TL;DR#
Current MLLM benchmarks have gaps evaluating visual-creative intelligence. Existing benchmarks feature simple questions that fail to assess model performance in real-life creative tasks. To address this, the paper introduces Creation-MMBench to divide the intelligence into three forms and comprehensively evaluate visual creative intelligence.
The paper proposed Creation-MMBench, a multimodal benchmark specifically designed to evaluate the creative capabilities of MLLMs in real-world, image-based tasks. Creation-MMBench provides more creative and discriminative questions and better evaluation of visual creative intelligence. The benchmark comprises 765 test cases spanning 51 fine-grained tasks.
Key Takeaways#
Why does it matter?#
This study introduces Creation-MMBench, a benchmark to evaluate creative capabilities of MLLMs, which will provide a valuable resource for researchers working on advancing MLLM creativity and generative intelligence. The benchmark and analysis can open new avenues for research in multimodal learning and evaluation.
Visual Insights#
🔼 The figure illustrates the motivation behind the creation of Creation-MMBench. It highlights the limitations of existing Multimodal Large Language Model (MLLM) benchmarks in assessing visual-creative intelligence. These benchmarks often lack comprehensive evaluation of visual creativity and rely on simple questions that do not reflect real-world creative tasks. In contrast, Creation-MMBench offers a more robust evaluation by incorporating four categories of tasks, more creative and discriminative questions, and a more thorough assessment of visual creative intelligence.
read the caption
Figure 1: Our Motivation for Creation-MMBench. The triarchic theory of intelligence divides intelligence into three forms. Current MLLM benchmarks have significant gaps in evaluating visual-creative intelligence compared to the other forms. Additionally, existing benchmarks feature simple questions that fail to assess model performance in real-life creative tasks. Therefore, we proposed Creation-MMBench, which includes four categories, more creative and discriminative questions, and better evaluation of visual creative intelligence.
Benchmarks | Num of Creative Questions | Criteria Level | multi-images task | Specific Role for each Questions | Visual Factuality Check |
VisIT-Bench | 65 | benchmark | ✓ | ✗ | ✓ |
MLLM-Bench | 20 | instance | ✗ | ✗ | ✓ |
Touch-Stone | 189 | benchmark | ✓ | ✗ | ✗ |
AlignMMbench | 353 | task | ✗ | ✗ | ✗ |
Creation-MMBench | 765 | instance | ✓ | ✓ | ✓ |
🔼 This table compares Creation-MMBench to other Multimodal Large Language Model (MLLM) benchmarks that focus on evaluating creative capabilities. It highlights key differences in the number of creative questions, whether multiple images are used per question, the level of detail in the questions (benchmark vs instance-level), whether specific roles are assigned in the questions, the importance of visual elements, the inclusion of a factuality check, and the availability of questions without visual content. This comparison helps showcase Creation-MMBench’s unique features and how it improves on existing benchmarks.
read the caption
Table 1: Comparison of Creation-MMBench with other partial-creation MLLM benchmarks.
In-depth insights#
Creative MLLM Gap#
The under-explored domain of creative capabilities in Multimodal Large Language Models (MLLMs), compared to LLMs, represents a significant ‘Creative MLLM Gap’. Current benchmarks inadequately assess visual-creative intelligence, especially in real-world, image-based tasks. Addressing this gap is crucial, as creativity, involving novel and appropriate solutions, is a fundamental aspect of intelligence. Existing benchmarks often feature simplistic questions, failing to challenge MLLMs in complex, real-life scenarios that demand broader cognitive abilities. The benchmarks lag significantly behind those conducted for LLMs. Assessing visual creativity requires benchmarks encompassing a range of cognitive abilities Visual fine-tuning can negatively impact the base LLM’s creative abilities. Therefore, dedicated benchmarks that evaluate the integration of visual perception with creative expression are essential for advancing MLLM capabilities in this domain.
Real-world Vision#
Real-world vision in MLLMs highlights the critical need for models to effectively translate visual inputs into creative outputs. This involves nuanced understanding, factual consistency, and the ability to generate contextually appropriate responses. Creativity benchmarks specifically designed help evaluate models for their real-world application. Models face challenges to maintain creativity while staying aligned with visual details, and the exploration of trade-offs becomes essential. Visual tuning affects creative abilities, understanding the necessity of the model.
Instance Criteria#
Instance-specific criteria are pivotal for MLLM evaluation, offering granular assessment of responses tailored to each unique test case. Unlike generic benchmarks, defining unique criteria ensures effective integration of visual and contextual cues. This tailored approach is vital for complex, creative tasks where a single evaluation model falls short. By assessing both general response quality and factual consistency, these criteria enable a comprehensive and nuanced understanding of the model’s proficiency. Crafting detailed instructions that consider the subtleties of individual tasks yields a more reliable and insightful evaluation, surpassing the limitations of one-size-fits-all metrics. These criteria provide a robust framework for advancing multimodal generative intelligence.
Visual hurts LLM?#
Visual fine-tuning’s impact on LLMs is complex. While enhancing multimodal capabilities, it can negatively affect the base LLM’s creative abilities, indicating a trade-off. The study’s findings suggest that visual training, designed to improve perception, might constrain the LLM’s original strength in text-based creative tasks. This implies that LLMs might over specialize during visual instruction tuning, losing the inherent ability to diverge in creative tasks. Careful tuning strategies are needed to mitigate such losses, ensuring multimodal LLMs retain robust creativity alongside enhanced visual understanding. Further research on LLM could be crucial
For Generative AI#
Assessing creative intelligence in multimodal models (MLLMs) opens intriguing avenues for generative AI. Unlike discriminative tasks, where models identify patterns, generative AI demands novel content creation aligned with context and constraints. Benchmarks like Creation-MMBench, focusing on visual-creative tasks, are vital. They push MLLMs beyond recognition to imaginative applications like story writing or design generation. A key challenge lies in evaluation: assessing not just accuracy, but originality and contextual fit. This necessitates new metrics and judge models adept at subjective assessment. By tackling this, we enhance generative AI’s potential for creative problem-solving and innovative expression.
More visual insights#
More on figures
🔼 The figure illustrates the brain regions associated with creativity, highlighting the frontal lobe’s crucial role in functions like concentration, planning, and problem-solving, which are essential components of the creative process. It visually represents the neural networks involved in creative thinking.
read the caption
Figure 2: Brain regions related to creativity and their respective functions [11, 6].
🔼 Creation-MMBench is a multimodal benchmark for evaluating the creative capabilities of large language models (LLMs), particularly in image-based tasks. This figure provides a visual overview of the benchmark’s structure and content. It shows the four main task categories within Creation-MMBench (Literary Writing, Common Functional Writing, Professional Functional Writing, and Creative Multimodal Understanding), each containing numerous sub-tasks. A selection of representative example tasks within each category is visually displayed, along with sample images illustrating the diverse visual content used across all tasks. The caption points out that this figure shows only a subset of the total tasks, with a complete list available in Appendix A of the paper.
read the caption
Figure 3: Overview of Creation-MMBench. Contains four task categories, each category consists of multiple tasks, and the types of images are diverse. Only a few representative tasks of each category are shown here. Complete list of tasks is detailed in the Appendix A.
🔼 The bar chart displays the performance of various Multimodal Large Language Models (MLLMs) on the Creation-MMBench benchmark when visual input is removed. It compares the reward scores for each model, providing insights into their creative capabilities in a text-only setting. Lower reward scores indicate weaker performance without visual context.
read the caption
Figure 4: Evaluation Result of MLLMs w/o visual input.
🔼 The figure shows a bar chart illustrating the distribution of query lengths in the Creation-MMBench dataset. The x-axis represents the range of query lengths, categorized into bins (e.g., <50, 50-500, 500-1000, >1000). The y-axis represents the frequency or proportion of queries falling within each length range. This visualization helps to understand the length distribution characteristics of questions used in Creation-MMBench for evaluating MLLMs’ creative capabilities.
read the caption
(a) Distribution of query lengths.
🔼 The figure shows the diversity of roles incorporated in the Creation-MMBench benchmark’s queries. The variety of roles reflects real-world scenarios and aims to stimulate creative responses from MLLMs by requiring them to leverage diverse disciplinary knowledge and prior experience.
read the caption
(b) Roles in Creation-MMBench.
🔼 This figure shows an example case from Creation-MMBench. It showcases two tasks, one focused on design and the other on creative writing. The design task presents a floor plan and asks the model to analyze the plan and provide improvement suggestions considering a family of four. The creative writing task shows an image and asks the model to write a short story based on the image. The figure illustrates the benchmark’s diverse tasks and its multimodal nature.
read the caption
(c) Example Case of Creation-MMBench.
🔼 Creation-MMBench is a new benchmark designed to evaluate the creative capabilities of multimodal large language models (MLLMs). Figure 6 highlights key aspects that differentiate Creation-MMBench from other existing benchmarks. First, the query design is more comprehensive, encompassing rich contexts to fully test creative abilities. Second, diverse roles are included in the prompts to encourage MLLMs to draw upon their existing knowledge and expertise in various fields. Finally, the benchmark utilizes a wide variety of images, enabling a thorough evaluation of MLLMs’ multifaceted capabilities.
read the caption
Figure 6: Statistics and Cases of Creation-MMBench. Compared to other widely used MLLM benchmarks, Creation-MMBench features a more comprehensive query design to capture abundant creative contexts. Diverse roles are introduced into the queries to stimulate MLLMs’ utilization of disciplinary and prior knowledge. As an MLLM benchmark, Creation-MMBench includes a rich variety of images to thoroughly evaluate multiple capabilities of MLLMs.
🔼 This figure compares the performance of various large language models (LLMs) on two different benchmarks: the OpenVLM Leaderboard and Creation-MMBench. The OpenVLM Leaderboard measures general objective performance, while Creation-MMBench specifically evaluates visual creativity. The graph highlights a significant difference between the scores achieved on these two benchmarks for some open-source models. While some models perform well on the OpenVLM Leaderboard (indicating strong general capabilities), their performance on Creation-MMBench is substantially lower, demonstrating a weakness in visual creativity. This discrepancy emphasizes that general objective metrics may not accurately reflect the visual creative capabilities of LLMs, especially for open-source models.
read the caption
Figure 7: Comparing OC Score and Creation-MMBench Reward. This figure shows the model performance on the OpenVLM Leaderboard and Creation-MMBench, highlighting a significant gap between objective performance and visual creativity in some open-source models.
🔼 This figure presents a qualitative comparison of responses generated by InternVL-2.5-78B and GPT-40-1120 for a creative multimodal understanding task within the Creation-MMBench benchmark. It highlights the differences in the models’ abilities to interpret visual information and formulate coherent, creative responses. The analysis focuses on aspects like the accuracy of visual description, the clarity and coherence of the explanation, and the overall engagement level of the response. It showcases how GPT-40-1120 produces a superior response demonstrating a better grasp of the image’s key elements and a more compelling narrative. The detailed breakdown helps illustrate the strengths and weaknesses of each model in a complex creative task.
read the caption
Figure 8: Qualitative study Case between InternVL-2.5-78B and Reference Answer (GPT4o-1120).
🔼 Creation-MMBench is a multimodal benchmark designed to evaluate the creative capabilities of Multimodal Large Language Models (MLLMs). This figure provides a visual overview of the benchmark’s structure. It shows the four main task categories within Creation-MMBench: Literary Writing, Common Functional Writing, Professional Functional Writing, and Creative Multimodal Understanding. Each category contains multiple individual tasks, illustrating the breadth of creative abilities assessed by the benchmark. The figure highlights the diversity of tasks within each category and the benchmark as a whole.
read the caption
Figure 9: Overview of Creation-MMBench Complete Task. Contains four task categories, each category consists of multiple tasks.
🔼 The figure shows a bar chart illustrating the distribution of query lengths in the Creation-MMBench dataset. The x-axis represents different ranges of query lengths (likely categorized into bins such as 0-50 words, 50-100 words, etc.), and the y-axis shows the relative frequency or proportion of queries falling into each length category. This visualization helps to understand the complexity and length variation of the prompts used in the benchmark.
read the caption
(a) Distribution of query lengths.
🔼 The figure shows the various roles that large language models (LLMs) are tasked to assume when responding to prompts in the Creation-MMBench benchmark. The wide variety of roles highlights the diverse contexts and situations in which the LLMs need to generate creative text. Examples of roles include: ‘media head’, ‘customer’, ‘writer’, ‘guide’, and many others that span numerous professional and non-professional fields. The distribution of these roles aims to test the LLMs’ ability to adapt their creative responses to different perspectives and situations.
read the caption
(b) Roles in Creation-MMBench.
🔼 This figure displays the results of a redundancy analysis comparing Creation-MMBench with several other widely used Multimodal Large Language Model (MLLM) benchmarks. The analysis measures the correlation between Creation-MMBench’s evaluation scores and those of other benchmarks. The heatmaps visualize the Spearman’s Rank Correlation Coefficient (SRCC) and the coefficient of determination (R-squared) for all benchmark pairs. High correlations indicate redundancy, suggesting that those benchmarks assess similar aspects of MLLM capabilities. Low correlations indicate that Creation-MMBench measures unique aspects of MLLM performance not captured by the other benchmarks.
read the caption
Figure 10: Redundancy Analysis of Creation-MMBench with other widely used MLLM Benchmarks.
🔼 This histogram displays the distribution of reference answer lengths across different benchmarks, including Creation-MMBench, MM-Vet, MLLM-Bench, AlignMMBench, MEGABench_core, and MEGABench_open. The x-axis represents the length of the reference answers, categorized into bins (e.g., <50, 50-1500, 1500-3000, >3000 tokens), and the y-axis shows the proportion of reference answers falling into each bin. The bars in the histogram visually compare the distribution of reference answer lengths across the different benchmarks.
read the caption
(a) Distribution of reference answers lengths.
🔼 The figure shows a word cloud summarizing the instructions given to large language models (LLMs) in the Creation-MMBench benchmark. The words represent the various tasks or types of creative text generation that the LLMs are instructed to perform, reflecting the diverse range of creative tasks in the benchmark. The size of each word roughly corresponds to its frequency of occurrence in the instructions, indicating the relative emphasis placed on certain types of creative writing tasks.
read the caption
(b) Instructions in Creation-MMBench.
