TL;DR#
In an era dominated by rampant misinformation, deciphering the interplay between humor and deception is crucial. Current methods struggle to differentiate harmless comedy from harmful misinformation. Addressing this challenge, this paper introduces the Deceptive Humor Dataset (DHD), a novel resource designed for studying humor derived from fabricated claims and misinformation. This new dataset is crucial for analyzing how humor intertwines with deception and for helping develop better methods to distinguish harmless comedy from harmful misinformation.
DHD comprises humor-infused comments generated using the ChatGPT-4o model from false narratives and manipulated information. Each instance is meticulously labeled with a Satire Level and classified into five distinct Humor Categories: Dark Humor, Irony, Social Commentary, Wordplay, and Absurdity. Spanning multiple languages, including English, Telugu, Hindi, Kannada, Tamil, and their code-mixed variations, DHD serves as a valuable multilingual benchmark. The paper establishes strong baselines for the proposed dataset, setting a strong foundation for future exploration and for advancing deceptive humor detection models.
Key Takeaways#
Why does it matter?#
This paper introduces a new dataset that enables the analysis of how humor can be used to spread misinformation. This research provides a test case for humor detection models and opens avenues for research into combating deceptive humor online.
Visual Insights#
🔼 This flowchart illustrates the process of generating the Deceptive Humor Dataset (DHD). It starts with gathering fake claims from various fact-checking websites. These claims are then fed into a structured prompt for the ChatGPT-40 model, which generates humorous comments in multiple languages (including code-mixed variations). Human reviewers then evaluate and refine these comments, ensuring quality and appropriateness before labeling them with satire level (1-3) and humor attributes (Dark Humor, Irony, Social Commentary, Wordplay, Absurdity). This process creates a diverse and controlled dataset ready for research.
read the caption
Figure 1: Flowchart for the DHD Data Generation
Statistic | Train | Validation | Test |
---|---|---|---|
Total Samples | 7,200 | 900 | 900 |
Satire Level Distribution | |||
Low Satire | 2,092 | 251 | 253 |
Moderate Satire | 3,125 | 378 | 388 |
High Satire | 1,983 | 271 | 259 |
Humor Attribute Distribution | |||
Irony | 2,180 | 270 | 284 |
Absurdity | 1,639 | 196 | 208 |
Social Commentary | 1,217 | 149 | 142 |
Dark Humor | 1,101 | 154 | 129 |
Wordplay | 1,063 | 131 | 137 |
🔼 This table presents the distribution of samples in the Deceptive Humor Dataset (DHD). It shows the counts of training, validation, and test samples. Furthermore, it breaks down the distribution by Satire Level (Low, Moderate, High) and Humor Attribute (Irony, Absurdity, Social Commentary, Dark Humor, Wordplay). This provides a comprehensive overview of the dataset’s composition, showing the balance of different humor types and satire levels across the different sets.
read the caption
Table 1: DHD Distribution
In-depth insights#
Humor & Deception#
The intersection of humor and deception is a complex and under-explored area. Humor can mask deceptive intent, making it difficult to discern harmless jokes from harmful misinformation. Traditional humor relies on exaggeration or irony, while deceptive humor distorts narratives for comedic effect. This is especially concerning in online spaces where jokes spread rapidly, blurring the lines between comedy and harmful falsehoods. The subjective nature of humor means that what one person finds funny, another may find offensive or misleading. Detecting deceptive humor requires considering the audience’s awareness of the underlying claim’s truthfulness, as well as deeper contextual understanding. The increasing volume of online content makes addressing deceptive humor more critical than ever, since the uncritical consumption can lead to the spread of misinformation and societal consequences. Research must develop detection mechanisms to differentiate between deceptive humor and harmless comedy, ensuring that humor is not used to spread harmful narratives.
Multilingual DHD#
The concept of a “Multilingual DHD” (Deceptive Humor Dataset) is intriguing, suggesting a resource that transcends linguistic boundaries to analyze how humor and deception intersect across cultures. This is crucial because humor is often deeply rooted in cultural context, making it challenging to detect deceptive intent without understanding the nuances of each language and its associated social norms. A multilingual DHD would need to account for variations in satire, irony, and other forms of humor across different languages, and how these are used to subtly spread misinformation. Constructing such a dataset requires careful consideration of the linguistic diversity and potential biases present in each language. It also opens doors to cross-cultural comparisons of deceptive strategies, revealing whether certain techniques are more prevalent or effective in specific linguistic contexts. The successful creation of a Multilingual DHD can significantly advance our understanding of global communication patterns and improve the accuracy of misinformation detection models, especially those deployed in diverse online environments. Such a resource could also be valuable for training AI models to identify and flag deceptive humor in multiple languages, enhancing their ability to moderate content and prevent the spread of harmful narratives.
ChatGPT-4o Bias#
While the provided document doesn’t explicitly address ‘ChatGPT-4o Bias,’ we can infer potential biases by analyzing the methodologies employed. The paper relies on ChatGPT-4o for data generation, which inherently introduces biases present in the model’s training data. These biases could skew the dataset towards certain perspectives or humor styles, affecting the generalizability of the benchmark. The manual review process may not entirely eliminate these underlying biases. Language experts, while assessing appropriateness and engagement, might inadvertently introduce their own cultural or regional biases into the evaluation. The document also acknowledges the limitations of synthetic data not fully replicating the nuances of human-generated content, which can further compound the impact of inherent biases in the ChatGPT-4o model. Therefore, the absence of explicit discussion on ‘ChatGPT-4o Bias’ is a significant omission, potentially undermining the reliability and validity of the dataset for broader applications. Future research should focus on quantifying and mitigating such biases for more robust and trustworthy results.
Context is Key#
Understanding that ‘Context is Key’ underscores the complex nature of humor and its interpretation, especially within deceptive narratives. The paper recognizes that humor’s impact heavily relies on audience awareness, differing based on individual perception; what one finds humorous, another may find offensive or misleading, especially if the underlying claim’s falsity is unknown, thereby presenting challenges in its detection. Contextual awareness becomes vital in distinguishing between harmless comedy and harmful misinformation. The pervasiveness of deceptive humor in social media, often interwoven with fabricated claims, necessitates robust detection mechanisms to prevent the unintentional spread of harmful content, the paper’s success hinges on crafting synthetic data that mimics human-like complexity, and therefore careful prompt design is essential to contextual relevance, linguistic diversity. By focusing on a nuanced understanding of intent and surrounding information, the proposed research addresses critical gaps in both humor and misinformation detection, emphasizing that effective analysis requires considering the broader social, political, or cultural landscape.
Future Mitigation#
Future mitigation strategies for deceptive humor require a multi-faceted approach. Improved detection models integrating fact verification and intent recognition are vital. The development of datasets that more accurately reflect the complexities of real-world human-generated content is crucial, going beyond synthetic data limitations. Ethical guidelines for the use of AI-generated content must be strictly enforced to prevent malicious applications. Finally, educational initiatives can raise awareness about deceptive humor’s impact and promote critical thinking skills, empowering individuals to discern and resist its influence.
More visual insights#
More on figures
🔼 This figure displays heatmaps visualizing the relationship between satire level (low, medium, high) and humor attribute (Absurdity, Dark Humor, Irony, Social Commentary, Wordplay) across three datasets: training, validation, and testing. The color intensity in each cell of the heatmap corresponds to the frequency of comments exhibiting that specific combination of satire level and humor attribute within the dataset. Darker colors indicate higher frequency. This allows for a visual analysis of the distribution of different humor types across varying satire levels and how the proportions change between the training, validation and test sets.
read the caption
Figure 2: Satire Level vs Humor Attribute. The color intensity represents the frequency distribution across datasets.
🔼 This heatmap visualizes how different humor attributes (Irony, Absurdity, Social Commentary, Dark Humor, Wordplay) are distributed across various languages (English, Telugu, Hindi, Kannada, Tamil, and their code-mixed versions). The intensity of color in each cell represents the frequency of a particular humor attribute within a given language. This allows for a comparison of humor styles and preferences across different linguistic groups and helps understand the linguistic nuances in expressing humor within those groups. Darker colors indicate higher frequencies.
read the caption
Figure 3: Language Variants vs Humor Attribute. The heatmap highlights humor variations across languages.
🔼 This figure presents a heatmap visualization analyzing the relationship between different languages and the levels of satire in deceptive humor. Each heatmap represents a different dataset split (train, validation, test). The x-axis represents satire levels (low, medium, high), and the y-axis shows the languages used (English, Telugu, Hindi, Kannada, Tamil, and their code-mixed variants). The color intensity of each cell reflects the frequency of comments in that language category at that particular satire level. Darker colors indicate higher frequencies. This helps illustrate whether certain languages tend to be associated with different satire levels in the dataset.
read the caption
Figure 4: Language Variants vs Satire Level. The color intensity represents frequency distribution, showing how different languages align with satire levels.
