TL;DR#
Generating high-quality videos is challenging because ensuring consistent motion and realistic dynamics across frames is hard. Current methods struggle with temporal artifacts like flickering and repetitive motions. This is because they depend on simplified temporal patterns and lack explicit temporal augmentation during training, making them prone to overfitting. Unlike images, videos need models to understand dynamic transitions, but current spatial augmentation methods don’t address the temporal dimension. As a result, models show temporal inconsistency and rely on similar temporal patterns, limiting diversity.
To address these issues, this paper introduces FLUXFLOW, a data augmentation strategy that injects controlled temporal perturbations during video generation training. Inspired by how humans infer missing frames, FLUXFLOW disrupts fixed temporal orders to force the model to learn motion dynamics. It operates at two levels: shuffling individual frames and reordering contiguous frame blocks. Experiments show that FLUXFLOW significantly improves temporal coherence and diversity across various video generation models, while preserving spatial fidelity.
Key Takeaways#
Why does it matter?#
This paper introduces a novel and effective approach to enhance video generation quality, paving the way for more robust and temporally consistent video generation. The proposed method demonstrates significant improvements across various models and benchmarks, highlighting the potential of temporal augmentation as a simple yet viable solution.
Visual Insights#
πΌ This figure shows the effectiveness of FluxFlow in improving the temporal quality of generated videos. The top row displays a dog chasing a butterfly with the butterfly moving randomly, and the bottom row shows a person running along a beach with waves. In each case, the left-hand images represent results without FluxFlow, demonstrating artifacts like flickering textures or unnatural motion. The images on the right-hand side show results with FluxFlow applied, resulting in smoother, more realistic motion and improved temporal coherence.
read the caption
Figure 1: FluxFlow improves the temporal quality of video generators. Captions: (Top) A dog chasing a butterfly in a garden, with the butterfly flying in random directions. (Bottom) A person is running along a beach with waves crashing in the background.
Β Method | \adl@mkpreamc\@addtopreamble\@arstrut\@preamble | \adl@mkpreamc\@addtopreamble\@arstrut\@preamble | ||||||||||
---|---|---|---|---|---|---|---|---|---|---|---|---|
FVD | IS | Subject | Back. | Flicker | Motion | Dynamic | Aesthetic | Imaging | Quality | Semantic | Total | |
Β β \adl@mkpreamc\@addtopreamble\@arstrut\@preamble | ||||||||||||
VideoCrafter2Β [5] | 463.80 | 36.57 | 96.85 | 98.22 | 98.41 | 97.73 | 42.50 | 63.13 | 67.22 | 82.20 | 73.42 | 80.44 |
Original | ||||||||||||
Β β β Β β β Β | ||||||||||||
37.89β1.32 | 49.58β7.08 | 63.55β0.42 | 67.94β0.72 | |||||||||
451.43β12.37 | 99.15β0.93 | 98.66β0.93 | 83.31β1.11 | 73.39β0.03 | 81.33β0.89 | |||||||
97.93β1.08 | 98.69β0.28 | |||||||||||
Β β \adl@mkpreamc\@addtopreamble\@arstrut\@preamble | ||||||||||||
NOVAΒ [7] | 428.12 | 38.44 | 94.71 | 94.81 | 96.38 | 96.34 | 54.35 | 54.52 | 66.21 | 78.96 | 76.57 | 78.48 |
Original | 54.89β0.37 | |||||||||||
Β β β Β β β Β | ||||||||||||
420.17β7.95 | 96.18β1.47 | 95.56β0.75 | 96.87β0.49 | 97.40β1.06 | 58.64β4.29 | 66.86β0.65 | 80.52β1.56 | 76.11β0.46 | 79.64β1.16 | |||
96.24β1.43 | 97.45β1.07 | 97.21β0.87 | 57.88β3.53 | 80.91β1.95 | 76.84β0.27 | 80.10β1.62 | ||||||
39.24β0.89 | 94.57β0.24 | 97.12β0.74 | 97.52β1.18 | 56.54β2.20 | 79.97β1.01 | 75.28β1.29 | 79.03β0.55 | |||||
Β β \adl@mkpreamc\@addtopreamble\@arstrut\@preamble | ||||||||||||
CogVideoXΒ [44] | 347.59 | 44.32 | 96.78 | 96.63 | 98.89 | 97.73 | 59.86 | 60.82 | 61.68 | 82.18 | 75.83 | 80.91 |
Original | 45.91β1.59 | |||||||||||
Β β β Β β β Β | ||||||||||||
343.23β4.36 | 97.15β0.52 | 99.14β0.25 | 98.20β0.47 | 61.26β1.40 | 82.88β0.70 | 75.98β0.15 | 81.50β0.59 | |||||
62.02β0.34 | ||||||||||||
98.04β1.26 | ||||||||||||
Β |
πΌ Table 1 presents a comprehensive evaluation of the FluxFlow-Frame technique, a data augmentation method designed to enhance temporal quality in video generation. The table compares the performance of three different video generation models (VideoCrafter2, NOVA, and CogVideoX) trained with and without FluxFlow-Frame. For each model, multiple variations of FluxFlow-Frame are tested, each denoted by ‘+ Num Γ 1’ where ‘Num’ represents the number of frames shuffled. The table includes quantitative metrics from the UCF-101 and VBench benchmarks, evaluating various aspects of video quality, including temporal coherence (FVD, Subject, Background, Flicker, Motion, Dynamic), frame-wise quality (Aesthetic, Imaging), and overall quality (Total, Quality, Semantic). The best-performing results for each metric are shaded, while the second-best are underlined, offering a clear comparison of the effectiveness of different FluxFlow-Frame strategies across diverse video generation models.
read the caption
Table 1: Evaluation of FluxFlow-Frame. β+++ Originalβ refers to training without FluxFlow, while β+++ NumΓ1Num1\text{Num}\times 1Num Γ 1β indicates the use of different FluxFlow-Frame strategies. We shade the best results and underline the second-best results for each model.
In-depth insights#
Temporal Augment#
Temporal augmentation, as a concept, seeks to enrich video generation by introducing varied and realistic time-based perturbations. It moves beyond static image augmentations to address the unique challenges of maintaining coherence and diversity across video frames. By intelligently disrupting the fixed temporal order, models are forced to learn more robust motion dynamics, rather than overfitting to simple, repetitive patterns. This approach unlocks a model’s potential to generalize across diverse motion scenarios, handle varying speeds, and generate temporally plausible content even with imperfect or noisy prompts. It’s a key to generating more expressive and convincing videos. Furthermore, by employing temporal perturbations, these method can help the video generation model to learn better optical flow dynamics.
FluxFlow Details#
Based on the paper, FluxFlow seems to be focused on enhancing video generation by addressing temporal inconsistencies. It introduces novel data augmentation techniques to improve temporal coherence. The method centers around perturbing the order of frames during training, either at the frame level or by reordering blocks of frames. This is designed to force the model to learn more robust motion dynamics rather than memorizing fixed sequences. Frame-level shuffling disrupts the exact frame order, while block-level reordering simulates real-world motion changes while preserving some continuity. The idea is to make the model less brittle to specific frame-by-frame dependencies. The application of FluxFlow doesn’t require any architectural changes to the video generation model, as it operates at the data level, which makes it easily integrated into different models. This could include U-Nets, Transformers or other autoregressive models. It is thought to improve a model’s ability to generalize to diverse motions and dynamic scenes. It can achieve better temporal stability and visual quality in the generated videos.
Model Agnostic#
The concept of ‘Model Agnostic’ is vital in research, emphasizing the development of techniques or frameworks applicable across diverse models without significant alterations. It aims for versatility and broad applicability, reducing dependency on specific model architectures. A model-agnostic approach promotes fair comparison by evaluating methods uniformly across different models, highlighting genuine improvements rather than model-specific quirks. This approach typically involves data-level manipulations or loss function modifications, avoiding direct interference with model architecture. Challenges include maintaining effectiveness across diverse model types and balancing simplicity and generalizability. It fosters broader adoption, greater robustness, and increased accessibility within the research community.