🔼 This figure shows the top 15 image categories used in Creation-MMBench, a multimodal benchmark designed to evaluate the creative capabilities of Multimodal Large Language Models (MLLMs). The categories illustrate the diverse range of visual content included in the benchmark, encompassing various genres, such as animation, people, products, architecture, events, education, art, food and beverages, nature, science and technology, news, user interfaces, interior design, history and culture, and statistical data. This diversity is a key feature of the benchmark, enabling a comprehensive assessment of MLLMs’ ability to handle varied visual inputs and generate creative outputs.
read the caption
(c) Top 15 Image Categories in Creation-MMBench.
🔼 This figure presents supplementary statistics for Creation-MMBench, comparing it against other widely used MLLM benchmarks. Subfigure (a) shows the distribution of reference answer lengths, highlighting that Creation-MMBench features significantly longer answers than others. Subfigure (b) displays a word cloud of the instructions used in Creation-MMBench’s tasks, demonstrating the diversity and complexity of the tasks. Finally, subfigure (c) illustrates the distribution of image categories present in the dataset, emphasizing the rich and diverse visual content.
read the caption
Figure 11: Other Statistics of Creation-MMBench.
🔼 This figure illustrates the process of human pairwise comparison used to evaluate the responses generated by different language models. Specifically, it shows how two model responses are presented side-by-side to human evaluators, along with detailed criteria for comparison. Evaluators are asked to determine which response is superior, or if they are equal. The process is designed to minimize bias by randomly changing the positions of the model responses.
read the caption
Figure 12: The Process of Human Pairwise Comparison.
🔼 Figure 13 presents a qualitative comparison of two different large language models (LLMs), GPT-40-1120 and Qwen2.5-VL-72B, on a software engineering diagram explanation task. The figure showcases how each model interprets a swimlane diagram. Assistant A (GPT-40-1120) accurately identifies the type of diagram, clearly explains its purpose and stages in software development, and provides a comprehensive step-by-step explanation of the credit approval process shown. In contrast, Assistant B (Qwen2.5-VL-72B) misidentifies the diagram type, leading to inaccuracies in its explanation. The evaluation highlights Assistant A’s superior performance due to its correct diagram identification and more thorough explanation.
read the caption
Figure 13: Qualitative Case in Professional Functional Writing. This case comes from Software Engineering Diagram Explanation Task, Assistant A is GPT-4o-1120, assistant B is Qwen2.5-VL-72B.
🔼 This figure showcases a qualitative comparison of two different large language models (LLMs), GPT-40-1120 (Assistant A) and InternVL-2.5-78B (Assistant B), in performing a travel itinerary planning task. The task required generating a 2-day travel plan for art and architecture enthusiasts in Barcelona, utilizing a provided map. The figure highlights the differences in the quality and completeness of the travel plans generated by each model. GPT-40-1120’s plan is presented as superior in organization, detail, and adherence to the user’s preferences.
read the caption
Figure 14: Qualitative Case in Creative Multimodal Understanding. This case comes from Travel Itinerary Planning and Recommendations Task, Assistant A is GPT-4o-1120, assistant B is InternVL2.5-78B.
🔼 This figure shows an example from the Creation-MMBench dataset, specifically from the ‘Story continue’ task within the Literary Writing category. It displays a series of images from a children’s animation, along with the task prompt, requirements, and evaluation criteria. The task requires the model to continue the story based on the provided images. The evaluation criteria assess aspects like narrative coherence, vivid descriptions, character consistency, and the introduction of new challenges.
read the caption
Figure 15: Example Case of Literary Writing, from Task story continue.
🔼 This figure shows an example of a creative writing task from the Creation-MMBench benchmark. The task is to generate a daily conversation between two people based on a given image. The figure displays the image used in the task, and excerpts from two model responses, demonstrating the diversity of outputs that can be achieved. One response is marked as significantly better, illustrating the benchmark’s ability to differentiate between various levels of creative writing quality. The criteria used to assess each response are also presented.
read the caption
Figure 16: Example Case of Literary Writing, from Task daily conversation creation.
🔼 This figure shows an example of a literary writing task from the Creation-MMBench benchmark. The task was ’landscape to poem.’ It displays the prompt given to the large language model (LLM), including instructions and evaluation criteria, along with two example responses (Assistant A and Assistant B) from different LLMs. The evaluation criteria included subjective aspects like coherence and the use of imagery, as well as objective aspects such as adherence to the sonnet form. The image used in the prompt is also included, to show how the generated poems should reflect the visual aspects of the image. The goal of this example is to demonstrate the capability of Creation-MMBench to evaluate the creative writing abilities of LLMs in response to visual prompts.
read the caption
Figure 17: Example Case of Literary Writing, from Task landscape to poem.
🔼 This figure displays an example from the Creation-MMBench benchmark dataset, specifically showcasing a task that involves generating a historical narrative based on a provided image. The image in question shows a photograph from 1944 of the Central Epidemic Prevention Office in Xishan, Kunming, Yunnan Province. The task requires the model to create a story based on this image, incorporating relevant historical context and imagining the life experiences of the people depicted. The criteria for evaluation include historical accuracy, emotional engagement, and narrative connection to the image itself. The response should create a compelling story that is both historically grounded and emotionally resonant.
read the caption
Figure 18: Example Case of Literary Writing, from Task historical story creation.
🔼 This figure shows an example of a response from the Common Functional Writing task in Creation-MMBench. The specific task is to write a Facebook post about a daily achievement: receiving a certificate of achievement. The figure displays the images used as input to the model, the prompt given to the model, and the generated response (Facebook post). The response includes a description of the event, the student’s reflections on their achievement, and the significance of receiving the award.
read the caption
Figure 19: Example Case of Common Functional Writing, from Task daily achievement show off.
🔼 Figure 20 shows an example from the Creation-MMBench dataset, specifically from the Common Functional Writing section. It presents a Reddit post written by an LLM in response to an image prompt depicting a trip to Krakow, Poland. The goal of this task is to assess the LLM’s ability to generate engaging and informative social media content based on visual input. The figure showcases the LLM’s response, along with the evaluation criteria used to assess aspects like engagement, clarity, and factual accuracy related to the image.
read the caption
Figure 20: Example Case of Common Functional Writing, from Task social media travel content.
🔼 This figure shows an example from the Common Functional Writing section of the Creation-MMBench benchmark. It specifically illustrates a task where the model is asked to compose a Reddit post seeking help with a technical problem. The image shows a screenshot of an iPhone with several apps frozen. The associated text provides detailed criteria for evaluating the generated Reddit post, including aspects like clarity, tone, and accuracy of problem description. It highlights the multimodal nature of the Creation-MMBench by incorporating visual information into a creative writing task.
read the caption
Figure 21: Example Case of Common Functional Writing, from Task daily affairs inquiries.
🔼 This figure shows an example from the Creation-MMBench dataset, specifically from the ‘personal event summaries’ task within the Common Functional Writing category. It displays the Reddit year-end report of a user, including their top categories (Psychology, Q&As, and Memes), a significant post, and engagement metrics. The task requires generating a blog post summarizing this report, explaining a user’s question about placing an object on Mars, and encouraging interaction.
read the caption
Figure 22: Example Case of Common Functional Writing, from Task personal event summaries.
🔼 This figure shows an example of a professional functional writing task from the Creation-MMBench benchmark. It displays a teaching plan created by an AI model for a biology lesson on prokaryotic cells. The plan includes teaching objectives, a breakdown of lesson sections with specific content, and an estimated time allocation for each section. The plan also demonstrates consideration of differentiated instruction for different student levels using visual materials from the textbook. This example showcases the complexity and detailed evaluation criteria involved in assessing AI’s ability to generate functional and coherent text suitable for real-world applications.
read the caption
Figure 23: Example Case of Professional Functional Writing, from Task teaching plan.
🔼 Figure 24 shows an example from the Creation-MMBench benchmark’s ‘Professional Functional Writing’ task, specifically the ‘Product marketing strategy’ subtask. It presents a case where a model generates a marketing strategy and promotional copy for Estee Lauder skincare products targeting the Asia-Pacific market. The figure highlights the model’s response, the evaluation criteria (both general subjective and visual factuality criteria), and the visual input (images of Estee Lauder products). It illustrates how the benchmark evaluates both the creativity and factual accuracy of MLLM responses in a professional context.
read the caption
Figure 24: Example Case of Professional Functional Writing, from Task product marketing strategy.
🔼 This figure shows an example from the Creation-MMBench dataset, specifically from the ‘Professional Functional Writing’ task category focusing on ’nutritional formulation of recipe’. It presents the prompt given to the model, the model’s response, evaluation criteria (both general subjective and visual factuality), and the scoring of the model’s response. The prompt requires the model to analyze a recipe (shown in an image), identify its advantages and disadvantages for a marathon runner, and suggest supplementary foods to optimize the diet. The evaluation criteria assess the completeness and accuracy of the analysis, the depth of nutritional insights provided, and the alignment of the response with the dish description and nutritional needs. This demonstrates the benchmark’s ability to evaluate nuanced, practical creative tasks involving multimodal reasoning.
read the caption
Figure 25: Example Case of Professional Functional Writing, from Task nutritional formulation of recipe.
🔼 Figure 26 presents an example from the ‘Professional Functional Writing’ task category, specifically focusing on ‘clothing match design’. It showcases a response from a model to a prompt requesting a clothing outfit suitable for working from home while remaining presentable for video calls. The figure highlights the model’s ability to propose a well-reasoned outfit considering both comfort and professionalism, along with detailed explanations justifying the choices made.
read the caption
Figure 26: Example Case of Professional Functional Writing, from Task clothing match design.
More on tables
Model | Overall | LW | CFW | PFW | CMU | OC Score | Avg Tokens | |||||
VFS | Reward | VFS | Reward | VFS | Reward | VFS | Reward | VFS | Reward | |||
Proprietary MLLMs | ||||||||||||
Gemini-2.0-pro-exp | 8.53 | 4.48 | 8.66 | -1.88 | 8.98 | 12.71 | 8.01 | 3.33 | 8.65 | -8.06 | 73.4 | 718 |
\hdashlineGPT-4o-1120[Baseline] | 8.72 | 0.00 | 8.86 | 0.00 | 8.93 | 0.00 | 8.26 | 0.00 | 9.38 | 0.00 | 72.0 | 497 |
\hdashlineGemini-1.5-pro-002 | 8.41 | -5.49 | 8.66 | -6.04 | 8.59 | -2.04 | 8.05 | -4.82 | 8.75 | -17.22 | 72.2 | 444 |
GPT-4.5-0227 | 8.54 | -5.88 | 8.63 | -4.38 | 8.76 | -8.33 | 8.05 | -5.88 | 9.29 | -0.56 | / | 394 |
GPT-4o-mini | 8.07 | -13.56 | 8.30 | -4.38 | 8.44 | -15.28 | 7.50 | -16.05 | 8.40 | -12.78 | 64.1 | 436 |
Doubao-VL | 8.38 | -14.09 | 8.28 | -19.17 | 9.01 | -3.33 | 7.65 | -18.72 | 8.77 | -25.00 | / | 516 |
Claude-3.5-Sonnet | 7.96 | -15.46 | 8.44 | -16.46 | 7.45 | -21.57 | 7.98 | -11.14 | 8.88 | -9.44 | 70.6 | 336 |
Moonshot-v1-32k-vision | 7.43 | -20.58 | 7.30 | -21.46 | 8.20 | -8.80 | 6.91 | -26.50 | 6.91 | -36.11 | / | 485 |
Open-Source MLLMs | ||||||||||||
Qwen2.5-VL-72B-Instruct | 8.33 | -5.82 | 8.04 | -10.83 | 8.91 | 4.44 | 7.68 | -11.49 | 8.86 | -11.94 | 76.1 | 553 |
InternVL2.5-78B-MPO | 8.06 | -12.55 | 8.22 | -9.17 | 8.60 | -5.00 | 7.45 | -16.32 | 8.22 | -27.78 | 77.0 | 461 |
InternVL2.5-8B-MPO | 7.65 | -15.10 | 8.09 | -16.25 | 8.30 | -3.80 | 6.80 | -23.95 | 7.88 | -19.44 | 70.3 | 548 |
InternVL2.5-78B | 7.91 | -16.43 | 8.05 | -17.50 | 8.45 | -7.69 | 7.26 | -20.53 | 8.18 | -28.33 | 75.2 | 473 |
Qwen2-VL-72B-instruct | 7.87 | -22.45 | 7.75 | -24.58 | 8.17 | -15.56 | 7.42 | -26.84 | 8.43 | -26.39 | 74.8 | 439 |
InternVL2.5-8B | 7.38 | -25.42 | 7.91 | -23.33 | 7.95 | -15.83 | 6.62 | -33.95 | 7.45 | -30.00 | 68.1 | 500 |
Qwen2.5-VL-7B-Instruct | 7.55 | -29.80 | 7.34 | -39.38 | 8.40 | -21.67 | 6.71 | -33.25 | 7.78 | -30.56 | 70.9 | 510 |
MiniCPM-o-2.6 | 7.49 | -34.77 | 7.79 | -35.42 | 7.95 | -27.31 | 6.76 | -40.88 | 8.08 | -36.94 | 70.2 | 389 |
DeepSeek-VL2 | 7.24 | -38.52 | 7.58 | -33.75 | 7.58 | -32.50 | 6.61 | -44.02 | 7.81 | -45.56 | 66.4 | 440 |
LLaVA-OneVision-72B | 7.16 | -39.87 | 7.26 | -36.32 | 7.72 | -30.61 | 6.43 | -47.98 | 7.62 | -46.37 | 68.0 | 315 |
LLaVA-OneVision-7B | 6.75 | -43.49 | 7.36 | -43.54 | 7.27 | -31.85 | 6.04 | -50.53 | 6.82 | -56.11 | 60.2 | 373 |
Qwen2-VL-7B-instruct | 7.12 | -43.76 | 6.99 | -55.83 | 7.67 | -36.30 | 6.57 | -45.26 | 7.25 | -45.28 | 67.1 | 456 |
🔼 This table presents a comprehensive evaluation of various Multimodal Large Language Models (MLLMs) on the Creation-MMBench benchmark. It shows the overall performance, broken down by four task categories: Literary Writing (LW), Common Functional Writing (CFW), Professional Functional Writing (PFW), and Creative Multimodal Understanding (CMU). Key metrics include the Visual Factuality Score (VFS), which measures the accuracy of the model’s responses in relation to the provided visual input, and a Reward score, reflecting the overall quality of the model’s creative output. The table also includes the average score from the OpenVLM Leaderboard (OC Score), which provides a measure of the model’s objective performance, as well as the average number of tokens generated by each model. The token count is calculated using the tiktoken GPT-4o-1120 tokenizer.
read the caption
Table 2: Evaluation Result of MLLMs on Creation-MMBench. VFS stands for Visual Factuality Score. LW, CFW, PFW, and CMU stand for four categories in Creation-MMBench: Literary Writing, Common Functional Writing, Professional Functional Writing, and Creative Multimodal Understanding. OC Score represents the average score of the OpenVLM Leaderboard and mainly demonstrates the objective performance of the model. The token number is calculated with tiktoken GPT-4o-1120 tokenizer.