More on tables
Language | Total Records | Satire Level | Humor Attribute | ||||||
---|---|---|---|---|---|---|---|---|---|
1 | 2 | 3 | Absurdity | Dark Humor | Irony | Social Commentary | Wordplay | ||
Train Data | |||||||||
English | 877 | 190 | 323 | 364 | 243 | 147 | 297 | 101 | 89 |
Telugu | 819 | 300 | 345 | 174 | 178 | 111 | 321 | 162 | 47 |
Hindi | 822 | 264 | 386 | 172 | 166 | 90 | 285 | 169 | 112 |
Kannada | 771 | 268 | 348 | 155 | 203 | 95 | 218 | 185 | 70 |
Tamil | 725 | 231 | 343 | 151 | 183 | 99 | 238 | 143 | 62 |
Telugu-English | 815 | 194 | 345 | 276 | 195 | 153 | 206 | 114 | 147 |
Hindi-English | 831 | 219 | 349 | 263 | 122 | 137 | 233 | 130 | 209 |
Kannada-English | 768 | 199 | 329 | 240 | 189 | 162 | 175 | 110 | 132 |
Tamil-English | 772 | 227 | 357 | 188 | 160 | 107 | 207 | 103 | 195 |
Validation Data | |||||||||
English | 112 | 21 | 31 | 60 | 38 | 23 | 31 | 12 | 8 |
Telugu | 110 | 33 | 49 | 28 | 19 | 19 | 48 | 23 | 1 |
Hindi | 100 | 38 | 40 | 22 | 20 | 9 | 30 | 24 | 17 |
Kannada | 84 | 30 | 30 | 24 | 18 | 10 | 27 | 21 | 8 |
Tamil | 88 | 29 | 43 | 16 | 17 | 11 | 34 | 16 | 10 |
Telugu-English | 104 | 23 | 49 | 32 | 20 | 23 | 32 | 10 | 19 |
Hindi-English | 99 | 23 | 53 | 23 | 11 | 12 | 24 | 21 | 31 |
Kannada-English | 108 | 27 | 45 | 36 | 32 | 25 | 17 | 14 | 20 |
Tamil-English | 95 | 27 | 38 | 30 | 21 | 22 | 27 | 8 | 17 |
Test Data | |||||||||
English | 110 | 21 | 37 | 52 | 32 | 20 | 39 | 11 | 8 |
Telugu | 117 | 38 | 51 | 28 | 33 | 15 | 43 | 18 | 8 |
Hindi | 80 | 23 | 47 | 10 | 22 | 6 | 27 | 16 | 9 |
Kannada | 81 | 29 | 32 | 20 | 22 | 15 | 19 | 17 | 8 |
Tamil | 100 | 32 | 49 | 19 | 28 | 9 | 44 | 13 | 6 |
Telugu-English | 114 | 26 | 44 | 44 | 23 | 20 | 25 | 15 | 31 |
Hindi-English | 95 | 25 | 41 | 29 | 14 | 13 | 23 | 17 | 28 |
Kannada-English | 115 | 32 | 55 | 28 | 21 | 19 | 36 | 16 | 23 |
Tamil-English | 88 | 27 | 32 | 29 | 13 | 12 | 28 | 19 | 16 |
🔼 This table presents a detailed breakdown of the distribution of satire levels and humor attributes across various languages included in the Deceptive Humor Dataset (DHD). It shows the frequency of different satire levels (low, medium, high) and humor attributes (irony, absurdity, social commentary, dark humor, wordplay) within each language (English, Telugu, Hindi, Kannada, Tamil, and their code-mixed variations). This distribution helps to understand the diversity and balance of the dataset, showing how humor and satire are expressed differently across languages and linguistic styles. Appendix E provides a visualization for a clearer understanding.
read the caption
Table 2: Distribution of Satire Levels and Humor Attributes Across Languages (Appendix E provides a visualization of these distributions).
Hyperparameter | Value |
---|---|
Max Len | 128 |
Batch Size | 16 |
Epochs | 5 |
Learning Rate | 3e-5 |
Drop Out | 0.3 |
🔼 This table details the hyperparameters used in the experiments evaluating the performance of encoder-only and encoder-decoder models on the Deceptive Humor Dataset (DHD). It lists the specific values used for key parameters such as maximum sequence length, batch size, number of training epochs, learning rate, and dropout rate. These settings are crucial for reproducibility and understanding the experimental setup. The table is divided into two sections, one for Encoder-Only models and one for Encoder-Decoder models, reflecting the different model architectures used in the study.
read the caption
Table 3: Hyperparameter settings for Encoder-Only and Encoder-Decoder Models.
Hyperparameter | Value |
---|---|
LoRA Rank () | 16 |
LoRA Alpha | 64 |
LoRA Dropout | 0.2 |
Target Modules | {k_proj, q_proj, v_proj} |
Learning Rate | 2e-5 |
Batch Size | 8 |
Epochs | 3 |
Weight Decay | 0.01 |
🔼 This table details the hyperparameter settings used for fine-tuning large language models (LLMs) in the experiments. These settings are crucial for reproducibility and understanding how these parameters influenced the model’s performance on the Deceptive Humor Dataset (DHD). The hyperparameters listed likely include values for LORA (Low-Rank Adaptation) parameters such as rank, alpha, and dropout; along with other standard hyperparameters like learning rate, batch size, number of epochs, and weight decay. The specific parameters and values were chosen to optimize the LLMs’ ability to classify deceptive humor effectively.
read the caption
Table 4: Hyperparameter settings for the LLM Models.
Model | Satire Level | Humor Attribute | Parameters | ||||||
---|---|---|---|---|---|---|---|---|---|
Accuracy | F1-Score | Precision | Recall | Accuracy | F1-Score | Precision | Recall | ||
Encoder-Only | |||||||||
BERTKenton and Toutanova (2019) | 49.44 | 46.47 | 49.22 | 46.19 | 40.44 | 34.73 | 44.37 | 34.42 | 110M |
DistilBERTSanh (2019) | 49.44 | 45.60 | 49.42 | 45.60 | 37.89 | 34.58 | 38.95 | 34.09 | 66M |
mBERT | 50.44 | 50.00 | 49.98 | 50.06 | 36.00 | 35.77 | 37.19 | 35.00 | 110M |
XLM-RoBERTaConneau (2019) | 49.33 | 48.87 | 48.86 | 48.93 | 34.44 | 33.70 | 33.95 | 33.60 | 125M |
DeBERTaHe et al. (2020) | 47.89 | 42.52 | 49.07 | 43.29 | 37.33 | 28.76 | 30.00 | 31.51 | 184M |
ALBERTLan (2019) | 46.33 | 43.52 | 46.24 | 43.25 | 38.89 | 33.33 | 39.91 | 33.56 | 11M |
XLNetYang (2019) | 50.44 | 46.72 | 50.84 | 46.63 | 36.78 | 25.49 | 31.41 | 28.52 | 117M |
Encoder-Decoder | |||||||||
BARTLewis (2019) | 49.11 | 46.44 | 49.34 | 46.09 | 37.89 | 33.84 | 37.93 | 33.93 | 407M |
mBARTLiu (2020) | 51.00 | 50.24 | 51.04 | 49.82 | 36.11 | 35.60 | 36.32 | 35.22 | 680M |
T5Raffel et al. (2020) | 46.78 | 43.64 | 46.37 | 43.53 | 37.67 | 28.48 | 36.02 | 30.50 | 738M |
Decoder-Only (Zero-Shot) | |||||||||
GemmaTeam et al. (2024) | 35.78 | 28.76 | 30.03 | 31.46 | 23.44 | 14.66 | 13.44 | 19.47 | 7000M |
LlamaTouvron et al. (2023) | 27.78 | 19.23 | 27.10 | 32.73 | 21.11 | 16.65 | 20.55 | 20.60 | 8000M |
Phi-4Abdin et al. (2024) | 42.40 | 20.35 | 20.93 | 32.90 | 15.00 | 11.35 | 10.33 | 20.22 | 14000M |
Decoder-Only (Few-Shot) | |||||||||
Gemma | 43.11 | 20.08 | 14.37 | 33.33 | 31.56 | 9.59 | 6.31 | 20.00 | 7000M |
Llama | 28.78 | 14.90 | 9.59 | 33.33 | 29.67 | 12.08 | 10.04 | 19.93 | 8000M |
Phi-4 | 28.78 | 14.90 | 9.59 | 33.33 | 14.33 | 5.01 | 2.87 | 20.00 | 14000M |
Decoder-Only (QLoRA Fine-Tuned) | |||||||||
Gemma | 34.00 | 27.00 | 40.00 | 33.00 | 24.00 | 21.00 | 26.00 | 24.00 | 7000M |
Llama | 30.00 | 24.00 | 34.00 | 35.00 | 22.00 | 18.00 | 21.00 | 21.00 | 8000M |
Phi-4 | 35.00 | 29.00 | 34.00 | 34.00 | 26.00 | 12.00 | 35.00 | 18.00 | 14000M |
🔼 This table presents the performance of various NLP models on the Deceptive Humor Dataset (DHD) in classifying satire levels and humor attributes. It compares the accuracy, F1-score, precision, and recall of several encoder-only models, encoder-decoder models, and large language models (LLMs). The results show the effectiveness of different architectures in identifying different types of humor and varying degrees of satire. The top performing model for each metric is bolded, while the second-best is underlined, providing a clear comparison of model performance.
read the caption
Table 5: Baseline Metrics of Models Across Satire Levels and Humor Attributes. The top results are represented in bold, and the second-best results are underlined.
Language | Education | Gender | State |
---|---|---|---|
English | B.Tech | Male | Andhra Pradesh |
Telugu* | B.Tech | Male | Andhra Pradesh |
Hindi* | PhD Candidate | Male | Delhi |
Kannada* | PhD Candidate | Male | Karnataka |
Tamil* | B.Tech | Male | Tamil Nadu |
Country: India |
🔼 This table provides demographic information about the human evaluators who assessed the quality of the synthetically generated data in the Deceptive Humor Dataset (DHD). It shows the evaluators’ language expertise (including native and code-mixed languages), educational background, and gender. This information is crucial for understanding the potential biases or perspectives that might have influenced their evaluations and for ensuring the reproducibility and reliability of the study’s findings.
read the caption
Table 6: Profile of Human Evaluators Across Languages (* indicates Indic languages along with their code-mixed variants).
Language | Avg Satire Score | Avg Humor Score | Overall Avg. Score |
---|---|---|---|
English | 4.21 | 4.14 | 4.17 |
Telugu | 3.67 | 4.00 | 3.83 |
Hindi | 3.50 | 3.60 | 3.55 |
Kannada | 3.00 | 2.80 | 2.95 |
Tamil | 3.00 | 3.87 | 3.43 |
Telugu-Eng | 4.20 | 4.00 | 4.10 |
Hindi-Eng | 4.00 | 4.00 | 4.00 |
Kannada-Eng | 3.00 | 3.00 | 3.00 |
Tamil-Eng | 3.74 | 4.34 | 4.04 |
🔼 This table presents the results of human evaluation conducted to assess the quality of the synthetically generated humor data in the Deceptive Humor Dataset (DHD). The evaluation involved native speakers rating the satire level and humor attributes of samples across different languages (English and four Indian languages, including code-mixed variations). The scores are presented as averages across various language variants, offering insights into the quality and consistency of the humor generated by the model. Specifically, it provides average scores for satire, humor, and an overall combined score for each language and code-mixed language variant in the dataset.
read the caption
Table 7: Human Evaluation results for Satire, Humor, and Average scores across various language variants.
