↗ OpenReview ↗ NeurIPS Homepage ↗ Chat
TL;DR#
Current single-particle cryo-electron microscopy (cryo-EM) struggles with reconstructing high-resolution 3D protein structures due to limited high-quality annotated training datasets. This necessitates extensive and laborious manual data annotation. The lack of sufficient data hinders the performance of crucial steps in cryo-EM data analysis such as particle picking and pose estimation.
CryoGEM, a novel physics-informed generative model, addresses this challenge by integrating physics-based cryo-EM simulation with generative unpaired noise translation. It leverages contrastive learning with a mask-guided sampling scheme to generate physically correct synthetic cryo-EM datasets with realistic noise. Extensive experiments demonstrate that CryoGEM generates authentic cryo-EM images and significantly improves the performance of existing deep models in downstream tasks, enhancing both particle picking and pose estimation. The synthetic dataset produced by CryoGEM serves as valuable training data, overcoming the limitations of real datasets.
Key Takeaways#
Why does it matter?#
This paper is crucial for researchers in cryo-EM and related fields. It presents CryoGEM, a novel physics-informed generative model that produces high-quality synthetic cryo-EM datasets. This significantly improves the accuracy of downstream tasks like particle picking and pose estimation, leading to better 3D reconstruction of proteins. This advance addresses a key limitation in cryo-EM—the lack of high-quality annotated data—and opens new avenues for research in biomolecular imaging.
Visual Insights#
The figure illustrates the cryo-EM data analysis pipeline. It shows how cryo-EM captures images of molecules in ice using electron beams. These images then undergo a multi-step processing pipeline for 3D reconstruction. CryoGEM is positioned within this pipeline to address the lack of high-quality training data for particle picking and ab-initio 3D reconstruction. CryoGEM augments the training data by synthesizing authentic single-particle micrographs from a coarse input.
This table presents a quantitative comparison of the visual quality of images generated by CryoGEM and various baselines using the Frechet Inception Distance (FID) metric. Lower FID scores indicate higher visual quality. The table shows that CryoGEM consistently outperforms the other methods across all five datasets, demonstrating its superior ability to generate realistic and high-quality cryo-EM images.
In-depth insights#
Cryo-EM Physics#
Cryo-electron microscopy (cryo-EM) is a technique used to determine the 3D structures of macromolecules, such as proteins. Cryo-EM physics underpins the entire process, from sample preparation to image analysis. It involves understanding the interaction of the electron beam with the frozen hydrated sample, including scattering and phase shifts. Ice thickness and crystalline structures within the sample significantly impact image quality, introducing noise and artifacts that must be addressed during image processing. Furthermore, the electron optics and detector technology influence the acquired images’ resolution and signal-to-noise ratio. Modeling these physical phenomena accurately is crucial for generating realistic synthetic cryo-EM data, as demonstrated in the CryoGEM model, improving the reliability and accuracy of downstream tasks such as particle picking and 3D reconstruction. Accurate modeling of electron scattering, ice effects, and detector noise is thus a cornerstone for advancing the field of cryo-EM and reaching higher resolutions.
Generative Model#
Generative models, particularly deep learning-based ones, are revolutionizing various fields by learning complex data distributions and generating new samples that resemble the training data. In the context of cryo-electron microscopy (cryo-EM), generative models offer significant potential for augmenting existing datasets. Data scarcity is a major hurdle in cryo-EM, limiting the performance of downstream tasks like particle picking and 3D reconstruction. Generative models can create synthetic cryo-EM images, effectively increasing the size and diversity of training data. Physics-informed generative models, which incorporate physical principles of cryo-EM image formation into the generative process, are particularly promising. They can generate more realistic and physically accurate images, improving the training of downstream models and ultimately enhancing the quality and resolution of cryo-EM reconstructions. However, challenges remain, including the need for carefully designed loss functions that appropriately balance realism, accuracy, and diversity in the generated data, and the need to address the computational cost of training such complex models.
Noise Translation#
The concept of ‘Noise Translation’ in the context of cryo-electron microscopy (cryo-EM) image generation is crucial for creating realistic synthetic datasets. Real cryo-EM images contain complex noise patterns arising from various sources, including detector limitations, specimen-electron interactions, and ice gradients. Simple noise models like Gaussian noise fail to capture this complexity. Therefore, a method that translates simulated, noise-free cryo-EM images into realistic noisy counterparts is needed. This translation can leverage various techniques such as Generative Adversarial Networks (GANs) or diffusion models, possibly in an unpaired image-to-image translation setting, meaning that the model learns the noise mapping without explicit paired training data (real and simulated images). A key challenge is designing a translation model capable of accurately capturing the statistical properties of real cryo-EM noise while maintaining the fidelity of the underlying structural information. Methods may employ contrastive learning, as suggested, focusing on differentiating particle signals from background noise to guide the noise translation, making the generated images more authentic. The success of noise translation directly impacts the downstream tasks such as particle picking and 3D reconstruction, as the quality of the synthetic data significantly affects the performance of deep learning models trained on it. In essence, a robust noise translation model is a cornerstone for physics-informed generative cryo-EM approaches.
Downstream Tasks#
In cryo-electron microscopy (cryo-EM), downstream tasks are crucial for achieving high-resolution 3D reconstructions of molecules. These tasks, such as particle picking and pose estimation, heavily rely on the quality of the input data. Poor quality data significantly hinders the performance of these downstream tasks, leading to inaccurate or low-resolution reconstructions. This research paper highlights the importance of using high-quality data to improve downstream tasks’ performance. The introduction of a physics-informed generative model, CryoGEM, which generates realistic cryo-EM datasets is a key innovation to address this challenge. By using CryoGEM to generate synthetic data, this study shows a significant improvement in the accuracy of particle picking and pose estimation, ultimately leading to better resolution in the final 3D reconstruction. The ability to generate controlled, high-quality synthetic data is therefore paramount for enhancing the overall efficacy and reliability of cryo-EM.
Future CryoEM#
Future CryoEM holds enormous potential for revolutionizing structural biology. Advances in hardware, such as improved detectors and electron sources, promise higher resolution images and faster data acquisition. Sophisticated image processing algorithms, leveraging machine learning and AI, will be essential to analyze the increasingly complex datasets generated by these advanced instruments. Integrating diverse imaging modalities, like CryoEM with X-ray crystallography or NMR, is crucial for a comprehensive understanding of macromolecular structures and dynamics. The development of novel sample preparation techniques to overcome current limitations in sample heterogeneity and stability will be a critical area of advancement. Finally, physics-informed generative models offer promising avenues for creating high-quality training data to improve various cryo-EM analysis steps, ultimately leading to improved model accuracy and accelerating the overall process.
More visual insights#
More on figures
This figure illustrates the pipeline of the CryoGEM method. It starts with creating a virtual specimen using initial reconstruction results, simulates the cryo-EM imaging process with physical priors, adds Gaussian noise for randomness, uses a particle-background mask for guided patch sampling in contrastive learning, and finally uses an adversarial loss to ensure realistic image synthesis. The figure details each step of the process, highlighting the key components and techniques used.
This figure compares the similarity maps generated by the proposed method’s encoder and CUT’s encoder. The input is a real cryo-EM micrograph with query patches (particle and background). The proposed method successfully distinguishes particle and background regions, shown in separate similarity maps, highlighting the effectiveness of the proposed mask-guided sampling scheme. In contrast, CUT fails to learn this distinction without the mask guidance.
This figure showcases the diverse synthetic cryo-EM images generated by CryoGEM. The top row displays examples from various proteins, demonstrating the model’s ability to generate realistic images of different molecules. The following rows illustrate CryoGEM’s control over particle orientation, conformation, and defocus, showing how it can generate images with variations in these parameters.
This figure presents a qualitative comparison of synthetic cryo-EM images generated by CryoGEM and several baseline methods across five different datasets. CryoGEM’s images exhibit more realistic noise patterns compared to baselines, which either fail to capture authentic noise or introduce visual artifacts.
This figure compares the particle picking results of different methods (Topaz, Ours, Poi-Gau, CycleGAN, CUT, and CycleDiffusion) on three different datasets (Ribosome, Integrin, and PhageMS2). Blue circles highlight correctly identified particles, while red circles indicate false positives (incorrectly identified particles) or false negatives (missed particles). The visualization shows that the ‘Ours’ method (CryoGEM) significantly outperforms the baselines in terms of accurately identifying particles.