Ablation Studies#
Ablation studies are essential for understanding the impact of individual components within a larger system. In the context of video generation, ablations can reveal the contribution of specific architectural choices, loss functions, or training strategies. These studies typically involve systematically removing or modifying elements, then observing the resulting changes in performance metrics, such as FVD, IS or user-rated qualities. For instance, one might ablate a specific layer in a neural network or a particular term in a loss function to assess its role. By analyzing the impact of each ablation, researchers can identify the most critical factors driving performance and gain insights into the underlying mechanisms. A well-designed ablation study helps ensure that the complexity introduced by new components is justified by demonstrable improvements. Furthermore, it can guide future research efforts by highlighting areas where further optimization is most likely to yield significant gains.
Quality Metrics#
Evaluating the quality of video generation is multifaceted, requiring metrics that capture both spatial fidelity and temporal coherence. The paper employs FrΓ©chet Video Distance (FVD), which assesses the realism and coherence of motion over time, and Inception Score (IS), focusing on the frame-level quality and diversity. It uses VBench, a benchmark designed for video generation quality across dimensions such as Subject/Background Consistency, Temporal Flickering/Motion Smoothness/Dynamic Degree, and Aesthetic/Imaging/Semantic Quality, as well as Overall Score. These metrics provide a comprehensive view, enabling insights into how well models maintain object identity, ensure smooth transitions, and generate visually pleasing and semantically relevant content. Addressing the limitations of relying solely on automated metrics, a user study is conducted, involving human evaluation on aspects like perceived motion realism and temporal coherence.
More visual insights#
More on figures
πΌ This figure compares the performance of the VideoCrafter2 video generation model with and without FluxFlow, using the VBench benchmark. The top part shows that FluxFlow significantly improves the temporal quality of generated videos, as measured by VBench’s Temporal Quality metrics. The bottom part shows that FluxFlow either maintains or even slightly improves the overall and frame-wise quality of the videos, indicating that the improvements in temporal quality do not come at the cost of decreased overall or frame-level quality. This demonstrates FluxFlow’s effectiveness in enhancing video generation quality.
read the caption
Figure 2: Comparison of VideoCrafter2 with FluxFlow using VBench metrics for Temporal Quality (Top) and Frame-wise and Overall Quality (Bottom). FluxFlow significantly enhances the temporal quality of generated videos while maintaining or even improving frame-wise and overall quality.
πΌ This figure illustrates the FluxFlow method for improving temporal quality in video generation. Panel (a) shows standard training with fixed frame order, highlighting the limitation in learning temporal dynamics. Panel (b) demonstrates FluxFlow’s plug-and-play augmentation strategy that introduces controlled temporal perturbations during training. Panel (c) details two levels of FluxFlow: frame-level (top), where a specified number of frames are shuffled, and block-level (bottom), where blocks of consecutive frames are rearranged. This controlled disruption forces the model to learn more robust and diverse temporal relationships.
read the caption
Figure 3: Overview of FluxFlow. (a) Standard video generation trains on fixed frame orders, which may limit the modelβs ability to learn temporal dynamics. (b) FluxFlow introduces controlled temporal perturbations during training as a plug-and-play augmentation strategy. (c) This study explores FluxFlow at two levels: frame-level (top) and block-level (bottom). In frame-level, NumΓ1Num1\text{Num}\times 1Num Γ 1 denotes the number of individual frames shuffled. In block-level, Num1ΓNum2Num1Num2\text{Num1}\times\text{Num2}Num1 Γ Num2 represents a block comprising Num2 consecutive frames.
πΌ Figure 4 illustrates how FluxFlow improves temporal coherence in video generation. The top part shows example frames from the CogVideoX model, both without and with FluxFlow applied. The difference highlights that FluxFlow enables the generation of videos with more dynamic and larger motion. The bottom part presents a quantitative analysis of this improvement by comparing the angular differences between consecutive frames. The consistently smaller angular differences produced with FluxFlow demonstrate significantly enhanced temporal coherence when compared to the base model. The example scenario depicts a skateboarder performing dynamic tricks in a skatepark, showcasing fast-paced movements and constantly changing camera angles.
read the caption
Figure 4: Illustration of FluxFlow in enhancing temporal coherence. (Top) Example frames from CogVideoX, without and with FluxFlow, showcasing larger motion dynamics in the latter. (Bottom) Comparison of temporal angle differences across frames. FluxFlow achieves consistently lower angle differences, indicating improved temporal coherence over the base model. Caption: A skateboarder performing tricks in a skatepark, with fast-paced movements and dynamic camera angles.
πΌ Figure 5 demonstrates the impact of FluxFlow on enhancing the diversity of temporal features learned by video generation models. Panel (a) shows that without FluxFlow, the model struggles to differentiate between videos with distinct temporal characteristics (static, slow, and fast motion). The model’s learned features from these different types of videos overlap significantly, indicating a lack of ability to discriminate diverse temporal dynamics. Panel (b) presents the results after applying FluxFlow. Here, the model’s temporal feature representation is dramatically improved, with clearly separated clusters corresponding to the different types of videos. This separation of features indicates the model now successfully distinguishes between and represents videos with varying temporal dynamics, highlighting FluxFlow’s effectiveness in improving temporal feature diversity.
read the caption
Figure 5: Illustration of FluxFlow in improving temporal feature diversity. (a) Without FluxFlow, the model trained on fixed original frame sequences fails to distinguish features across different temporal paradigms. (b) With FluxFlow, features are more distinctly separated, reflecting enhanced temporal representation.
πΌ Figure 6 presents a qualitative comparison of video generation results obtained using three different video generation models: VideoCrafter2, NOVA, and CogVideoX. Each model was tested both with and without the application of FluxFlow, a novel temporal augmentation technique. The figure showcases example video frames generated by each model under different conditions, illustrating the improvements in temporal coherence and quality achieved by incorporating FluxFlow. Specifically, the figure highlights that FluxFlow enhances the smoothness of motion, reduces temporal inconsistencies (such as flickering or abrupt transitions), and promotes more realistic and diverse motion patterns compared to videos generated without it. The top row shows results for VideoCrafter2, the middle row shows results for NOVA, and the bottom row shows results for CogVideoX.
read the caption
Figure 6: Qualitative results of FluxFlow on VideoCrafter2 [5] (Top), NOVA [7] (Middle), and CogVideoX [44] (Bottom).
πΌ Figure 7 presents an ablation and sensitivity analysis of the FLUXFLOW method using VBench temporal metrics. Panels (a) and (b) show how varying the shuffle interval constraintsβthe minimum distance between shuffled frames or blocksβaffects performance on the VideoCrafter2 (VC2) model, specifically using 2x1 and 2x2 configurations. Panels (c) and (d) demonstrate the impact of changing the perturbation degrees (the proportion of frames shuffled) on both the 16-frame VC2 and the 33-frame NOVA models, illustrating how sensitivity to this hyperparameter changes depending on model and sequence length.
read the caption
Figure 7: Ablation and sensitivity analysis on FluxFlow with VBench temporal metrics. (a, b) Impact of shuffle interval constraints on VC2 using 2Γ1212\times 12 Γ 1 and 2Γ2222\times 22 Γ 2 configurations. (c, d) Impact of perturbation degrees on 16-frame VC2 and 33-frame NOVA.
πΌ Figure 8 presents a user study comparing the performance of CogVideoX with and without FluxFlow. The top section shows example frames from a video sequence featuring a non-linear motion pattern (a fish swimming in circles), highlighting FluxFlow’s improved handling of complex trajectories compared to the baseline model. The bottom section displays the results of a user rating system, assessing five aspects of temporal dynamics: Motion Diversity, Motion Realism, Motion Smoothness, Temporal Coherence, and Optical Flow Consistency. More detailed information on the user study methodology can be found in Appendix Β§A.
read the caption
Figure 8: User study results comparing CVX and w/ FluxFLow. (Top) Examples frames from a non-linear motion pattern, where FluxFlow demonstrates superior handling of complex trajectories. Caption: A fish swims in circular loops in a clear blue pond. (Bottom) User ratings across temporal dynamics evaluation criteria. For more details please refer to AppendixΒ Β§A.