Language | Comment | Satire Level | Humor Attribute |
---|---|---|---|
En | Next up: redefining gravity because someone feels it’s oppressive. | 3 | Absurdity |
En | Mosques near stations now double as train wreck centers? Innovative blaming! | 3 | Dark Humor |
En | The Earth is so flat that NASA has been fooling us with Photoshop for decades. | 2 | Irony |
En | Even the beggars now ask, ’Do you have Paytm, sir? No cash, please!’ | 1 | Social Commentary |
En | They say sticks and stones break bones, but in lockdown, it was just ’discipline’! | 2 | Wordplay |
Te-En | So now Khalistan is getting into the protest business. Can I book a protest for my birthday? | 2 | Absurdity |
Te-En | Temples ni mosques chesthunnaru anta, next Ramayan ni alochinchi Game of Thrones laga chesthara? | 3 | Dark Humor |
Te-En | Corona ni jihadi tool ga use chesthunaru anta, inkem cheptaru? Wi-Fi lo use chesi live cheyyadam? | 2 | Irony |
Te-En | Arey mamsam tintaru ani cheppi kotha rule pettara WHO? | 1 | Social Commentary |
Te-En | Tablighi Jamaat spreading COVID-19 like it’s a secret mission, script lo plot twist thappa! | 3 | Wordplay |
Hi-En | Bhai, anthem ke jagah Azan bajegi kya ab? | 2 | Absurdity |
Hi-En | Bhai, China ke rice ke saath ek ’Recycle or Die’ sticker bhi milega! | 3 | Dark Humor |