VLM | Corresponding LLM | Text Input w. LLM | Text Input w. VLM | Vision+Text Input w. VLM | |||
VFS | Reward | VFS | Reward | VFS | Reward | ||
GPT-4o-1120 | GPT-4o-1120 | 8.71 | 6.96 | 8.71 | 6.96 | 8.72 | 0.36 |
Gemini-2.0-pro-exp | Gemini-2.0-pro-exp | 8.49 | 4.08 | 8.49 | 4.08 | 8.53 | 4.48 |
Qwen2.5-VL-72B-Instruct | Qwen2.5-72B-Instruct | 8.55 | 0.82 | 8.51 | -4.05 | 8.33 | -5.82 |
Qwen2.5-VL-7B-Instruct | Qwen2.5-7B-Instruct | 8.18 | -19.18 | 7.97 | -27.50 | 7.55 | -29.80 |
MiniCPM-o-2.6 | Qwen2.5-7B-Instruct | 8.18 | -19.18 | 7.78 | -36.57 | 7.49 | -34.77 |
InternVL2.5-8B | InternLM2.5-7B-Chat | 7.83 | -22.19 | 7.92 | -28.73 | 7.38 | -25.42 |
🔼 Table 3 presents the performance comparison between LLMs and VLMs on the Creation-MMBench-TO benchmark. Creation-MMBench-TO is a text-only variant of the Creation-MMBench benchmark, where image inputs are replaced with textual descriptions. The table shows the performance of several LLMs and VLMs in terms of visual factuality scores (VFS) and reward scores. GPT-4 was used to generate general image descriptions for all models. For proprietary models, their own text-based versions were used for a fairer comparison of performance when incorporating visual information. The results highlight how visual instruction tuning can impact the creative capabilities of VLMs.
read the caption
Table 3: LLM performance on Creation-MMBench-TO and Visual Instruction Tuning Impact on VLM creation capability. The image descriptions provided by GPT-4o are general. For the proprietary models, we point to themselves as corresponding LLM and report the performance with image descriptions and questions.
Judger | MLLM | Dual Eval | Single Eval | ||||||
MAE | Cons. | MAE | Cons. | ||||||
Gemini-2P | Gemini | 0.65 | 0.59 | 82.83 | 86.67 | 0.78 | 0.72 | 74.75 | 78.67 |
Qwen | 0.51 | 91.00 | 0.67 | 80.00 | |||||
MiniCPM | 0.61 | 86.14 | 0.69 | 81.19 | |||||
Claude-3.5 | Gemini | 0.56 | 0.50 | 89.90 | 90.60 | 0.61 | 0.59 | 83.84 | 85.23 |
Qwen | 0.46 | 92.00 | 0.59 | 85.00 | |||||
MiniCPM | 0.47 | 89.90 | 0.57 | 86.87 | |||||
GPT-4o | Gemini | 0.53 | 0.50 | 92.08 | 92.13 | 0.57 | 0.54 | 89.11 | 88.85 |
Qwen | 0.42 | 96.08 | 0.46 | 91.18 | |||||
MiniCPM | 0.53 | 88.24 | 0.59 | 86.27 |
🔼 This table presents a comparison of different evaluation strategies for assessing the creative capabilities of large language models (LLMs). It examines the alignment between evaluation methods (using different LLMs as judges) and human preferences. The table quantifies the alignment using Mean Absolute Error (MAE) and Consistency, which measures how closely automated evaluations match human judgments of creative quality. The results provide insights into the effectiveness and reliability of different approaches for evaluating LLM creativity.
read the caption
Table 4: The Alignment Between Different Evaluation Strategies and Human Preference.
Model | VFS | Reward | WR | MB | Better | Tie | Worse | MW | Fail |
Proprietary MLLMs | |||||||||
Gemini-2.0-pro-exp | 8.53 | 4.48 | 26.75 | 9 | 400 | 898 | 163 | 59 | 1 |
GPT-4o-1120 | 8.72 | 0.00 | - | - | - | - | - | - | - |
Gemini-1.5-pro-002 | 8.41 | -5.49 | 11.37 | 6 | 168 | 1032 | 300 | 24 | 0 |
GPT-4.5-0227 | 8.54 | -5.88 | 5.36 | 7 | 75 | 1186 | 255 | 7 | 0 |
GPT-4o-mini | 8.07 | -13.56 | 3.79 | 5 | 53 | 1022 | 422 | 28 | 0 |
Doubao-VL | 8.38 | -14.09 | 9.22 | 4 | 137 | 850 | 500 | 38 | 1 |
Claude-3.5-Sonnet | 7.96 | -15.46 | 12.55 | 4 | 188 | 843 | 321 | 174 | 0 |
Moonshot-v1-32k-vision | 7.43 | -20.58 | 6.09 | 1 | 92 | 822 | 500 | 111 | 4 |
Open-Source MLLMs | |||||||||
Qwen2.5-VL-72B-Instruct | 8.33 | -5.82 | 13.2 | 6 | 196 | 984 | 302 | 42 | 0 |
InternVL2.5-78B-MPO | 8.06 | -12.55 | 8.76 | 6 | 128 | 917 | 434 | 45 | 0 |
InternVL2.5-8B-MPO | 7.65 | -15.10 | 10.33 | 0 | 158 | 843 | 438 | 91 | 0 |
InternVL2.5-78B | 7.91 | -16.43 | 7.25 | 4 | 107 | 863 | 494 | 62 | 0 |
Qwen2-VL-72B-instruct | 7.87 | -22.45 | 4.64 | 0 | 71 | 764 | 632 | 63 | 0 |
InternVL2.5-8B | 7.38 | -25.42 | 5.62 | 2 | 84 | 699 | 624 | 121 | 0 |
Qwen2.5-VL-7B-Instruct | 7.55 | -29.80 | 4.25 | 0 | 65 | 620 | 713 | 132 | 0 |
MiniCPM-o-2.6 | 7.49 | -34.77 | 2.29 | 2 | 33 | 545 | 799 | 151 | 0 |
DeepSeek-VL2 | 7.24 | -38.52 | 1.77 | 0 | 27 | 504 | 791 | 207 | 1 |
LLaVA-OneVision-72B | 7.16 | -39.87 | 1.72 | 0 | 26 | 448 | 842 | 194 | 20 |
LLaVA-OneVision-7B | 6.75 | -43.49 | 1.96 | 1 | 29 | 411 | 816 | 273 | 0 |
Qwen2-VL-7B-instruct | 7.12 | -43.76 | 1.57 | 0 | 24 | 402 | 845 | 259 | 0 |
🔼 This table presents the win rates of various Multimodal Large Language Models (MLLMs) on the Creation-MMBench benchmark. The win rate is calculated by comparing the model’s responses to those of a baseline model (GPT-40-1120), using human judges to determine which response is superior. The table also breaks down the win rate into categories: ‘Much Better’, ‘Better’, ‘Tie’, ‘Worse’, and ‘Much Worse’, indicating the relative quality of the model’s performance. This allows for a nuanced understanding of each MLLM’s creative capabilities beyond just a simple win/loss metric.
read the caption
Table 5: Win Rate Result of MLLMs on Creation-MMBench. WR, MB, MW stands for Win Rate, Much Better and Much Worse
LLM | Generic | Query-Specific | ||
VFS | Reward | VFS | Reward | |
GPT-4o-1120 | 8.71 | 6.96 | 8.88 | 3.33 |
Qwen2.5-72B-Instruct | 8.55 | 0.82 | 8.82 | 4.80 |
InternLM2.5-7B-Chat | 7.83 | -22.19 | 8.33 | -15.29 |
🔼 This table presents a comparison of Large Language Model (LLM) performance on the Creation-MMBench-TO benchmark using two different types of image descriptions: generic and query-specific. Generic descriptions provide general overviews of images, while query-specific descriptions are tailored to the specific questions asked. The table shows the Visual Factuality Score (VFS) and Reward values for each LLM and description type, enabling a direct comparison of model performance under different visual input conditions. This allows for an assessment of how well the models integrate visual information based on the level of specificity within the description.
read the caption
Table 6: Comparison on Generic Descriptions and Query-Specific Descriptions on Creation-MMBench-TO.
Judging Method | Judging Model/Human | MLLM | Dual Evaluation | Non-Dual Evaluation | ||||||
MAE | Consistency | MAE | Consistency | |||||||
LLM-as-a-judge | Gemini-2.0-Pro | Gemini-1.5-pro-002 | 0.67 | 0.62 | 83.17 | 84.16 | 0.75 | 0.69 | 77.23 | 79.21 |
Qwen2-VL-72B | 0.59 | 84.16 | 0.65 | 78.22 | ||||||
MiniCPM-o-2.6 | 0.61 | 85.15 | 0.67 | 82.18 | ||||||
GPT-4o-mini | Gemini-1.5-pro-002 | 0.67 | 0.59 | 83.17 | 86.23 | 0.79 | 0.71 | 74.26 | 77.38 | |
Qwen2-VL-72B | 0.59 | 85.29 | 0.67 | 76.47 | ||||||
MiniCPM-o-2.6 | 0.52 | 90.20 | 0.66 | 81.37 | ||||||
Claude-3.5-Sonnet | Gemini-1.5-pro-002 | 0.63 | 0.52 | 89.11 | 91.80 | 0.73 | 0.63 | 78.22 | 81.97 | |
Qwen2-VL-72B | 0.46 | 94.12 | 0.58 | 82.35 | ||||||
MiniCPM-o-2.6 | 0.46 | 92.16 | 0.58 | 85.29 | ||||||
GPT-4o | Gemini-1.5-pro-002 | 0.56 | 0.51 | 93.07 | 91.48 | 0.56 | 0.56 | 90.10 | 87.54 | |
Qwen2-VL-72B | 0.46 | 92.16 | 0.54 | 87.25 | ||||||
MiniCPM-o-2.6 | 0.51 | 89.22 | 0.58 | 85.29 | ||||||
MLLM-as-a-judge | Gemini-2.0-Pro | Gemini-1.5-pro-002 | 0.65 | 0.59 | 82.83 | 86.67 | 0.78 | 0.72 | 74.75 | 78.67 |
Qwen2-VL-72B | 0.51 | 91.00 | 0.67 | 80.00 | ||||||
MiniCPM-o-2.6 | 0.61 | 86.14 | 0.69 | 81.19 | ||||||
GPT-4o-mini | Gemini-1.5-pro-002 | 0.64 | 0.55 | 84.16 | 89.51 | 0.71 | 0.66 | 76.24 | 80.33 | |
Qwen2-VL-72B | 0.53 | 93.14 | 0.65 | 82.35 | ||||||
MiniCPM-o-2.6 | 0.49 | 91.18 | 0.61 | 82.35 | ||||||
Claude-3.5-Sonnet | Gemini-1.5-pro-002 | 0.56 | 0.50 | 89.90 | 90.60 | 0.61 | 0.59 | 83.84 | 85.23 | |
Qwen2-VL-72B | 0.46 | 92.00 | 0.59 | 85.00 | ||||||
MiniCPM-o-2.6 | 0.47 | 89.90 | 0.57 | 86.87 | ||||||
GPT-4o | Gemini-1.5-pro-002 | 0.53 | 0.50 | 92.08 | 92.13 | 0.57 | 0.54 | 89.11 | 88.85 | |
Qwen2-VL-72B | 0.42 | 96.08 | 0.46 | 91.18 | ||||||
MiniCPM-o-2.6 | 0.53 | 88.24 | 0.59 | 86.27 | ||||||
Human-as-a-judge | H1 | Gemini-1.5-pro-002 | / | / | / | / | 0.65 | 0.64 | 84.16 | 87.21 |
Qwen2-VL-72B | / | / | 0.60 | 90.20 | ||||||
MiniCPM-o-2.6 | / | / | 0.66 | 87.25 | ||||||
H2 | Gemini-1.5-pro-002 | / | / | / | / | 0.82 | 0.75 | 74.26 | 78.69 | |
Qwen2-VL-72B | / | / | 0.72 | 82.35 | ||||||
MiniCPM-o-2.6 | / | / | 0.73 | 79.41 | ||||||
H3 | Gemini-1.5-pro-002 | / | / | / | / | 0.74 | 0.68 | 76.24 | 82.30 | |
Qwen2-VL-72B | / | / | 0.62 | 80.39 | ||||||
MiniCPM-o-2.6 | / | / | 0.72 | 90.20 | ||||||
H4 | Gemini-1.5-pro-002 | / | / | / | / | 0.64 | 0.63 | 87.13 | 87.87 | |
Qwen2-VL-72B | / | / | 0.61 | 89.22 | ||||||
MiniCPM-o-2.6 | / | / | 0.65 | 87.25 |
🔼 This table presents a comprehensive analysis of the alignment between model-generated evaluations and human judgments. It compares the Mean Absolute Error (MAE) and the percentage of consistent judgments between different model-based evaluation strategies and human evaluations. Specifically, it shows the results of using various LLMs as judges for comparing responses, examining the level of agreement between these machine-based assessments and human preferences. It also provides the degree of consistency in human judgments themselves to serve as a baseline for comparison. The results help to establish the reliability and validity of the proposed MLLM-as-a-judge approach within the Creation-MMBench evaluation framework.
read the caption
Table 7: The Results of Model-Human Alignment and Human-Human Alignment.