This figure shows the results of 3D reconstruction using particles picked by CryoGEM and other methods. It compares the initial low-resolution input structures to the final, refined structures achieved after using the CryoGEM particle picking model. The Fourier Shell Correlation (FSC) curves are also displayed to quantitatively assess the resolution achieved by each method. The figure demonstrates that using particles selected by CryoGEM leads to higher resolution reconstructions compared to the baselines.
This figure shows three experiments of cryoGEM with different control experiments. The first experiment shows that cryoGEM can precisely control the position of particles in the micrographs. The second experiment shows that cryoGEM can generate realistic ice gradients in micrographs by calculating the ice gradients in real micrographs. The third experiment shows that cryoGEM can generate convincing results on unseen datasets without retraining. This demonstrates the zero-shot transfer capability of cryoGEM.
This figure shows a qualitative comparison of CryoGEM’s generated images with real cryo-EM images from five different datasets. The red circles and arrows highlight specific features in both the real and generated images to demonstrate that CryoGEM can accurately reproduce noise patterns, preserve structural details, and replicate specific anomalies from the real images.
This figure shows the probability distribution of the number of particles present in cryo-EM micrographs for five different datasets: Proteasome, Ribosome, Integrin, PhageMS2, and HumanBAF. Each dataset’s distribution is represented by a separate curve, with the mean (µ) and standard deviation (σ) values indicated. The figure provides insights into the variability of particle counts across different datasets, highlighting that some datasets exhibit a wider range of particle counts than others. This variation in particle count is a characteristic feature of cryo-EM data that needs to be accounted for when developing methods for processing and analyzing cryo-EM micrographs.
The figure displays the probability distribution curves of the average defocus values for five different cryo-EM datasets: Proteasome, Ribosome, Integrin, PhageMS2, and HumanBAF. Each curve is a Gaussian distribution fitted to the data. The parameters (mean μ and standard deviation σ) of each Gaussian are also given in the legend.
