TL;DR#
Key Takeaways#
Why does it matter?#
AlphaSpace offers a new path for 3D spatial reasoning in AI, moving away from reliance on complex vision models. It is relevant to robotics, offering a lighter, adaptable approach. This approach encourages exploration of hybrid models and real-world applications. Future work in real-world deployment is promising.
Visual Insights#
🔼 This figure shows a simple robotic manipulation task. A black cube is to be placed on top of a green cube. This illustrates the type of 3D spatial reasoning and manipulation tasks that the AlphaSpace methodology is designed to solve. The figure serves as a visual representation of the core problem addressed in the paper.
read the caption
Figure 1: Put black cube onto green cube
Model | Picking | Stacking | Total (%) |
---|---|---|---|
AlphaSpace (Ours) | 10/12 | 6/12 | 66.67% |
GPT-4o | 6/12 | 3/12 | 37.5% |
Claude 3.5 Sonnet | 5/12 | 2/12 | 29.17% |
🔼 This table presents a comparison of the performance of three different models—AlphaSpace (the proposed model), GPT-40, and Claude 3.5 Sonnet—on the EmbodiedBench Manipulation Subtask. The subtask evaluates the models’ abilities to perform object manipulation tasks, specifically picking and stacking objects. The table shows the number of successes out of 12 trials for each task (picking and stacking) and the overall success rate (total accuracy) for each model.
read the caption
Table 1: Evaluation Results on EmbodiedBench Manipulation Subtask
Full paper#
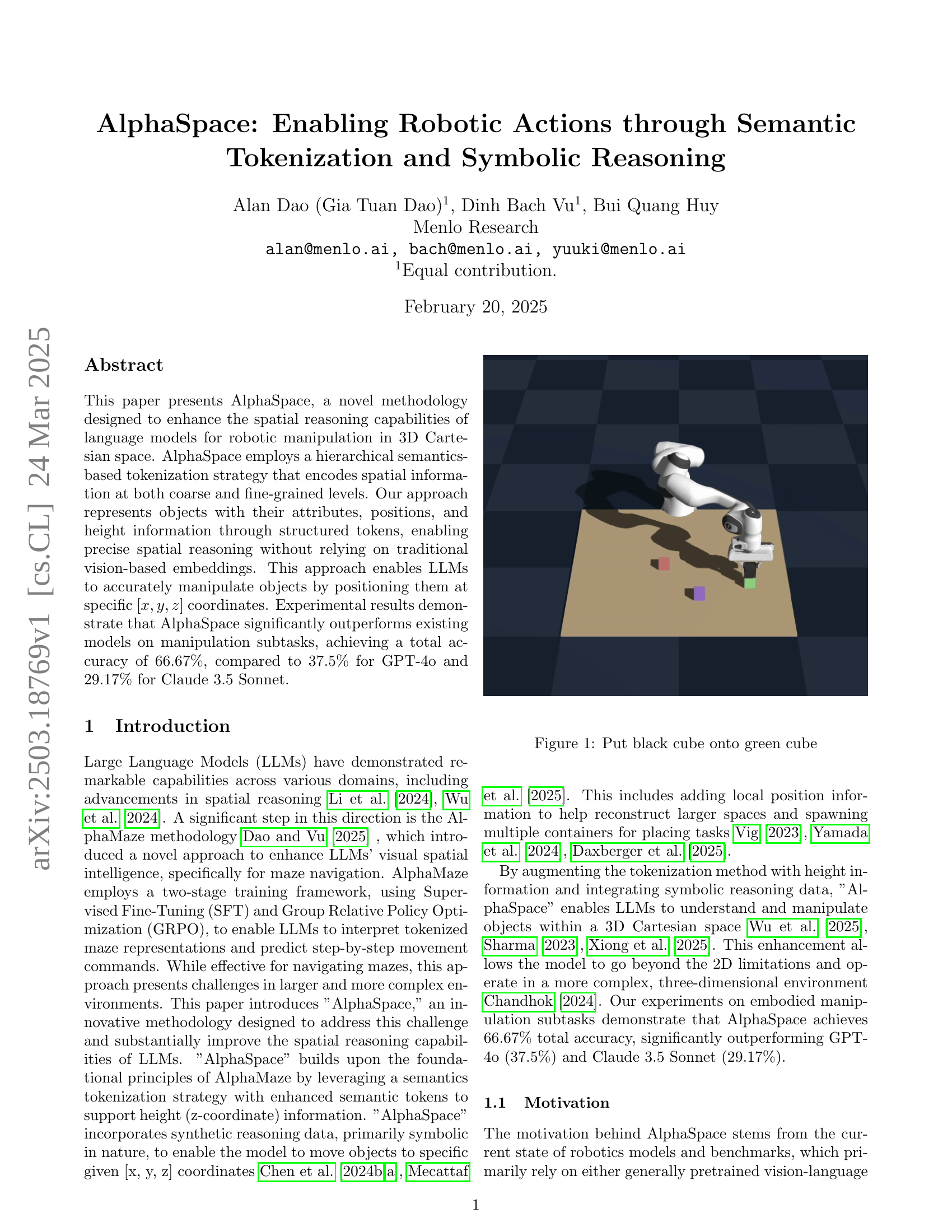