Full paper#
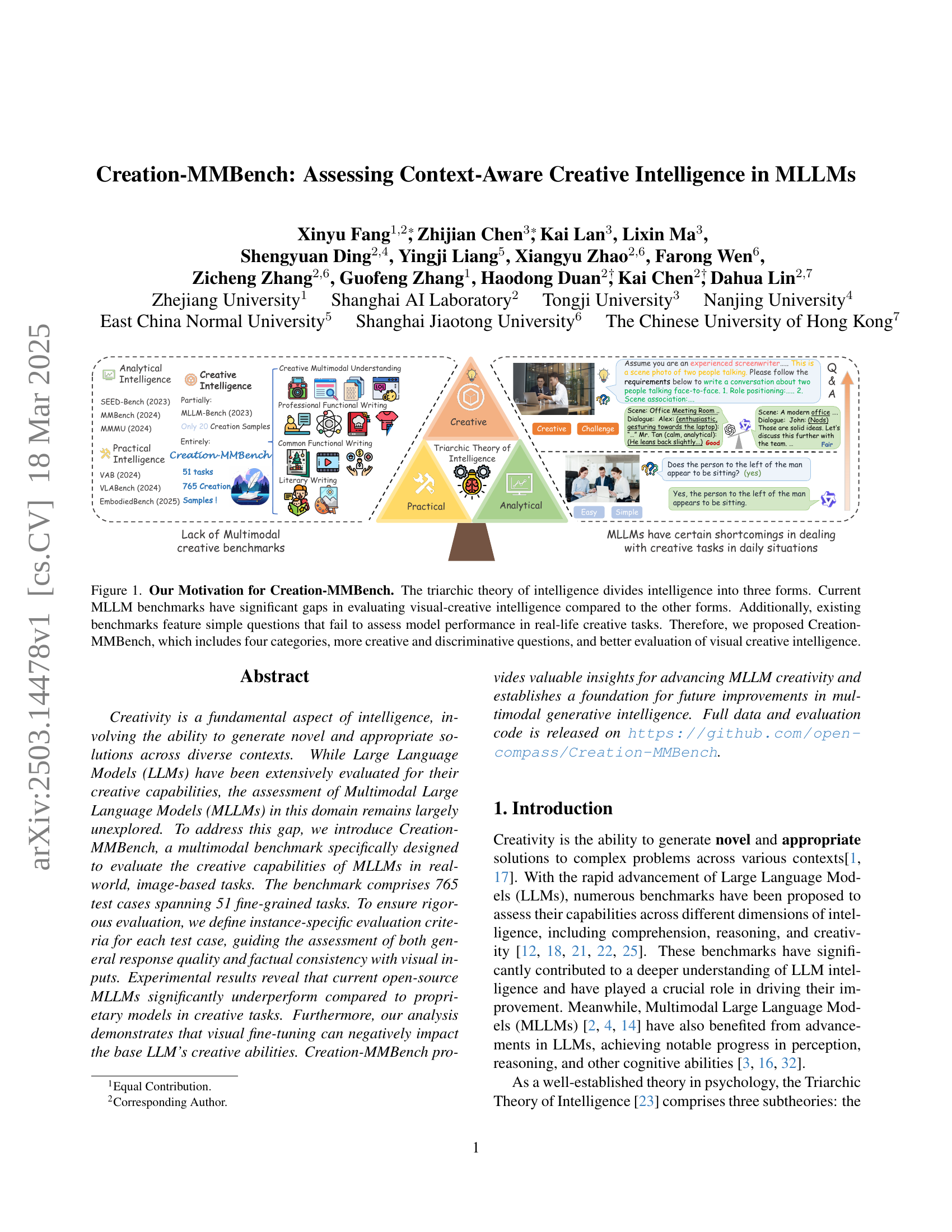
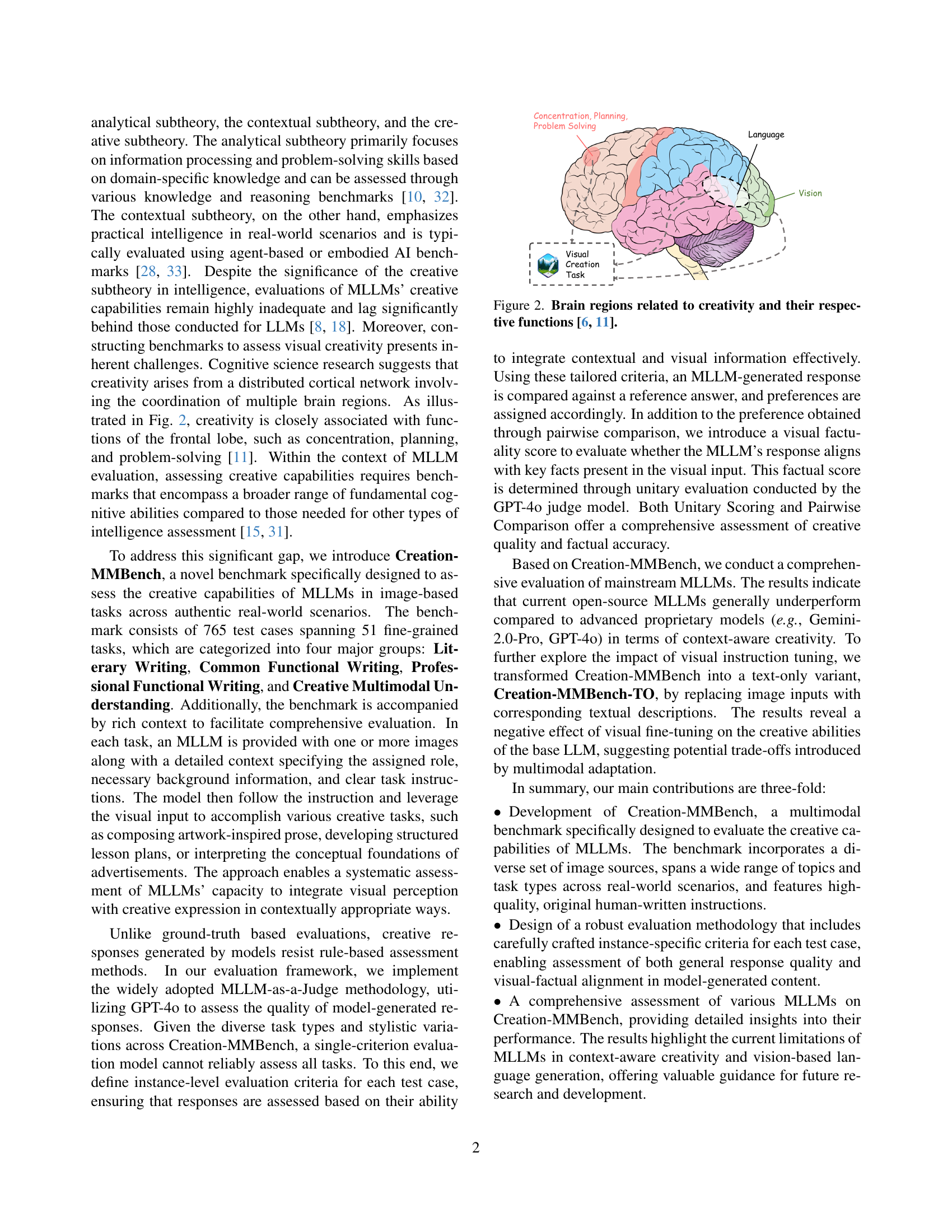
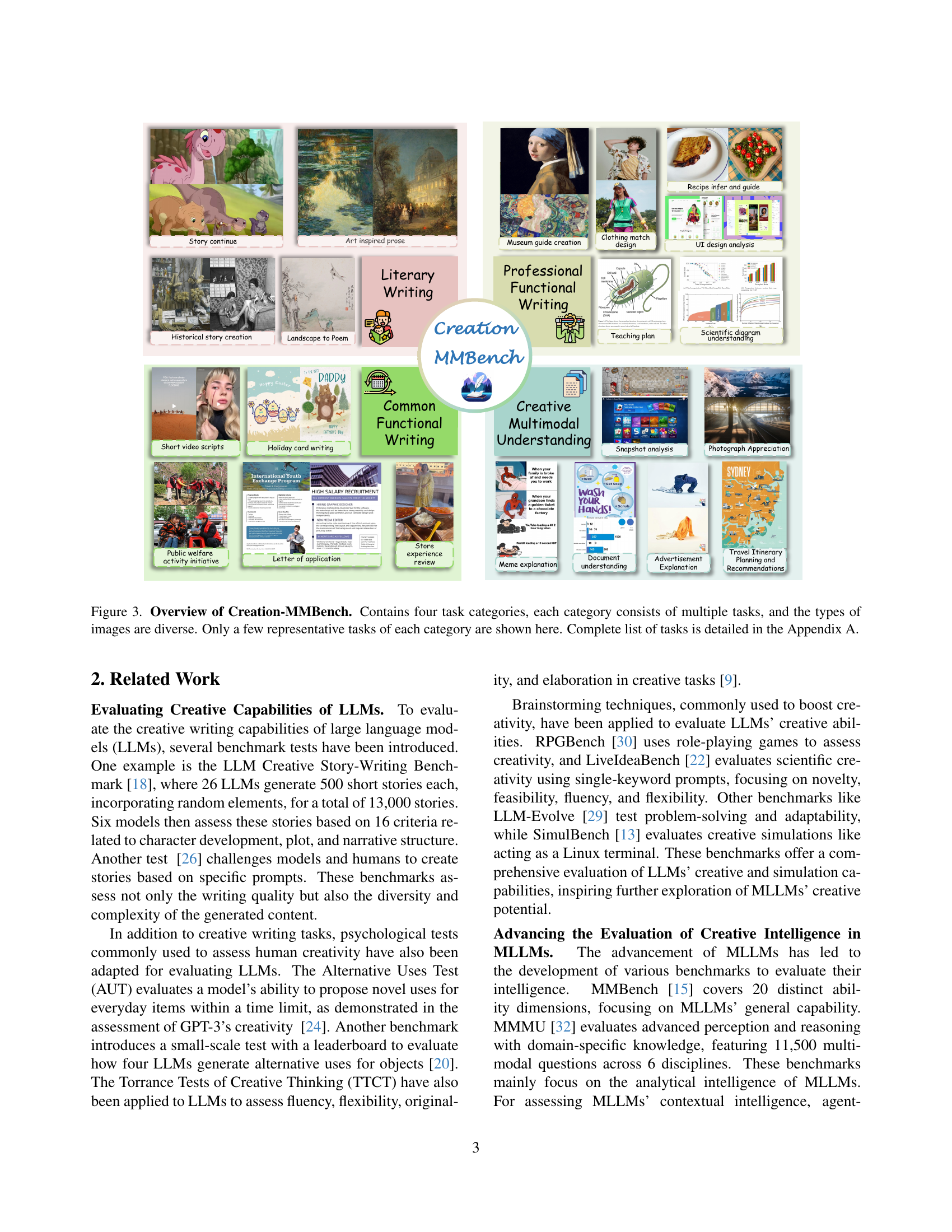
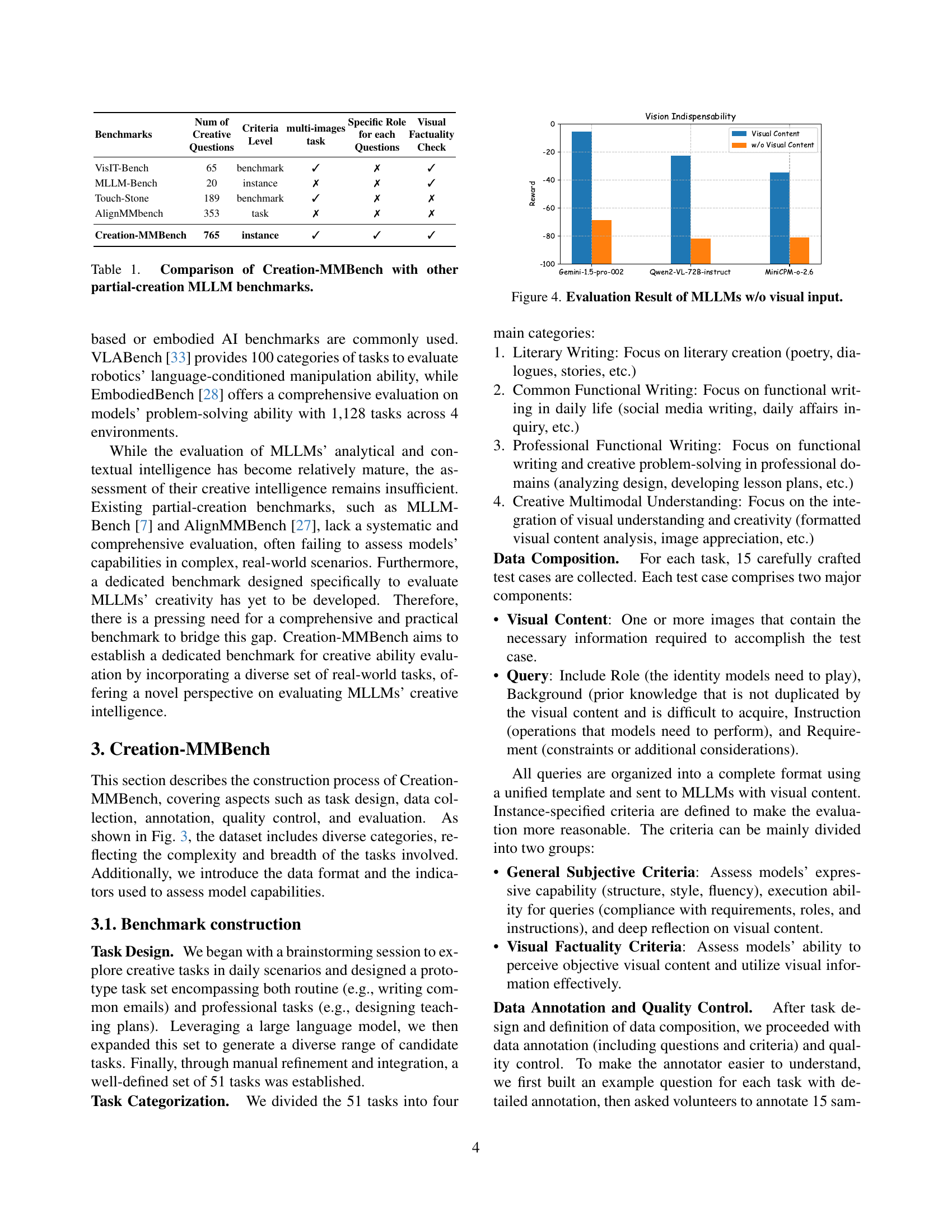