This figure shows the results of 3D reconstruction using particles picked by CryoGEM and compares the results to other baselines. It visually presents the initial coarse input density maps and the final refined 3D structures obtained after using CryoGEM for particle picking, illustrating the improvement in resolution. Quantitative comparisons using Fourier Shell Correlation (FSC) curves are also provided to demonstrate the resolution achieved by each method.
This figure shows a qualitative comparison of CryoGEM’s generated images with real cryo-EM images across five different datasets. The results demonstrate CryoGEM’s ability to generate realistic cryo-EM micrographs that closely match the characteristics of real images, including noise patterns and structural details. Specific anomalies present in the real data, such as crystalline ice in the Integrin dataset, are accurately reproduced by CryoGEM. The image highlights these features using red circles and arrows.
More on tables
This table presents a quantitative comparison of the visual quality of images generated by CryoGEM and several baseline methods. The comparison uses the Fréchet Inception Distance (FID) metric, a lower FID score indicating higher visual quality. The results show that CryoGEM consistently outperforms the baseline methods across five different datasets, demonstrating its superior ability to generate high-quality, realistic cryo-EM images.
This table presents a quantitative comparison of the visual quality of images generated by CryoGEM and several baselines using the Frechet Inception Distance (FID) metric. Lower FID scores indicate better visual quality. The results show that CryoGEM consistently outperforms the baselines across five different datasets, demonstrating its superior ability to generate high-quality synthetic cryo-EM images.
This table presents a quantitative comparison of the visual quality of images generated by CryoGEM and several baseline methods. The FID (Fréchet Inception Distance) score is used as a metric to assess the similarity between generated and real cryo-EM images. Lower FID scores indicate higher visual quality. The table shows that CryoGEM consistently outperforms the baseline