πΌ Figure 9 demonstrates the impact of FluxFlow on the temporal quality of videos when generating longer sequences than those used during training. The top part displays example frames of a 128-frame video generated from a 16-frame base video using VideoCrafter2 (VC2). The left sequence, without FluxFlow, shows inconsistencies in motion and background; the right sequence, with FluxFlow, maintains smooth motion and consistent background details. The bottom panel uses VBench metrics to quantify the impact. The grey shaded bars represent the decrease in performance compared to 16-frame generation, highlighting how FluxFlow mitigates the negative effects of generating much longer videos.
read the caption
Figure 9: Performance comparison under extra-term conditions. (Top) Example frames from 16-frame VC2 generating 128-frame, without and with FluxFlow, showcasing dynamic background consistency in the latter. Caption: A dog running along a beach, splashing water as it moves through the waves. (Bottom) Comparison of temporal quality metrics on VBench, where the gray regions indicate the performance drop under extra-term scenarios.
πΌ This figure presents example videos from a user study designed to evaluate the impact of FLUXFLOW on video generation quality. Each video is accompanied by its optical flow visualization to help assess the optical flow consistency, a key aspect of temporal quality. The example shown depicts a skier smoothly carving curves down a snowy slope, illustrating how FLUXFLOW improves the smoothness and coherence of motion in generated videos.
read the caption
Figure 10: User study examples. Each video is provided with its optical flow to assess the Optical Flow Consistency. Caption: A skier carves smooth curves as they descend a snowy slope.
πΌ Figure 11 presents further qualitative comparisons showcasing the effectiveness of FluxFlow across three different video generation models: VideoCrafter2, NOVA, and CogVideoX. For