGPT during the brainstorming process.
read the caption
Figure 3: Questionnaire used for collecting impossible text prompts for IPV-Txt.
🔼 This figure visualizes the distribution of prompts within the IPV-TXT benchmark across its taxonomy. The taxonomy categorizes impossible scenarios into four main categories: Physical Laws, Biological Laws, Geographical Laws, and Social Laws. Each category is further divided into sub-categories. The figure shows the number or proportion of prompts belonging to each category and sub-category, providing a clear overview of the benchmark’s coverage across different types of impossible scenarios.
read the caption
Figure 4: Distribution of the Prompt Suite Across the Taxonomy.
🔼 Figure 5 is a bar chart illustrating the sources of the impossible videos used in the IPV-VID dataset. It shows the relative contribution of various sources, including AI model outputs (Sora, Kling, Hailuo, Luma, Mochi1, PyramidFlow, HunyuanVideo, Open-Sora, CogVidX, and LTX), and internet-sourced videos. This visualization helps to understand the dataset’s composition and the diversity of methods employed in generating the impossible video samples.
read the caption
Figure 5: Sources of Impossible Videos.
🔼 This figure showcases two primary failure modes in generating impossible videos. The first example depicts a failure in visual quality, where attempting to generate impossible scenes results in visual artifacts or generation failures, likely due to the impossible prompts representing out-of-distribution data for the model. The second example highlights a failure to adhere to impossible prompts, where videos capture semantic aspects but not the crucial impossible events, likely due to an overemphasis on conforming to real-world physical laws. These examples illustrate limitations of current video generation models in handling impossible scenarios.
read the caption
Figure 6: Failure case of impossible video generation.
🔼 This figure shows an example of the multiple choice question answering (MCQA) task used in the Impossible Video Understanding section of the paper. It displays a video still along with a multiple choice question and its options. The correct answer is highlighted in red, and one incorrect option is highlighted in green. The MCQA task assesses a model’s ability to correctly identify the impossible phenomenon depicted in a video by choosing from several plausible options. The distractors (incorrect options) are carefully designed to challenge the model’s reasoning abilities, ensuring that simple visual element grounding is not sufficient to arrive at the correct response.
read the caption
Figure 7: Example of the MCQA task. We highlight the correct option in red and the incorrect option in green.
🔼 This figure showcases an example of the open-ended question answering (OpenQA) task within the Impossible Video Understanding section of the paper. The task challenges video understanding models to determine if a given video depicts an impossible event. The figure presents a video alongside multiple model responses, differentiating between correct and incorrect answers using color-coding (red for correct, green for incorrect). Each response highlights the model’s reasoning behind its classification.
read the caption
Figure 8: Example of the OpenQA task. We ask state-of-the-art video understanding models to analyze whether the video is impossible or not. We highlight the correct analysis in red and the incorrect analysis in green.
🔼 The figure displays a screenshot of the video annotation tool used in the IPV-BENCH benchmark. The tool is divided into two main sections: the ‘Display Zone’ which shows the video being annotated, the associated text prompt describing the impossible scenario, and the taxonomy label categorizing the type of impossibility (e.g., physical, biological, geographical, social laws). The ‘Annotation Zone’ presents a structured form for annotators to provide various labels including: visual quality assessment, prompt following accuracy, whether the impossibility is evident in a single frame or requires temporal analysis, and a textual explanation of the impossible scenario shown in the video. This tool facilitates efficient and consistent annotation for the dataset.
read the caption
Figure 9: Screenshot of the annotation tool.
🔼 This figure displays the correlation between automatic and human evaluations of impossible video generation. Spearman’s correlation coefficient (ρ) is calculated and shown for each of ten different video generation models across three metrics: visual quality, prompt following, and the combined IPV (Impossible Video) score. The high correlation coefficients indicate a strong agreement between automatic and human evaluation, demonstrating the reliability of the automated assessment method.
read the caption
Figure 10: Spearman’s correlation coefficient ρ𝜌\rhoitalic_ρ between automatic evaluation score and human annotation score on 10 different video generation models.
🔼 This figure presents the detailed instructions given to the large language model (LLM) for generating multiple-choice questions (MCQs) for evaluating video understanding models. The instructions emphasize creating high-quality distractors that challenge the model’s ability to distinguish between correct and incorrect answers. These instructions focus on various aspects to make the distractors realistic and difficult: diverse objects and attributes in the video, including details about objects and their properties. These instructions also include guidelines for creating distractors based on impossible phenomenons not explicitly shown in the video. The goal is to test the model’s ability to reason about counterfactual events and understand the content of the video instead of just relying on surface-level visual information.
read the caption
Figure 11: Instructional Prompt of MCQA Task.
🔼 Figure 12 shows examples of chain-of-thought (CoT) prompts used to evaluate the ability of GPT-4 to assess whether video generation models correctly follow impossible prompts. The prompts instruct GPT-4 to analyze video frames and determine if they accurately depict the impossible event described in the text prompt. Crucially, the instructions emphasize that GPT-4 should only focus on the specific impossible event and disregard other factors like video quality. The examples highlight GPT-4’s reasoning process by showing how it identifies the key elements of the impossible event, checks for their presence in the video, and then provides a [Yes] or [No] conclusion. It’s important to note that videos might include additional impossible events not mentioned in the prompt; these events are to be ignored in the evaluation.
read the caption
Figure 12: CoT examples we use to prompt GPT-4o for impossible prompt following evaluation. Videos may contain impossible events that are outside the scope of the prompt. We ignore such events when evaluating impossible prompt following.
🔼 Figure 13 presents a collection of diverse impossible video examples. Each example showcases a scene that violates established physical, biological, geographical, or social laws. These examples are visually rich and highlight the varied ways in which a video can depict an impossible scenario, including changes in material properties (paper turning to smoke), violations of mechanics (a car flying), optical illusions (a reflection mismatch), and more.
read the caption
Figure 13: More examples of impossible videos.
🔼 This figure showcases examples where video generation models fail to accurately create impossible scenarios. Each row presents a different type of impossible event and shows how the model’s output deviates from the intended result. The deviations highlight challenges models face when generating scenarios that defy established laws of physics, biology, geography, or social norms.
read the caption
Figure 14: Failures in Generating Impossible Videos.
🔼 This figure presents a case study illustrating the challenges faced by video understanding models when dealing with impossible videos. The figure showcases several video understanding models’ responses to a video depicting a person seemingly biting into a piece of bread, only for the bread to vanish. The responses highlight the varying degrees of understanding, from correctly identifying the impossibility to providing plausible but incorrect explanations.
read the caption
Figure 15: Case study of impossible video understanding.
🔼 This figure shows a case study of a video which is impossible in the real world. The video shows two identical bottles of water, one frozen and one not. Different video understanding models interpret this in various ways; some correctly identify the impossibility (violating expectations of phase transition), others fail to recognize the impossibility, and still others focus on seemingly secondary aspects of the video (e.g., the unnatural shaking of the bottles).
read the caption
Figure 16: Case study of impossible video understanding.
🔼 This figure presents a case study illustrating the challenges in impossible video understanding. It shows a video depicting a person pouring liquid into a container filled with sand. Several video understanding models are evaluated on their ability to identify what makes this video impossible or unusual in a real-world context. The responses from different models highlight varying levels of understanding, ranging from correctly identifying the impossible event (the sand not absorbing water) to incorrectly identifying other aspects of the scene as unusual or impossible. This case study emphasizes the difficulty that current models face in reasoning about counterfactual or physically impossible events shown in video.
read the caption
Figure 17: Case study of impossible video understanding.
🔼 This figure shows a case study of impossible video understanding. The task is to identify what makes the video impossible or unusual in a real-world setting. Different video understanding models provide their answers, revealing their capabilities and limitations in understanding situations that defy real-world physics or common sense. The example video shows a tomato being cut, but the cut is not consistent with how a real tomato would be cut. Models’ answers reveal various levels of comprehension of the impossible phenomenon.
read the caption
Figure 18: Case study of impossible video understanding.
🔼 This figure presents a case study illustrating the challenges video understanding models face when dealing with impossible videos. The example shows a book seemingly opening and closing on its own without external interaction. The responses of various video-language models to this impossible event are analyzed, highlighting their limitations in reasoning about impossible scenarios and applying world knowledge to video analysis.
read the caption
Figure 19: Case study of impossible video understanding.
More on tables
Model | Physical | Biological | Social | Geographical | Overall | ||||||
Visual | Prompt | Visual | Prompt | Visual | Prompt | Visual | Prompt | Visual | Prompt | IPV | |
Quality | Following | Quality | Following | Quality | Following | Quality | Following | Quality | Following | Score | |
Open-source Models | |||||||||||
LTX (HaCohen et al., 2024) | 58.3 | 14.1 | 35.3 | 21.6 | 44.0 | 16.0 | 57.1 | 23.8 | 52.3 | 16.5 | 10.0 |
Open-Sora (Zheng et al., 2024a) | 63.8 | 22.1 | 25.5 | 29.4 | 20.0 | 32.0 | 57.1 | 47.6 | 51.5 | 26.5 | 15.8 |
Pyramid-Flow (Jin et al., 2024) | 88.3 | 15.3 | 92.2 | 21.6 | 72.0 | 28.0 | 100.0 | 52.4 | 88.5 | 20.8 | 19.6 |
CogVidX-1.5 (Yang et al., 2024b) | 38.7 | 29.4 | 35.3 | 52.9 | 40.0 | 56.0 | 81.0 | 61.9 | 41.5 | 39.2 | 16.9 |
Mochi 1 (GenmoAI, 2025) | 68.7 | 44.2 | 64.7 | 56.6 | 80.0 | 60.0 | 71.4 | 76.2 | 69.2 | 50.8 | 37.3 |
HunyuanVid (Kong et al., 2024) | 95.1 | 23.9 | 88.2 | 35.3 | 88.0 | 40.0 | 90.5 | 42.9 | 92.7 | 29.2 | 26.2 |
Proprietary Models | |||||||||||
Luma (LumaLabs, 2025) | 88.3 | 11.7 | 90.2 | 19.6 | 82.6 | 17.4 | 85.7 | 52.4 | 88.0 | 17.1 | 14.3 |
Sora (OpenAI, 2025) | 98.8 | 15.3 | 98.0 | 43.1 | 100.0 | 30.4 | 95.2 | 61.9 | 98.4 | 26.0 | 25.2 |
Kling (KLING, 2025) | 98.8 | 21.5 | 94.1 | 33.3 | 95.7 | 43.5 | 81.0 | 42.9 | 96.1 | 27.5 | 26.7 |
Hailuo (Hailuo, 2025) | 96.3 | 30.1 | 94.1 | 45.1 | 100.0 | 52.0 | 90.5 | 61.9 | 95.8 | 37.7 | 36.2 |
🔼 Table 2 presents a comprehensive comparison of the performance of various state-of-the-art video generation models. These models were evaluated using the IPV-TXT benchmark, a collection of text prompts designed to generate impossible videos. The table details the model scores across several dimensions: visual quality, prompt following ability in various impossible scenarios (Physical, Biological, Social, Geographical), and an overall score. Higher scores indicate better performance. The best-performing model in each dimension is bolded, and the second-best is underlined.
read the caption
Table 2: Evaluation Results of IPV-Txt Across Dimensions. This table compares the performance of state-of-the-art video generation models using the IPV-Txt benchmark as text prompts in the T2V setting. A higher score indicates better performance in a given dimension. Bold denotes best, underline denotes second.