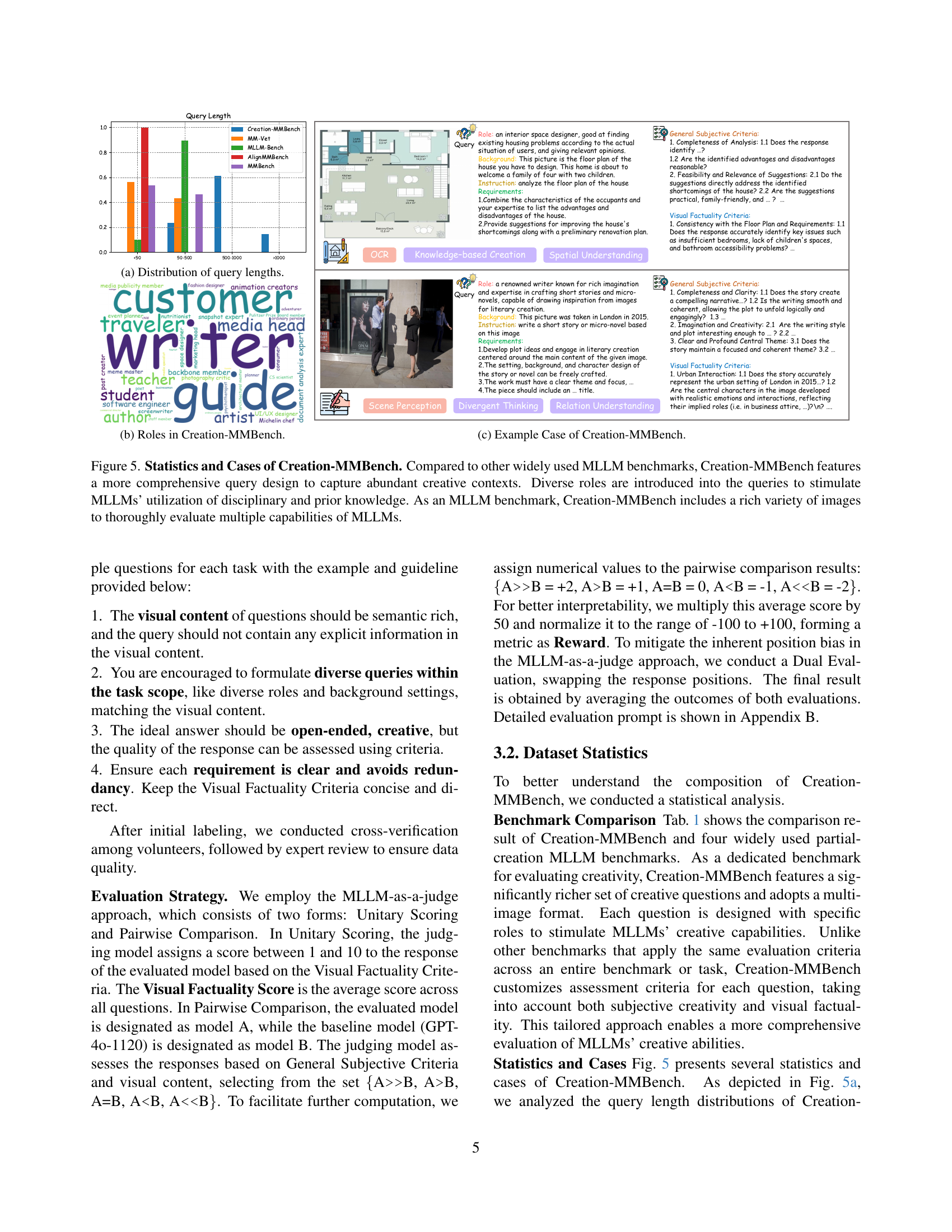
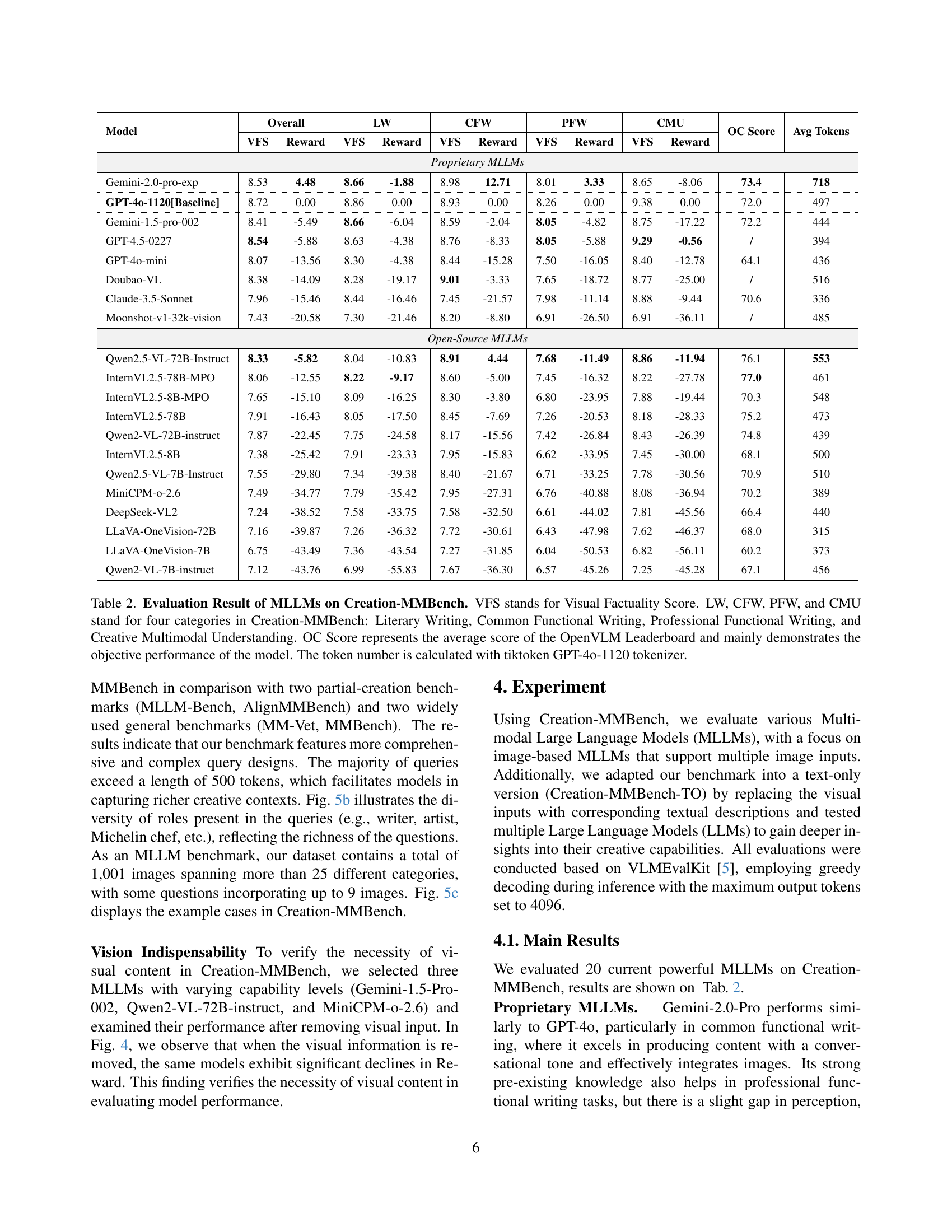
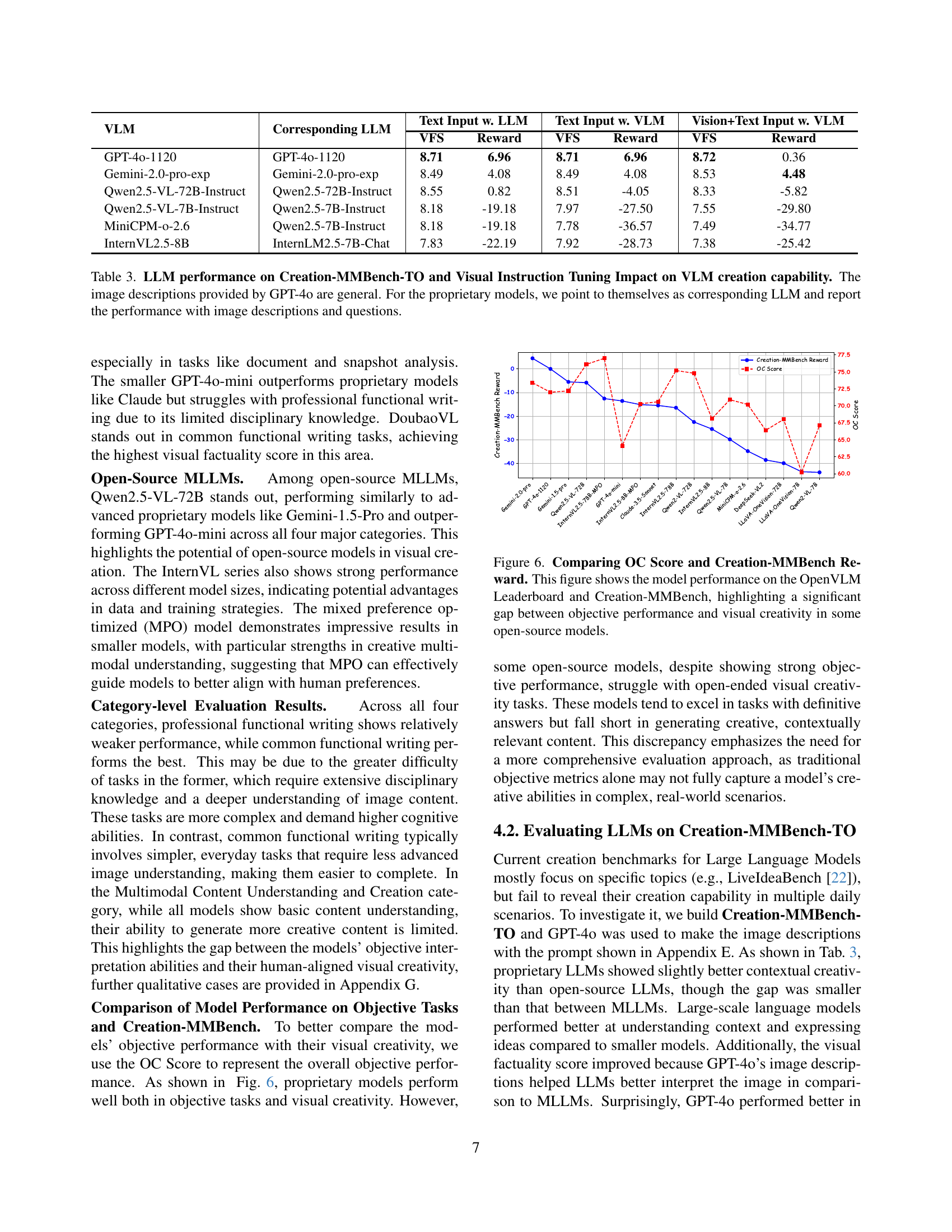
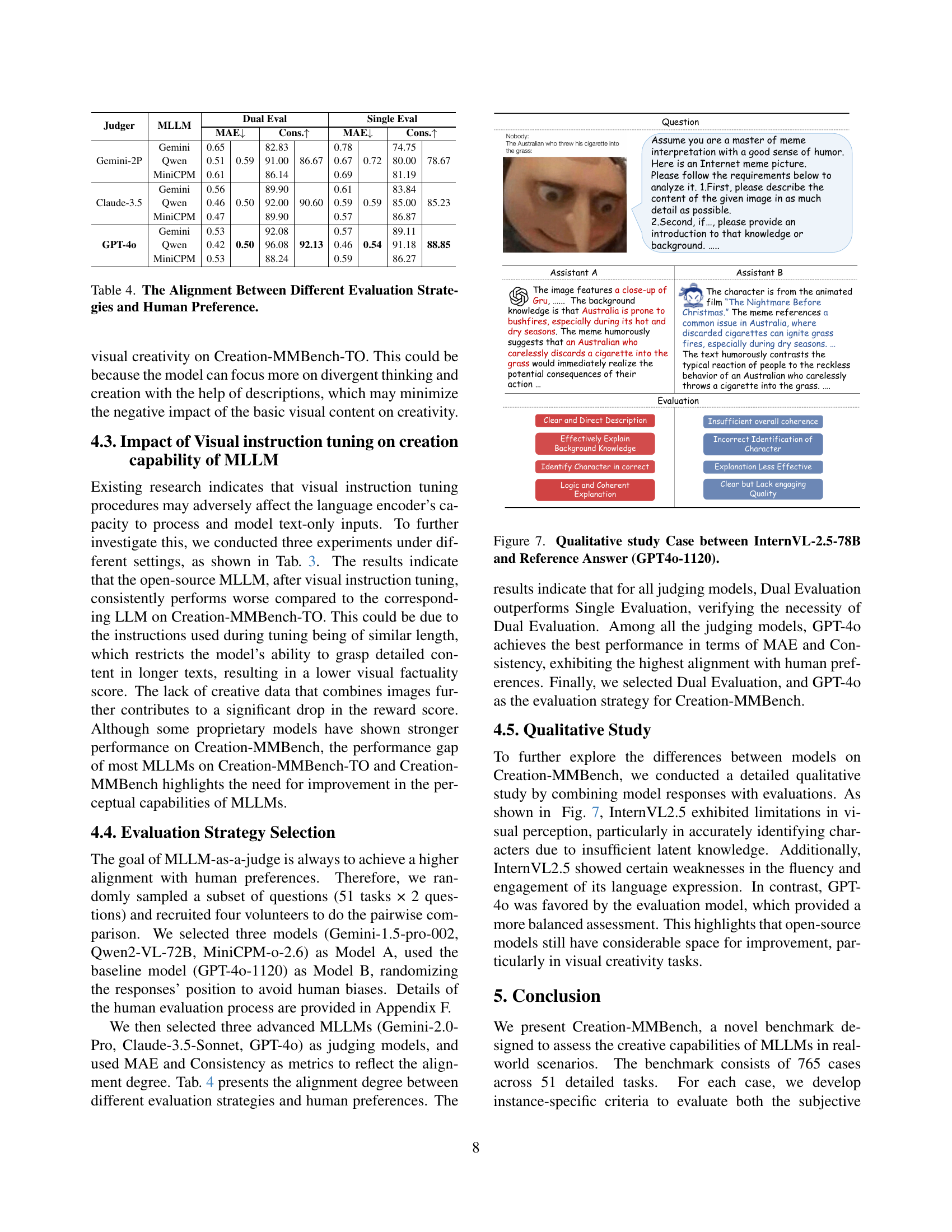