each model, it shows example video sequences generated both with and without FluxFlow. The figure aims to visually demonstrate the improved temporal quality achieved by FluxFlow, including smoother and more realistic motion in the FluxFlow-enhanced videos, compared to artifacts and inconsistencies in the base model videos.
read the caption
Figure 11: More comparison of FluxFlow on VideoCrafter2Β [5], NOVAΒ [7], and CogVideoXΒ [44].
Full paper#
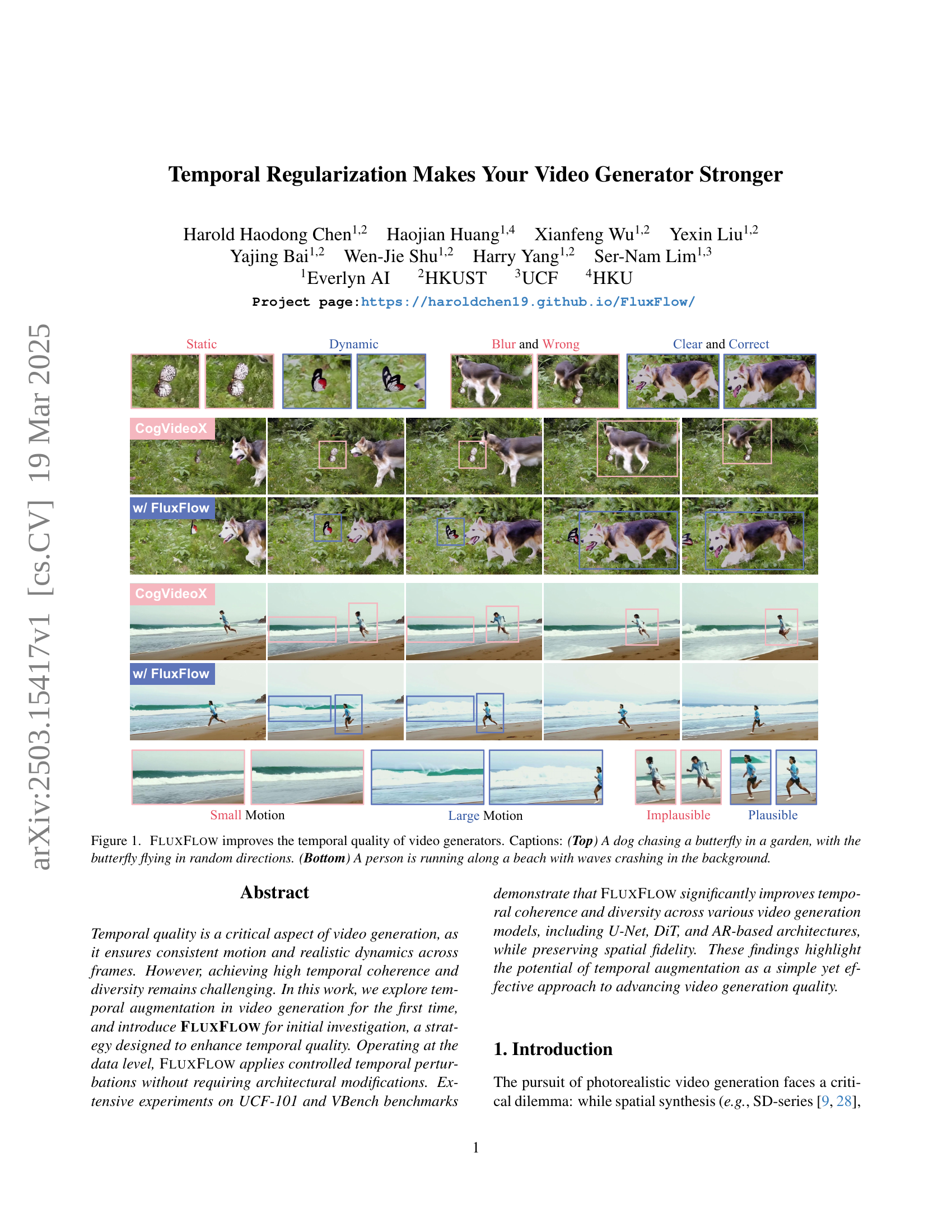