Model | Judgement | Physical | Biological | Social | Geographical | Overall | |||||||
Acc. | F1 | MC | Open | MC | Open | MC | Open | MC | Open | MC | Open | Open(C) | |
Open-source Models | |||||||||||||
Random | 50.0 | 50.0 | 20.0 | - | 20.0 | - | 20.0 | - | 20.0 | - | 20.0 | - | - |
Human | - | - | - | - | - | - | - | - | - | - | 94.0 | 82.7 | - |
Video-LLaVA (Lin et al., 2023a) | 72.7 | 72.9 | 23.0 | 13.3 | 34.6 | 29.7 | 31.8 | 16.9 | 24.1 | 25.9 | 26.8 | 14.2 | 18.7 |
Oryx (Liu et al., 2024e) | 58.6 | 44.6 | 52.0 | 16.7 | 68.8 | 33.9 | 70.3 | 32.6 | 83.9 | 35.2 | 60.4 | 18.7 | 22.7 |
Intern-VL-2.5 (Chen et al., 2024b) | 56.5 | 69.7 | 61.9 | 34.9 | 64.2 | 59.6 | 65.5 | 49.6 | 77.0 | 48.4 | 62.4 | 33.0 | 32.5 |
NVILA (Liu et al., 2024f) | 72.6 | 64.0 | 60.2 | 26.4 | 63.3 | 52.3 | 68.9 | 36.6 | 69.0 | 42.9 | 62.6 | 26.8 | 30.6 |
LongVU (Shen et al., 2024) | 70.3 | 68.0 | 69.3 | 21.8 | 79.6 | 38.3 | 77.0 | 35.6 | 77.0 | 35.6 | 73.3 | 21.9 | 25.4 |