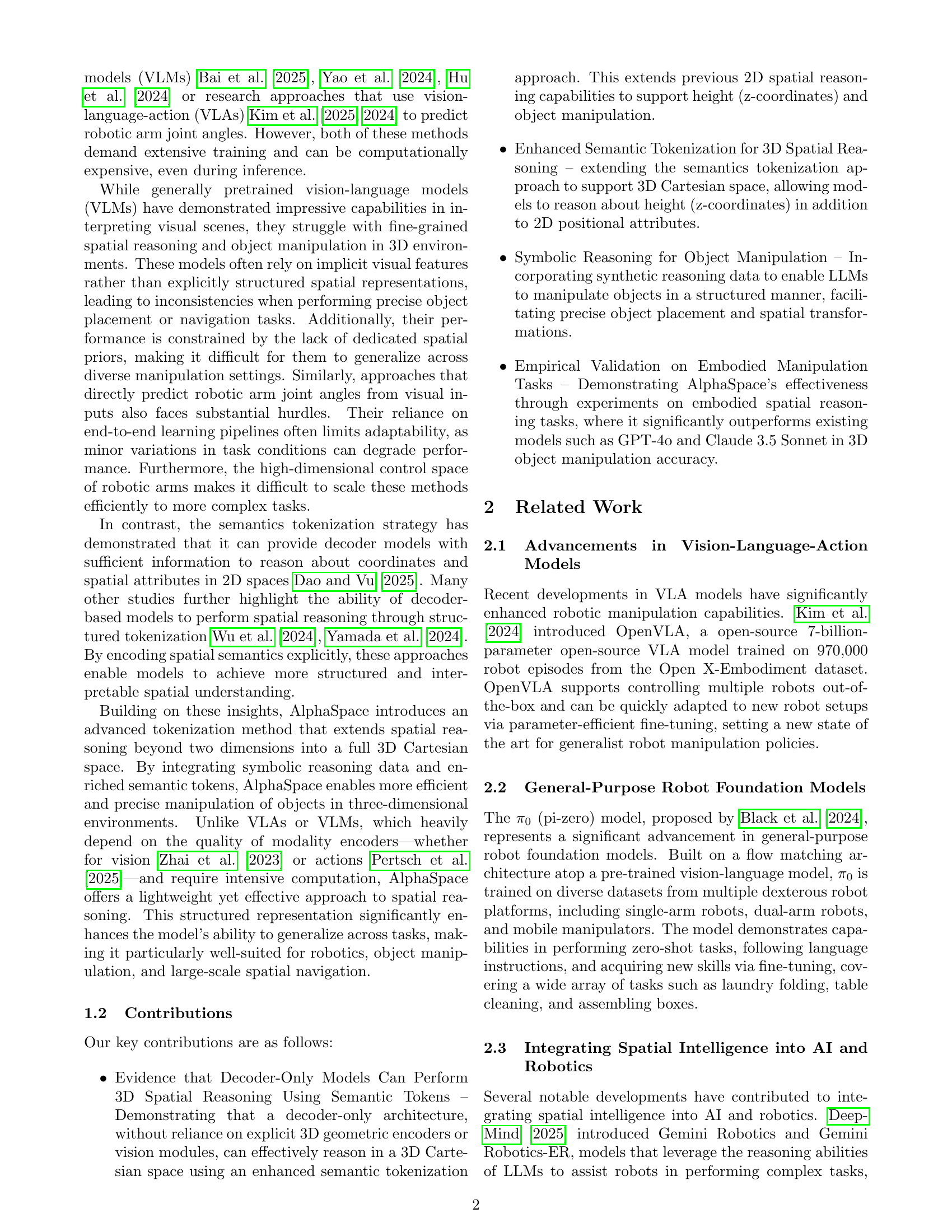
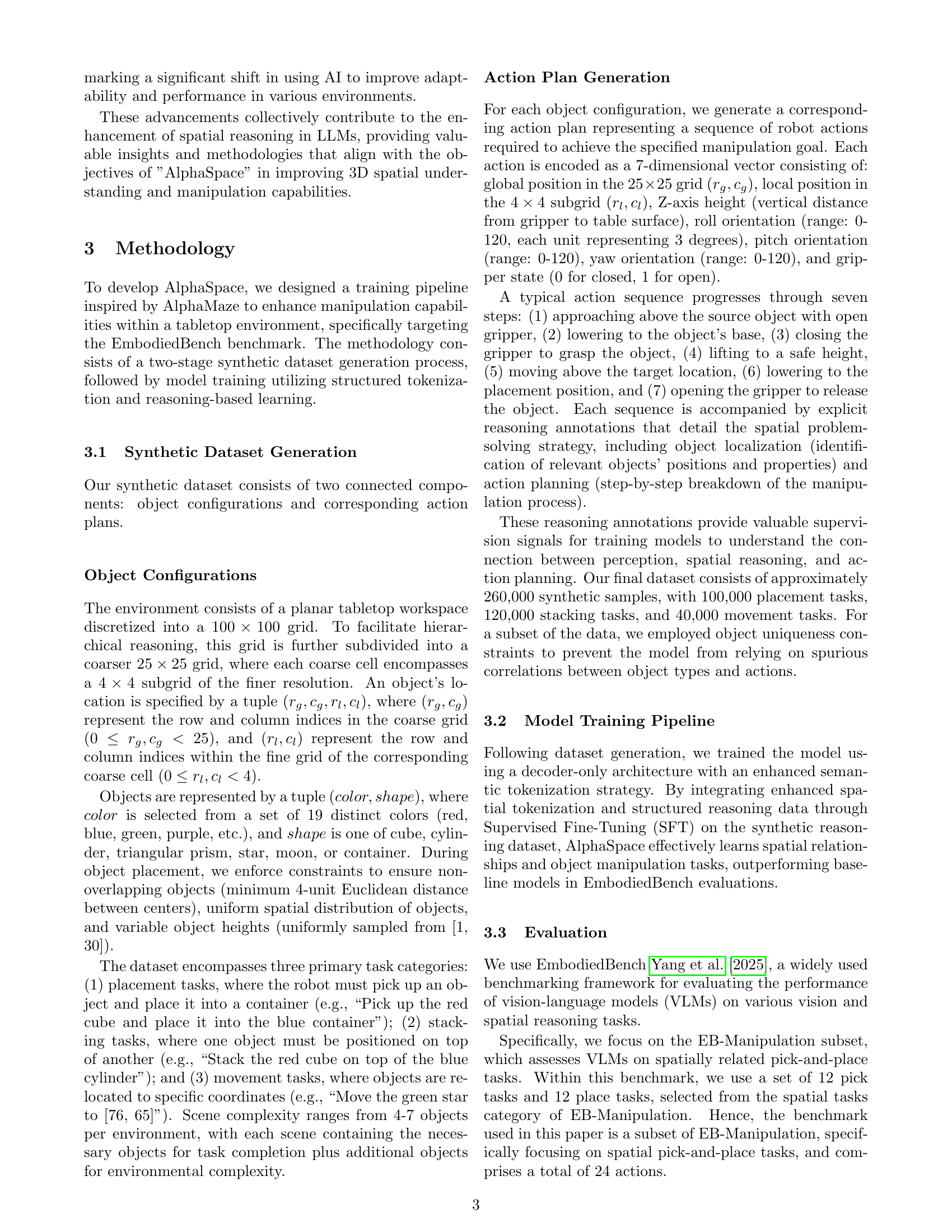
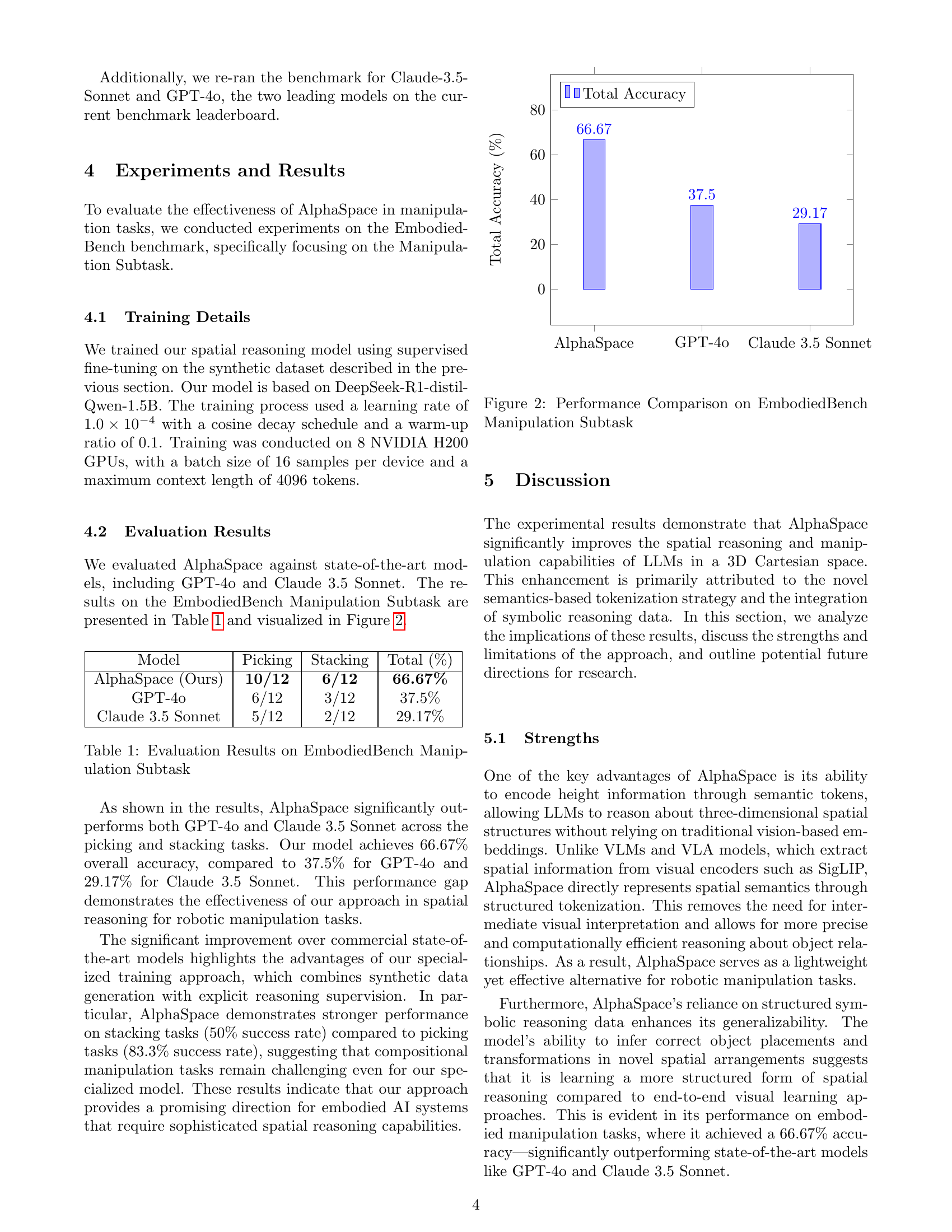
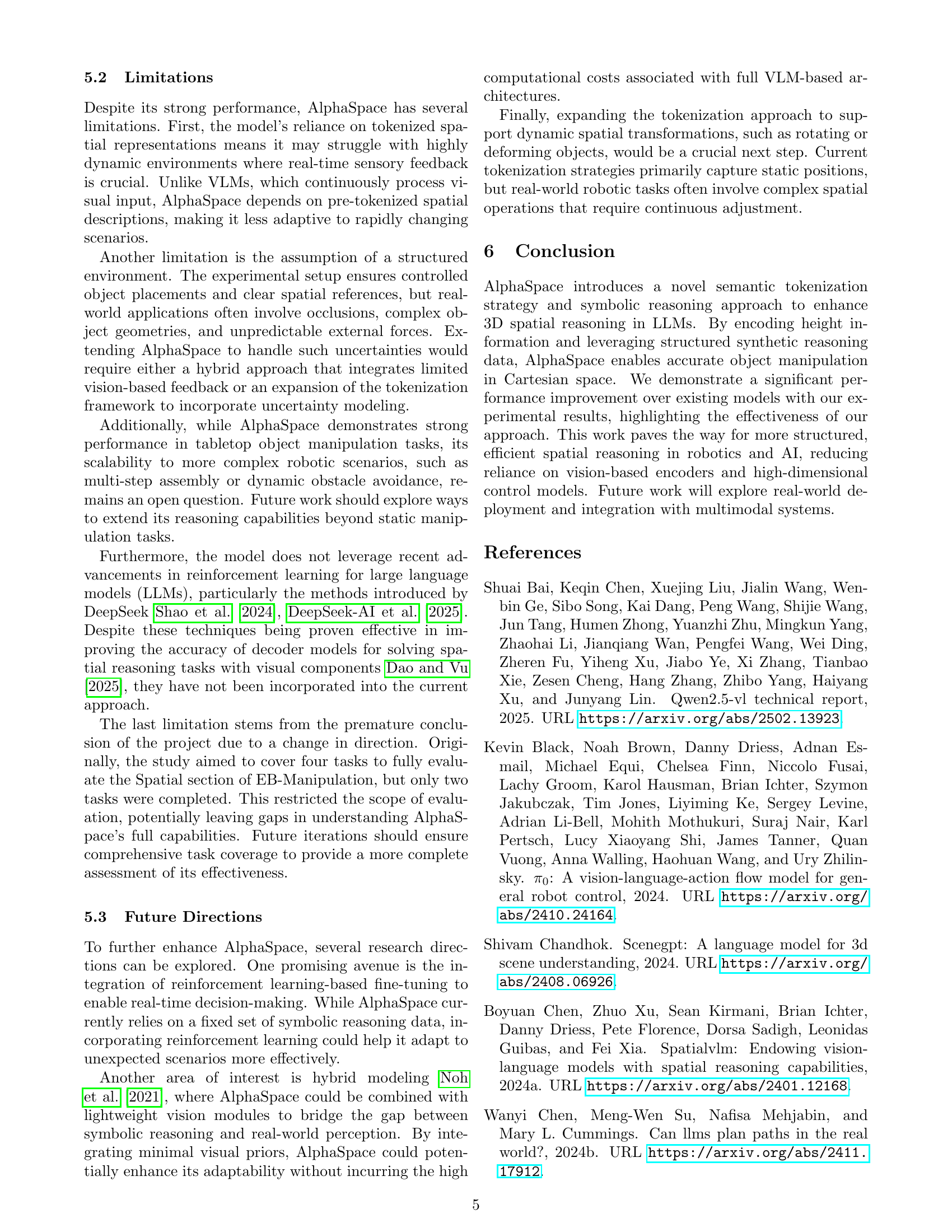
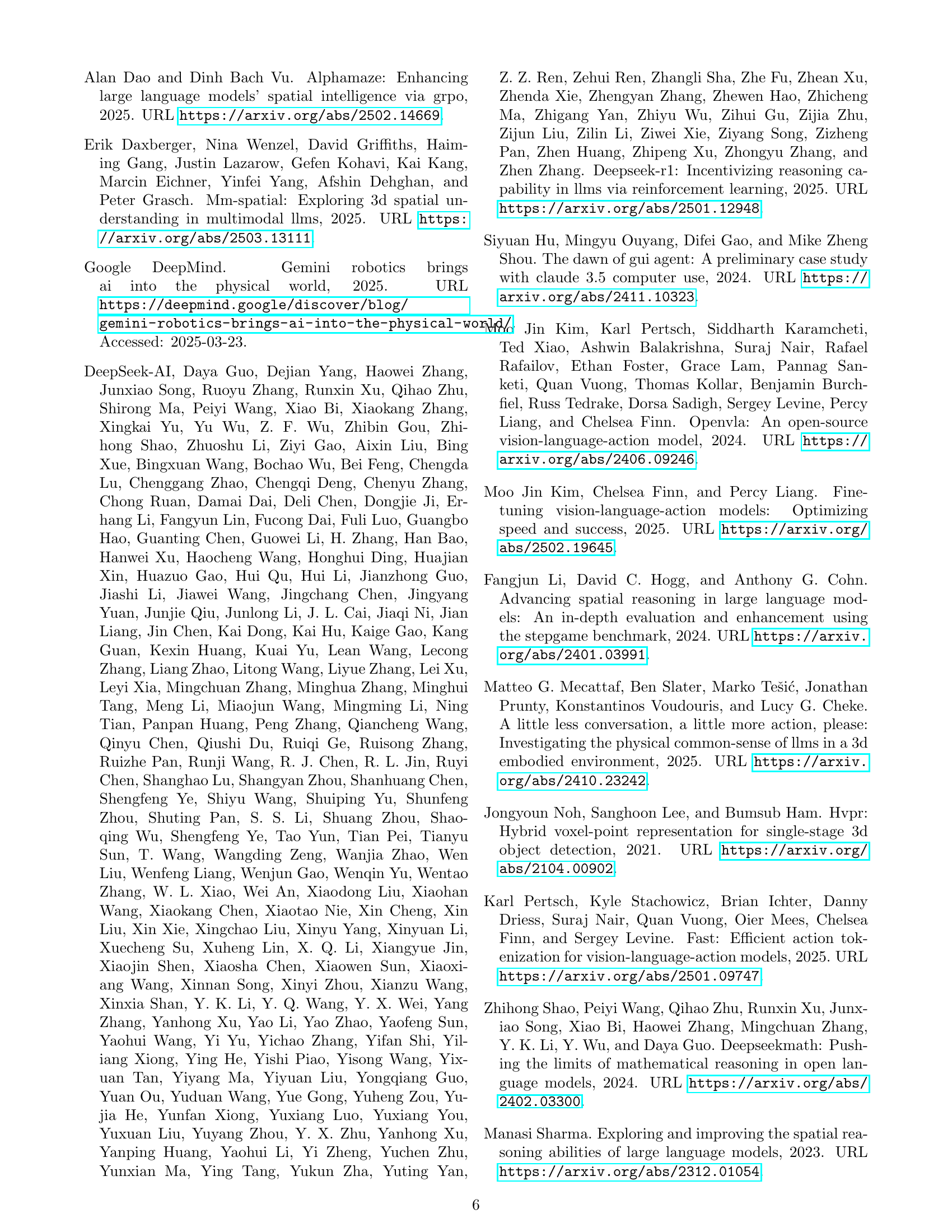
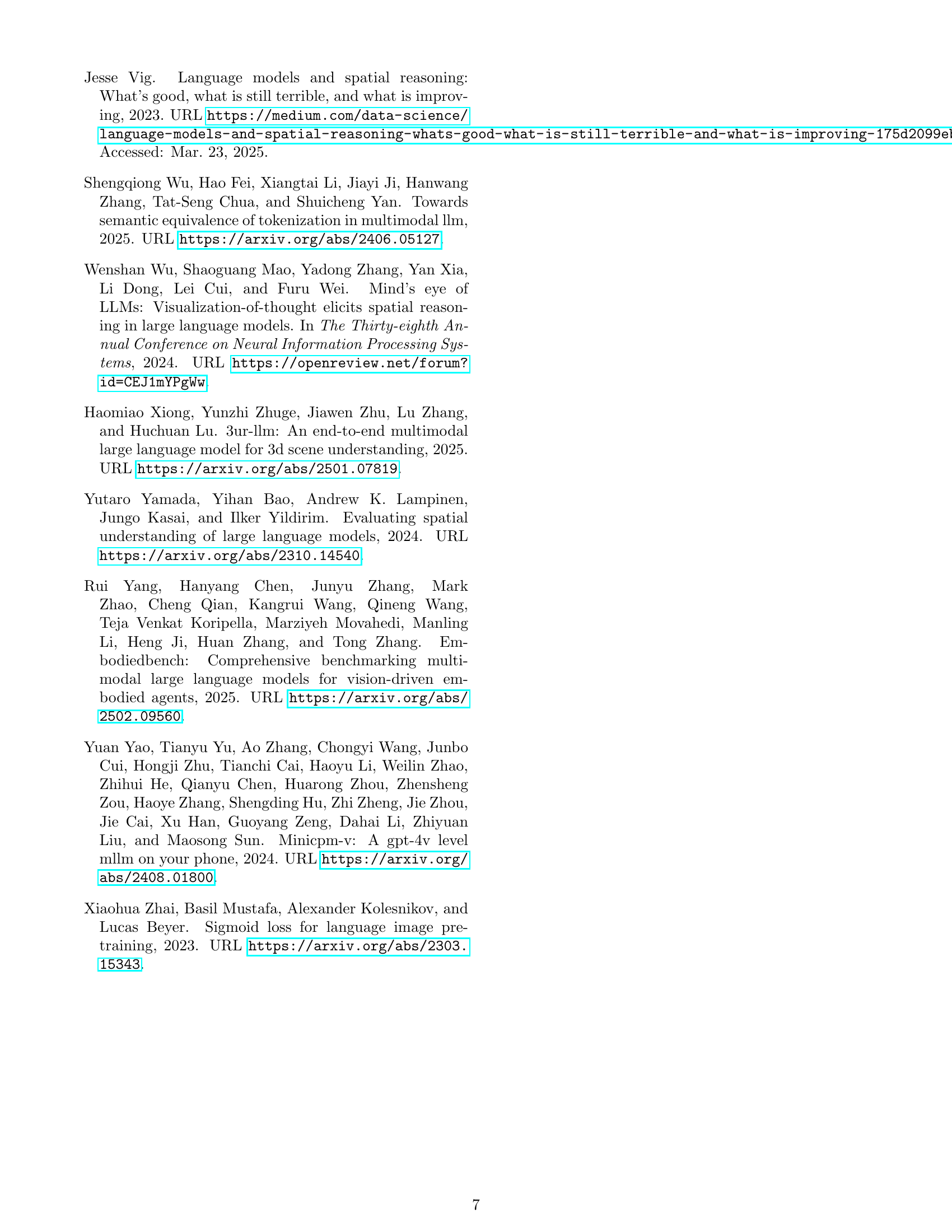