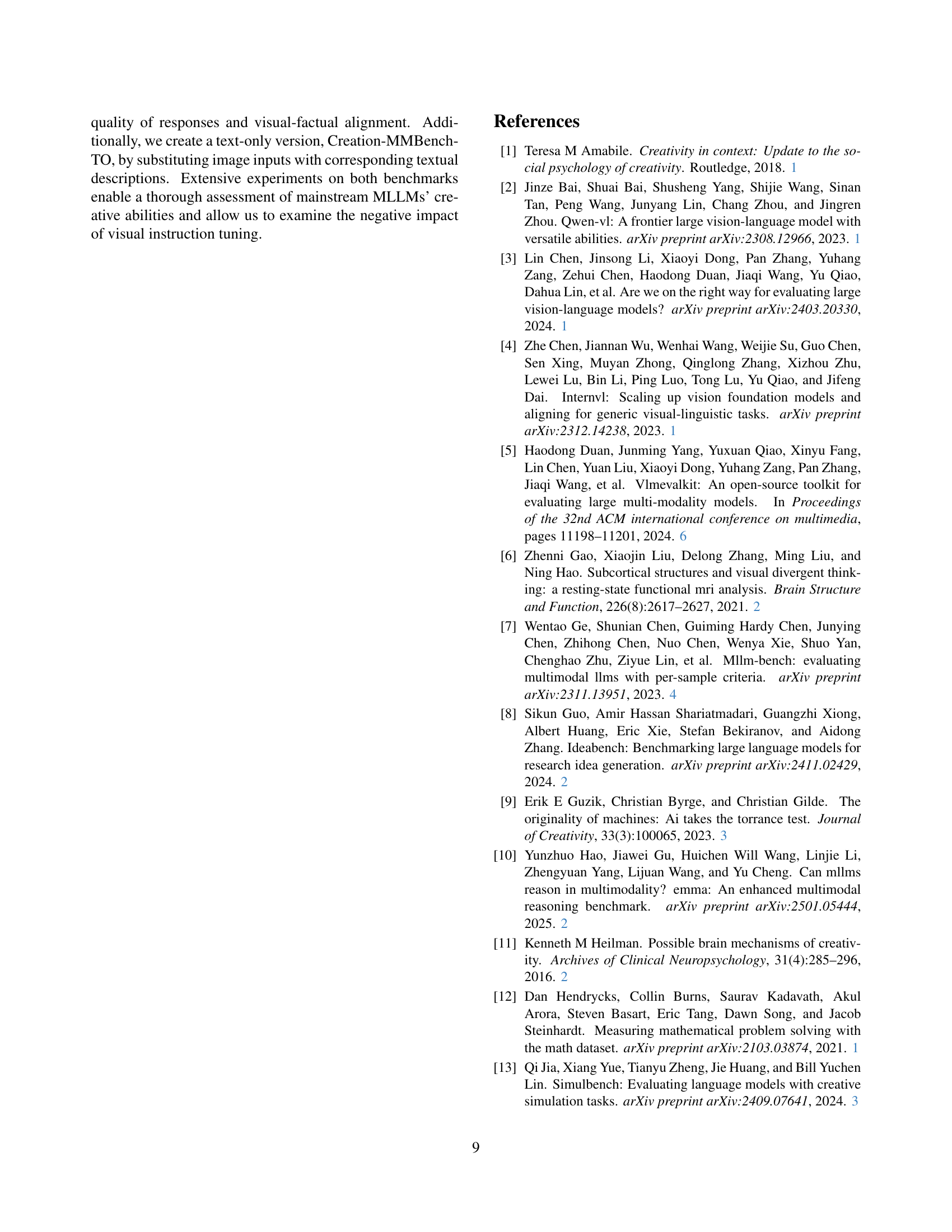
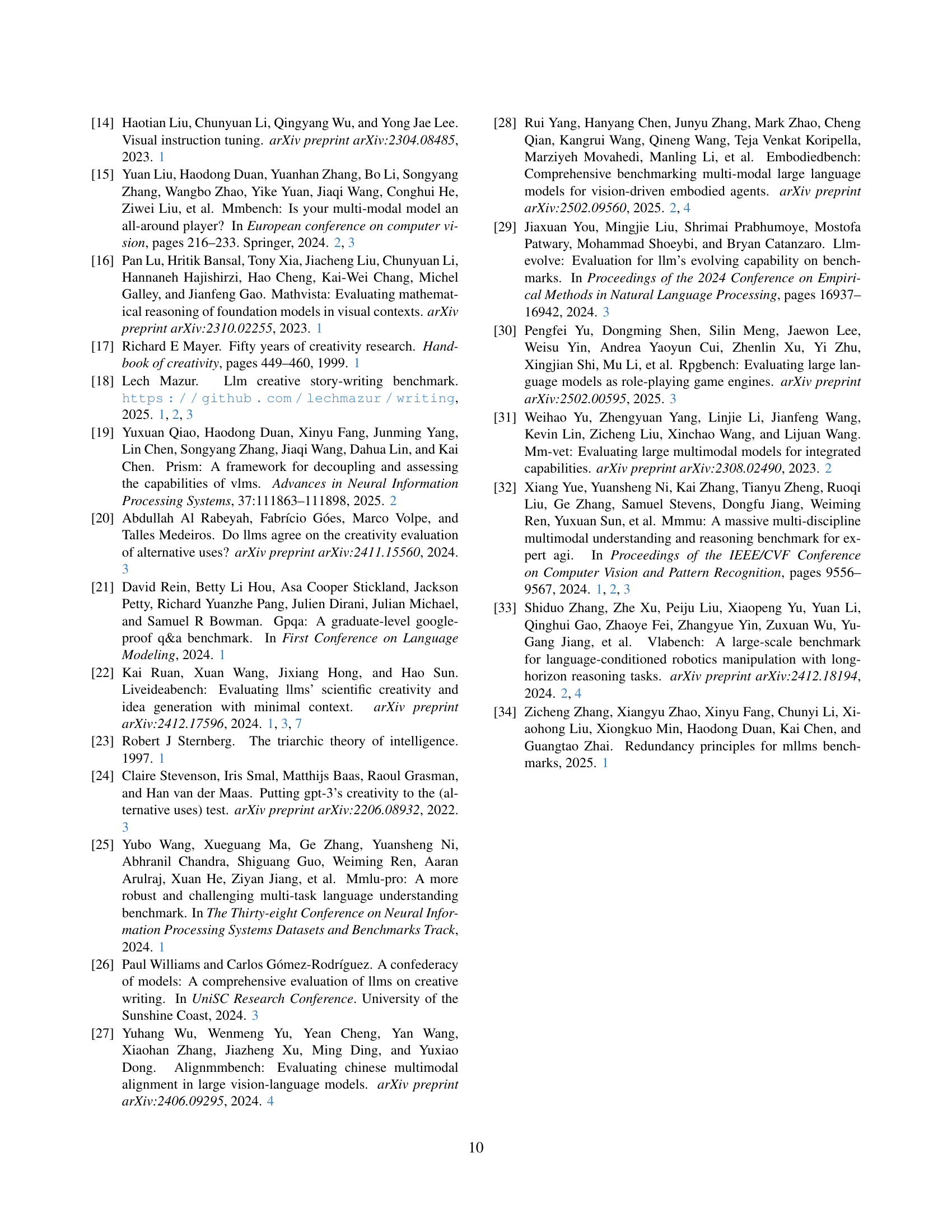
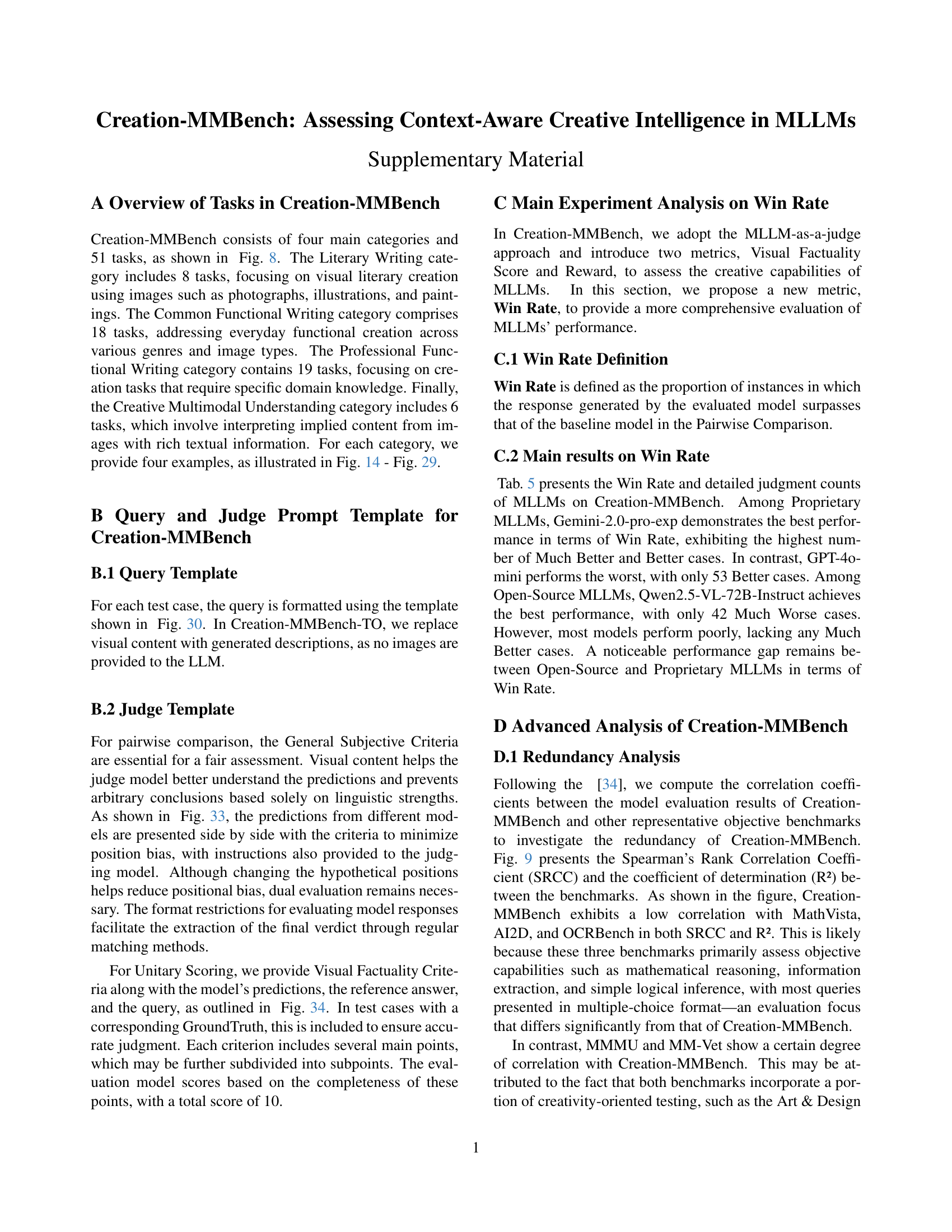
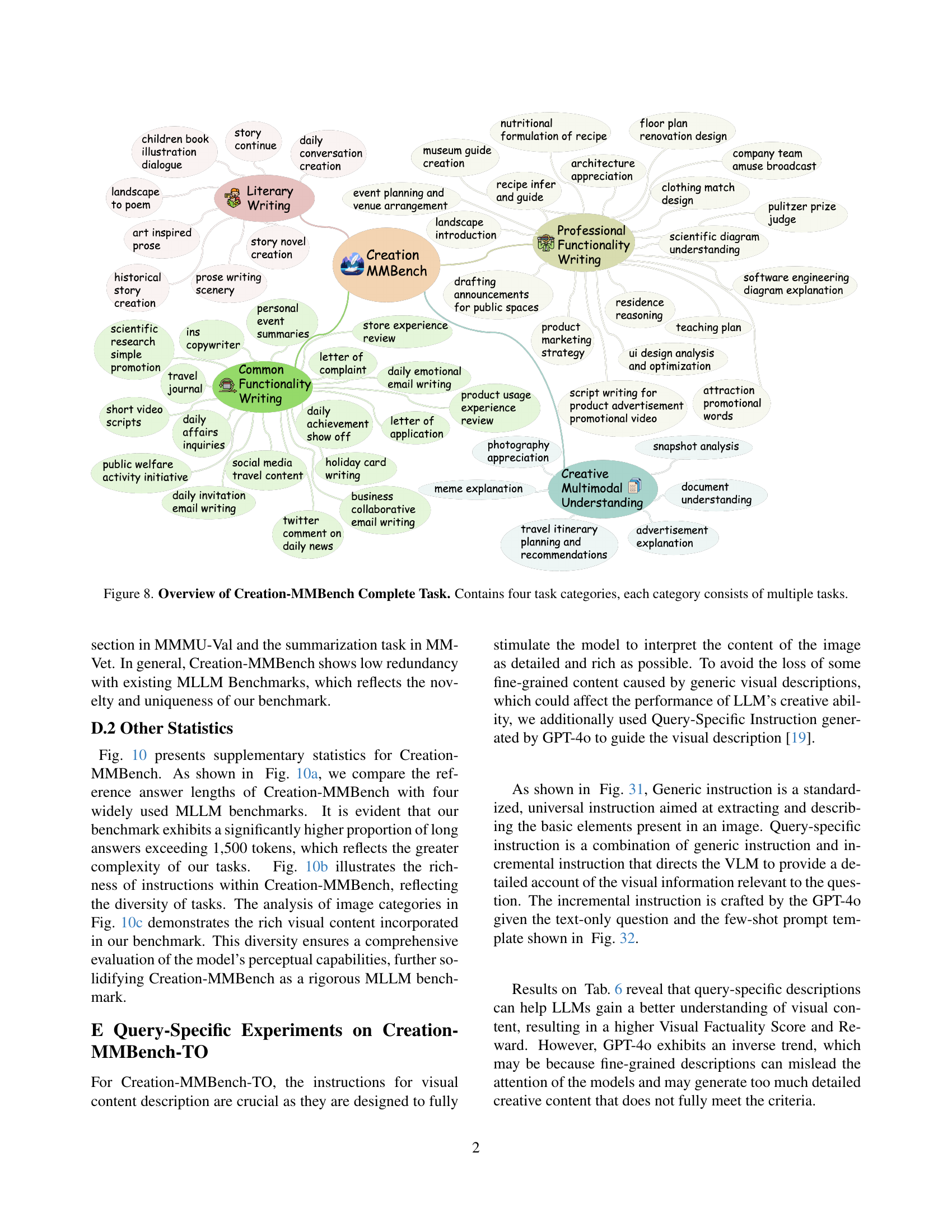