Qwen2-VL (Wang et al., 2024) | 76.2 | 71.1 | 69.1 | 32.8 | 75.8 | 56.3 | 75.0 | 48.3 | 75.9 | 53.0 | 71.4 | 31.7 | 33.7 |
LLaVA-Next (Liu et al., 2024a) | 73.2 | 70.4 | 82.8 | 37.7 | 92.9 | 57.2 | 90.5 | 51.3 | 90.8 | 51.4 | 86.4 | 34.4 | 38.6 |
Proprietary Models | |||||||||||||
Gemini-1.5-Flash (Team et al., 2024) | 73.1 | 64.0 | 80.5 | 48.5 | 90.8 | 66.4 | 89.2 | 59.3 | 93.1 | 65.3 | 84.4 | 42.5 | 36.8 |
GPT-4o (Hurst et al., 2024) | - | - | 76.7 | 58.2 | 83.8 | 75.5 | 84.5 | 64.9 | 92.0 | 71.1 | 79.7 | 49.1 | 45.1 |
🔼 Table 3 presents a comprehensive evaluation of state-of-the-art (SOTA) Video Large Language Models (Video-LLMs) on the Impossible Video Understanding task using the IPV-Vid benchmark. The table compares the performance across multiple dimensions, including accuracy and F1-score on a Judgment task, as well as performance on Multi-Choice Question Answering (MCQA) and Open-Ended Question Answering (OpenQA) tasks. For each task and model, the table