Hi-En | China ka corona bio weapon? Aur hum lockdown comedy mein atak gaye! | 3 | Irony |
Hi-En | Arre bhai, ab roadside vendors bhi bolenge ’QR lagao ya aage badho’! | 2 | Social Commentary |
Hi-En | Plastic rice mein agar fiber hai, toh diet ke liye perfect hoga! | 3 | Wordplay |
Ka-En | Dhoni Instagram alli photo haakidre issue complete solve agathe anta! | 2 | Absurdity |
Ka-En | Bill Gates created COVID-19 to implant tracking devices, well, where’s the tracking device for ‘common sense’? | 3 | Dark Humor |
Ka-En | Digital rupee anta, but bank queue sullu badlu illa yenadre? | 1 | Irony |
Ka-En | Gas cylinder throw madi Haldwani summit-anna join agidru anta! | 1 | Social Commentary |
Ka-En | Flat earth andre nimma GPS ge flat map kodutha? | 1 | Wordplay |
Ta-En | Bro unga ooru marriage la gift QR code la thaane kudupaanga? | 2 | Absurdity |
Ta-En | World-a kaapatharadhukku virus-a anupa China, evvalavo innovation! | 3 | Dark Humor |
Ta-En | Church-ai set pannina history save aaguma? | 1 | Irony |
Ta-En | Temple oru ’creative hub’ nu solra trend WhatsApp-la! | 1 | Social Commentary |
Ta-En | Vaccines-ku microchip install panna? Mari app store-la update varuma? | 1 | Wordplay |