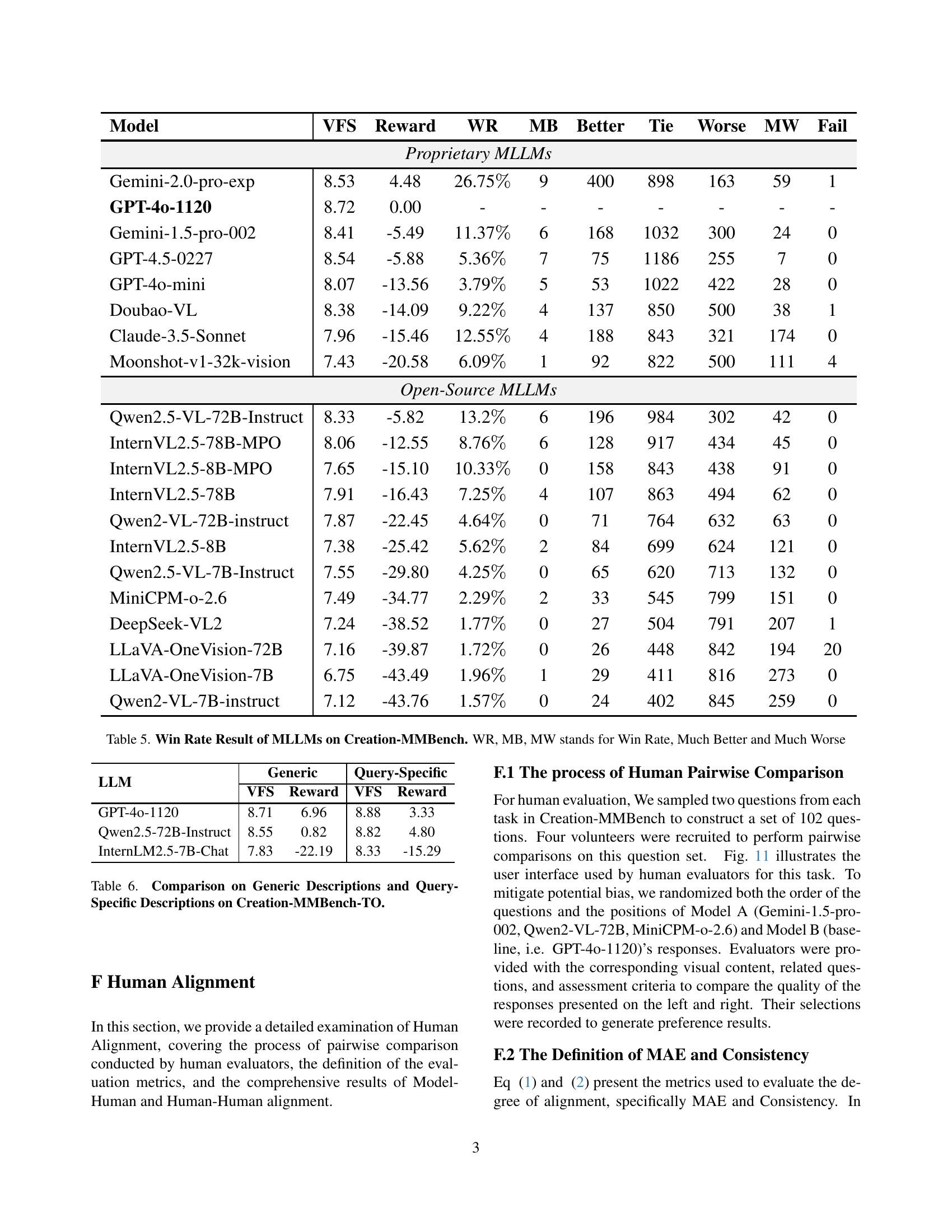
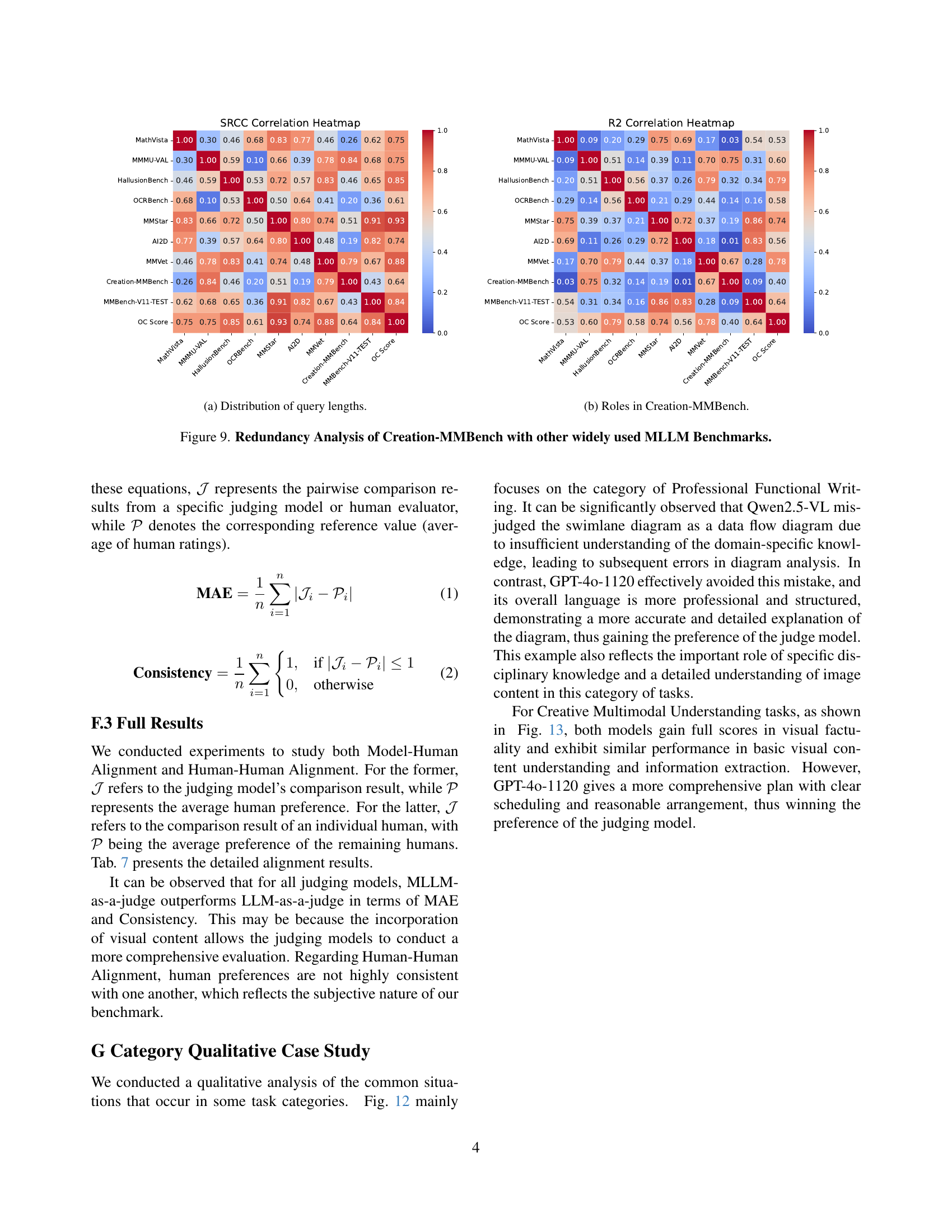
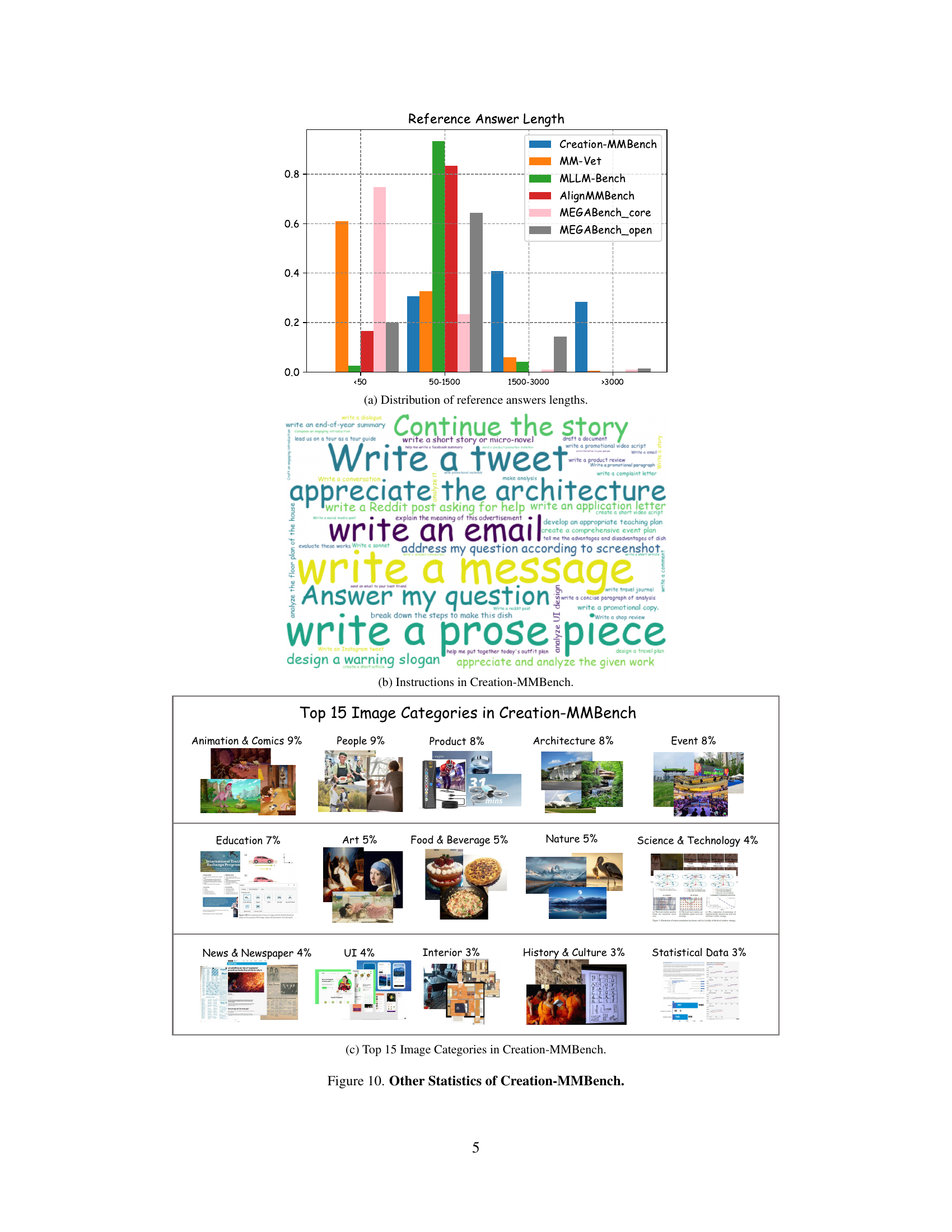
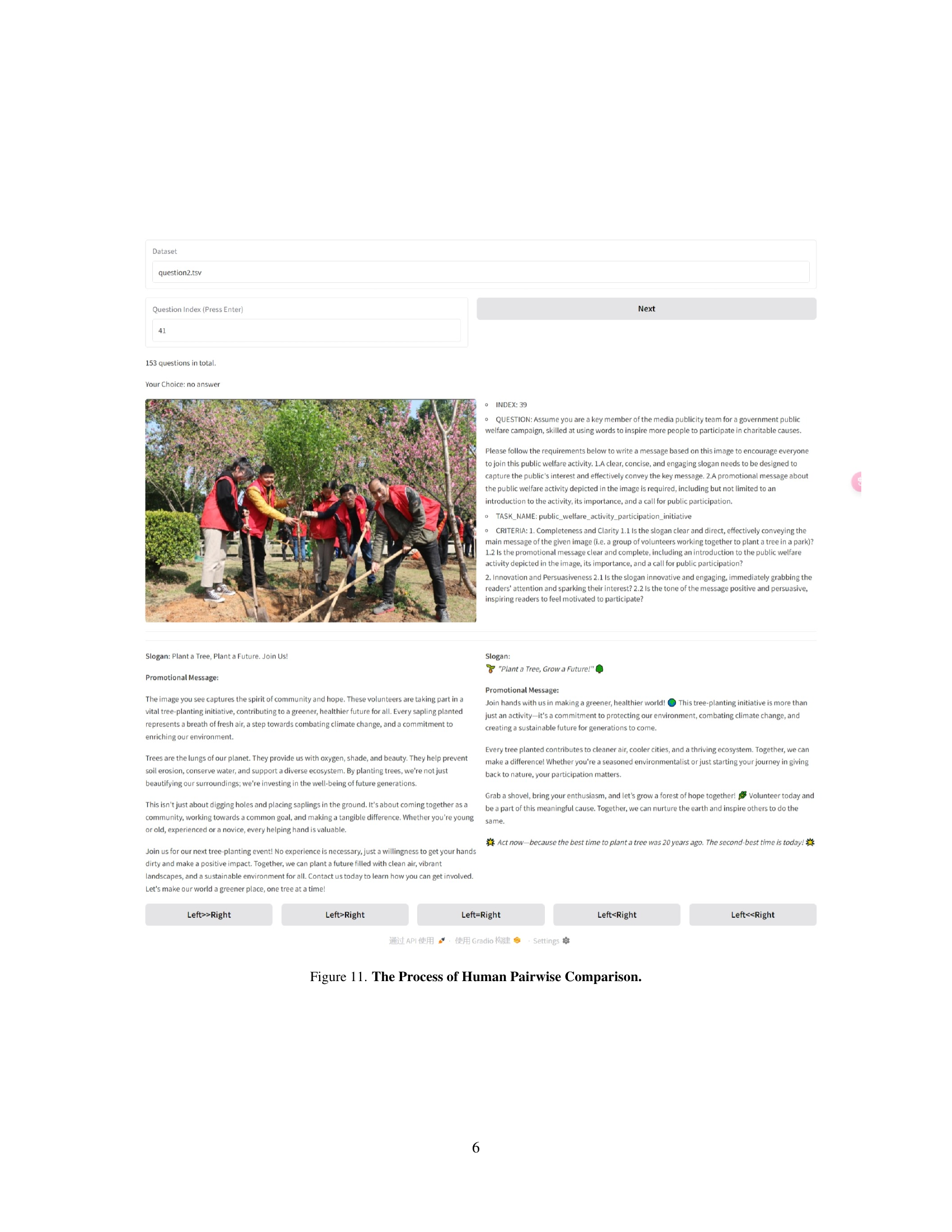