presents scores broken down by video category (Physical, Biological, Social, Geographical). Results are shown for both open-source and proprietary Video-LLMs. A higher score indicates better performance, with the best score in each category bolded and the second-best underlined. Note that results for the OpenQA task include separate scores obtained using two different evaluators: GPT-40 and Claude.
read the caption
Table 3: Evaluation Results for Impossible Video Understanding. This table compares the performance of sota VideoLLMs using the IPV-Vid benchmark. A higher score indicates better performance in a given dimension. Bold denotes best, underline denotes second, Open(C) denotes using Claude as the evaluator.
Model | Spatial | Temporal | ||
MC | Open | MC | Open | |
Open-source Models | ||||
Random | 20.0 | - | 20.0 | - |
Video-LLaVA (Lin et al., 2023a) | 34.6 | 30.3 | 23.8 | 13.7 |
Oryx (Liu et al., 2024e) | 83.2 | 41.0 | 51.4 | 17.5 |
Intern-VL-2.5 (Chen et al., 2024b) | 72.4 | 56.6 | 60.6 | 37.2 |
NVILA (Liu et al., 2024f) | 75.2 | 51.6 | 57.6 | 28.0 |
LongVU (Shen et al., 2024) | 85.7 | 42.6 | 68.5 | 22.7 |
Qwen2-VL (Wang et al., 2024) | 79.7 | 57.6 | 68.2 | 34.4 |
LLaVA-Next (Liu et al., 2024a) | 95.5 | 55.8 | 82.7 | 39.8 |
Proprietary Models | ||||
Gemini-1.5-Flash (Team et al., 2024) | 93.0 | 67.5 | 81.1 | 50.0 |
GPT-4o (Hurst et al., 2024) | 90.6 | 75.2 | 75.6 | 59.1 |
🔼 This table presents the performance of various VideoLLMs on two types of video understanding tasks. The first task involves videos where comprehension can be achieved through spatial scene understanding and world knowledge alone. The second task uses videos that necessitate temporal reasoning and comprehension for accurate understanding.
read the caption
Table 4: Video understanding evaluation results on two categories of videos: 1) videos that can be understood through spatial scene understanding and world knowledge, and 2) videos that require temporal reasoning for comprehension.