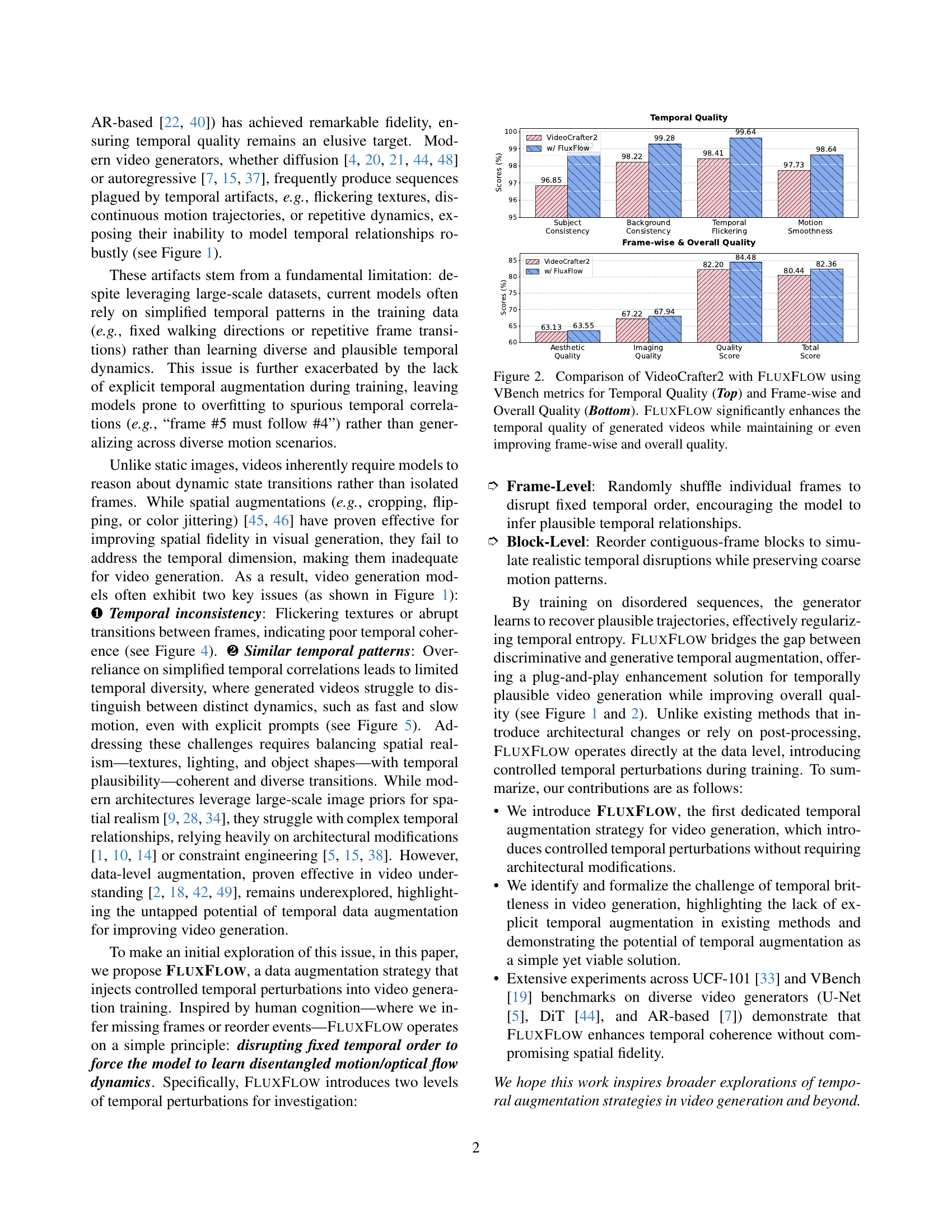
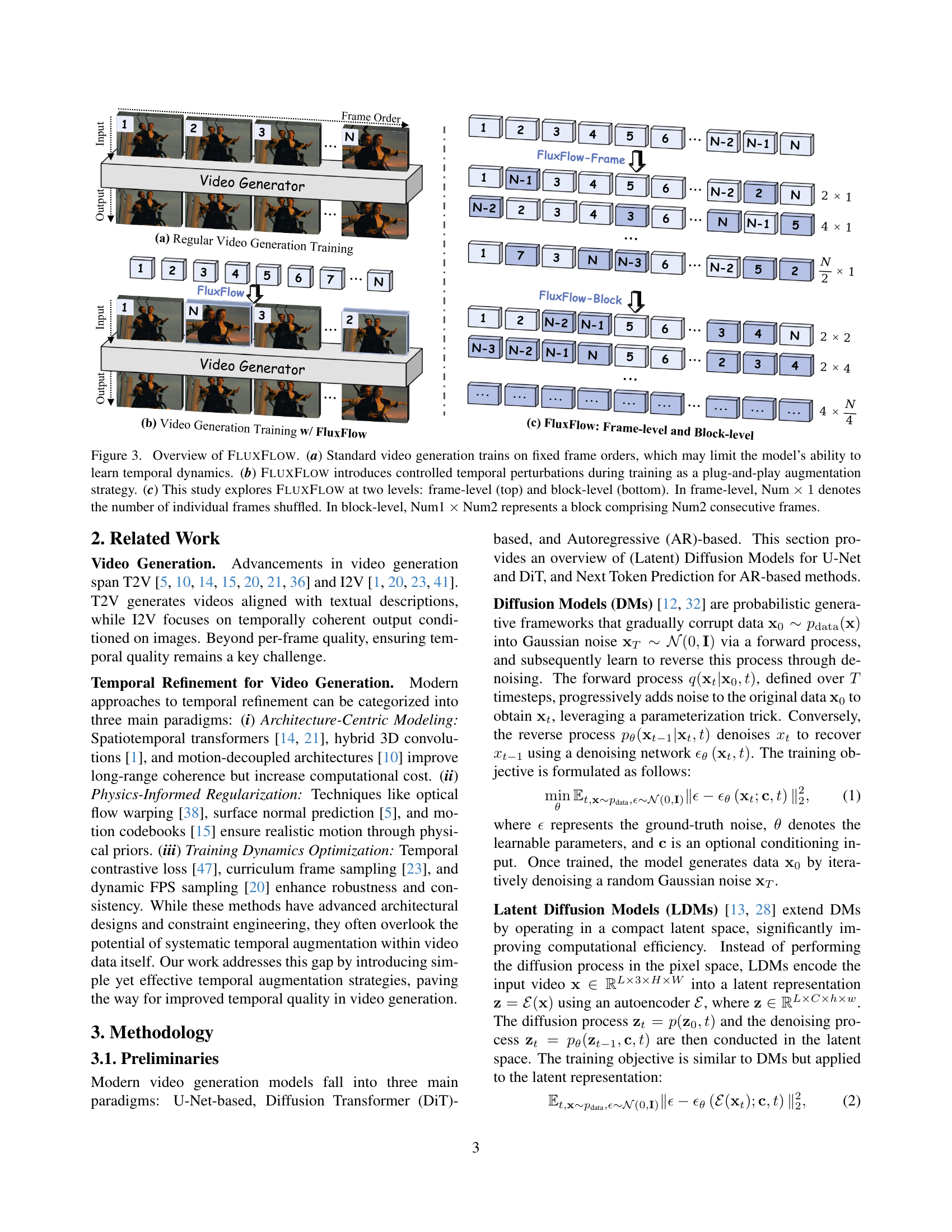
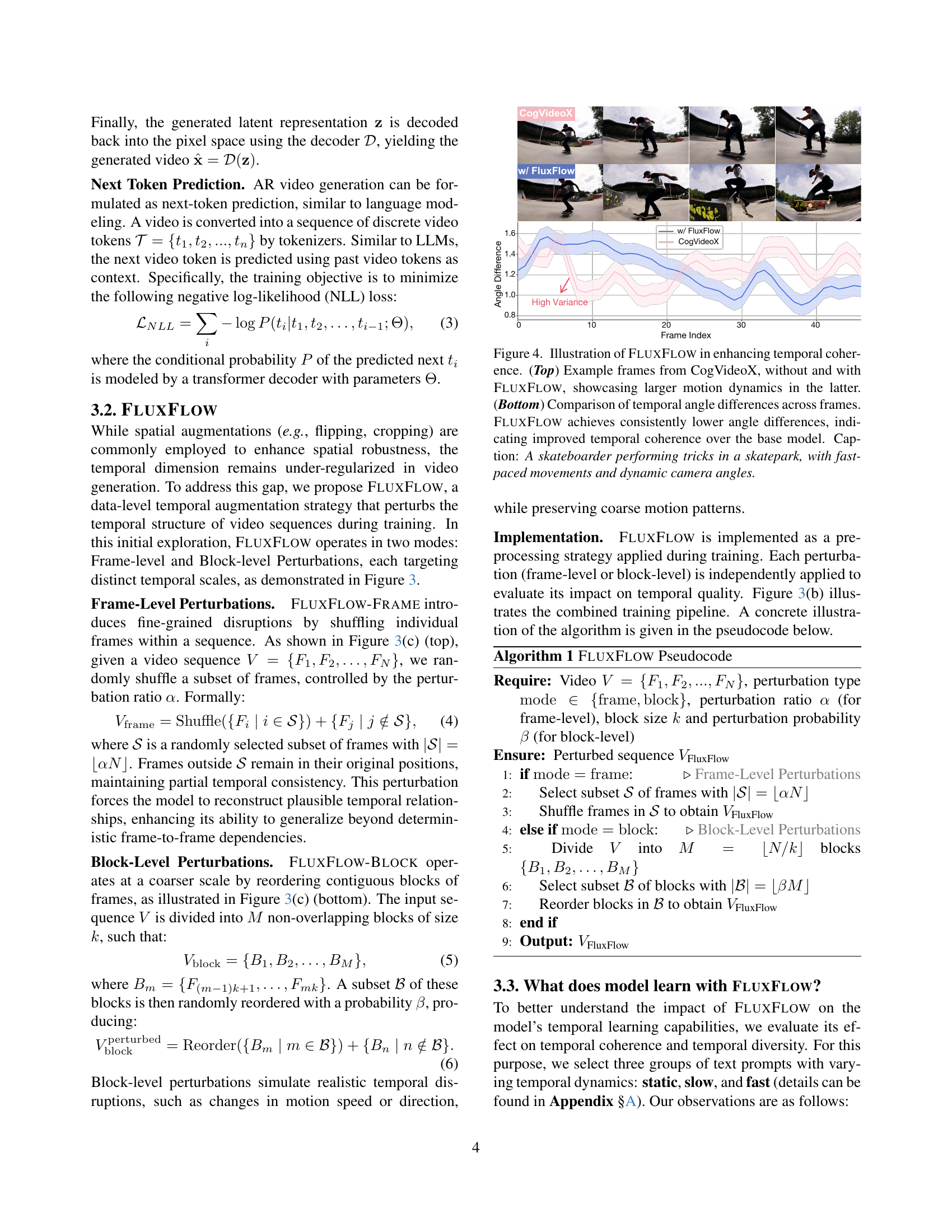
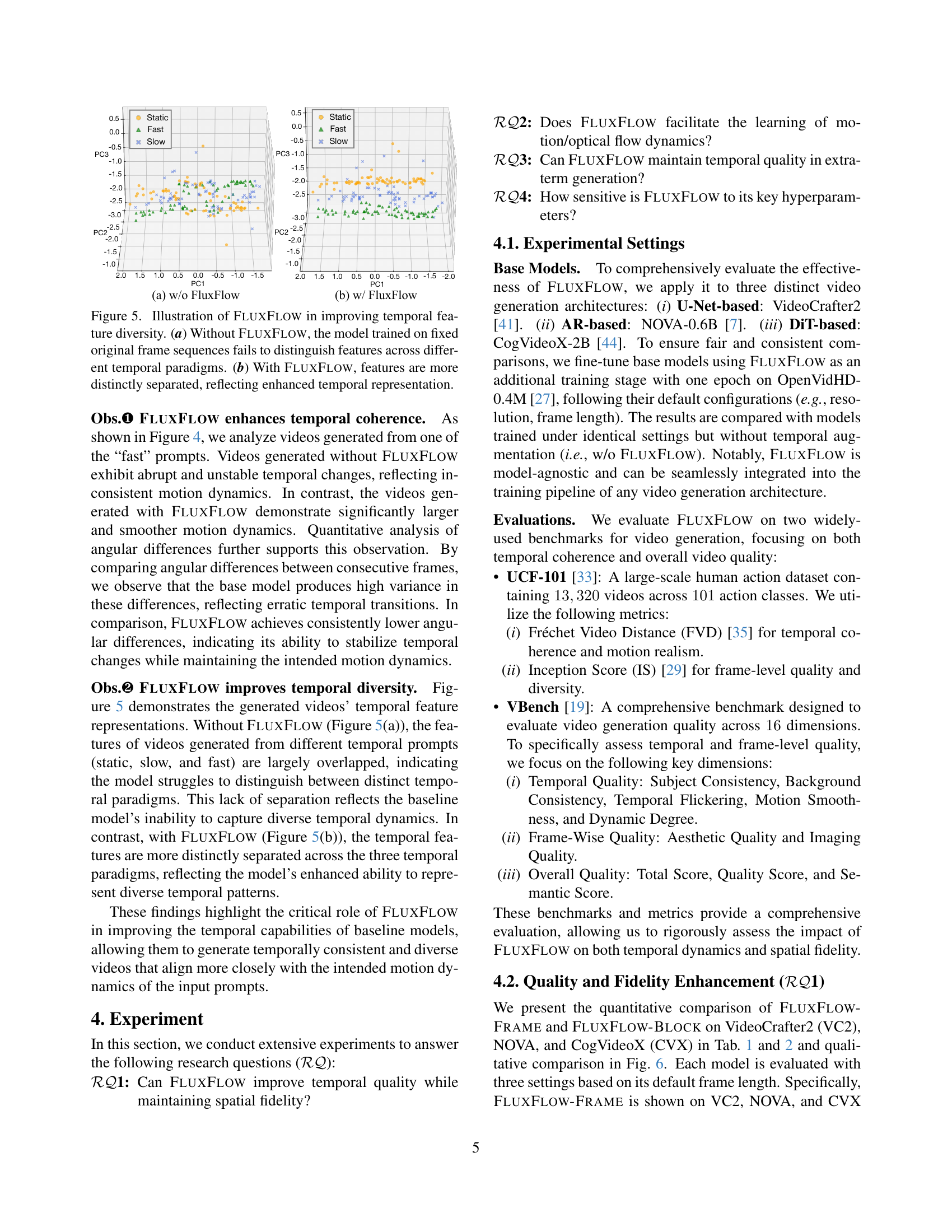
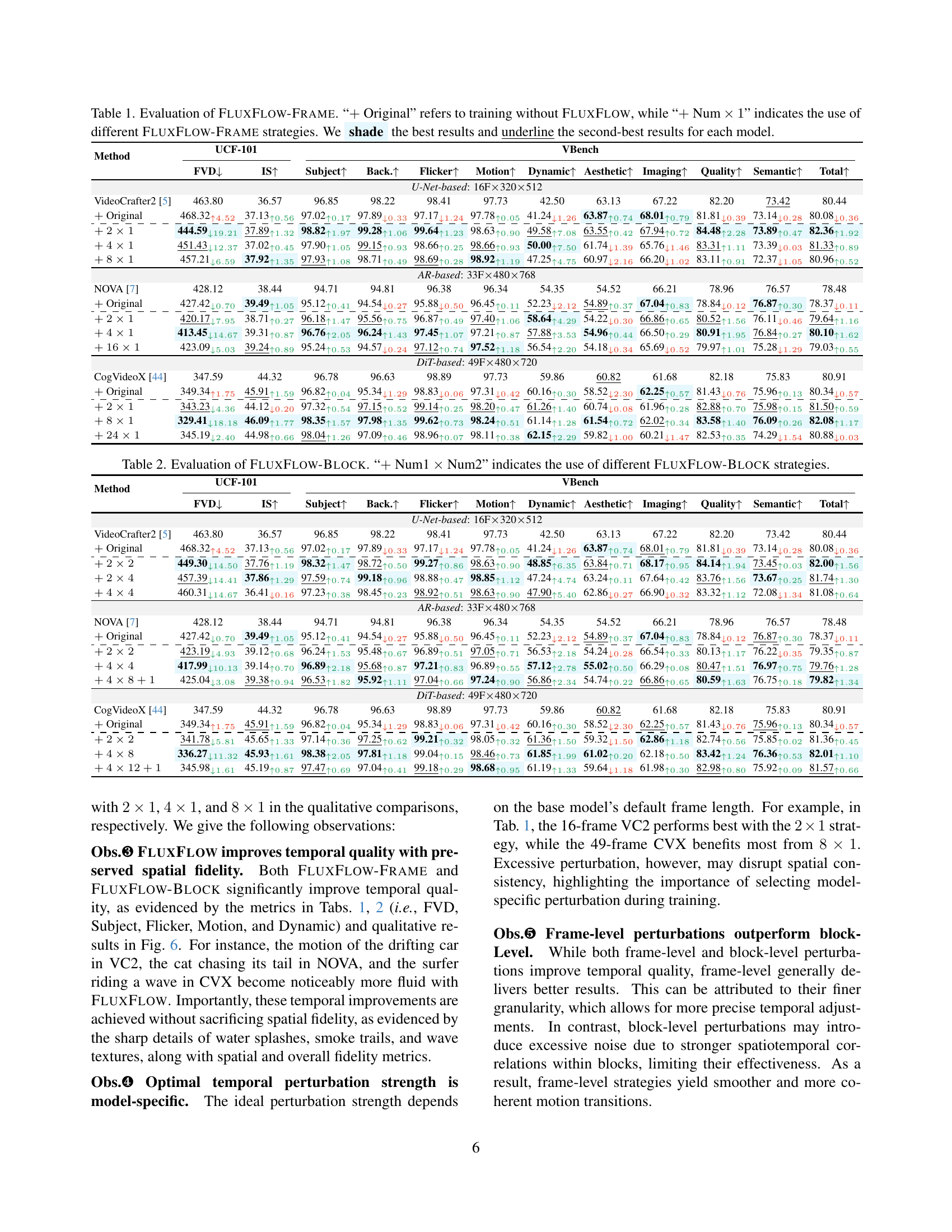
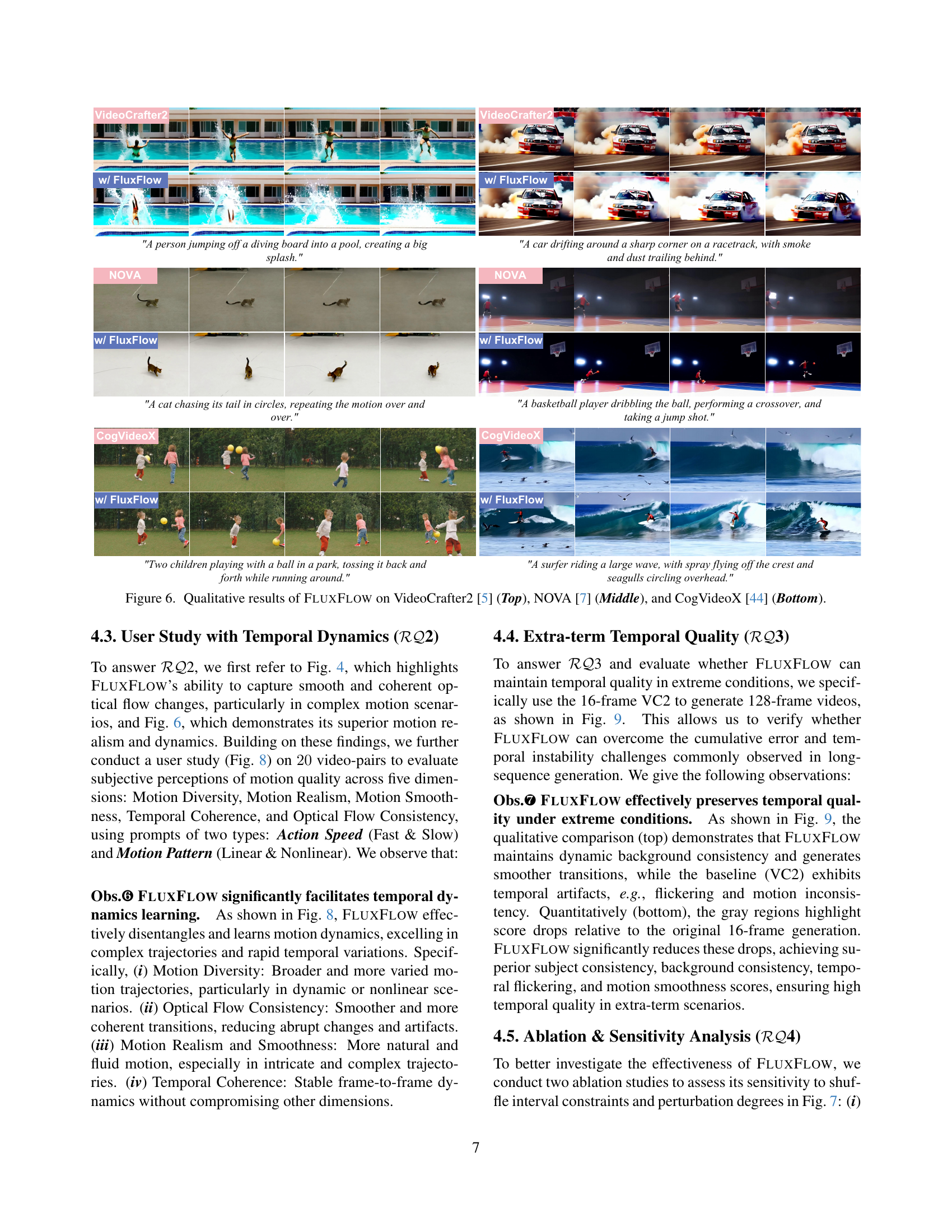
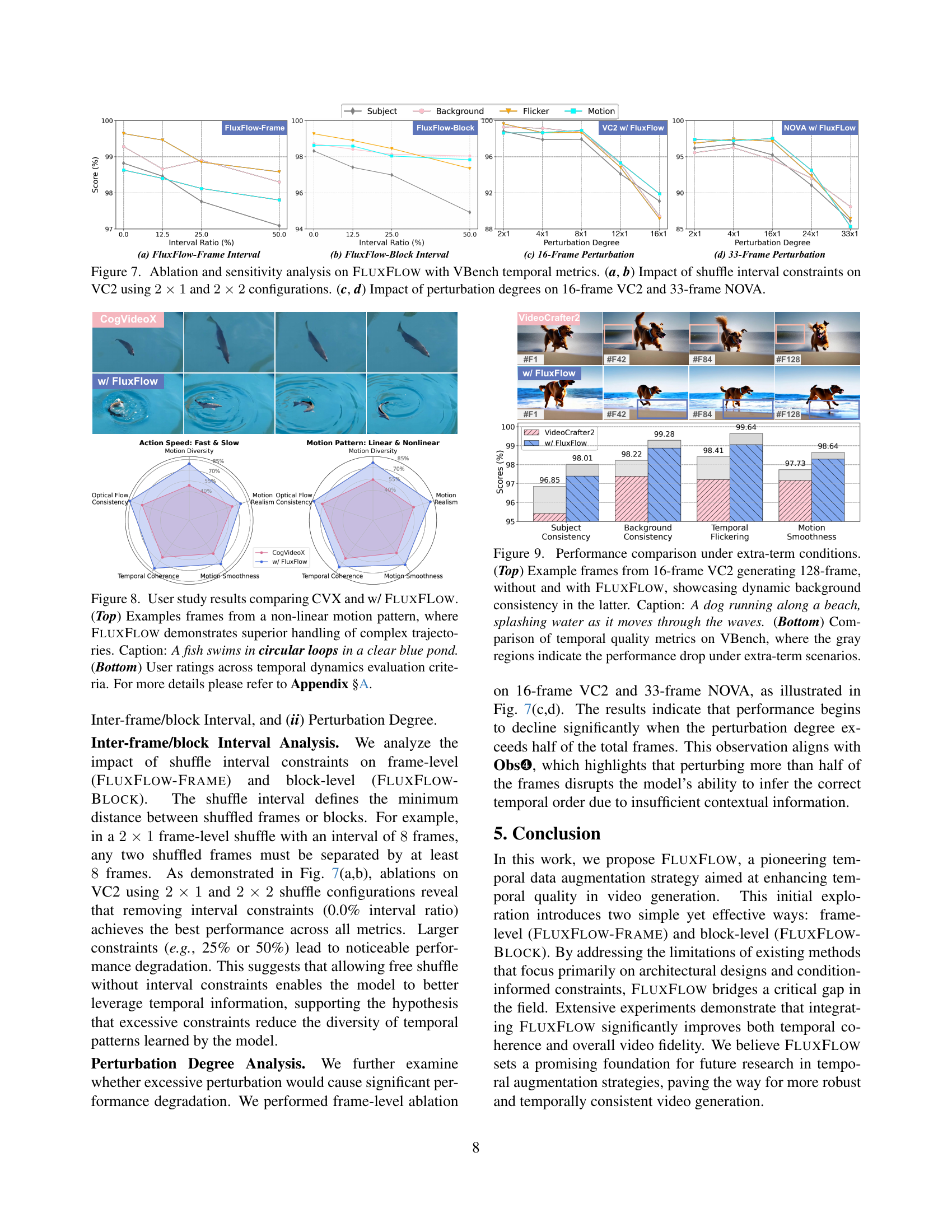
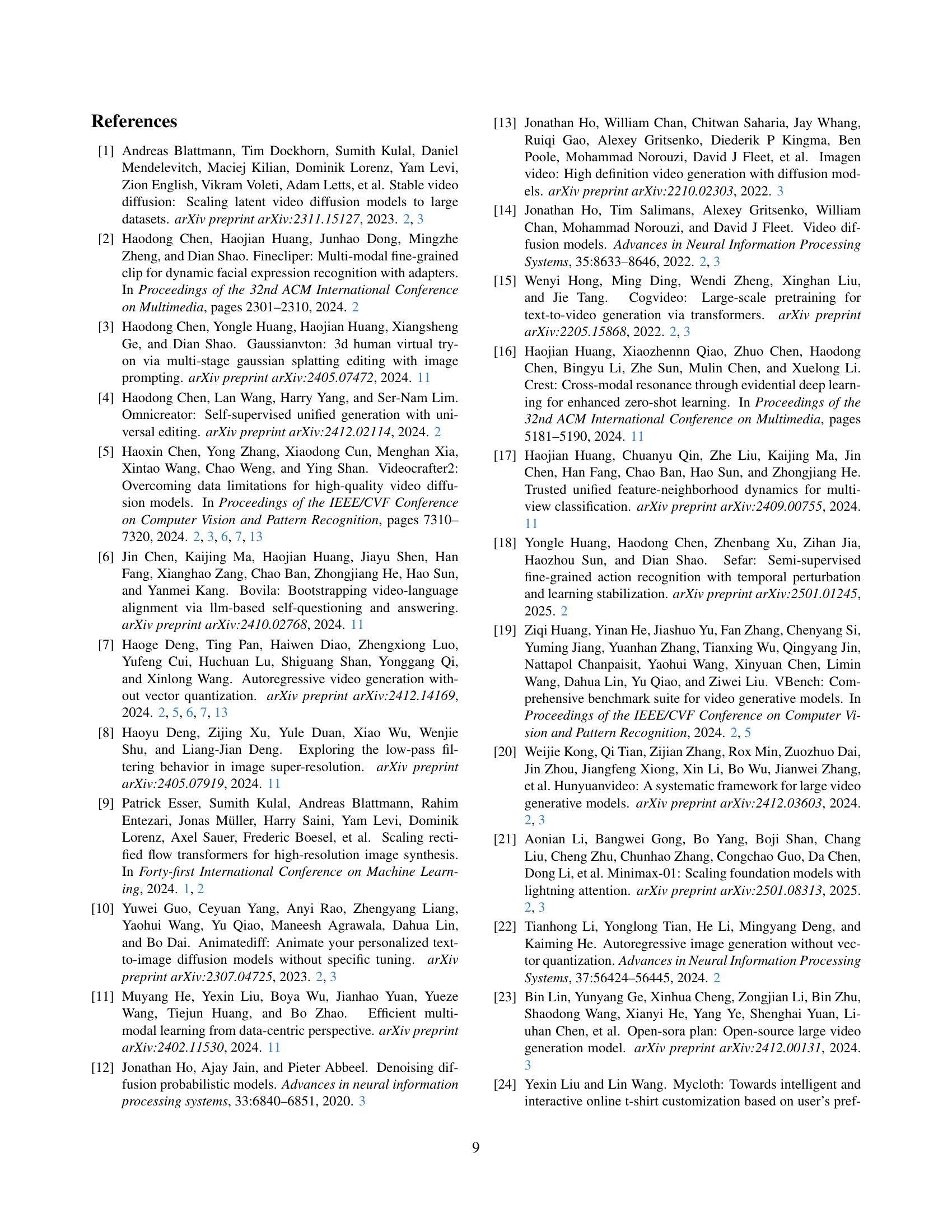
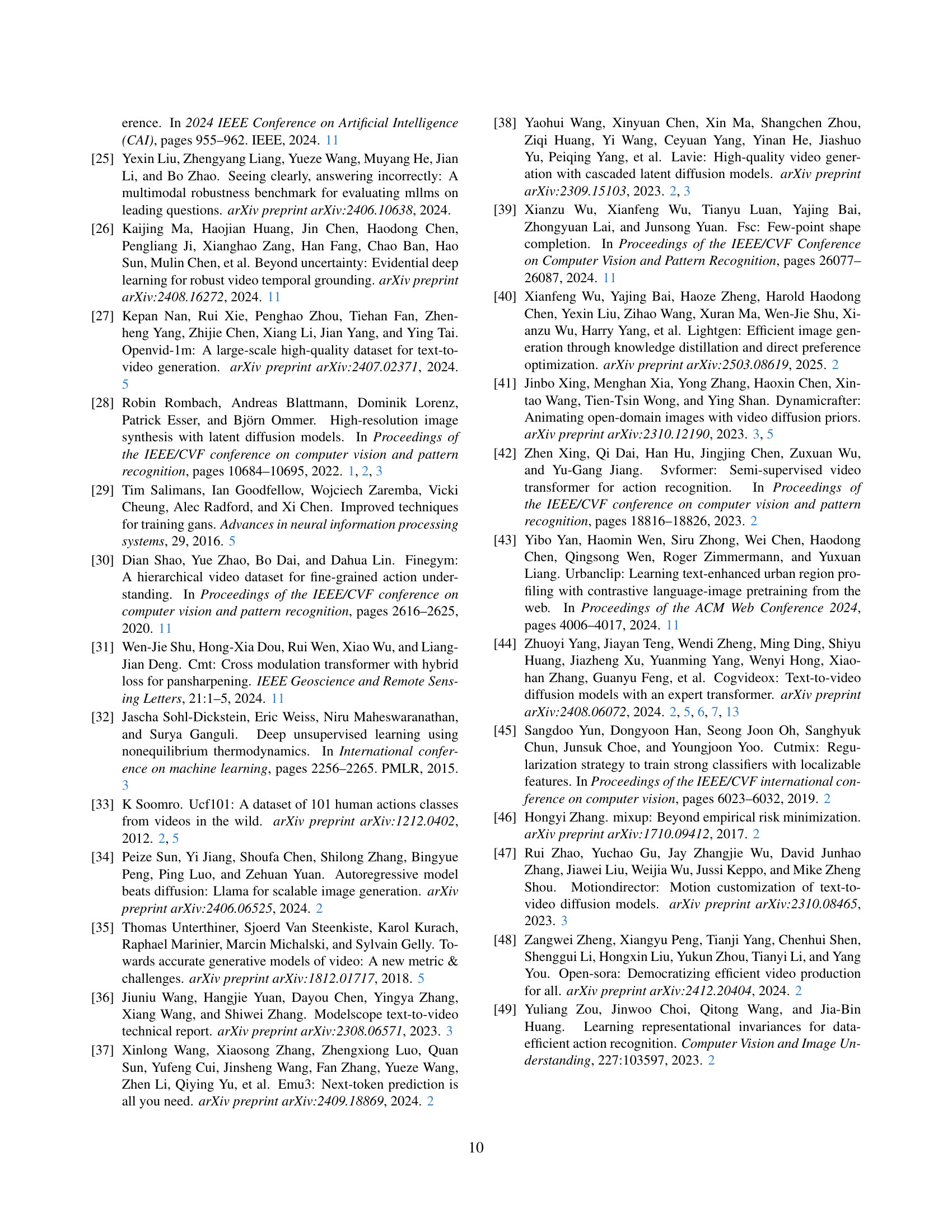
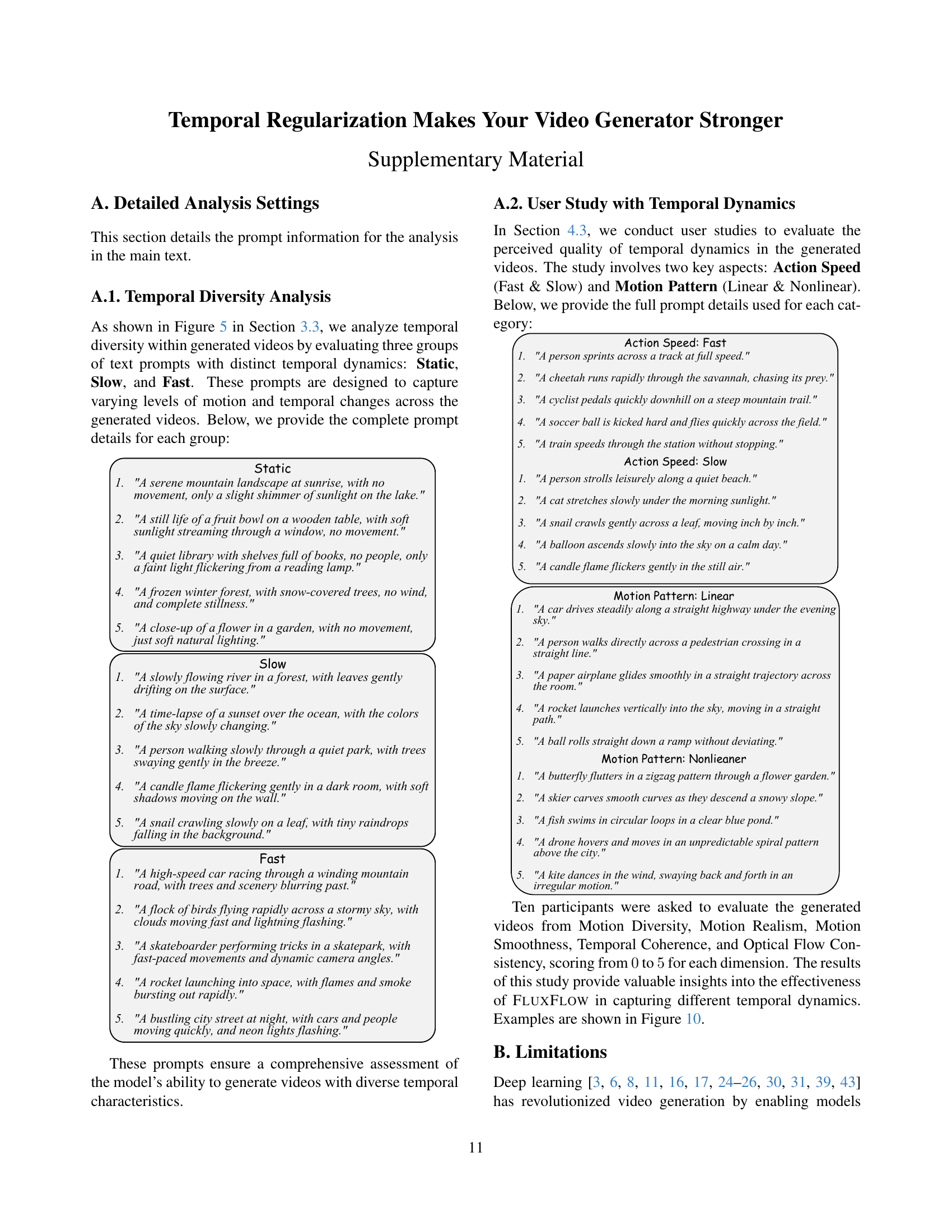
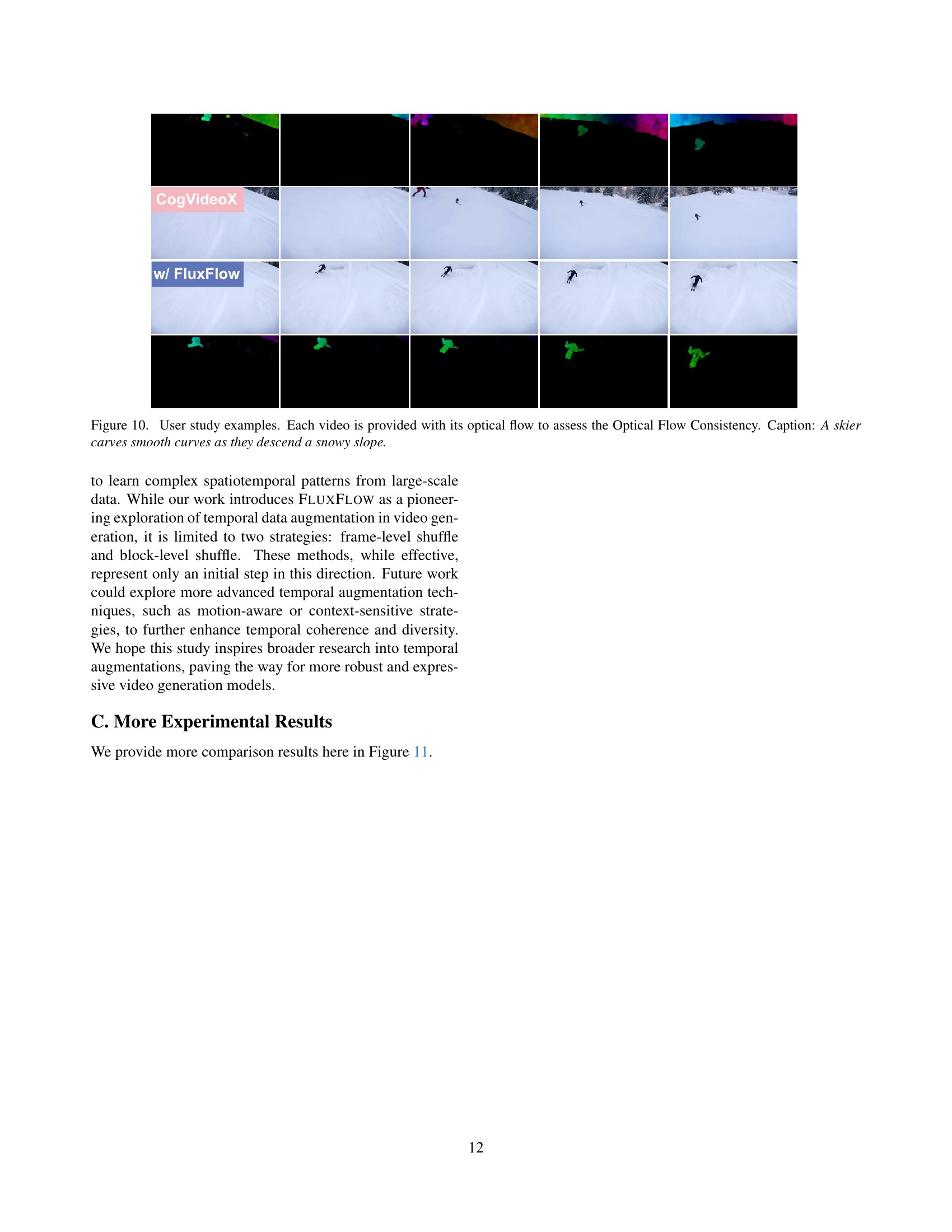
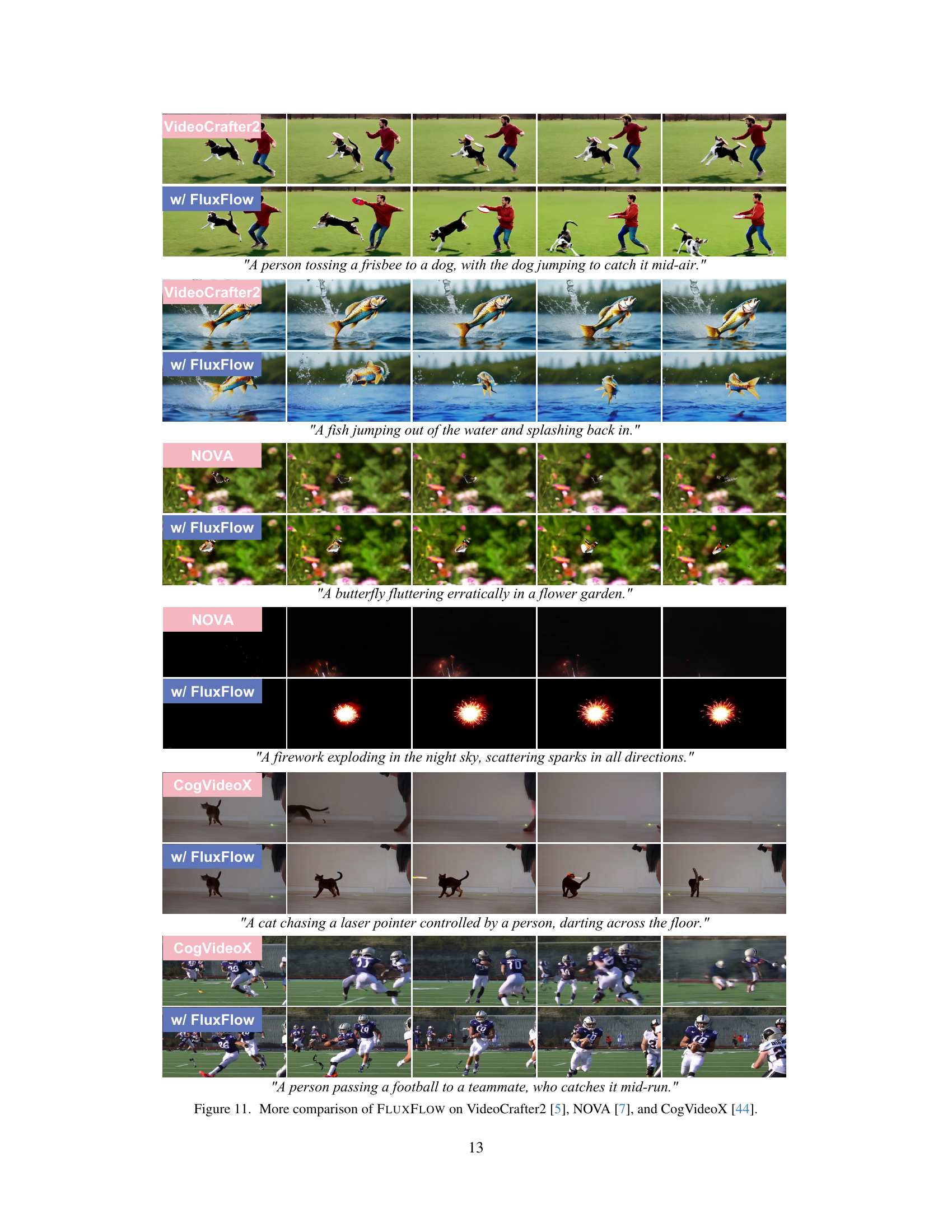