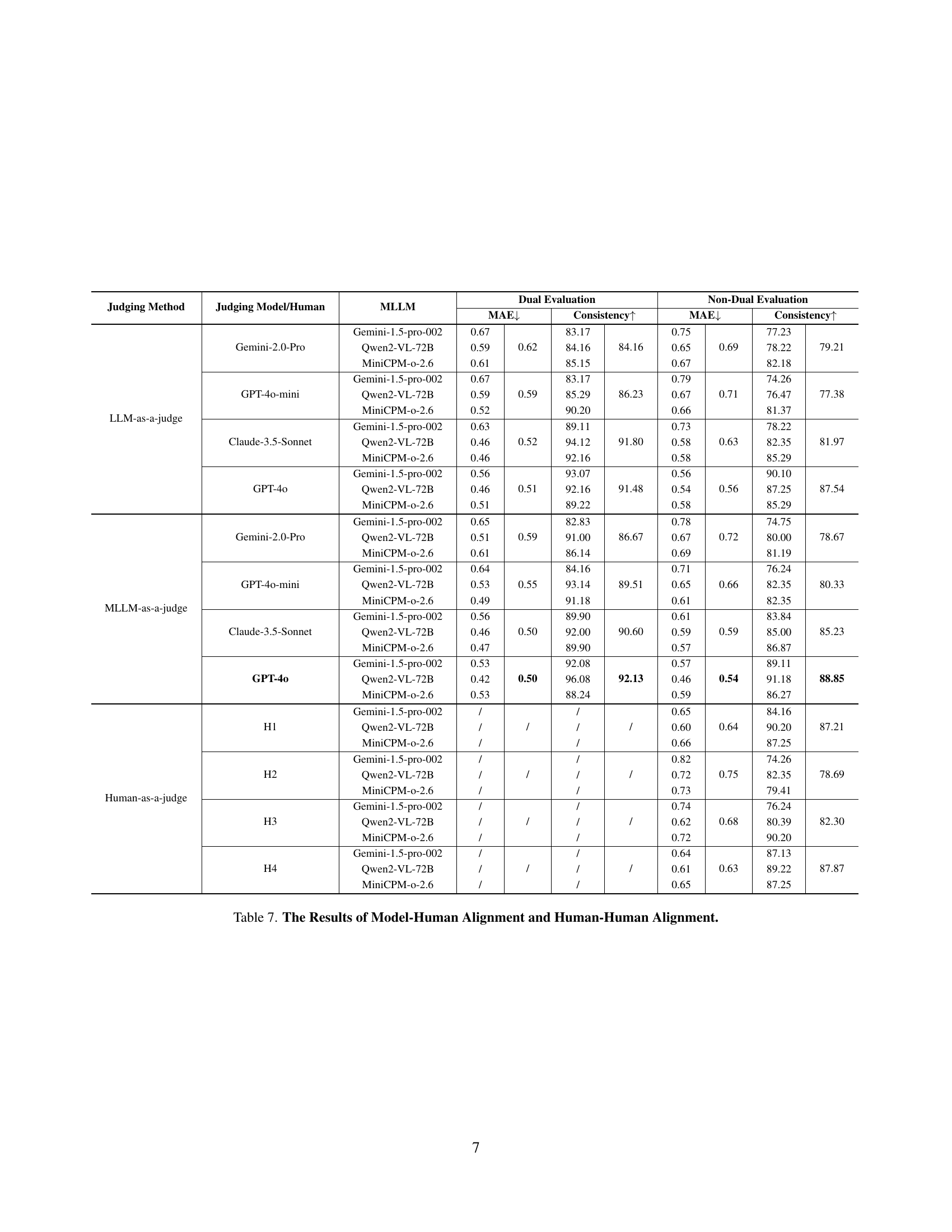
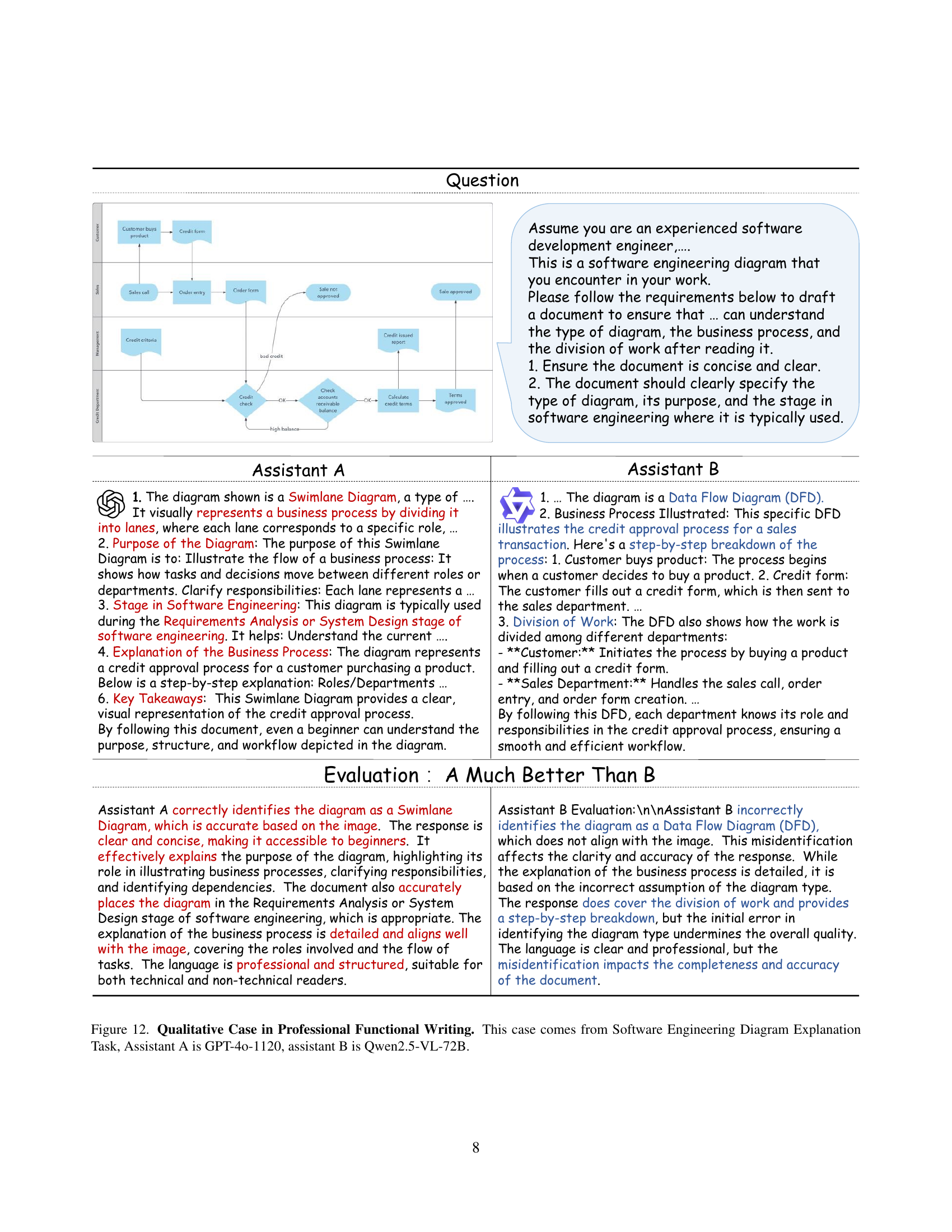
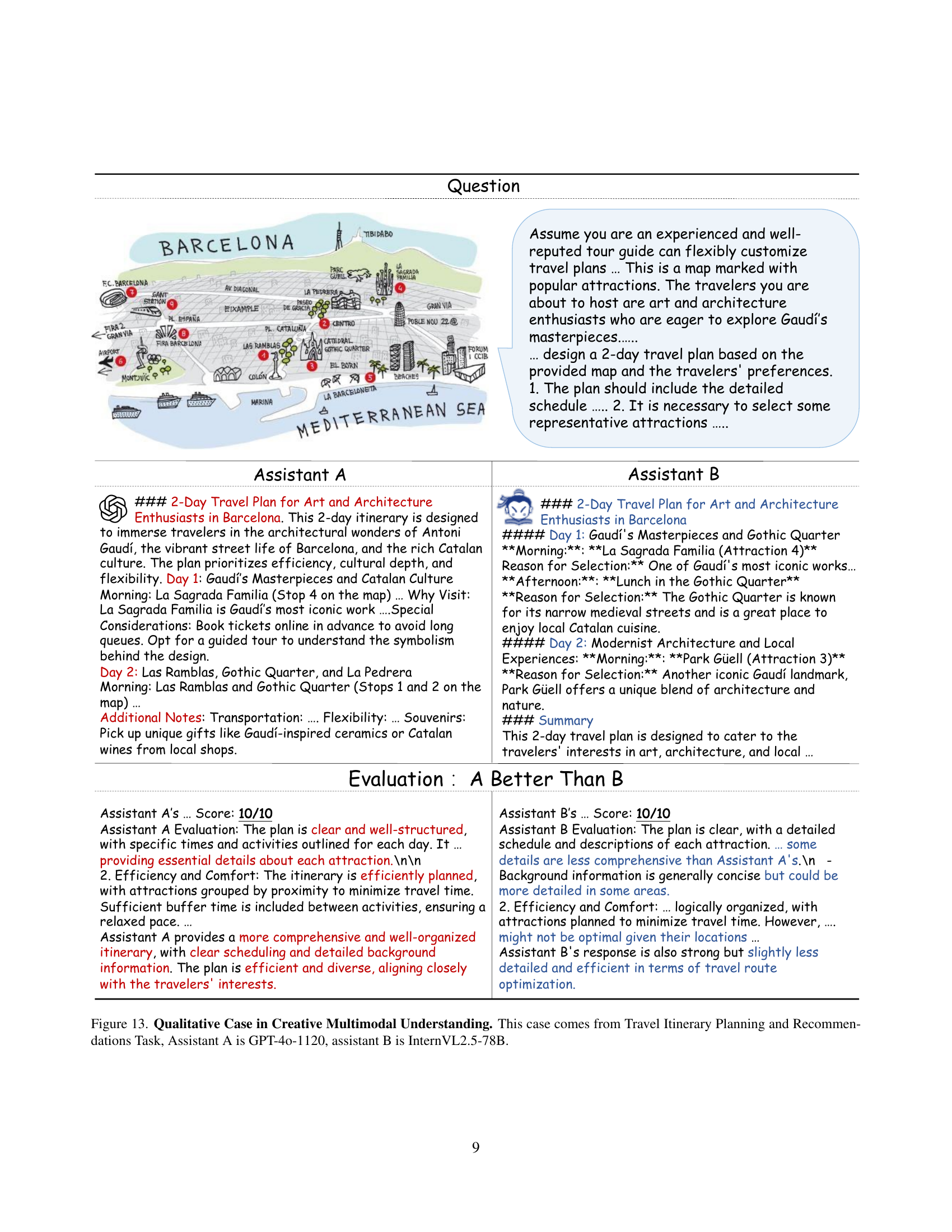
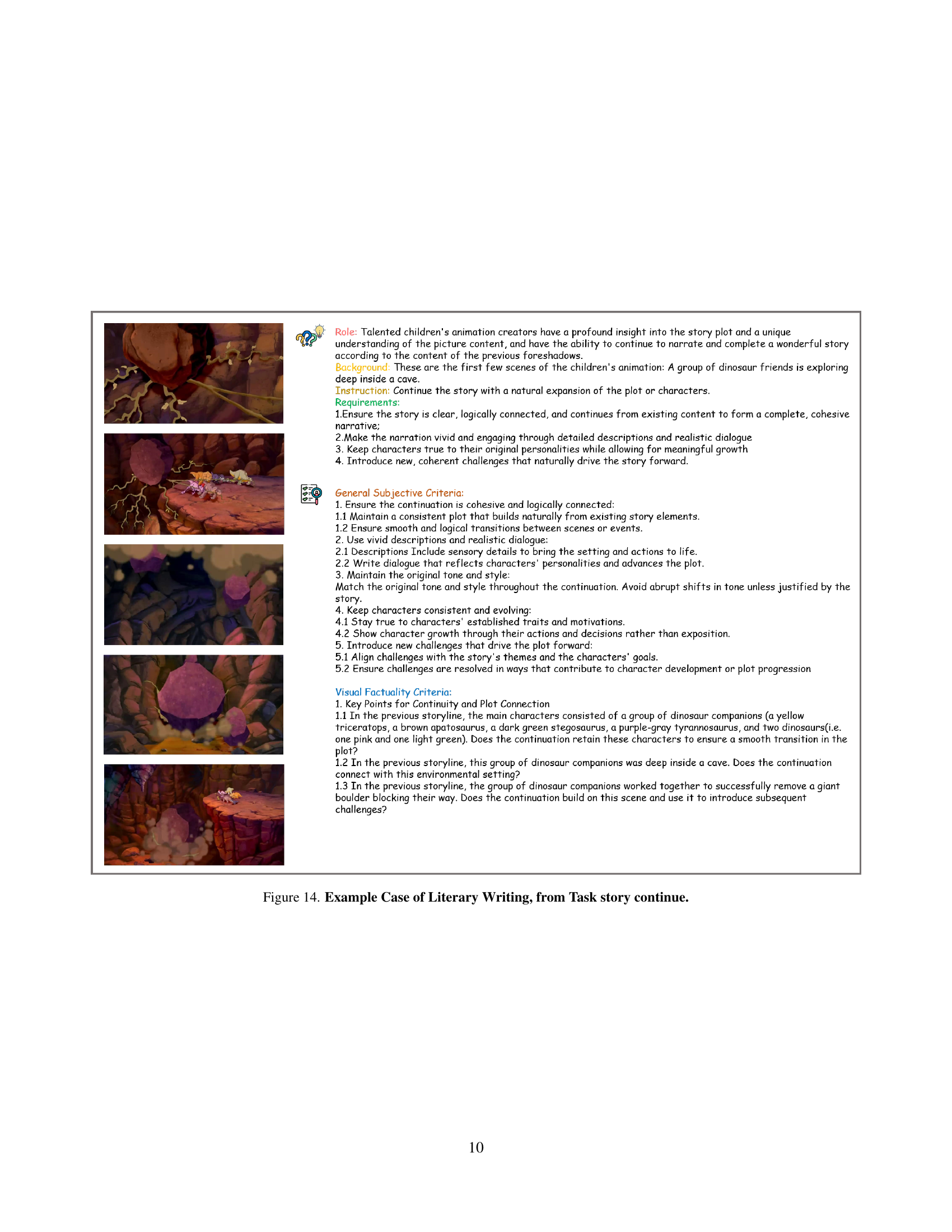