Model | Overall Acc. | Fake Acc. | Real Acc. | F1-Score | Yes Rate |
Video-LLaVA (Ning et al., 2023) | 72.7 | 72.9 | 72.5 | 72.9 | 50.3 |
Oryx (Liu et al., 2024e) | 58.6 | 33.2 | 84.3 | 44.6 | 24.5 |
Intern-VL-2.5 (Chen et al., 2024b) | 56.5 | 99.7 | 12.8 | 69.7 | 93.5 |
NVILA (Ni et al., 2025) | 72.6 | 48.4 | 97.1 | 64.0 | 25.8 |
LongVU (Shen et al., 2024) | 70.3 | 62.7 | 78.0 | 68.0 | 42.5 |
Qwen2-VL (Wang et al., 2024) | 76.2 | 58.3 | 94.3 | 71.1 | 32.1 |
LLaVA-Next (Liu et al., 2024a) | 73.2 | 63.3 | 83.2 | 70.4 | 40.2 |
Gemini-1.5-Flash (Team et al., 2024) | 73.1 | 47.6 | 98.8 | 64.0 | 24.6 |
🔼 This table presents a detailed breakdown of the performance of various video generation models on an AI-generated video judgment task. It shows the overall accuracy, accuracy on AI-generated videos, accuracy on real videos, F1-score, and the ‘yes rate’ (the percentage of times the model judged a video