🔼 This table presents a sample of deceptive humorous comments generated in multiple languages (English and four Indian languages along with their code-mixed variants). Each comment is labeled with its corresponding satire level (1-3, with 1 being subtle and 3 being highly exaggerated) and humor attribute (Irony, Absurdity, Social Commentary, Dark Humor, or Wordplay). The table showcases the diversity of humor styles and linguistic variations achieved in the dataset, highlighting the complexity of classifying deceptive humor.
read the caption
Table 8: Deceptive humorous comments across different languages with their corresponding satire levels and humor attributes.
Full paper#
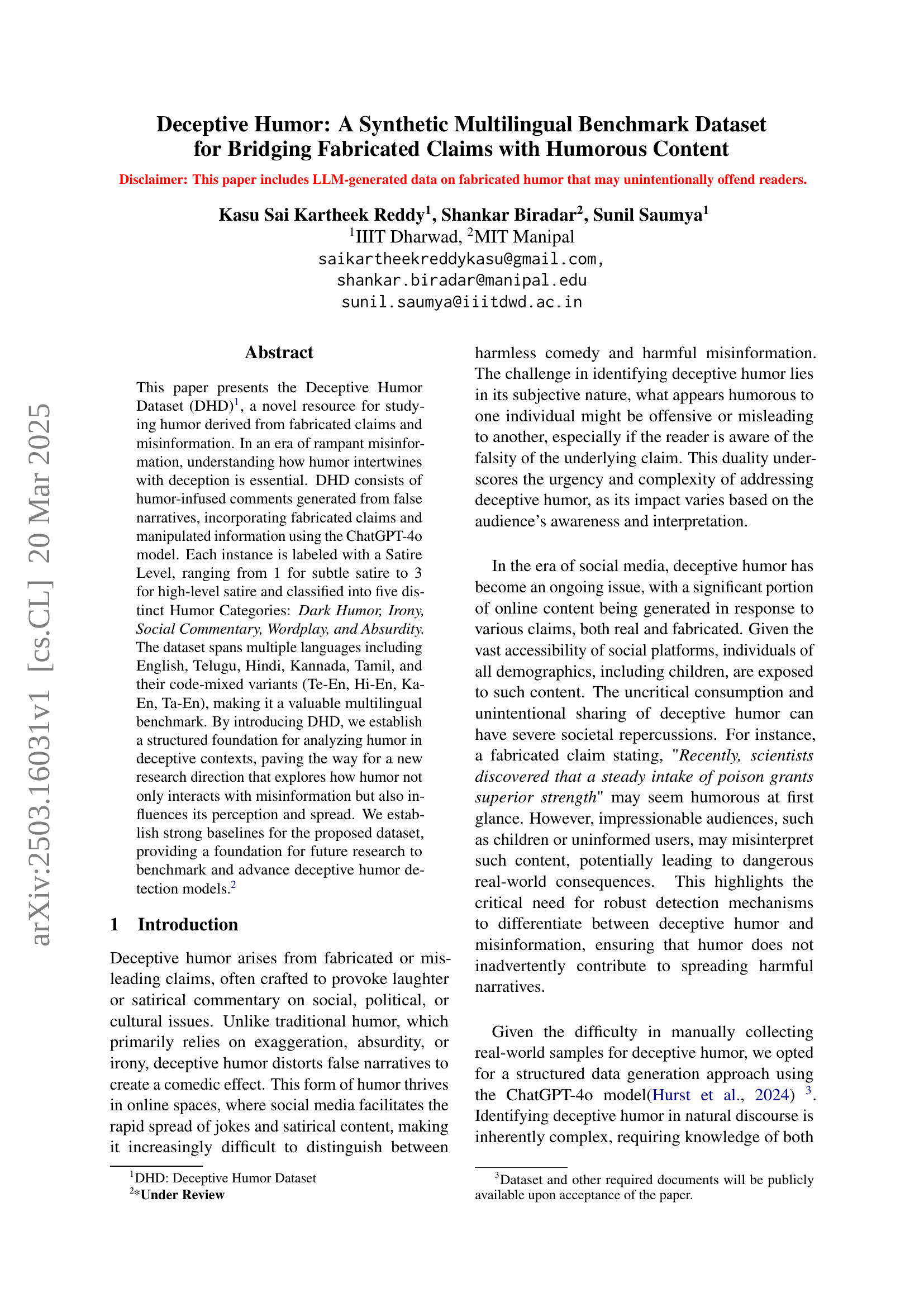
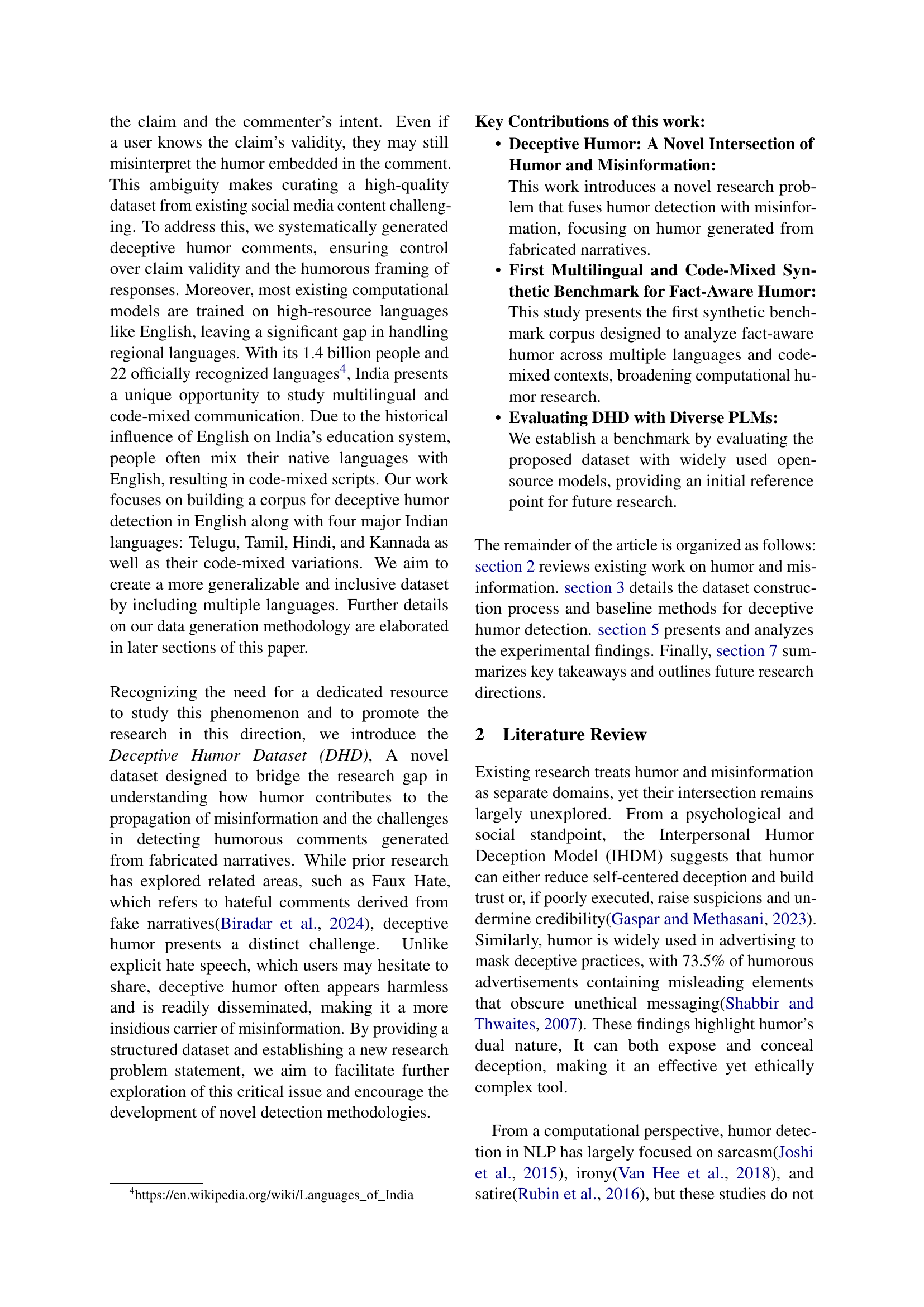
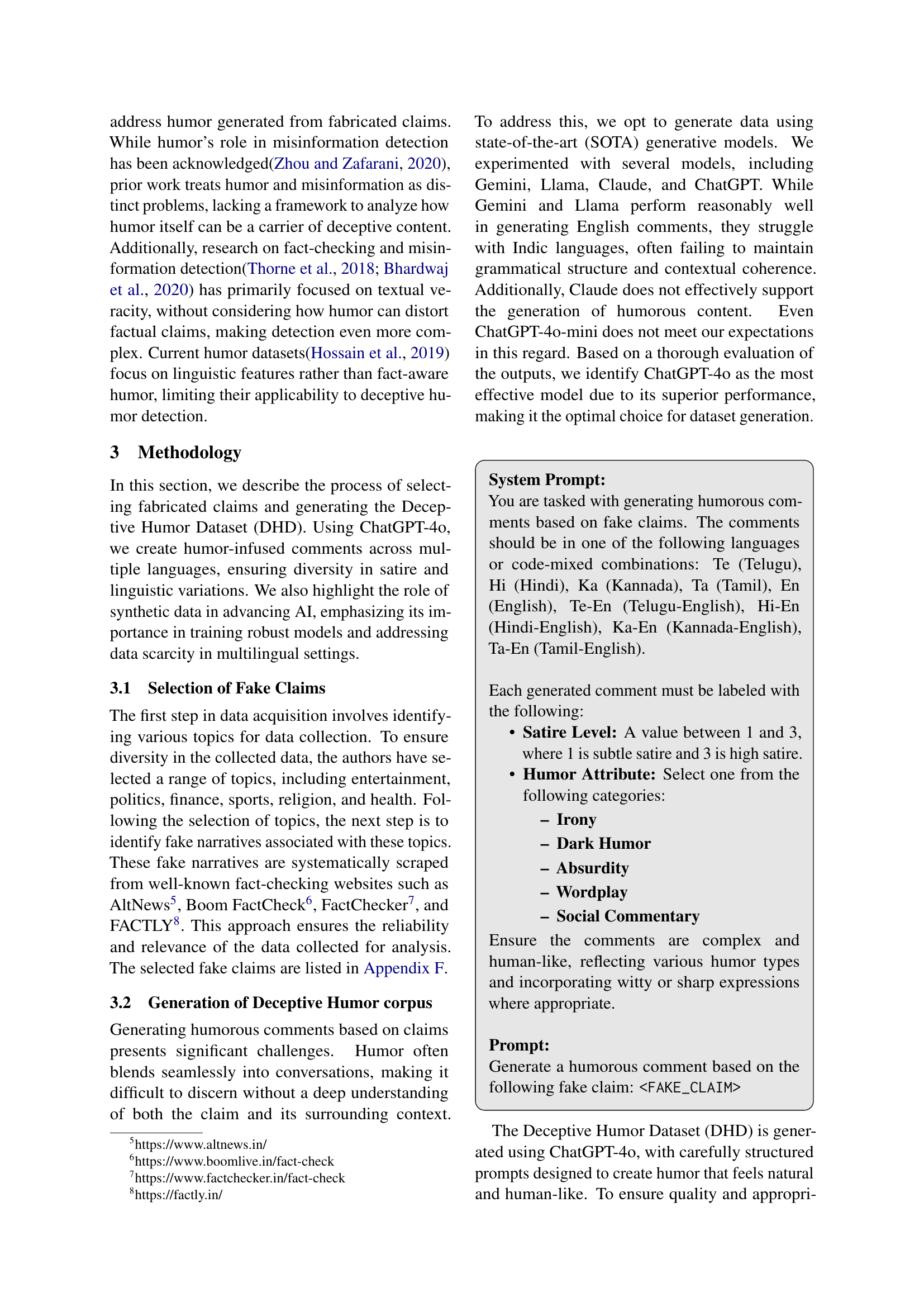
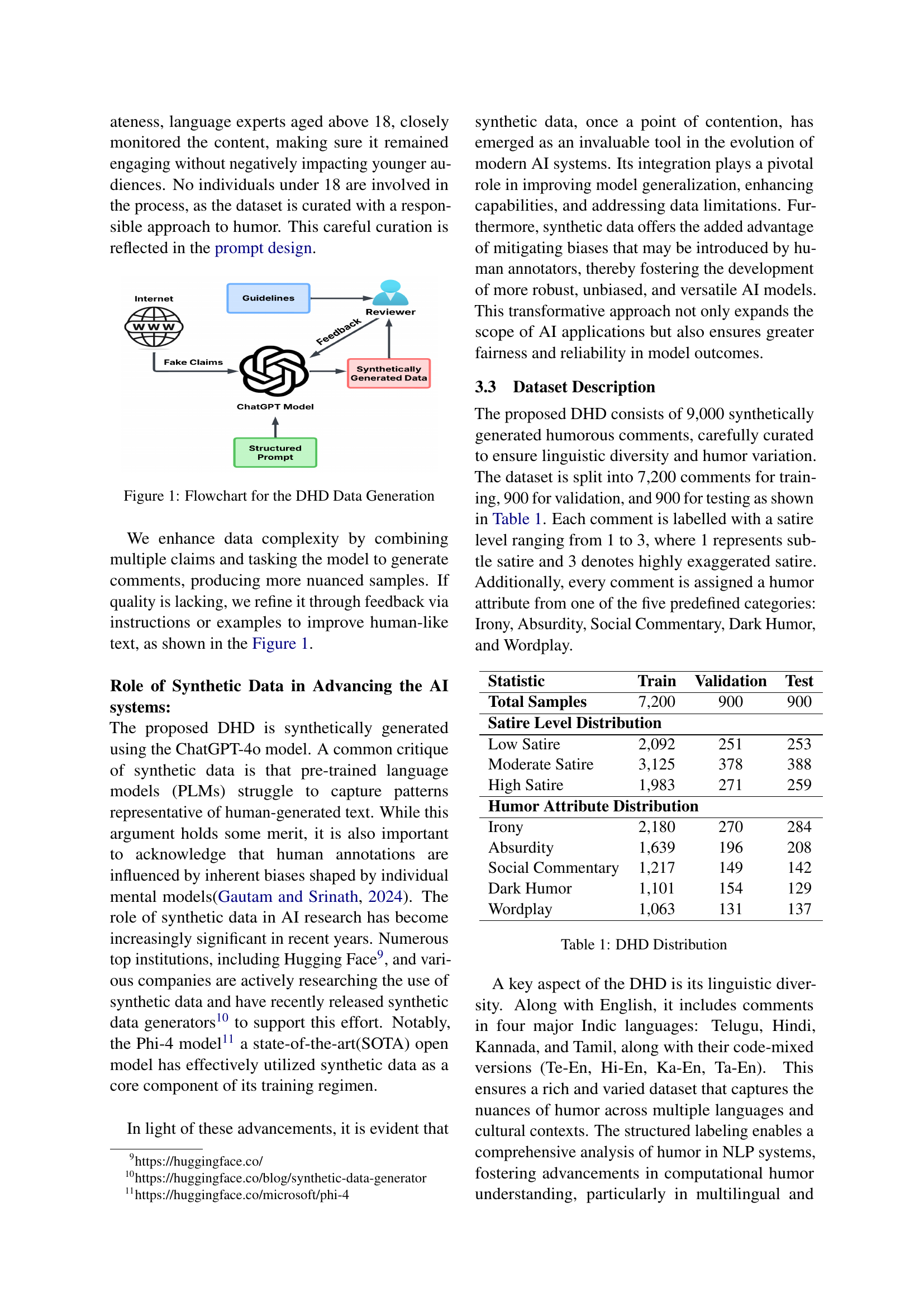
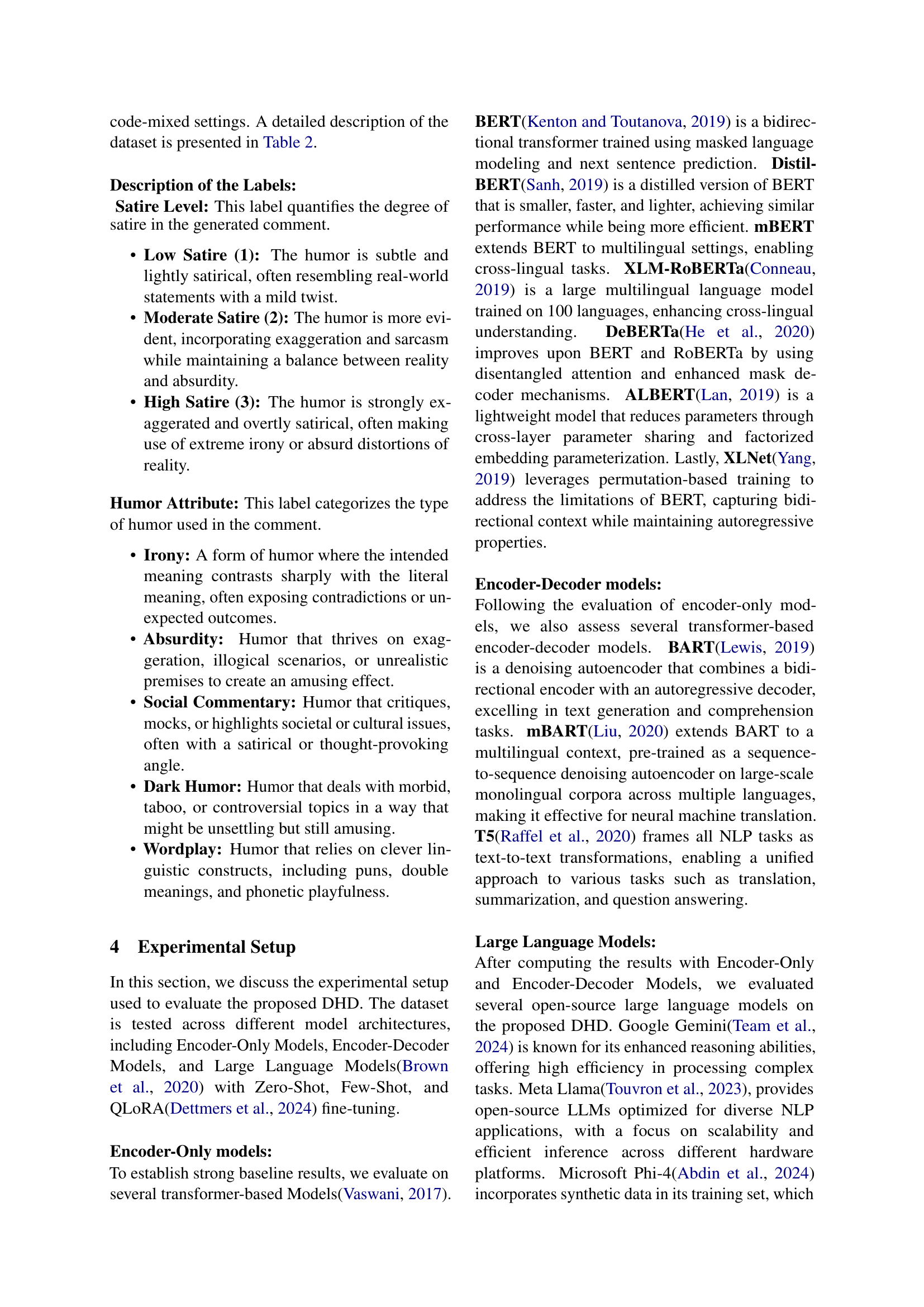
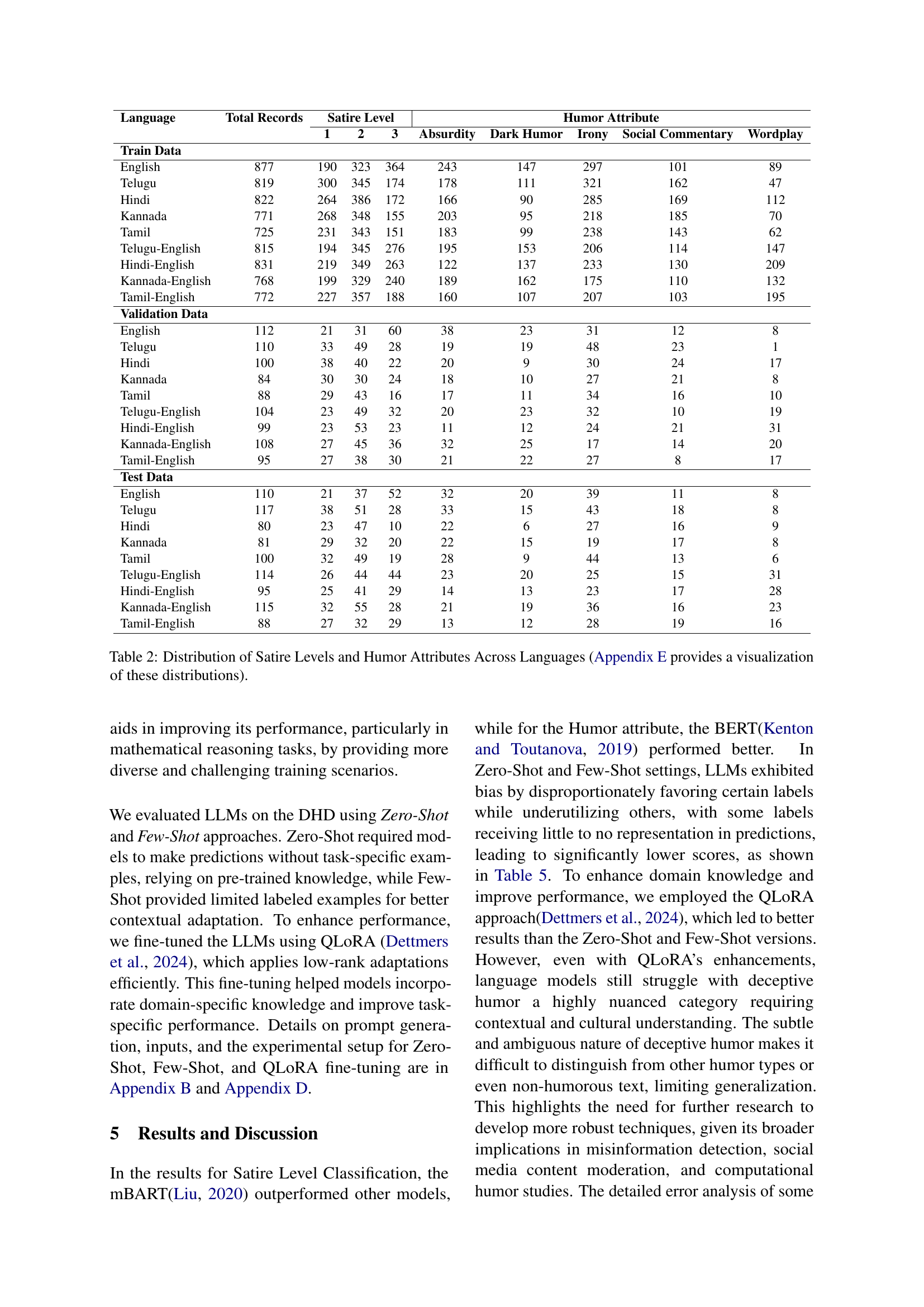
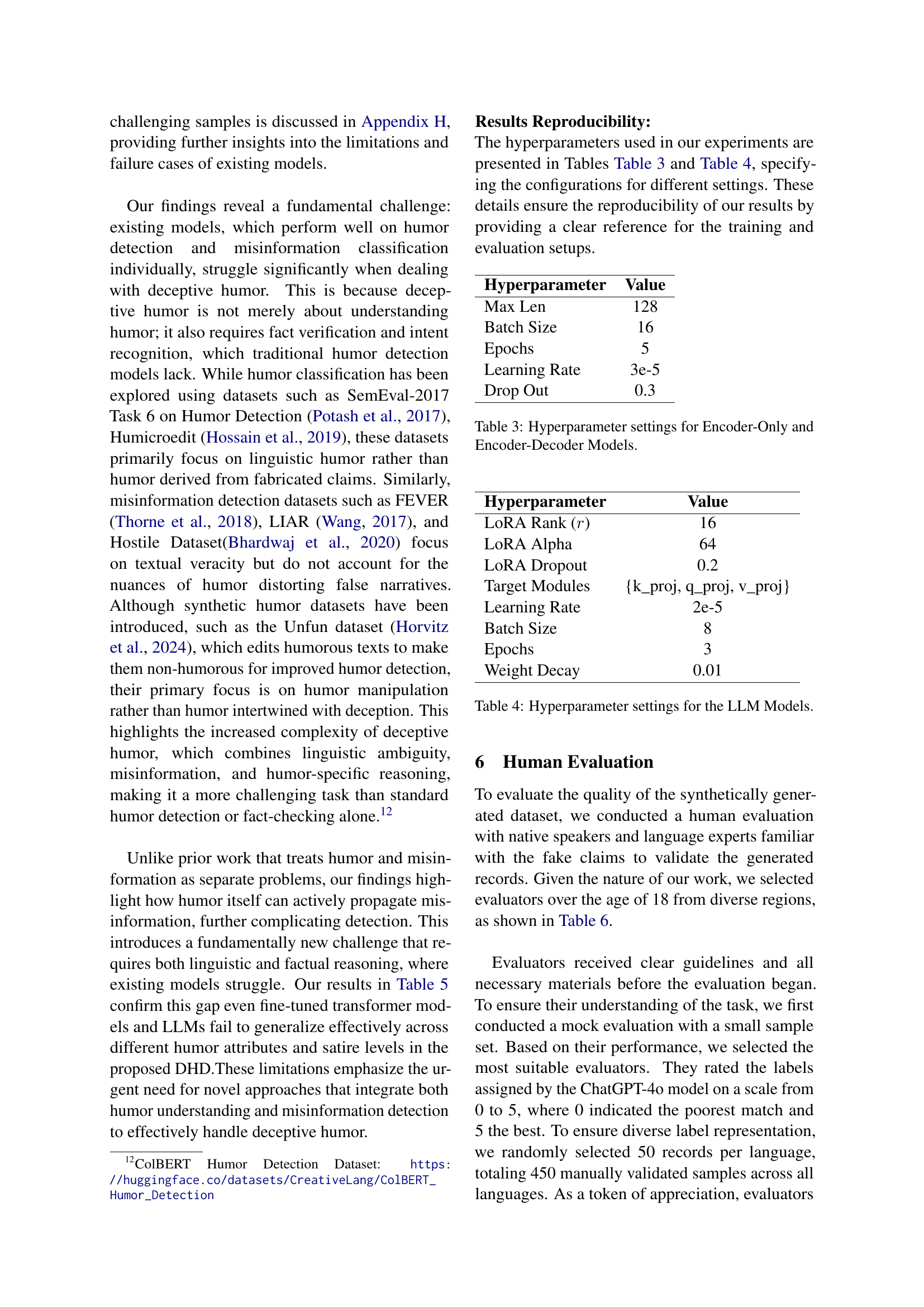
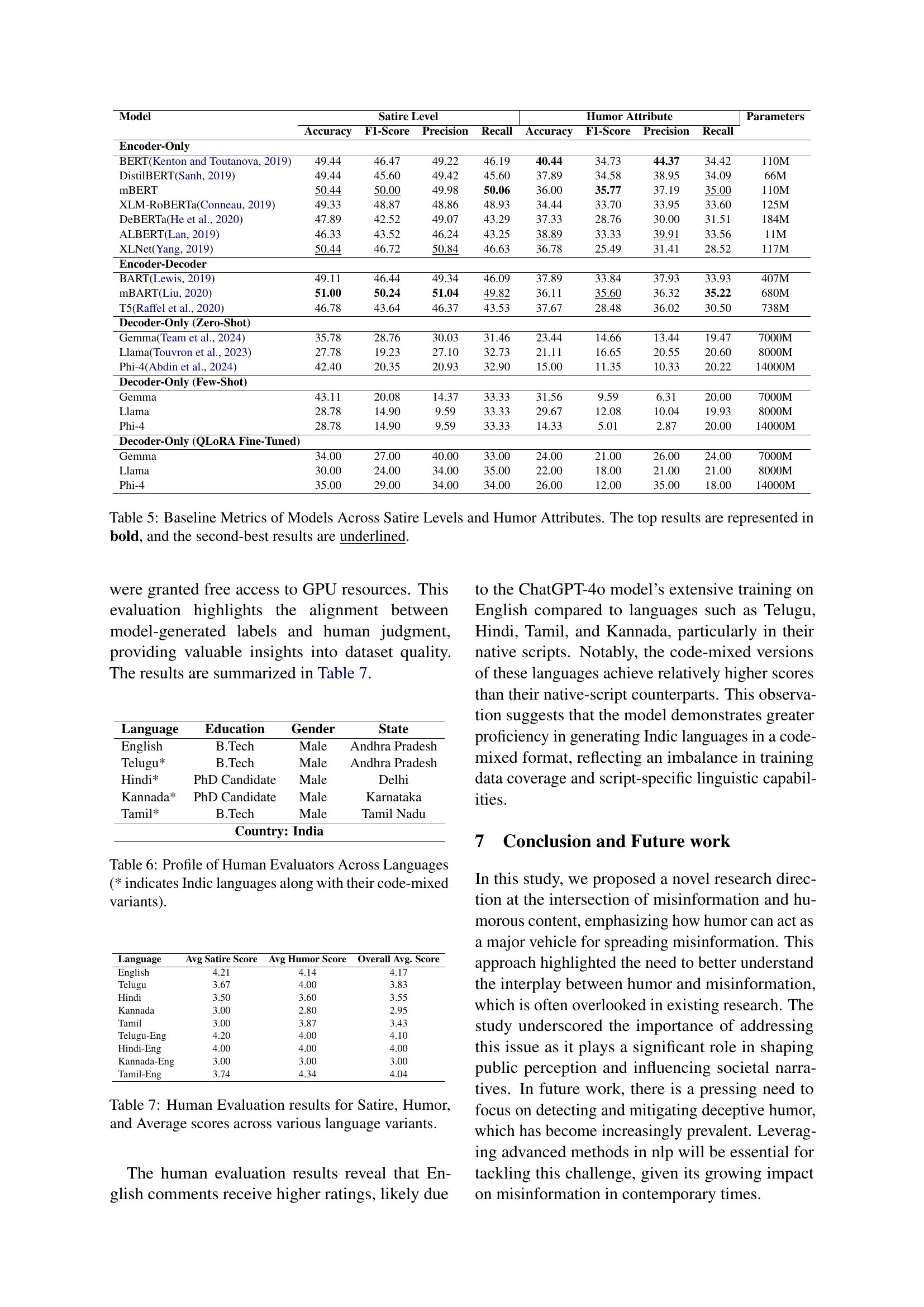
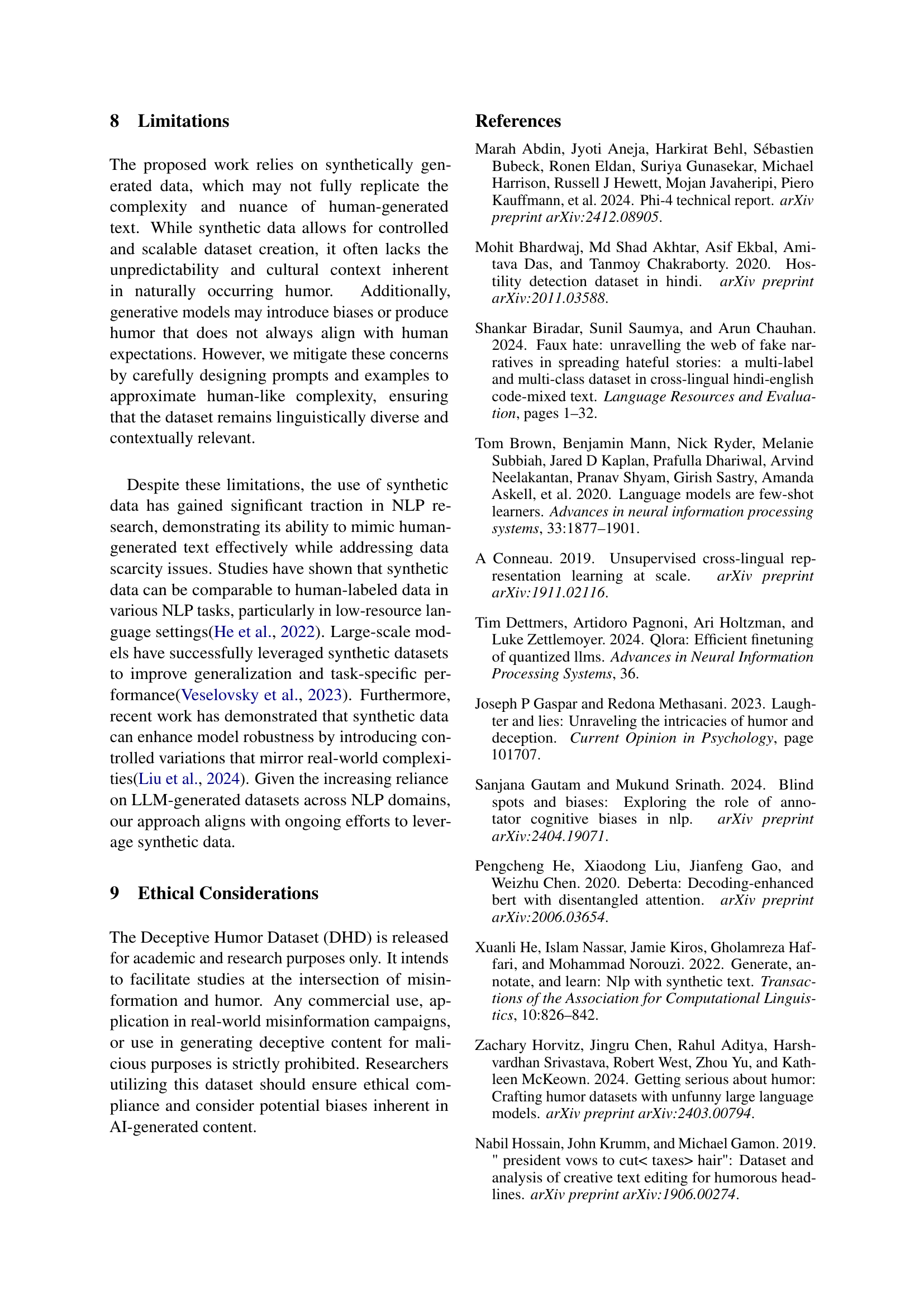
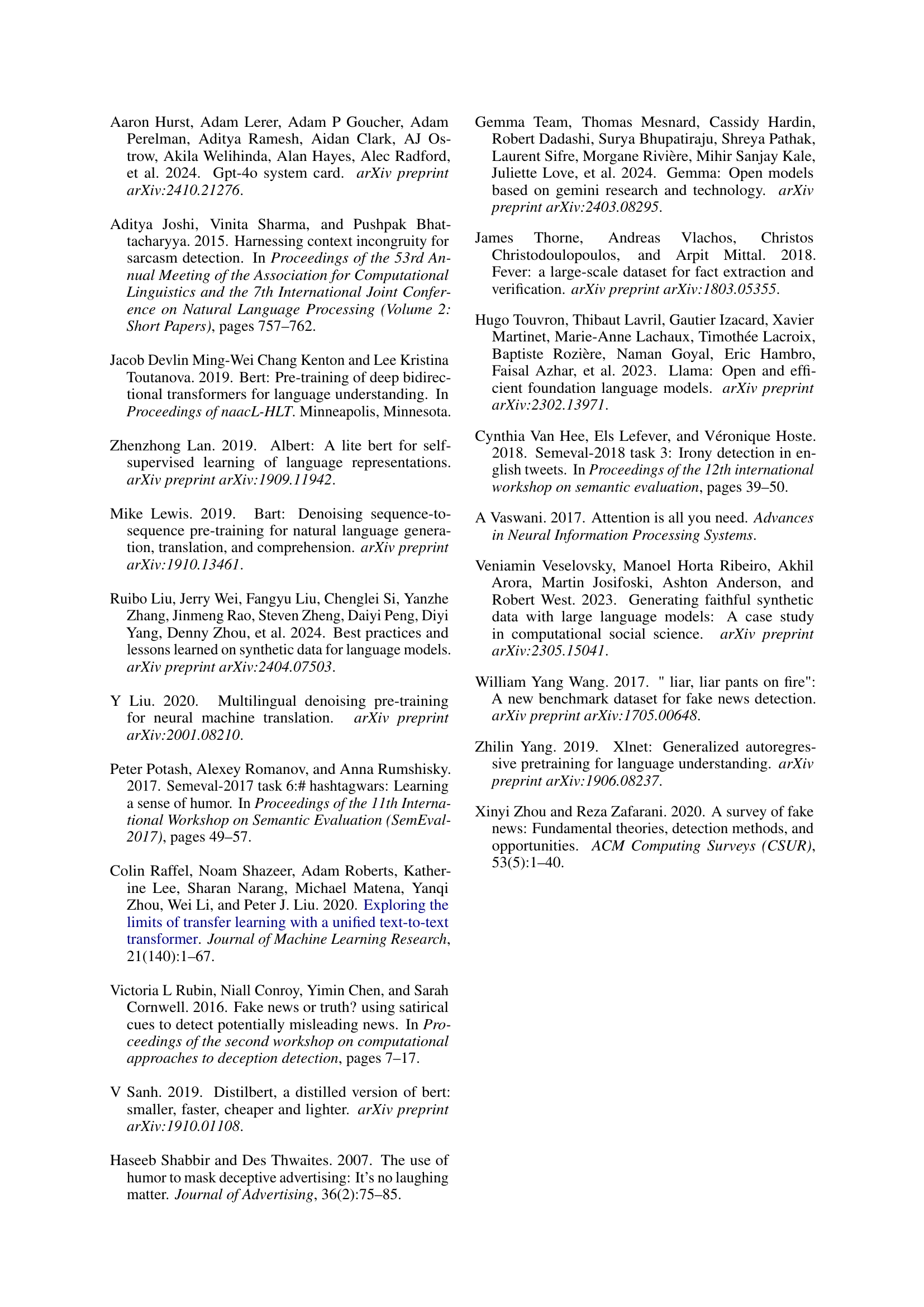
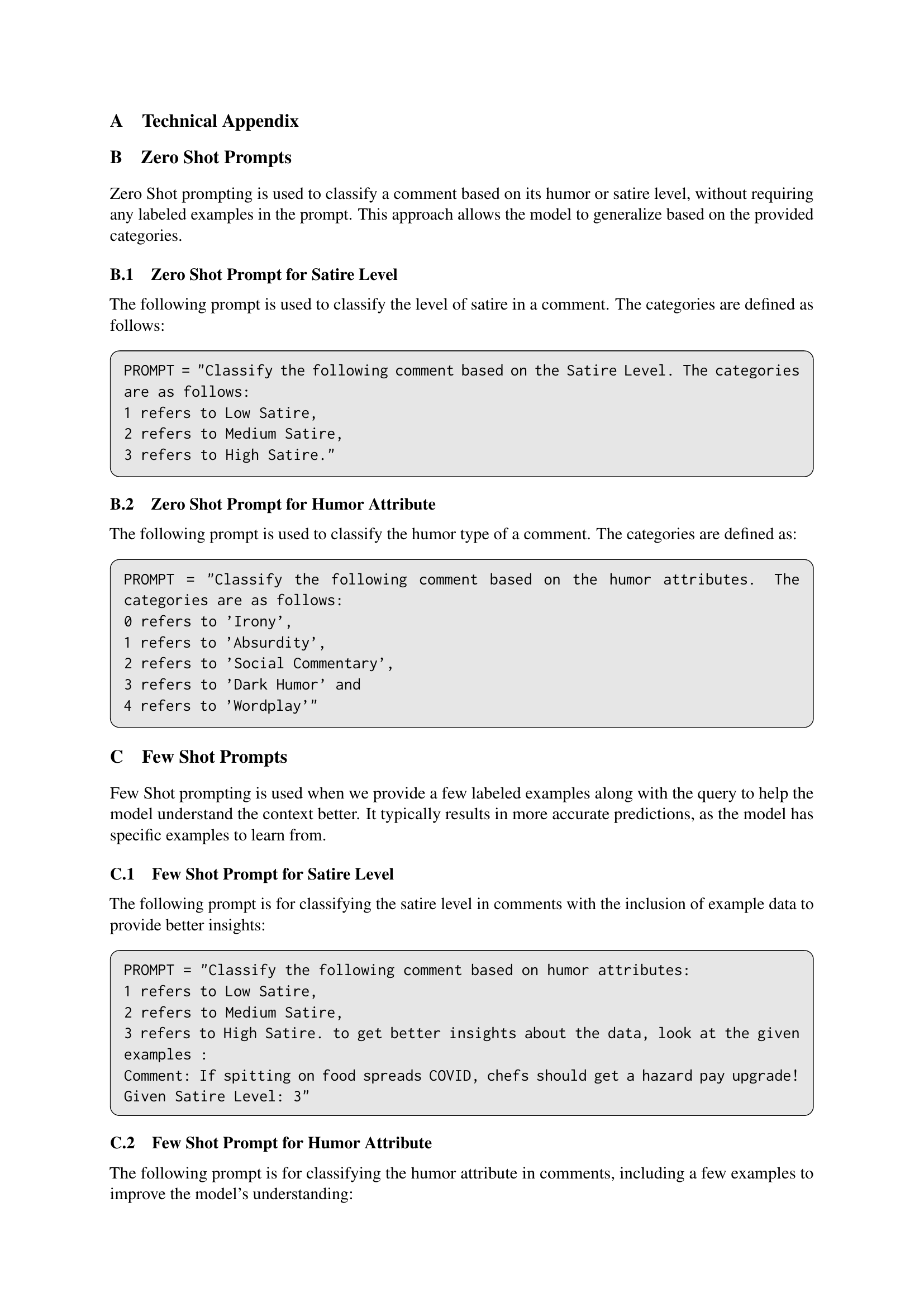
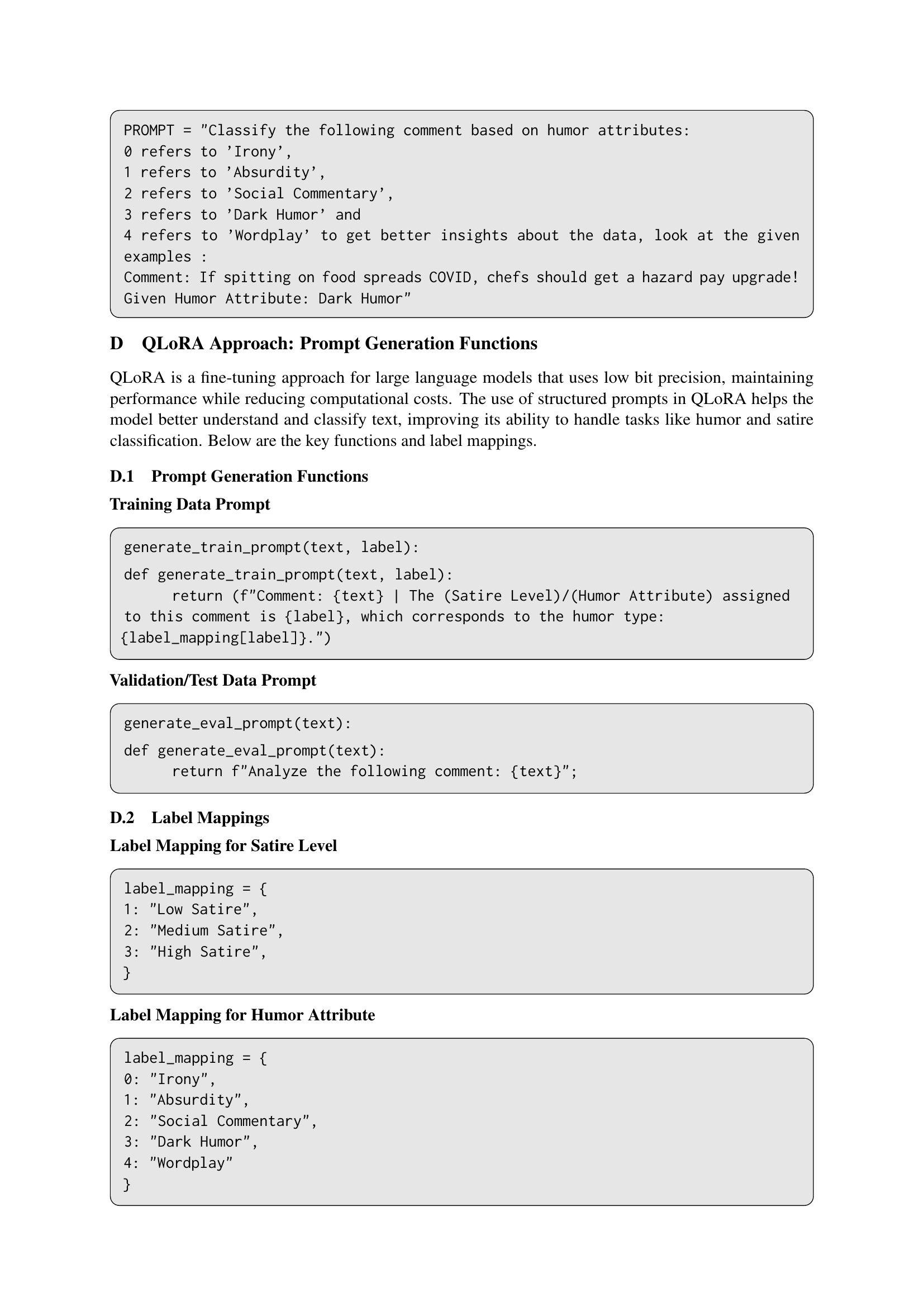
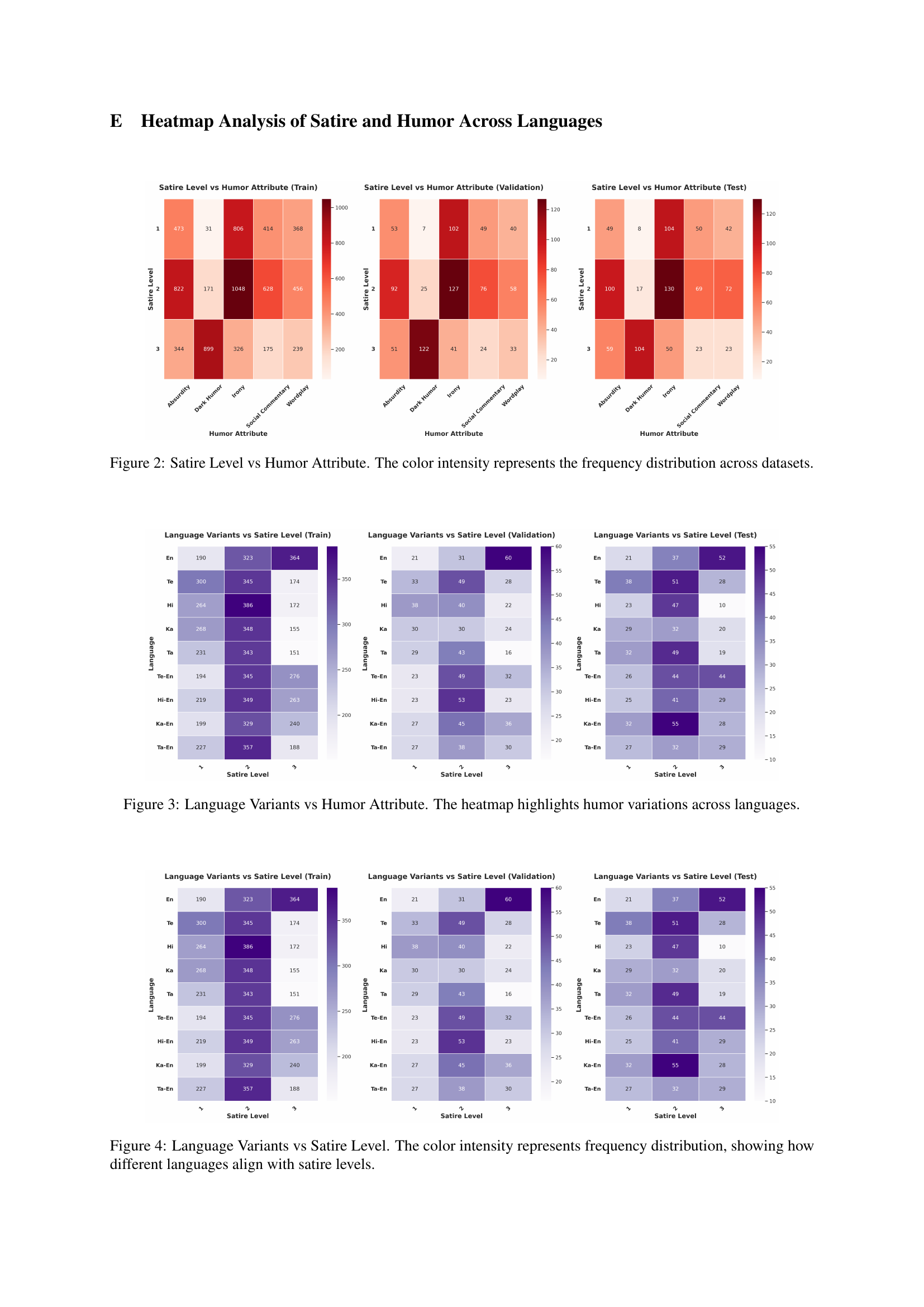
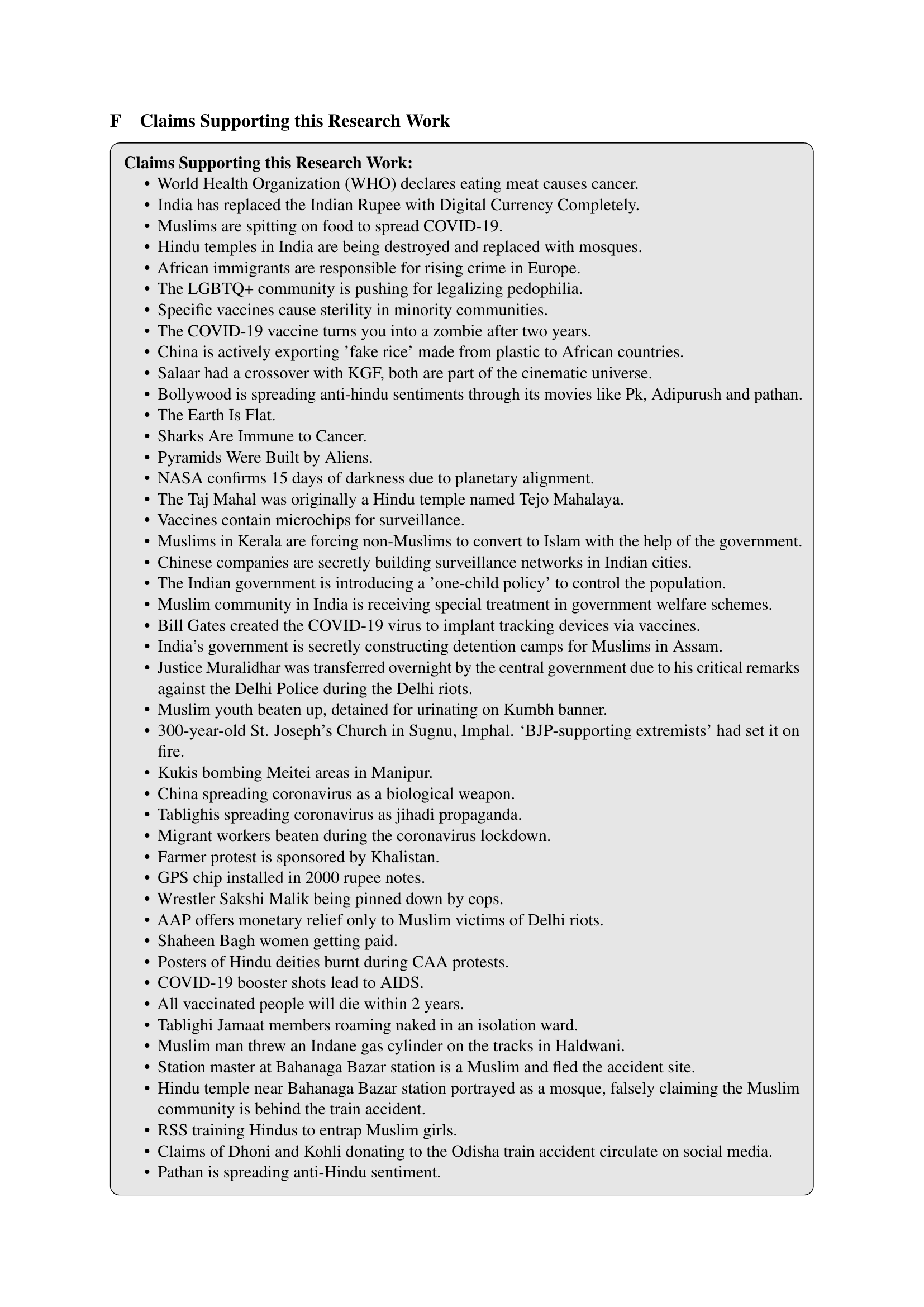
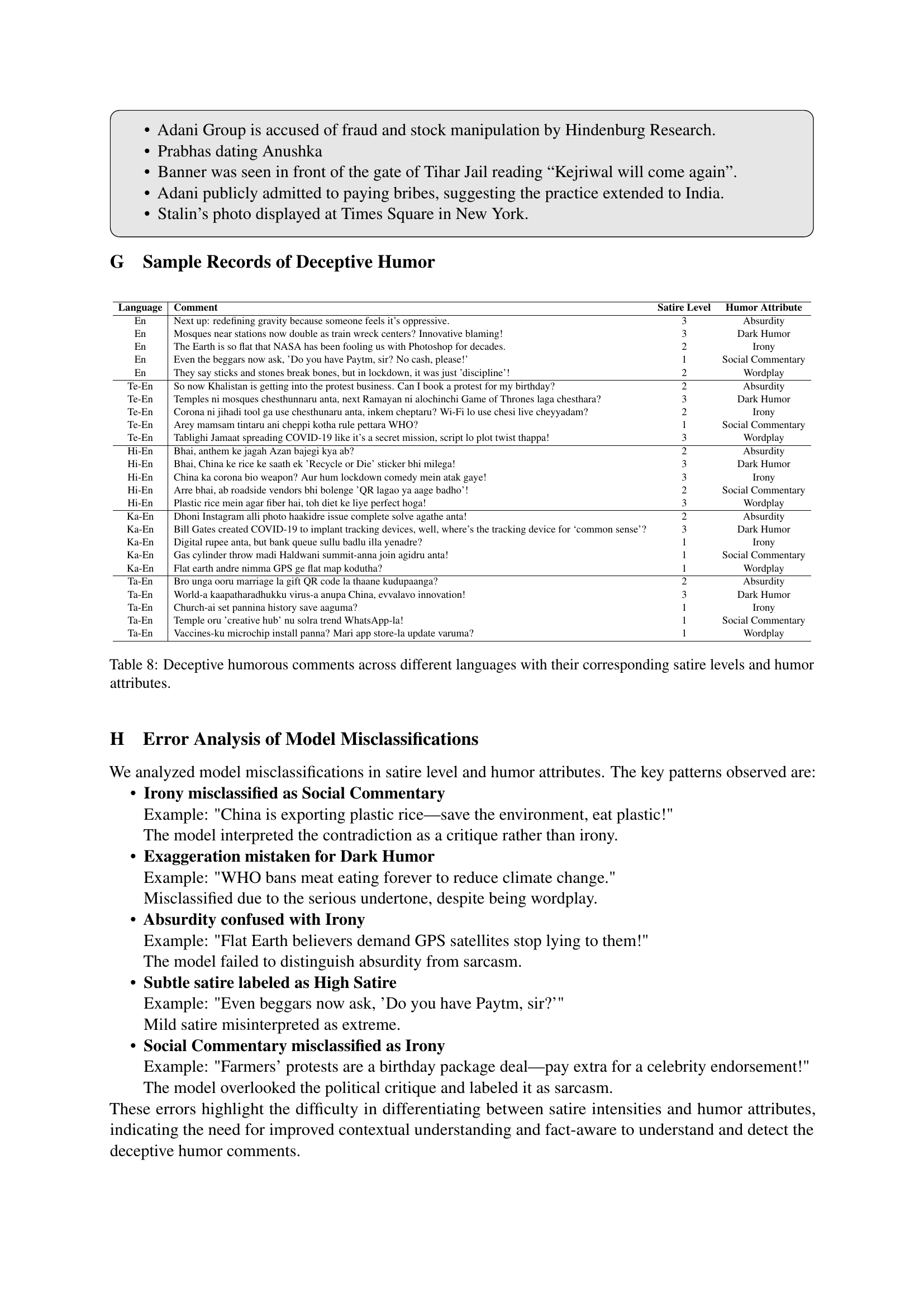