as AI-generated) for each model. The results offer insights into the models’ ability to distinguish between real and synthetic videos and highlight potential biases in their classifications.
read the caption
Table 5: Detailed Evaluation Results for AI-Generated Video Judgment Task.
Human Alignment | |
GPT-4o | 0.72 |
GPT-4o + our prompt strategy | 0.80 |
11.1% |
🔼 This table presents a comparison of two prompt strategies used to evaluate the impossible prompt following capability of video generation models. The first strategy is a baseline approach, while the second incorporates a refined methodology designed to improve the accuracy and consistency of model evaluations. The comparison highlights the effectiveness of the refined strategy in aligning model assessments with human judgment, quantified by the human alignment score. The percentage improvement in human alignment is also presented, demonstrating the positive impact of the refined prompt strategy.
read the caption
Table 6: Comparison between our prompt strategy and baseline prompt strategy for impossible prompt following evaluation.
Full paper#
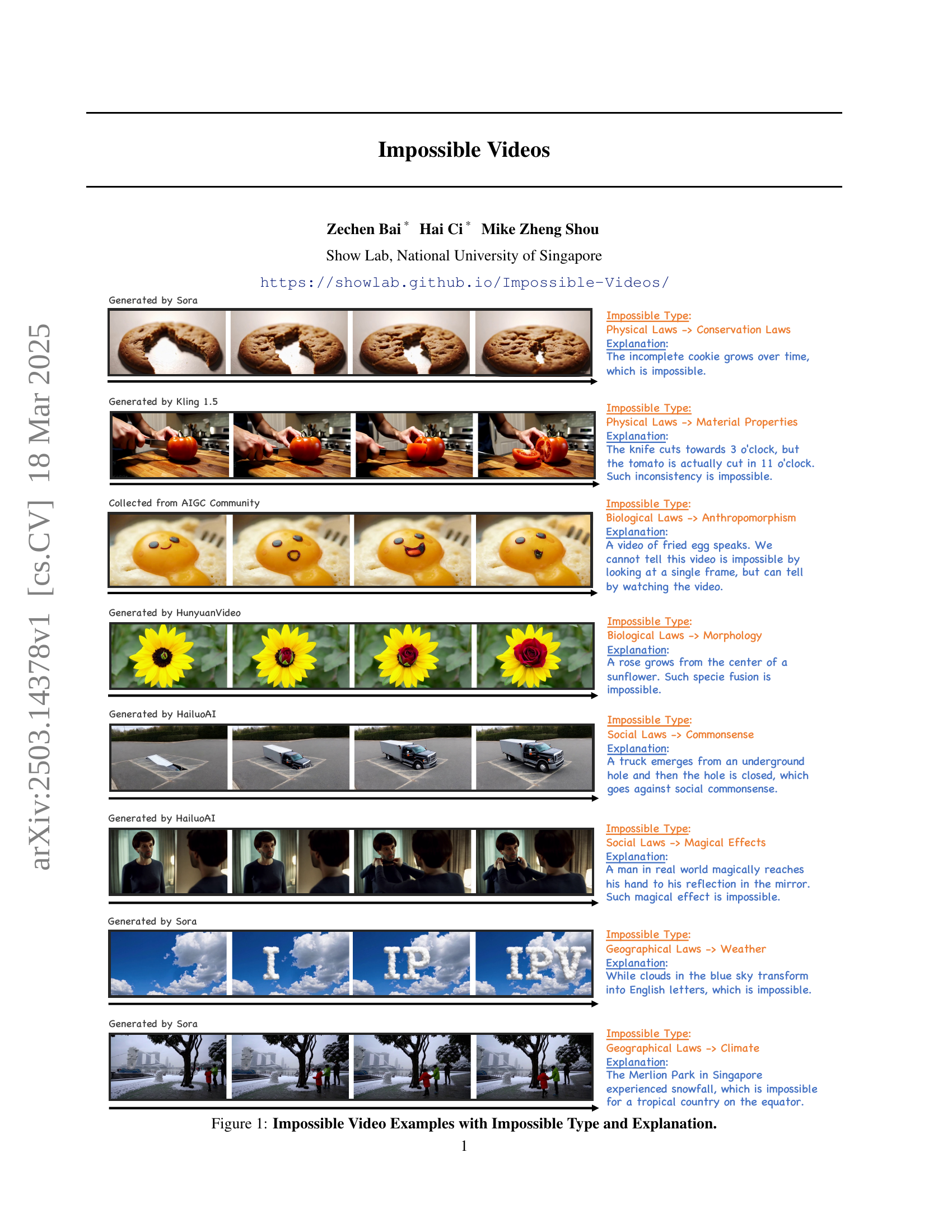
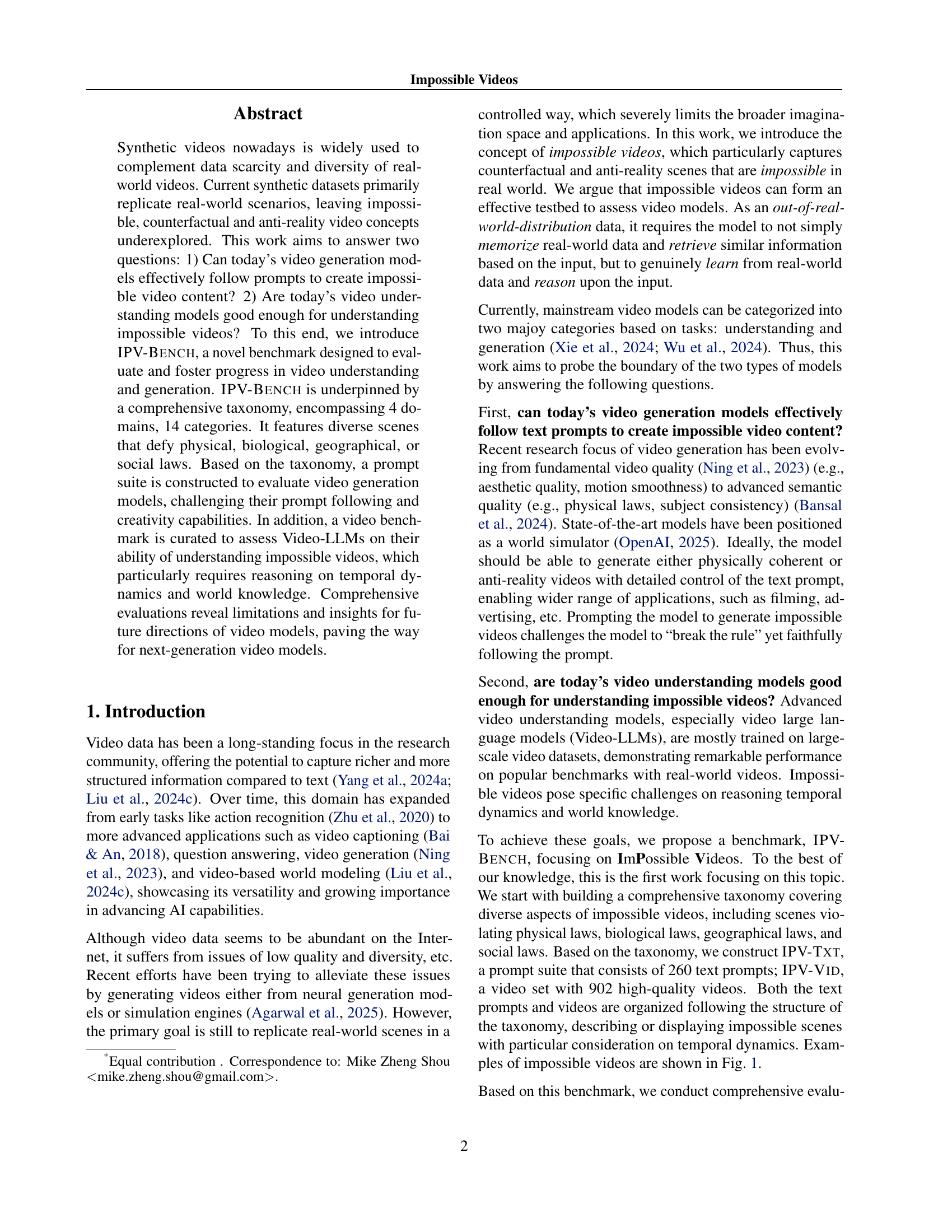
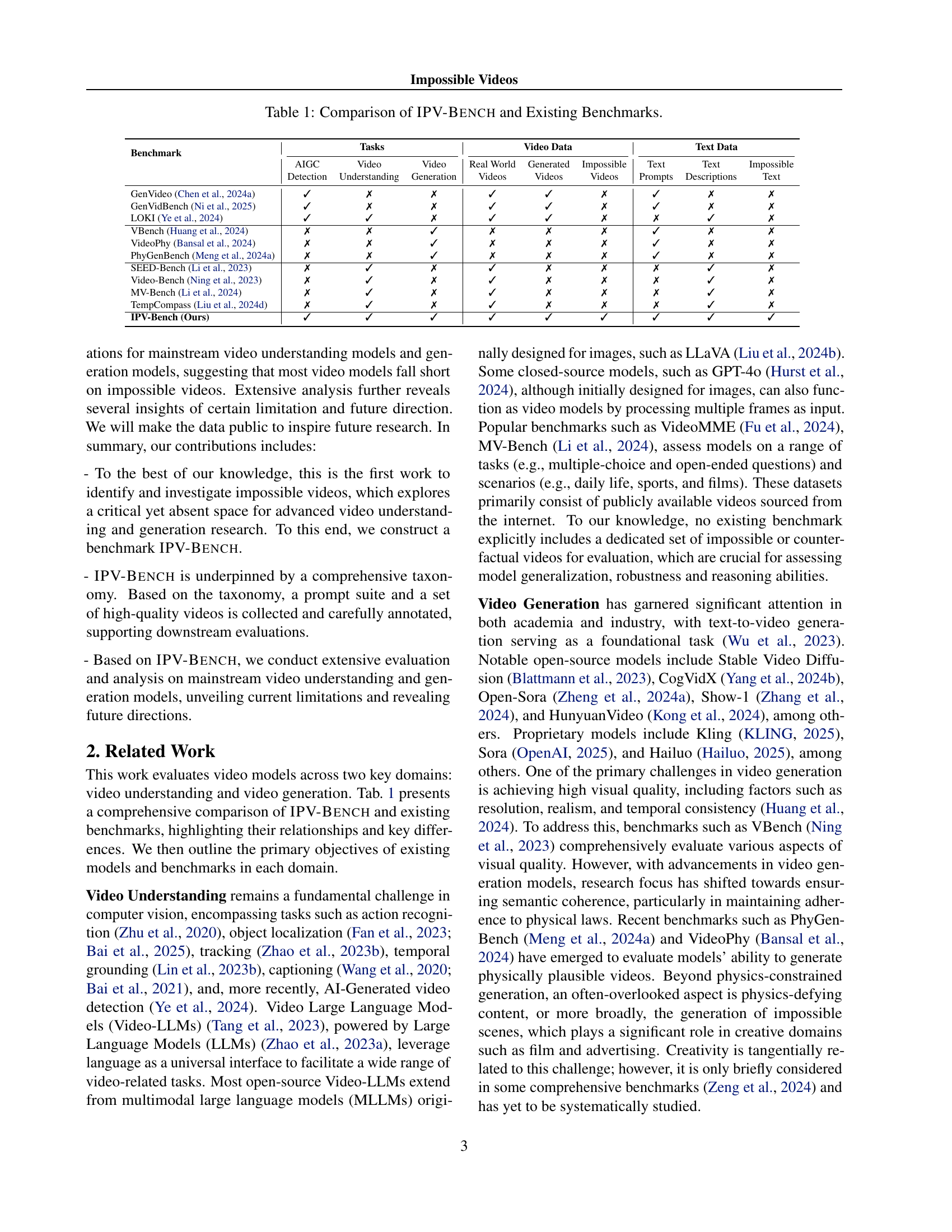
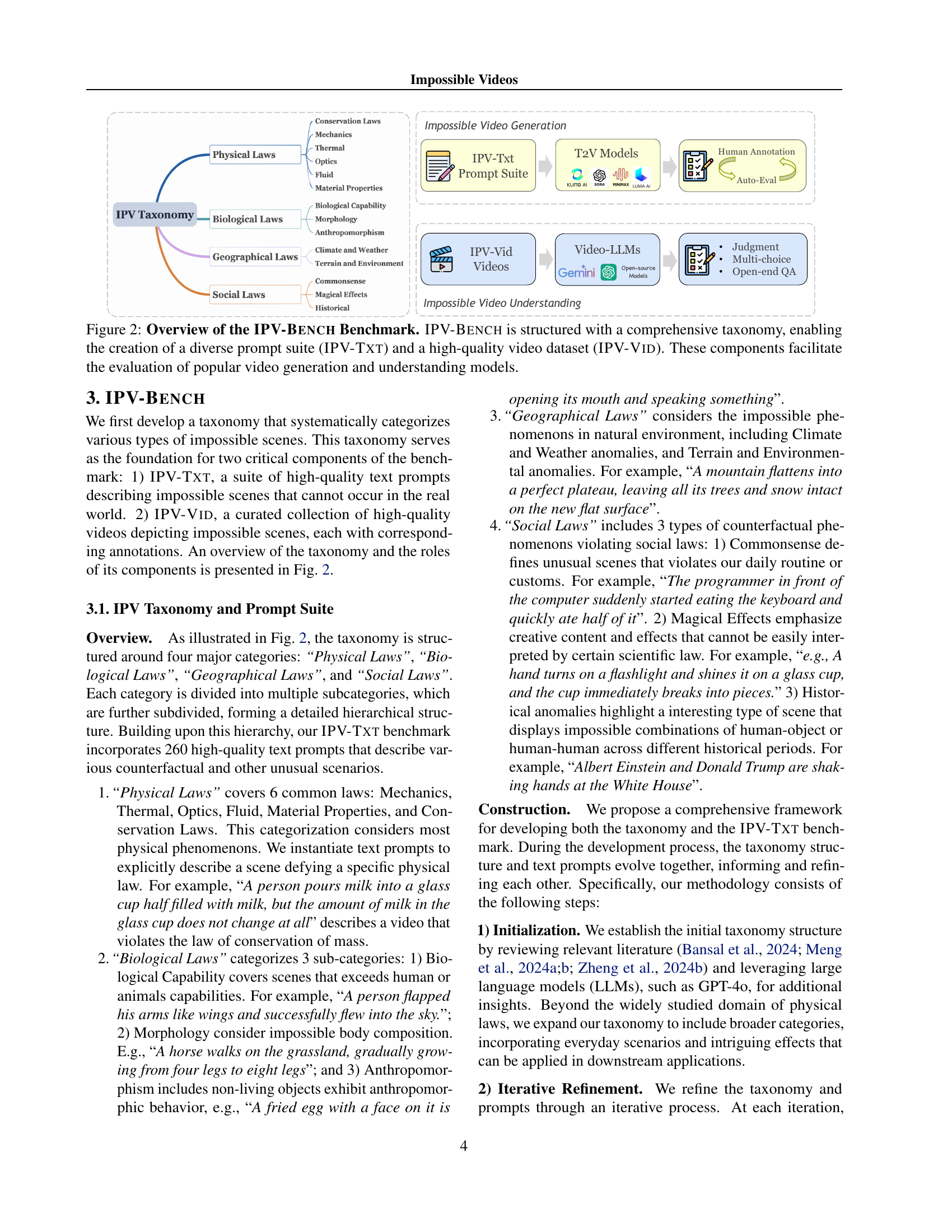
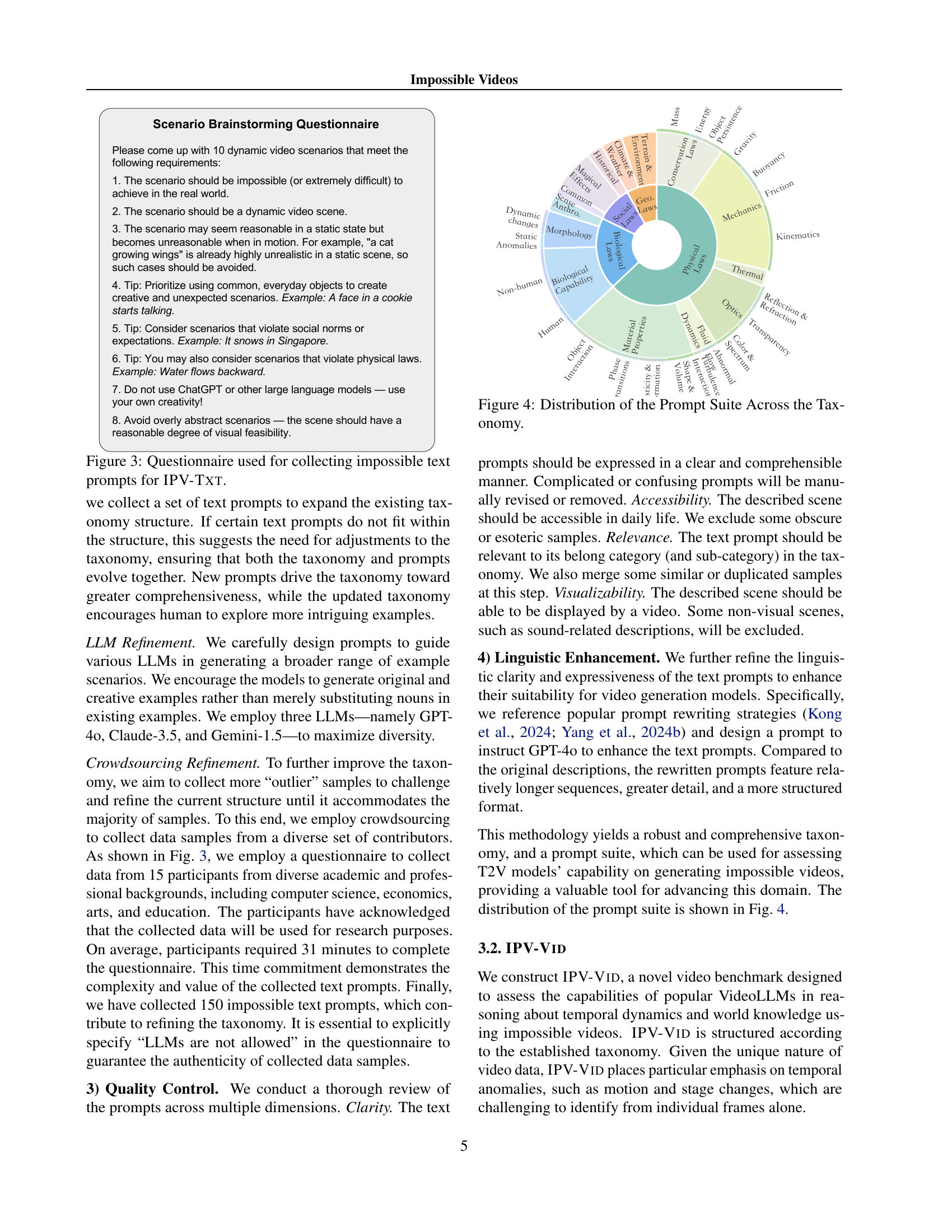
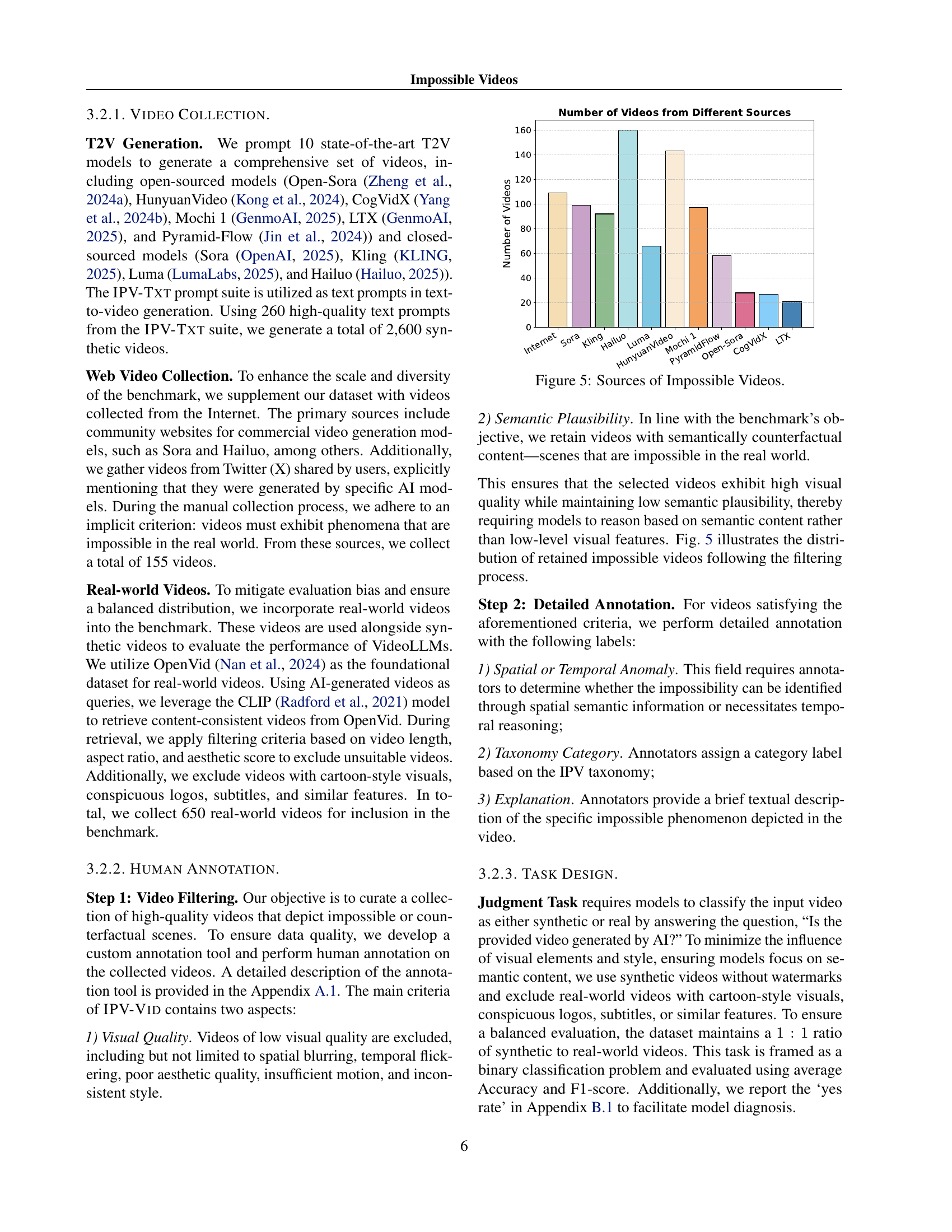
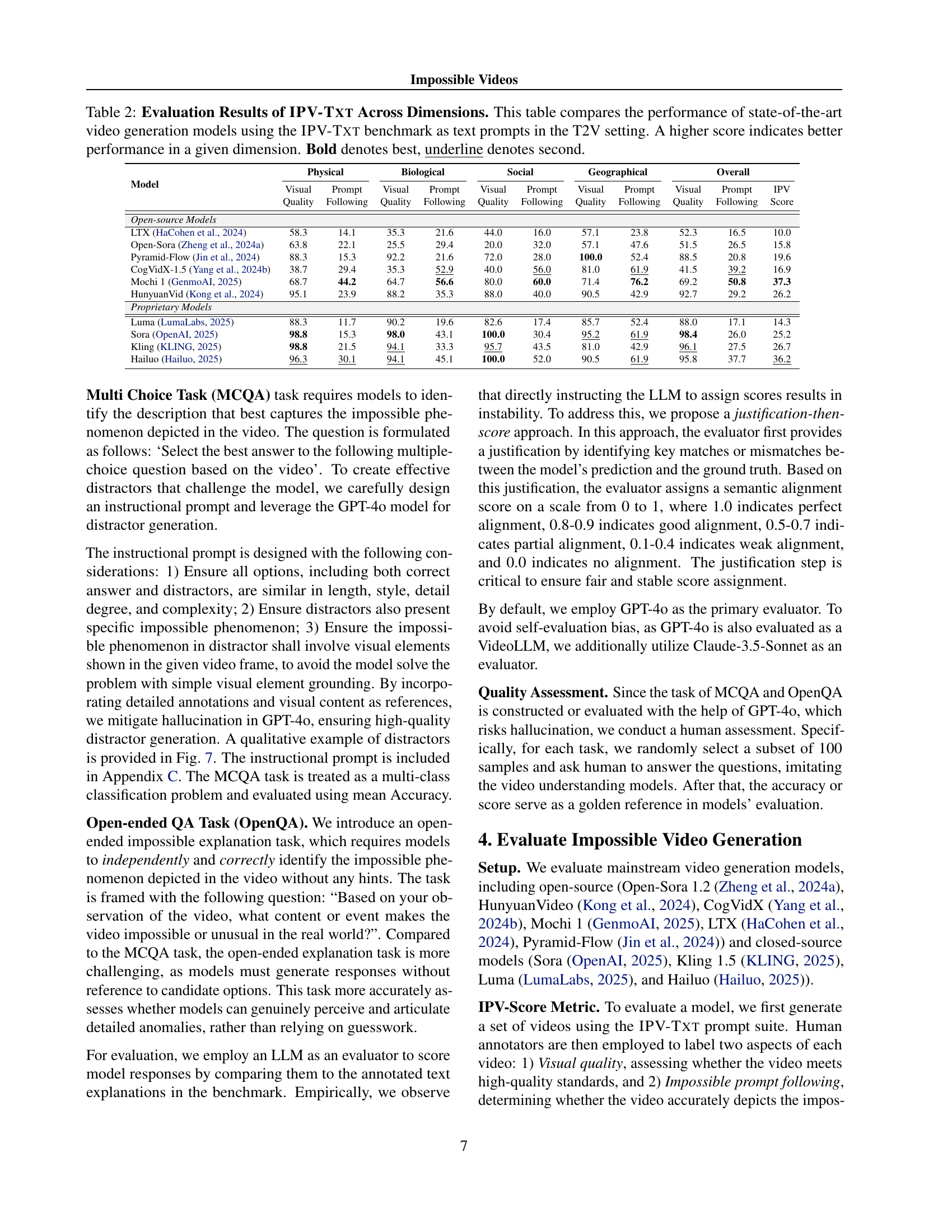
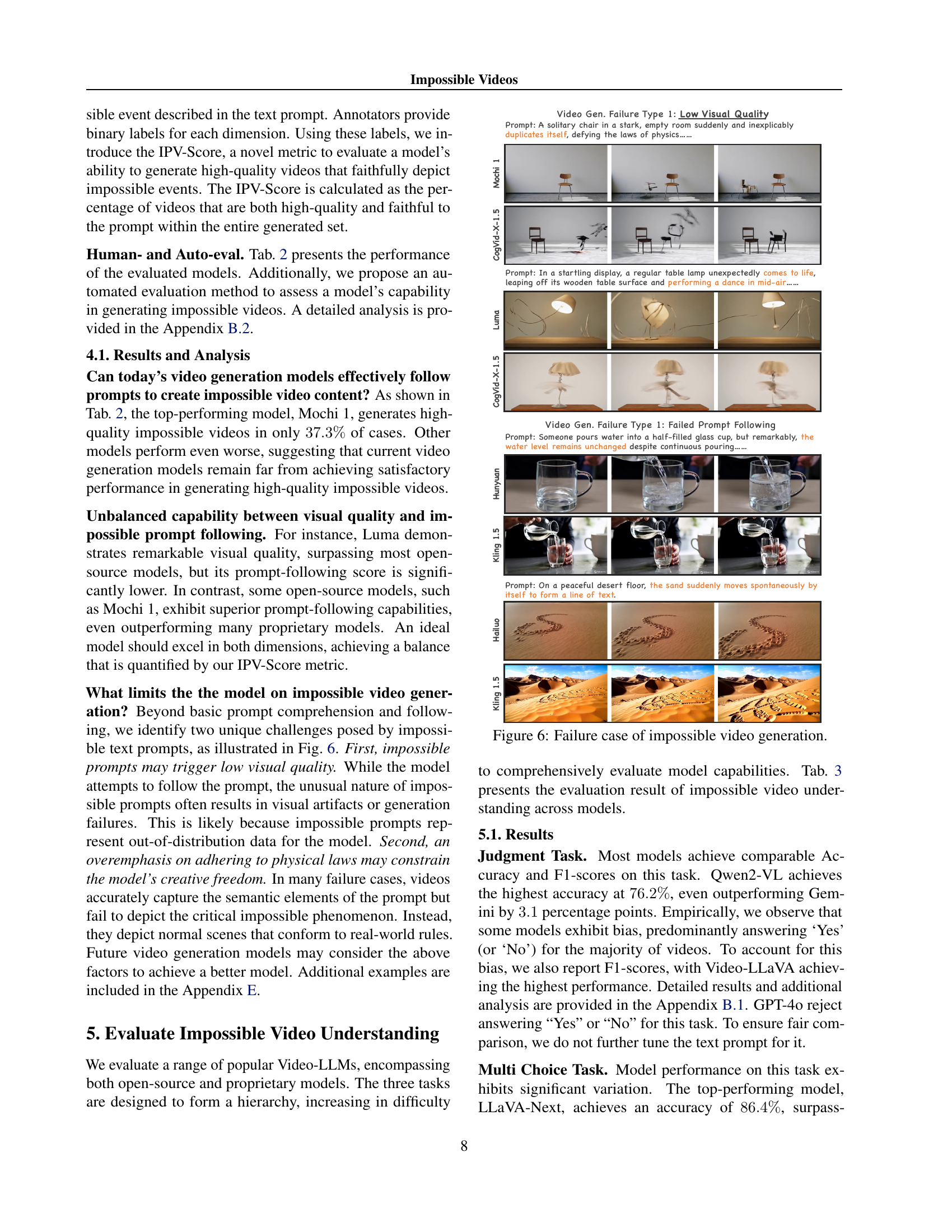
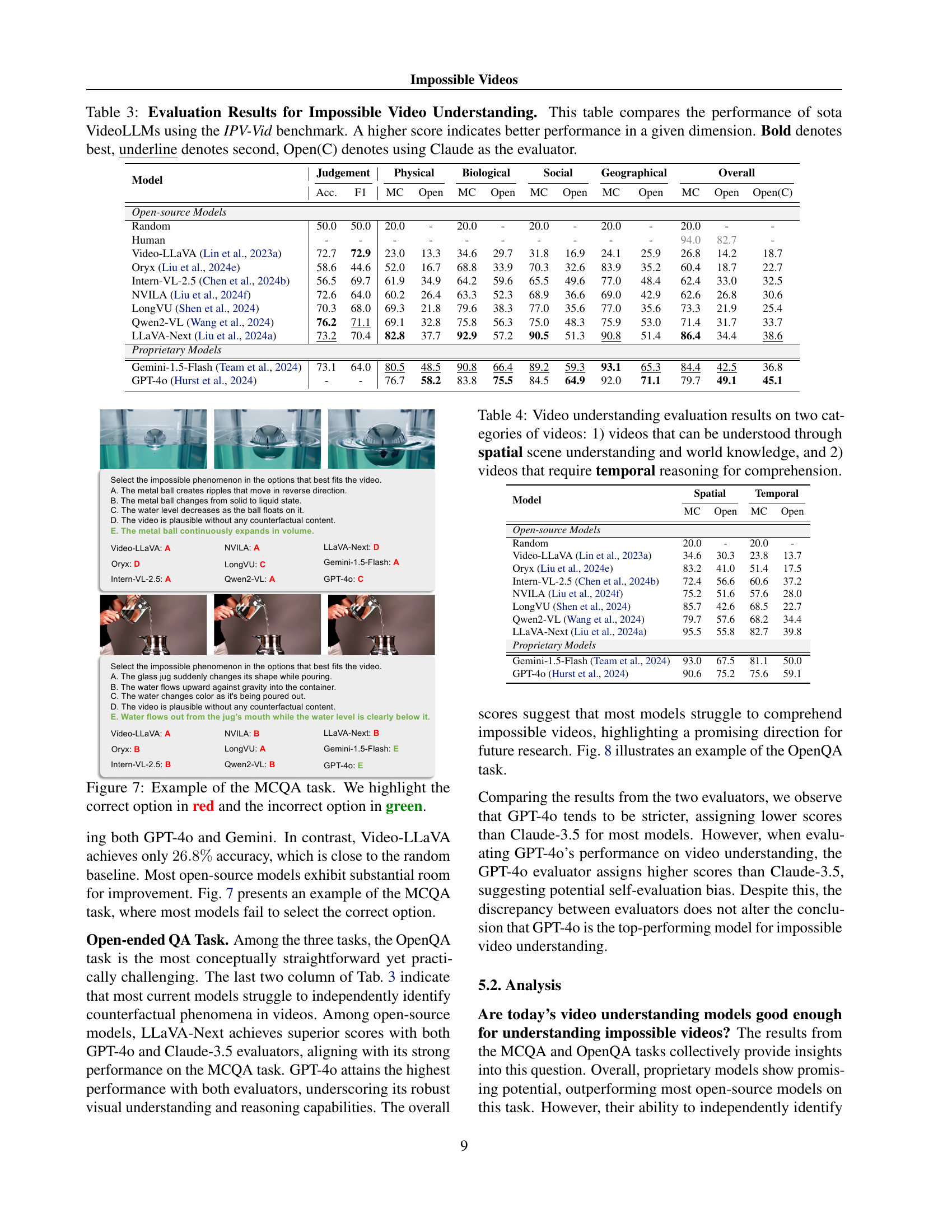
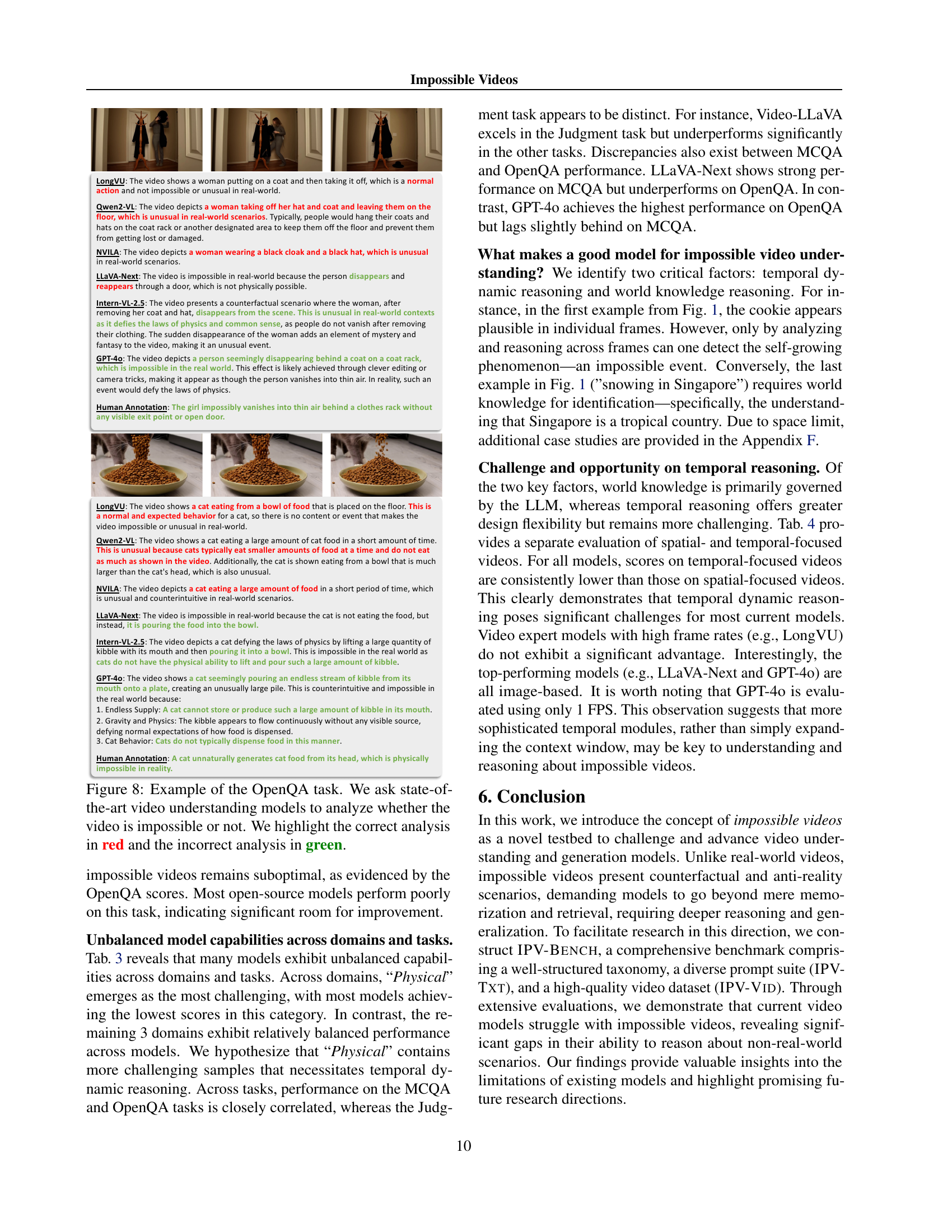
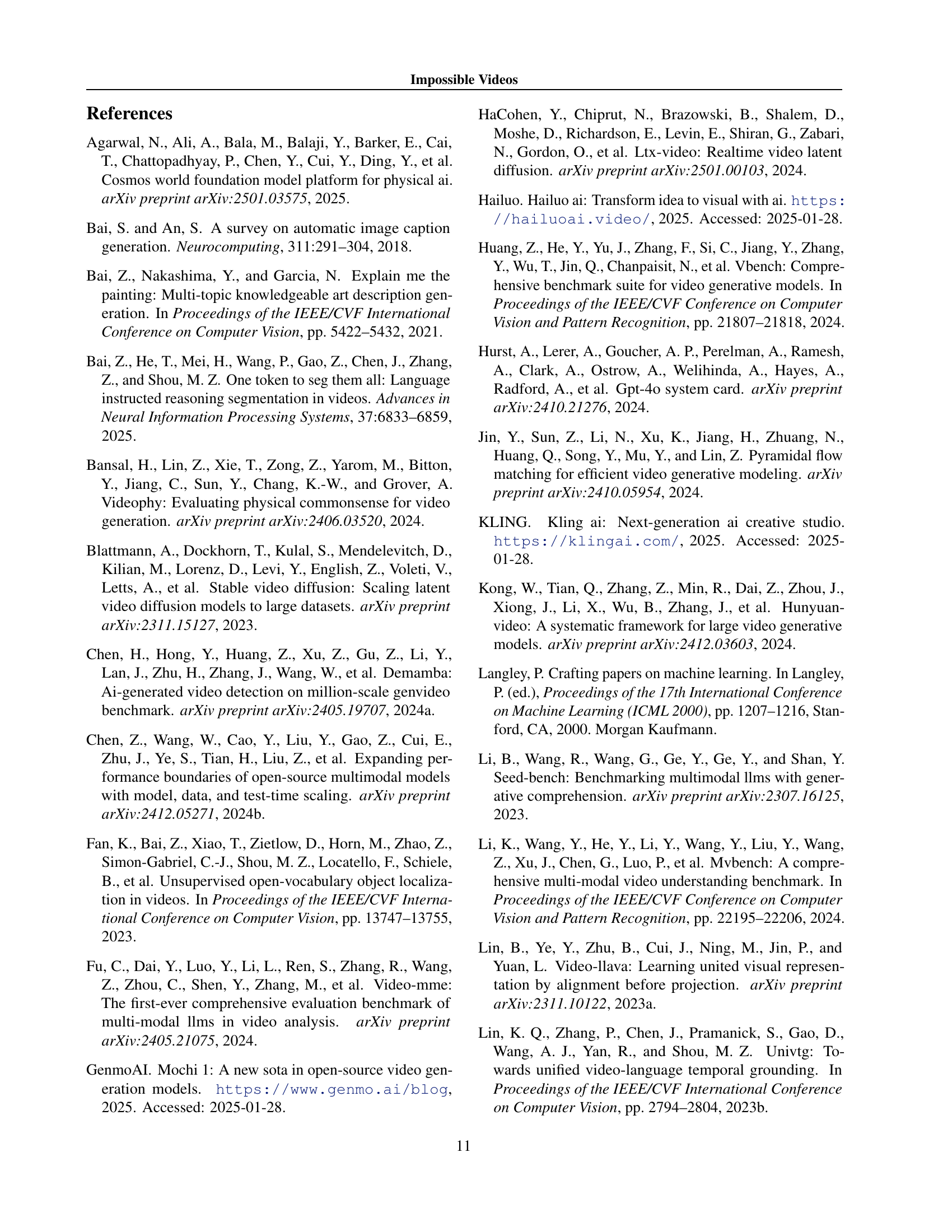
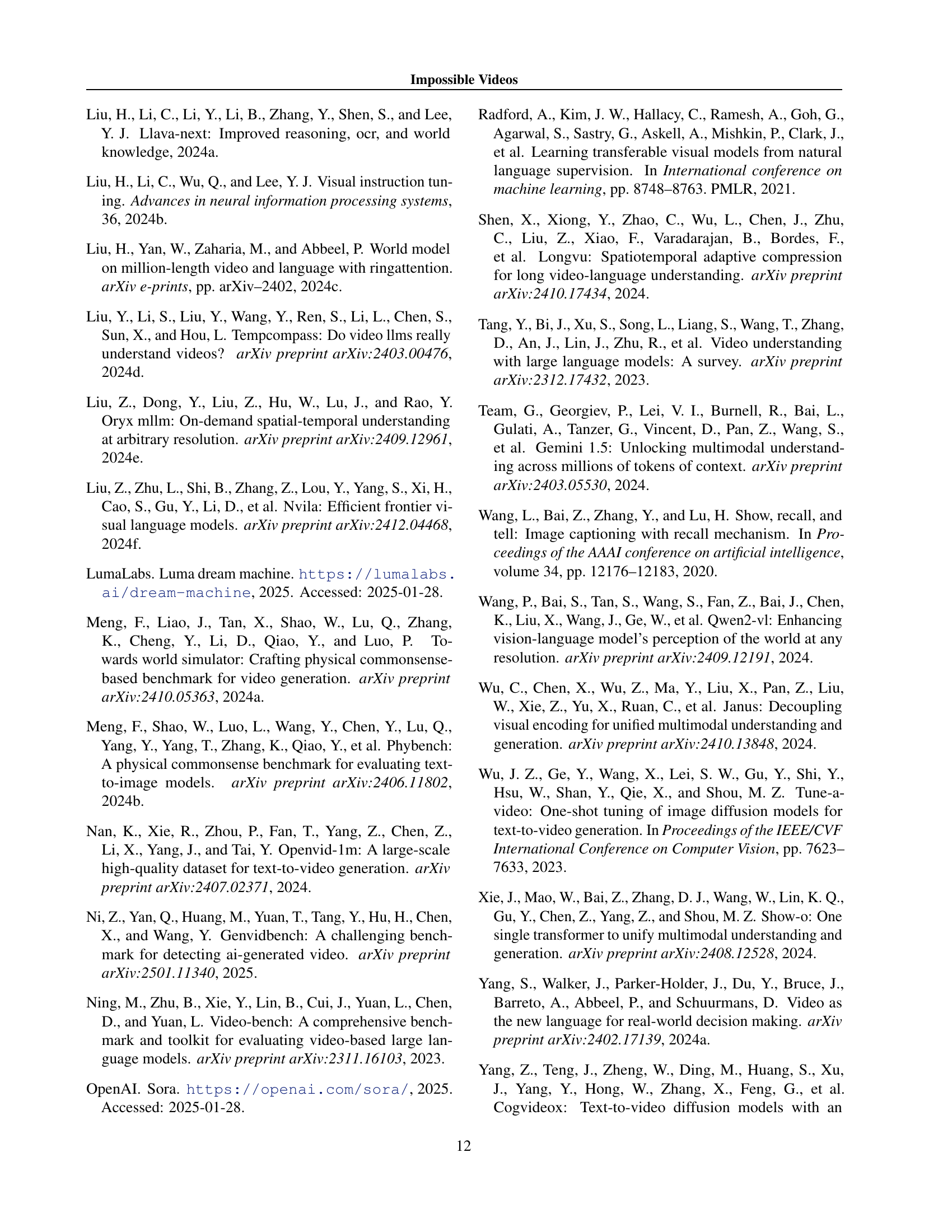
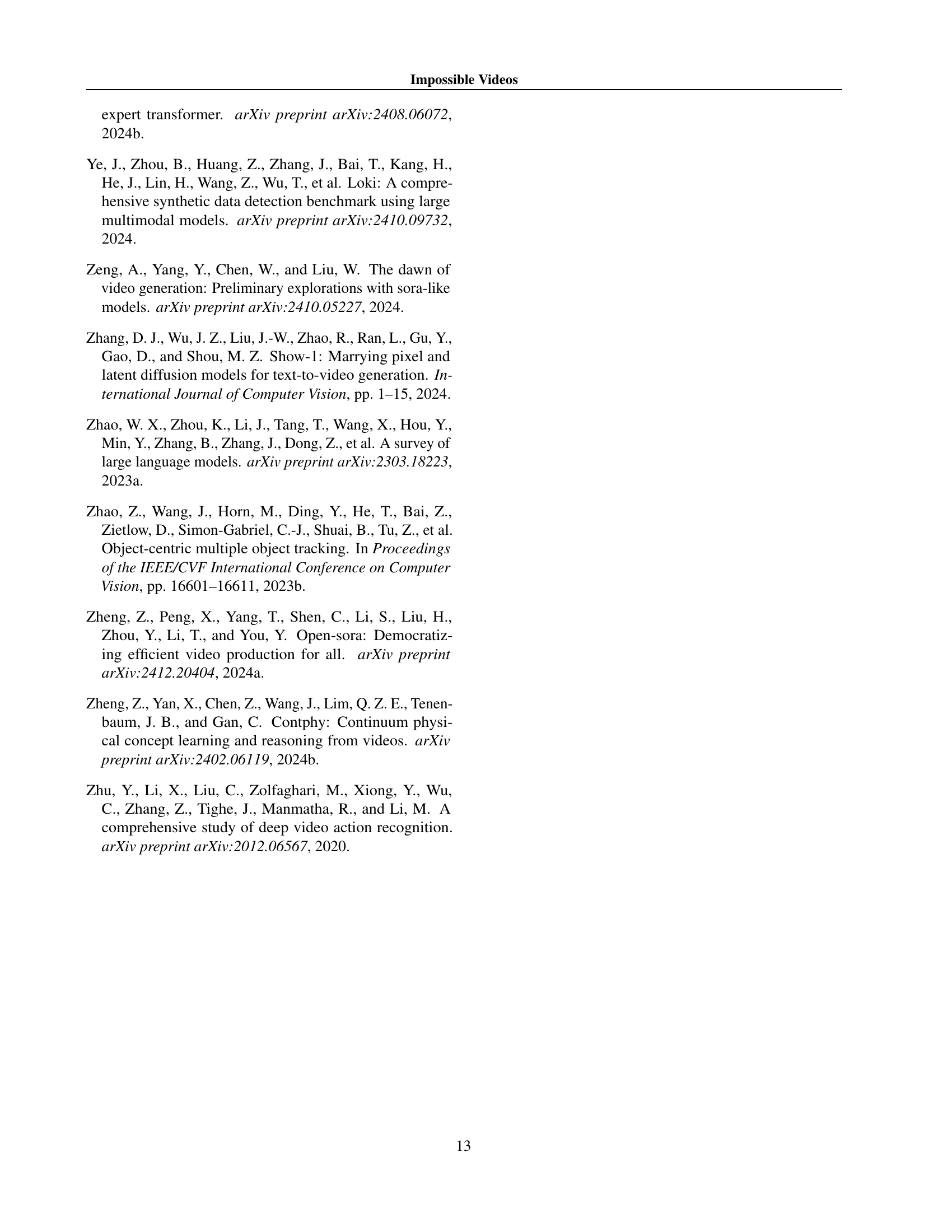
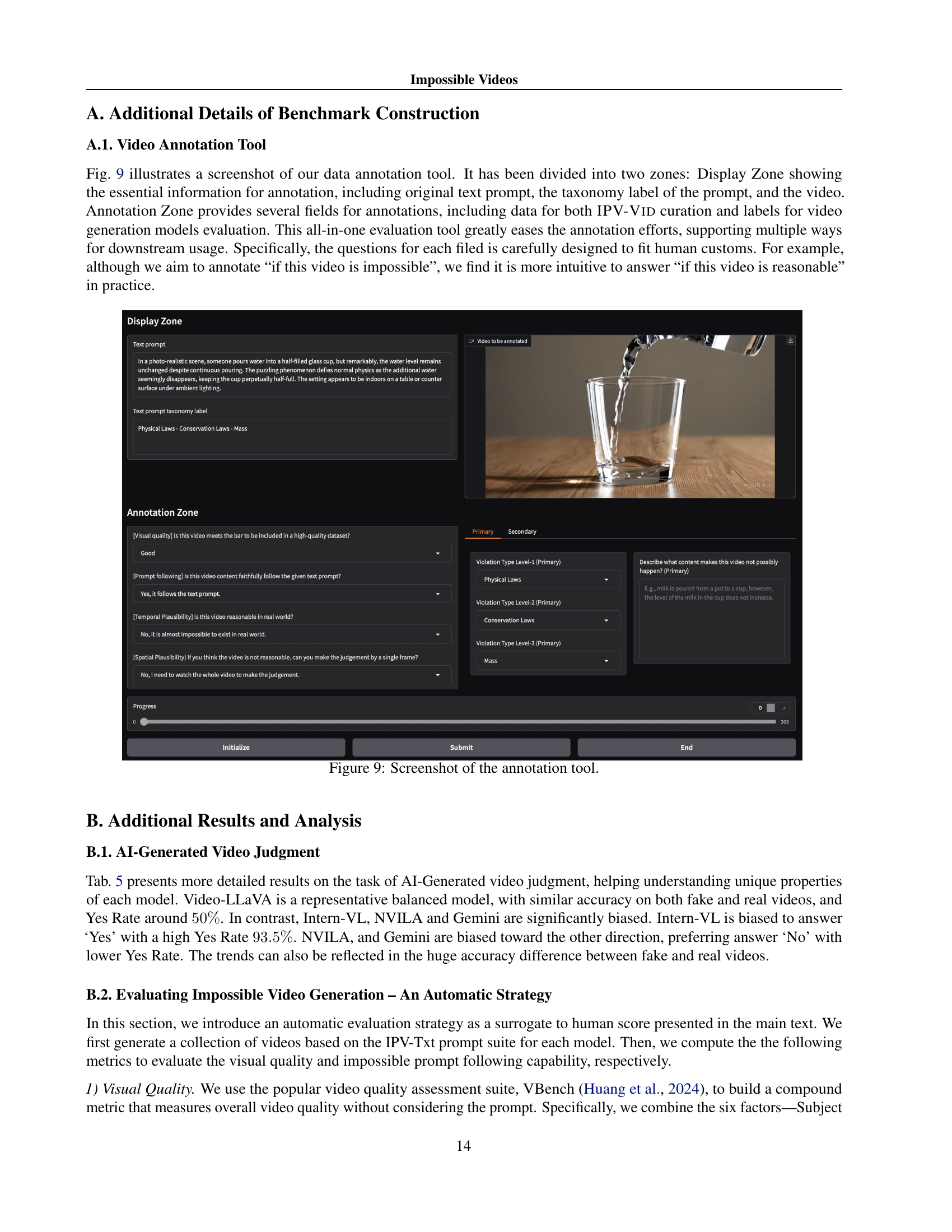
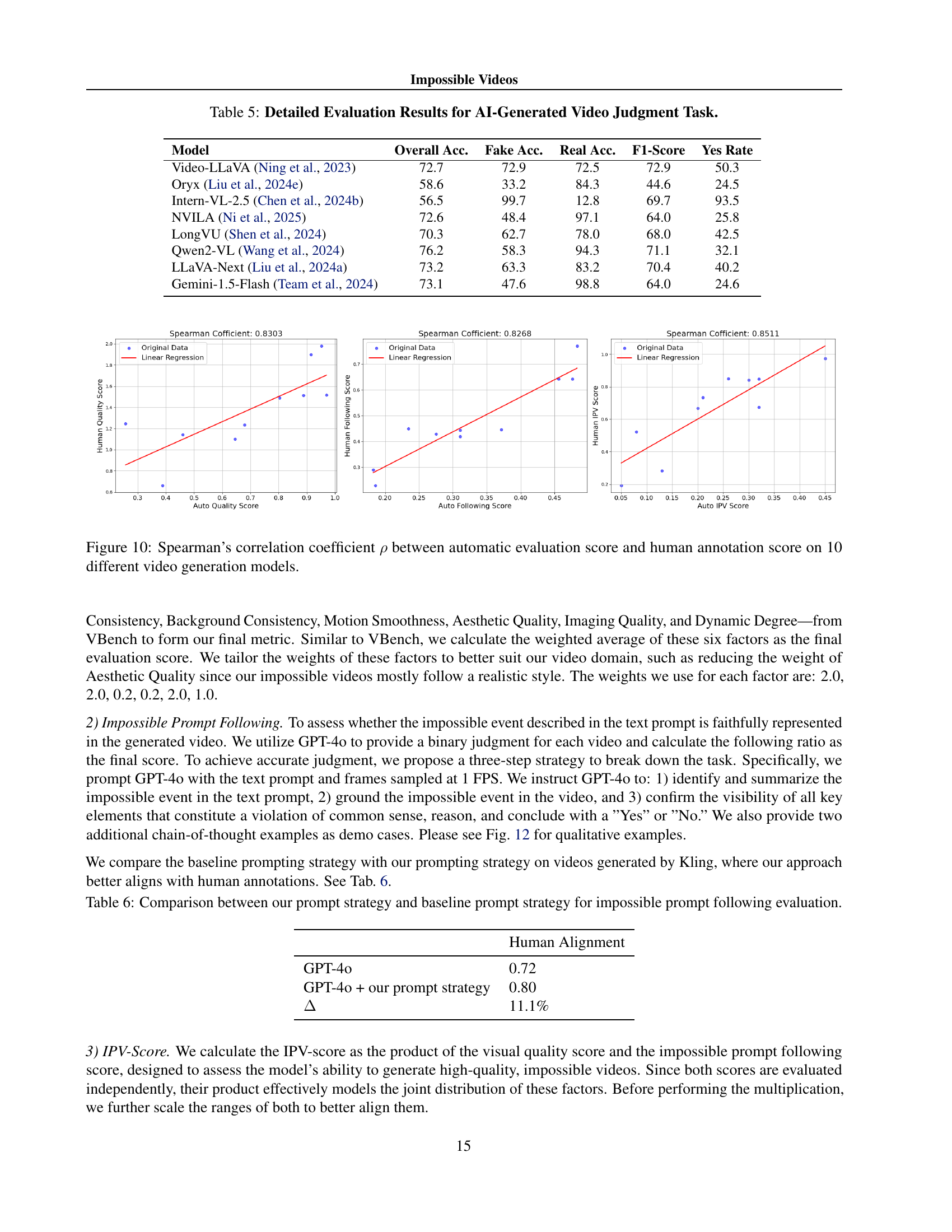
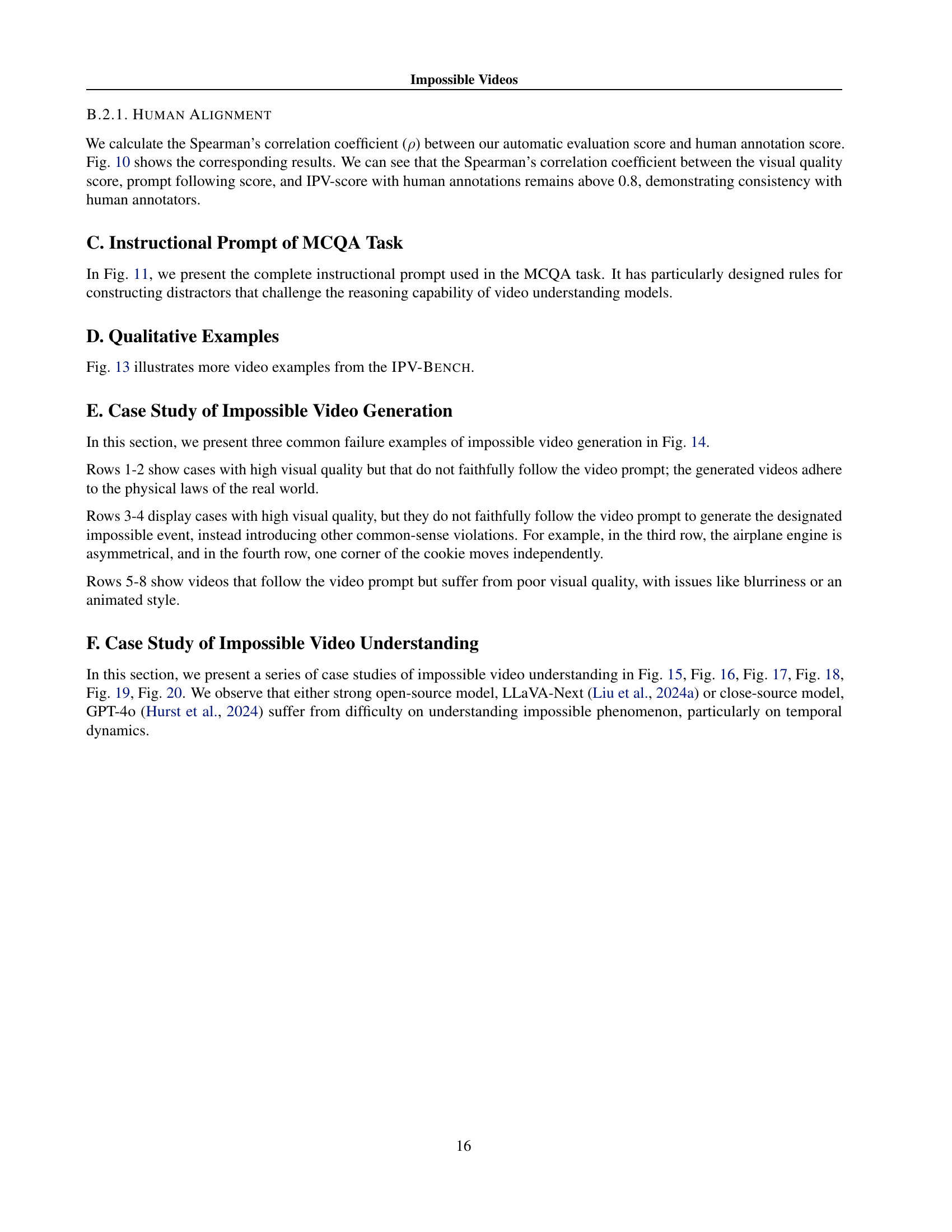
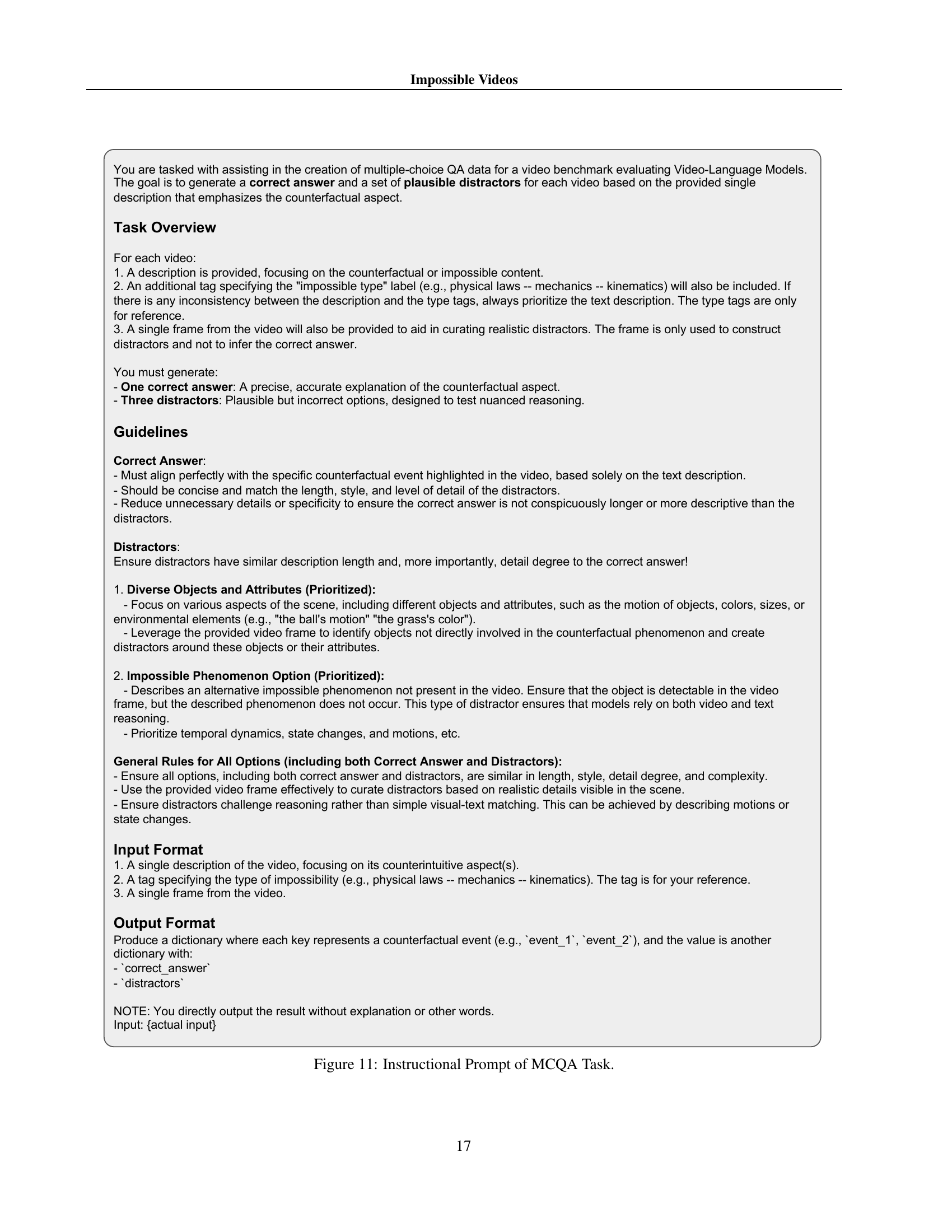
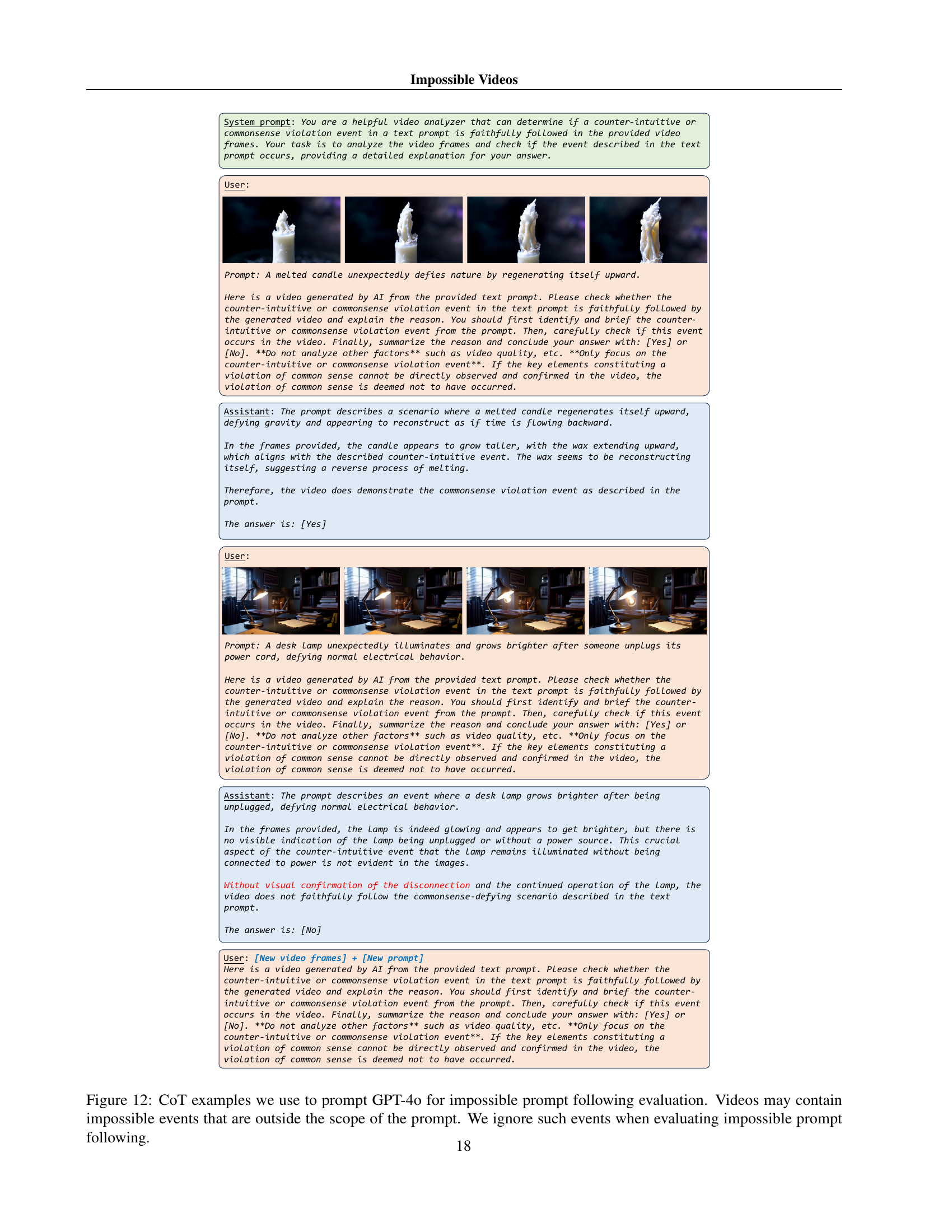
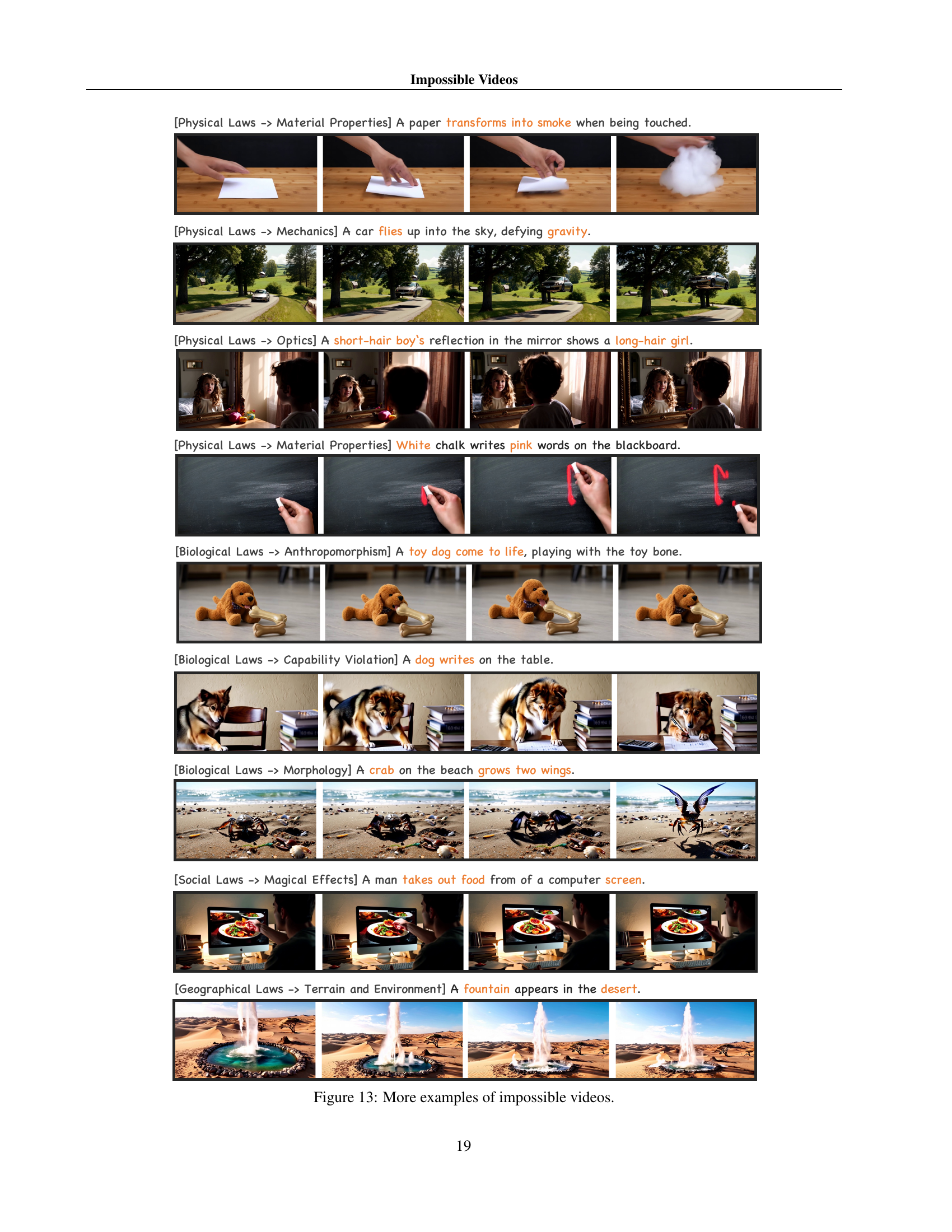
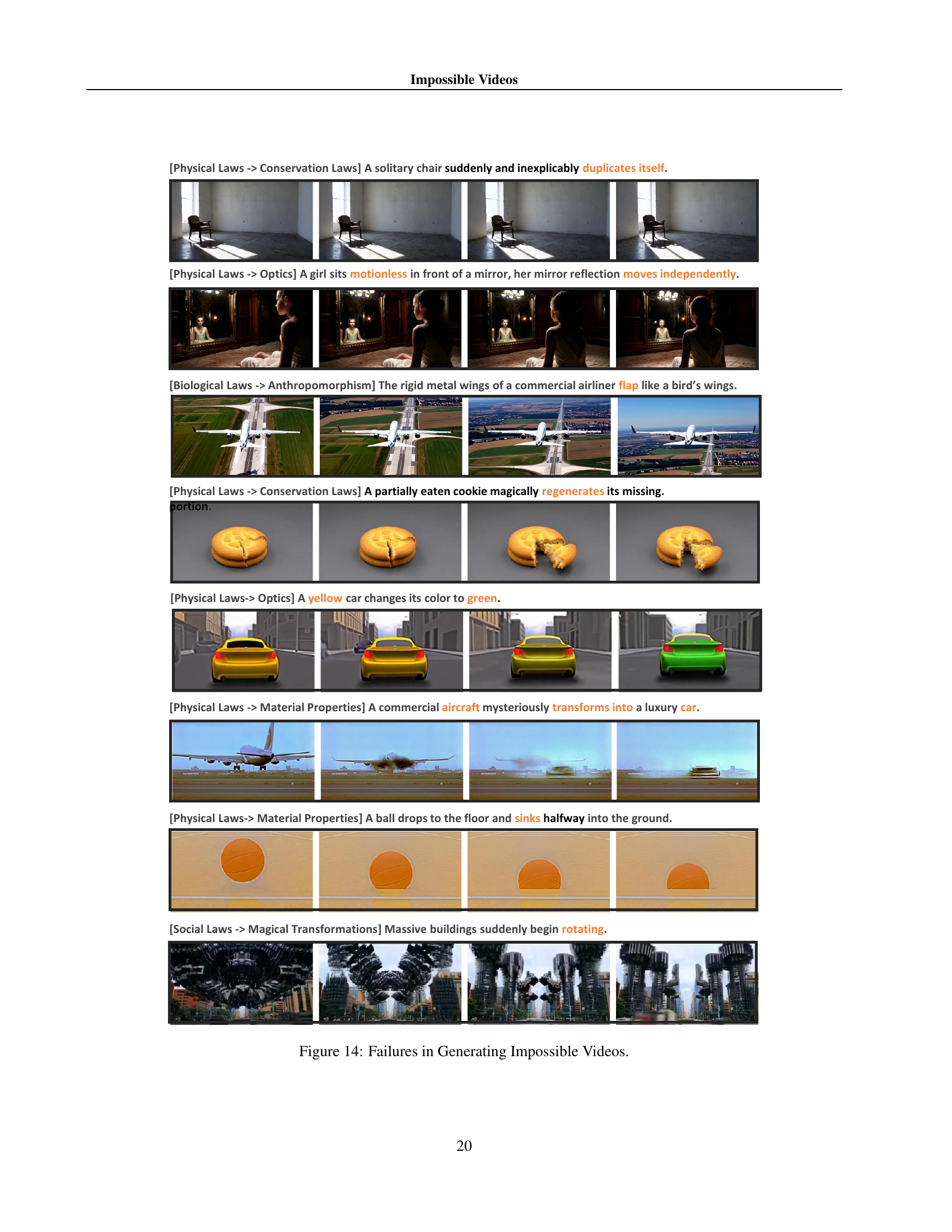