methods across five different datasets, demonstrating its superior ability to generate realistic and high-quality synthetic cryo-EM micrographs.
Full paper#
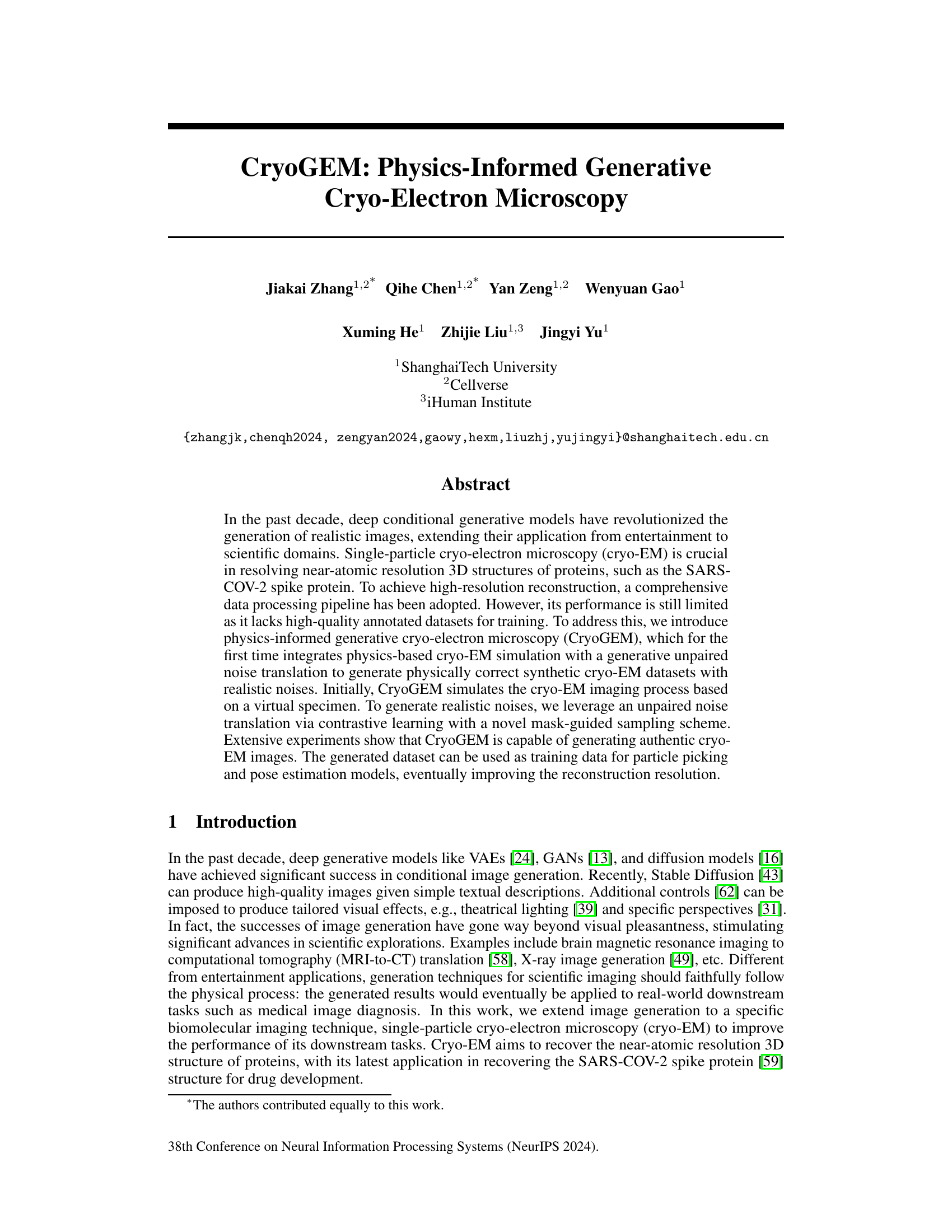
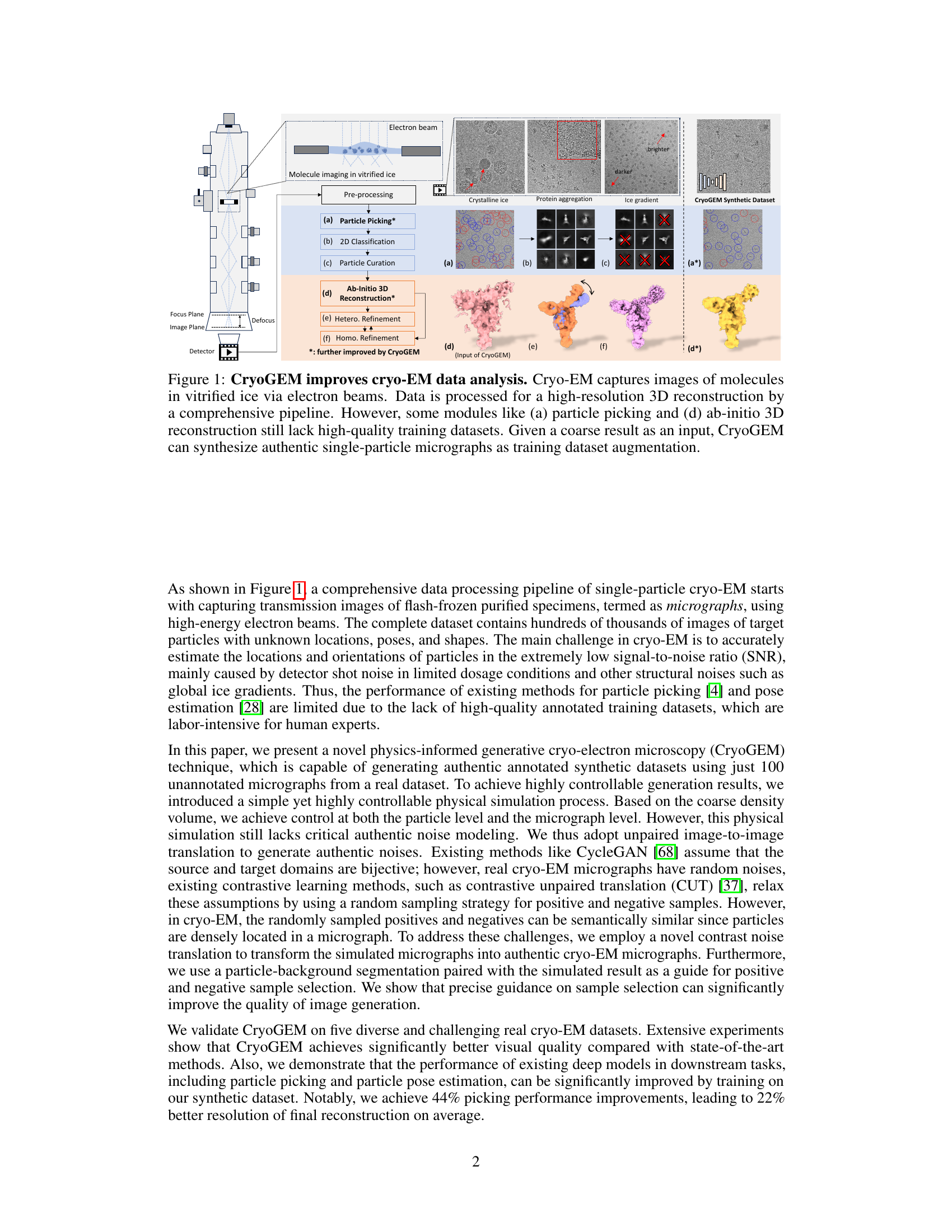
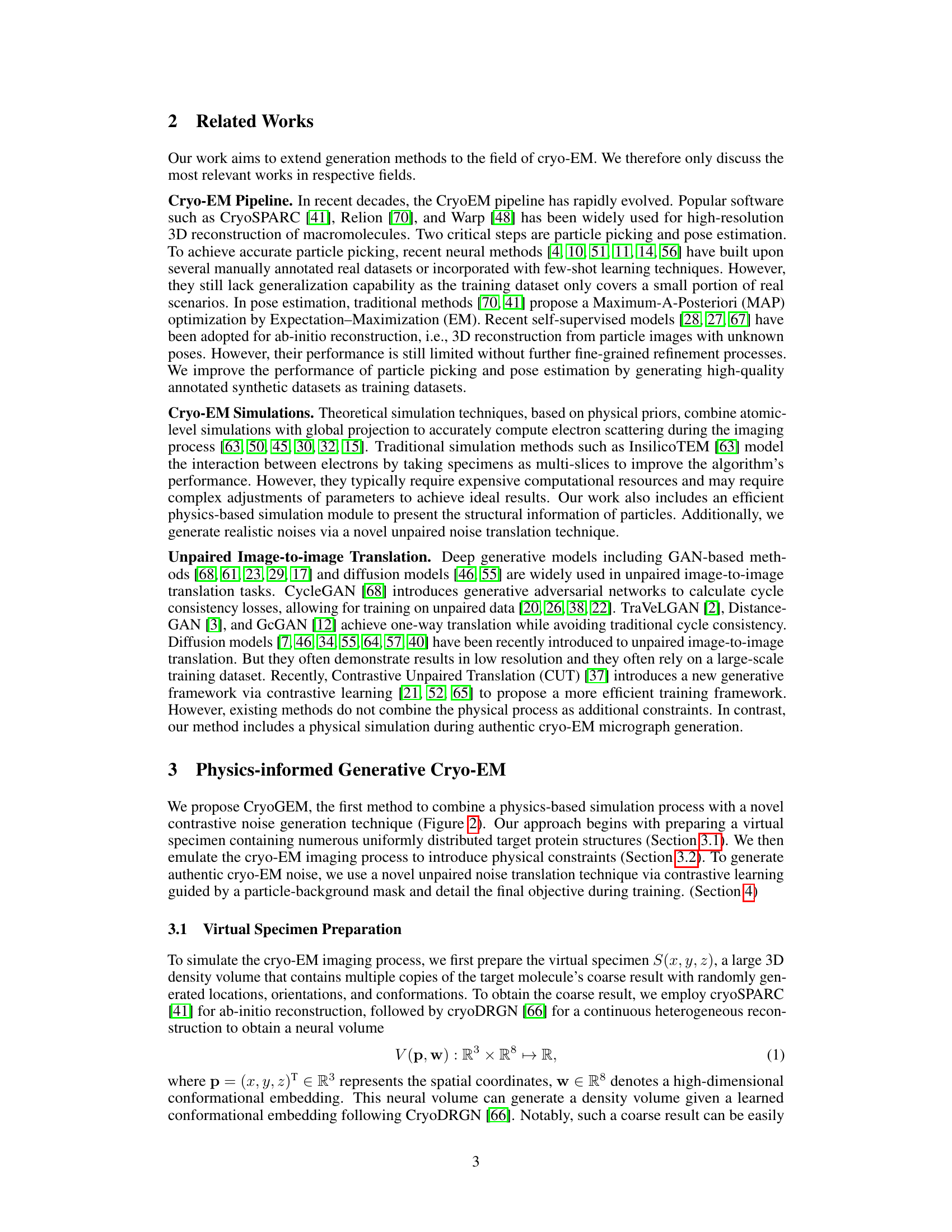
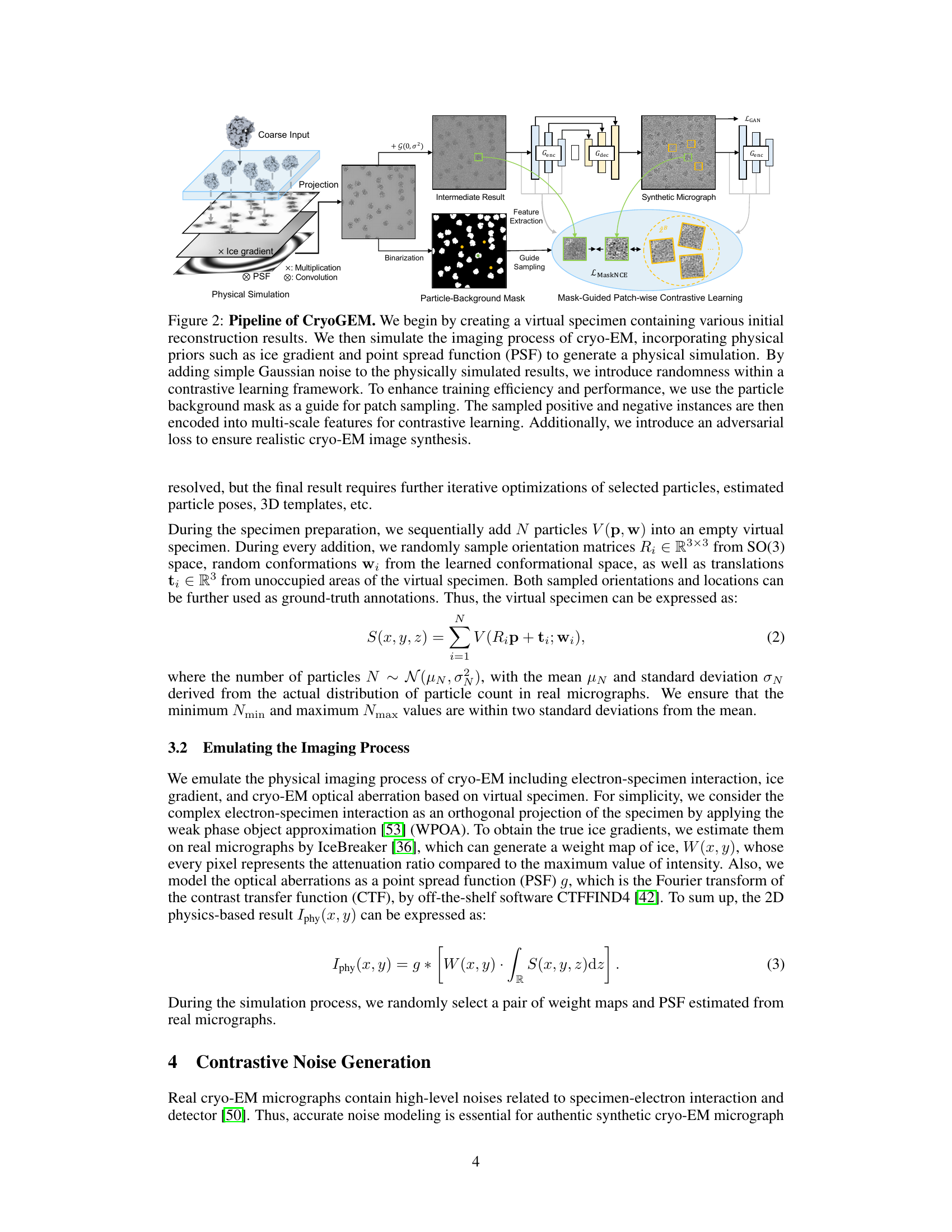
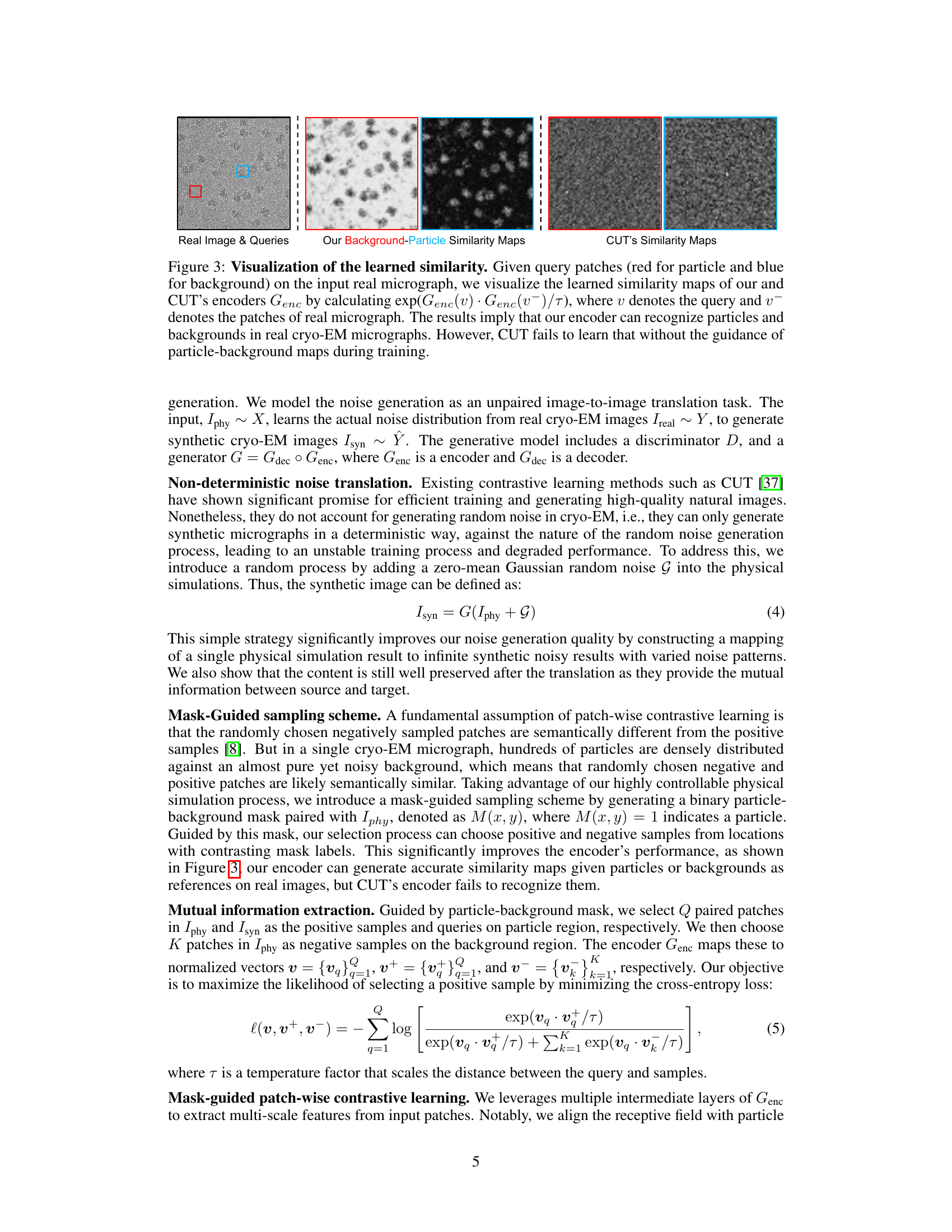
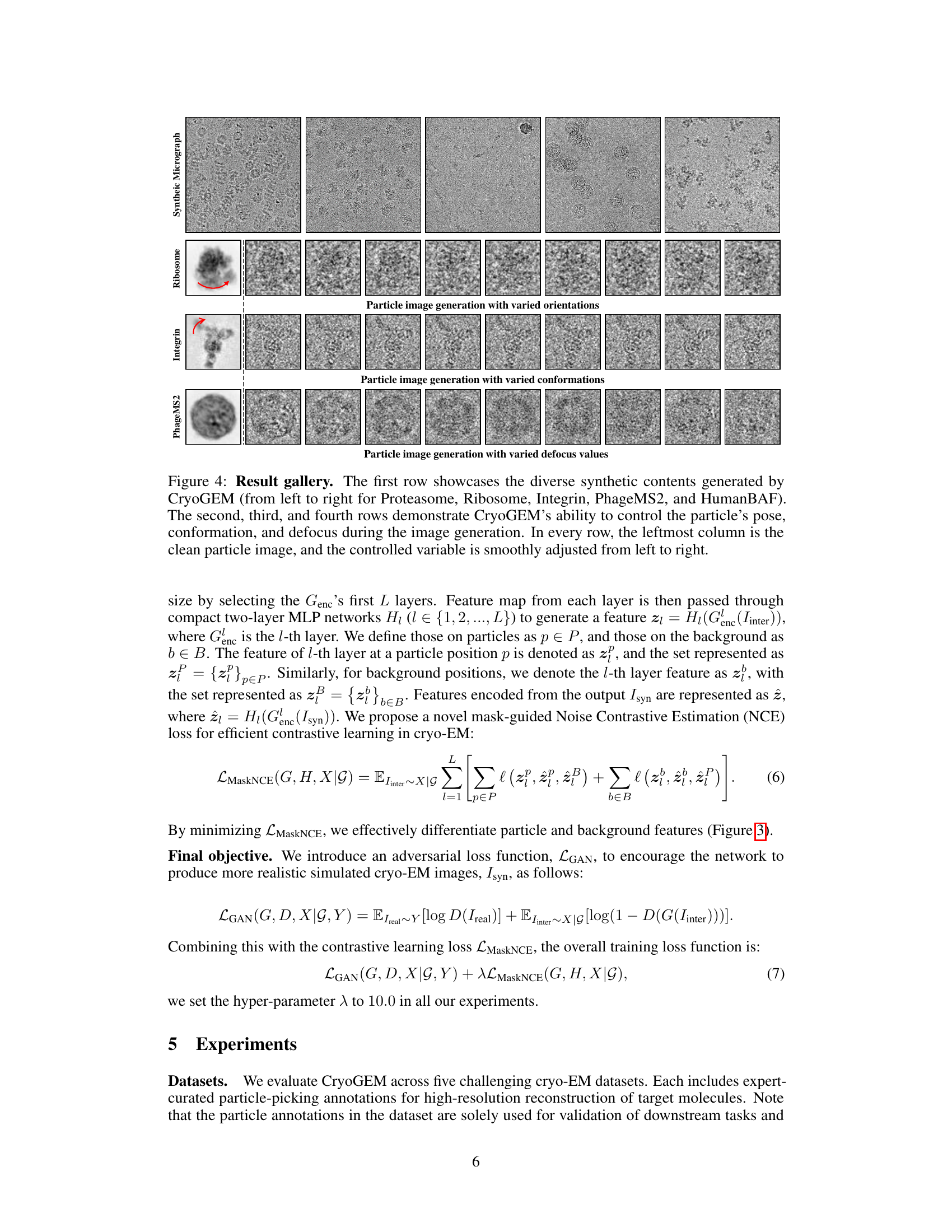
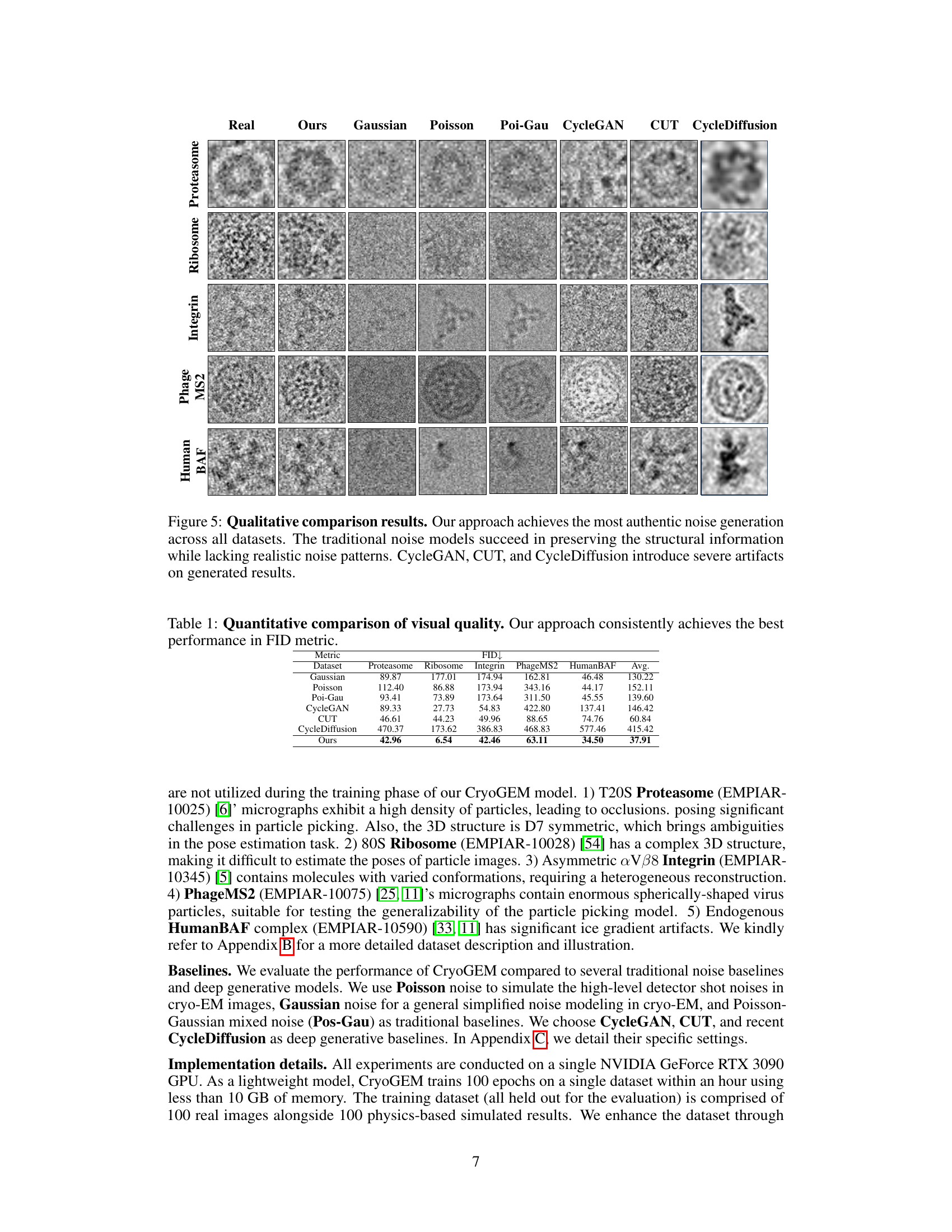
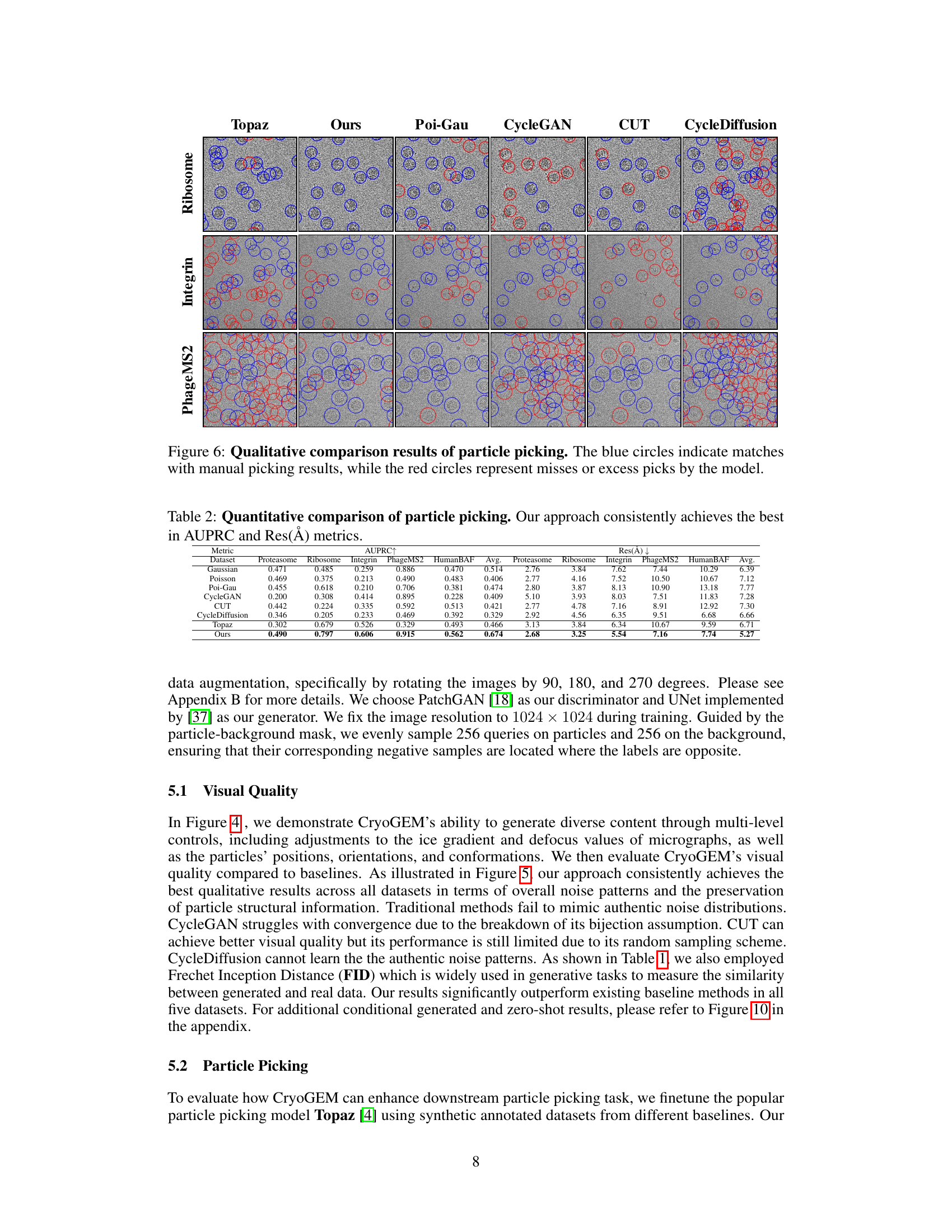
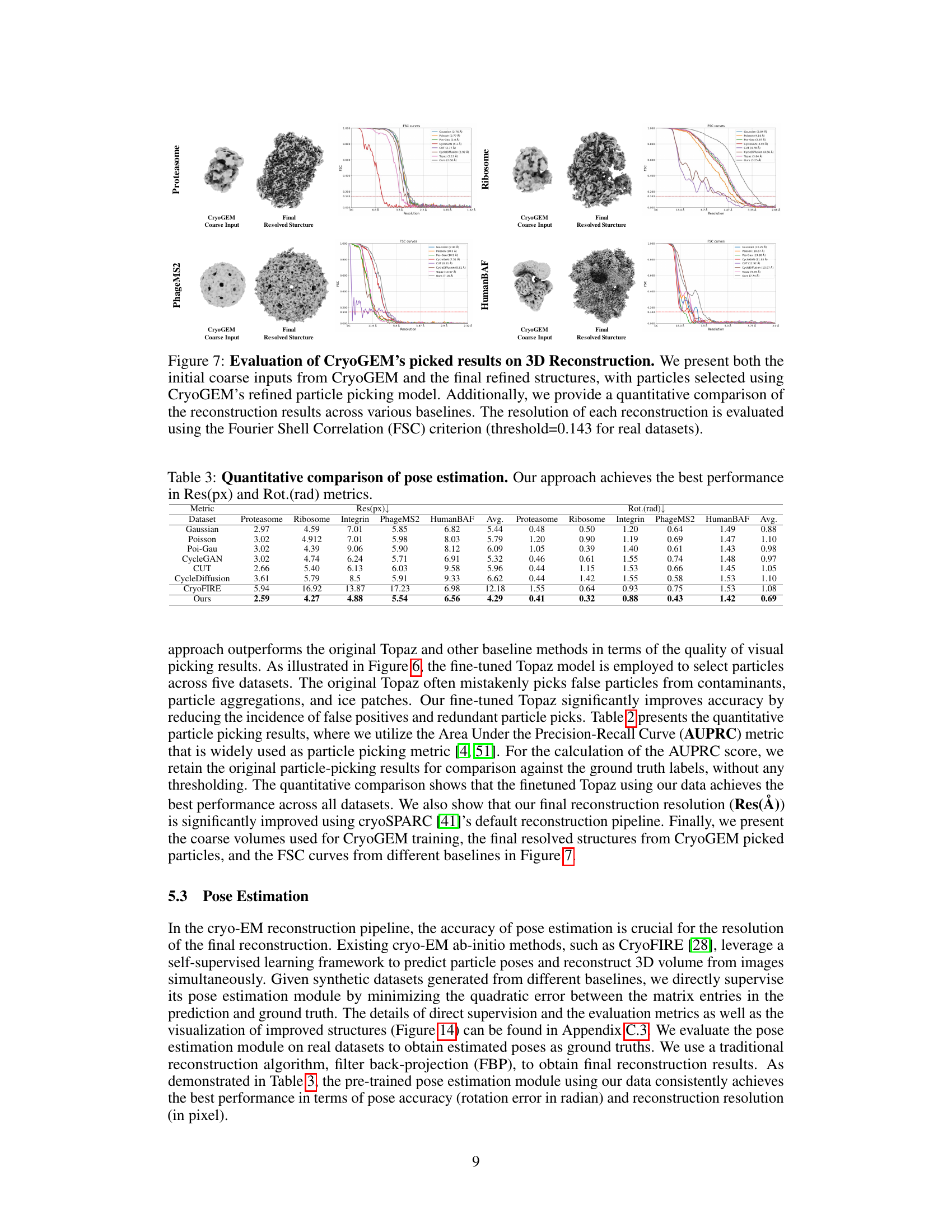
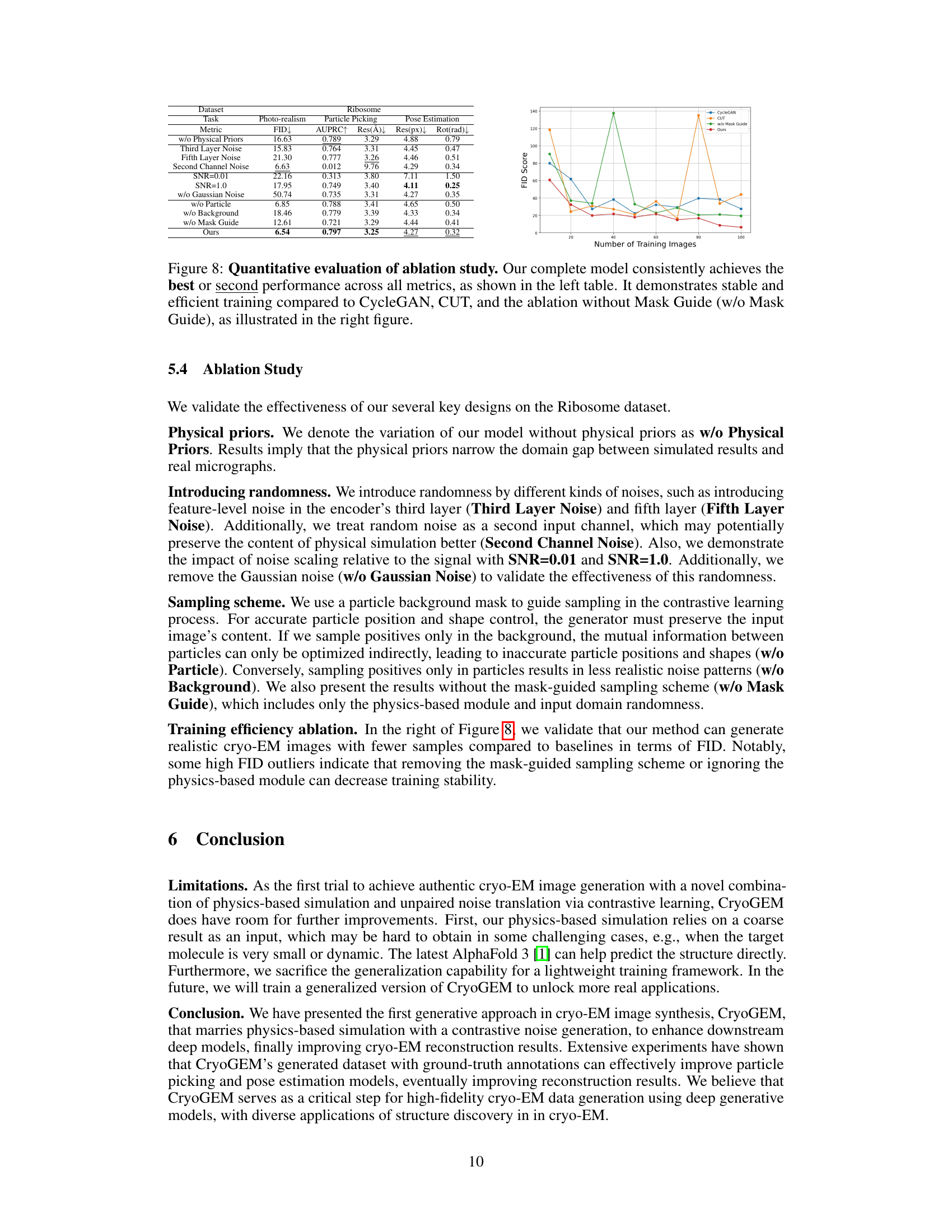
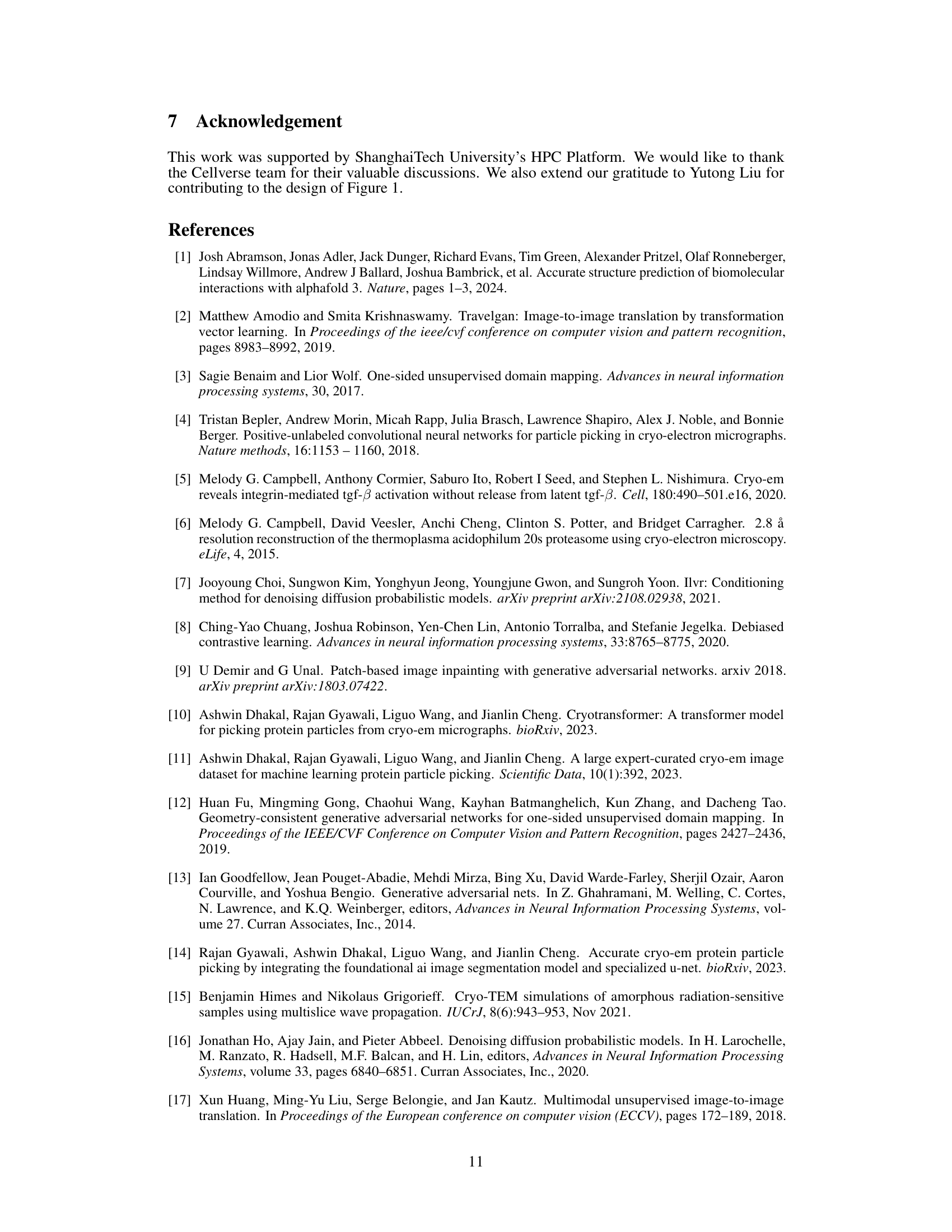
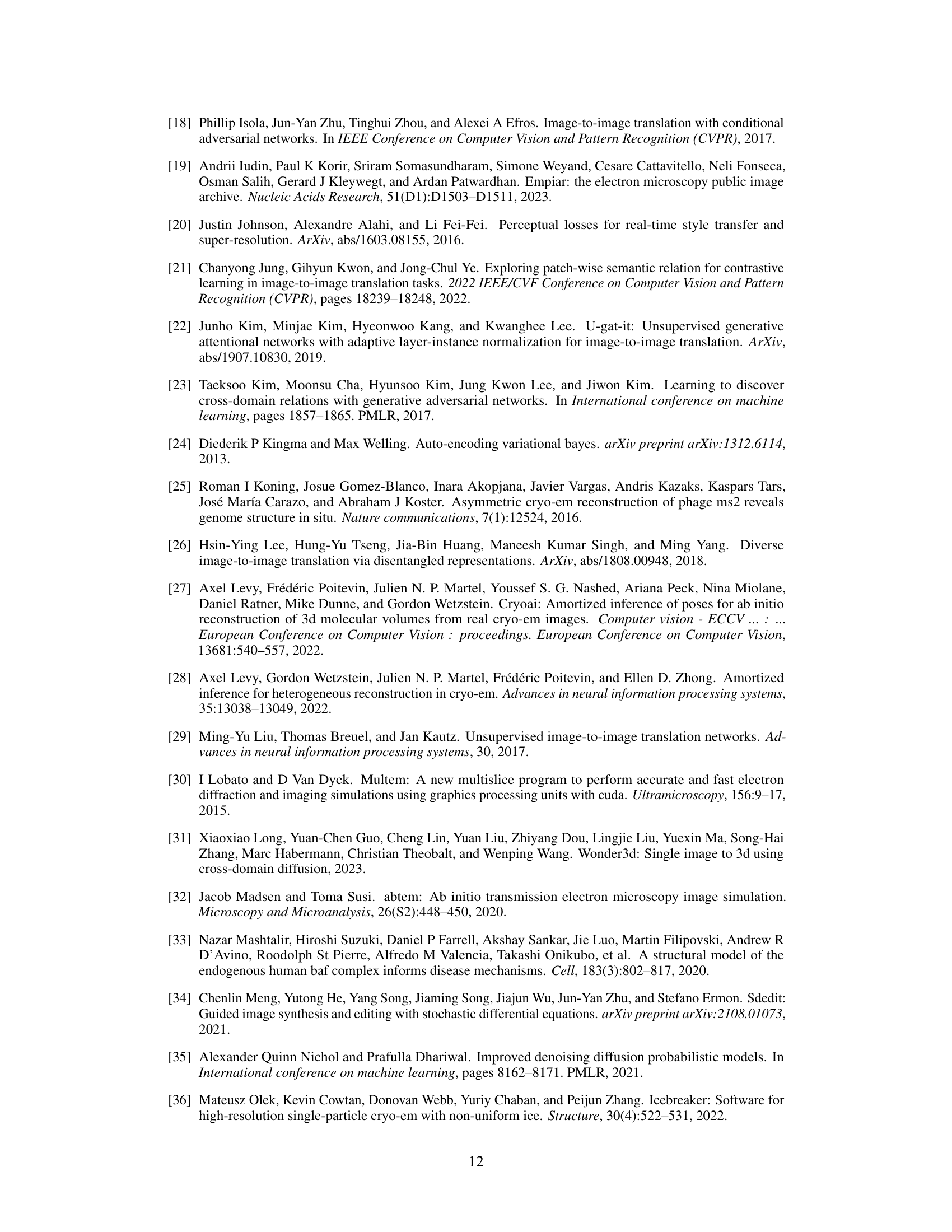
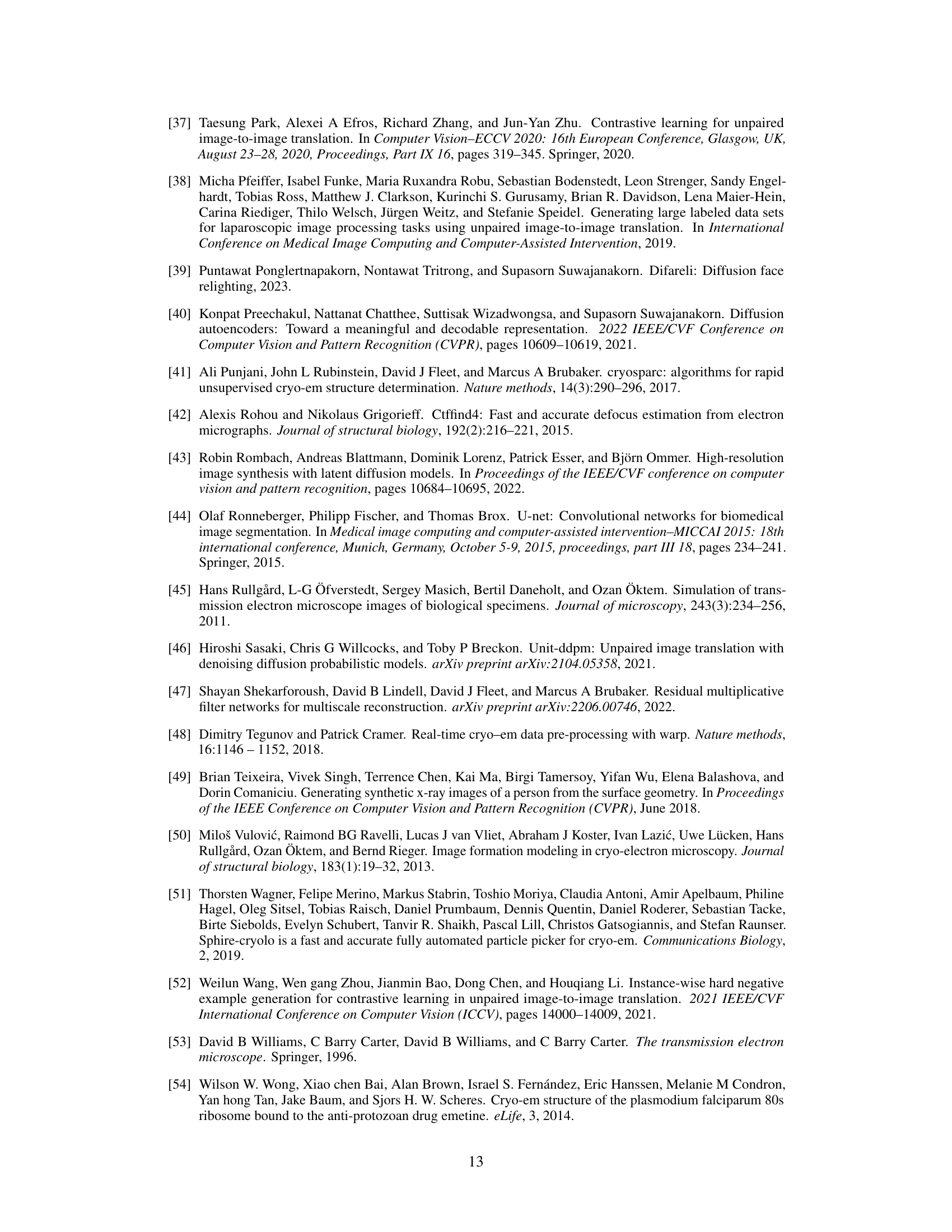
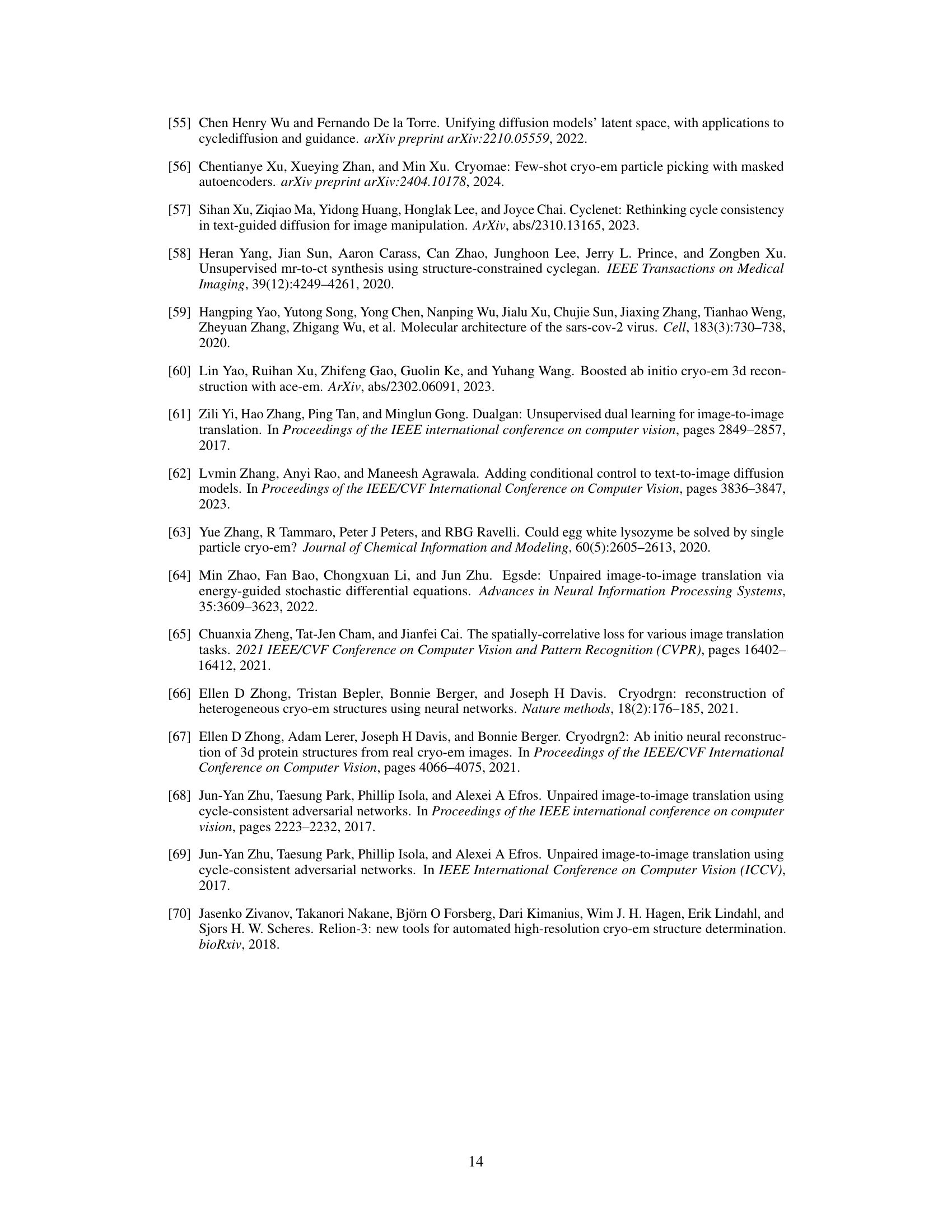
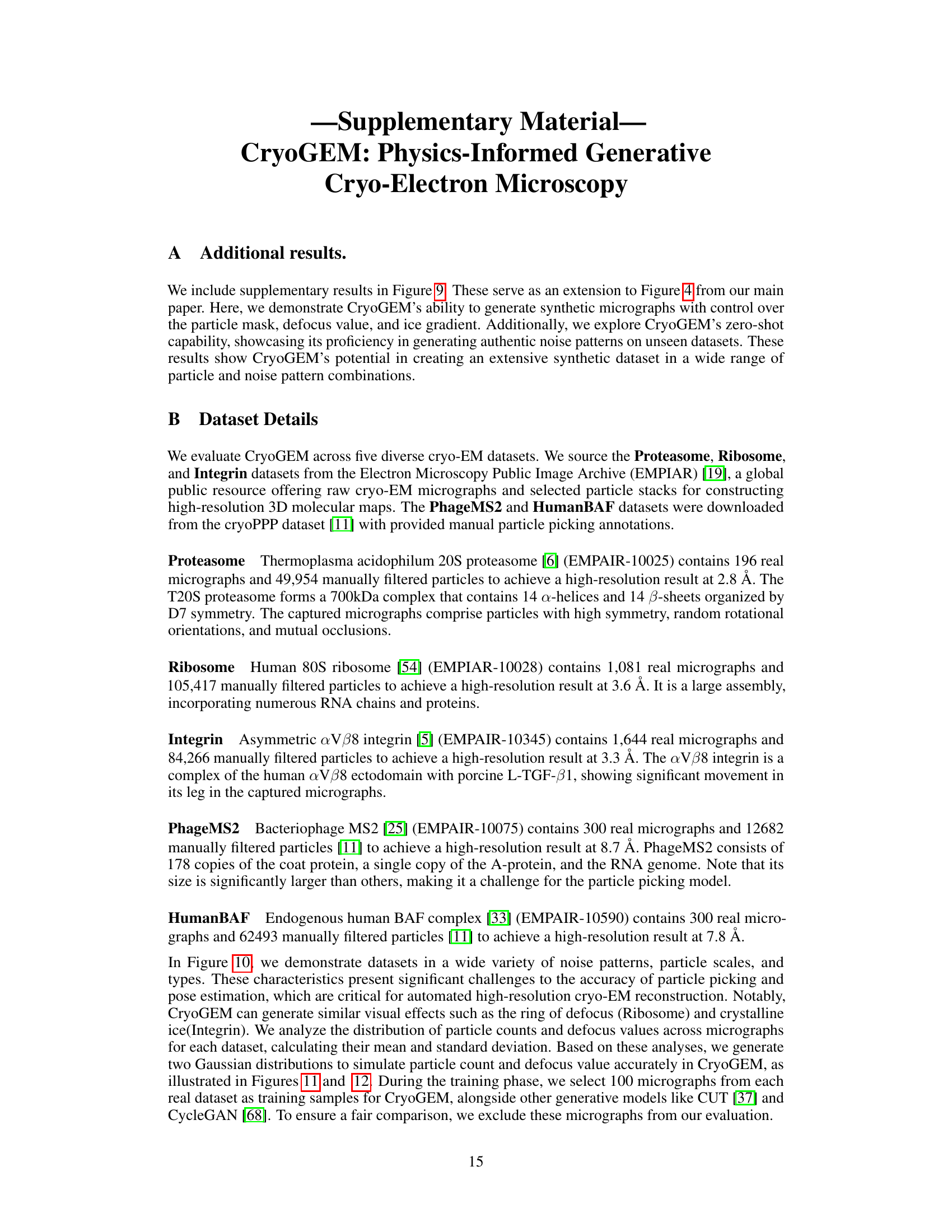
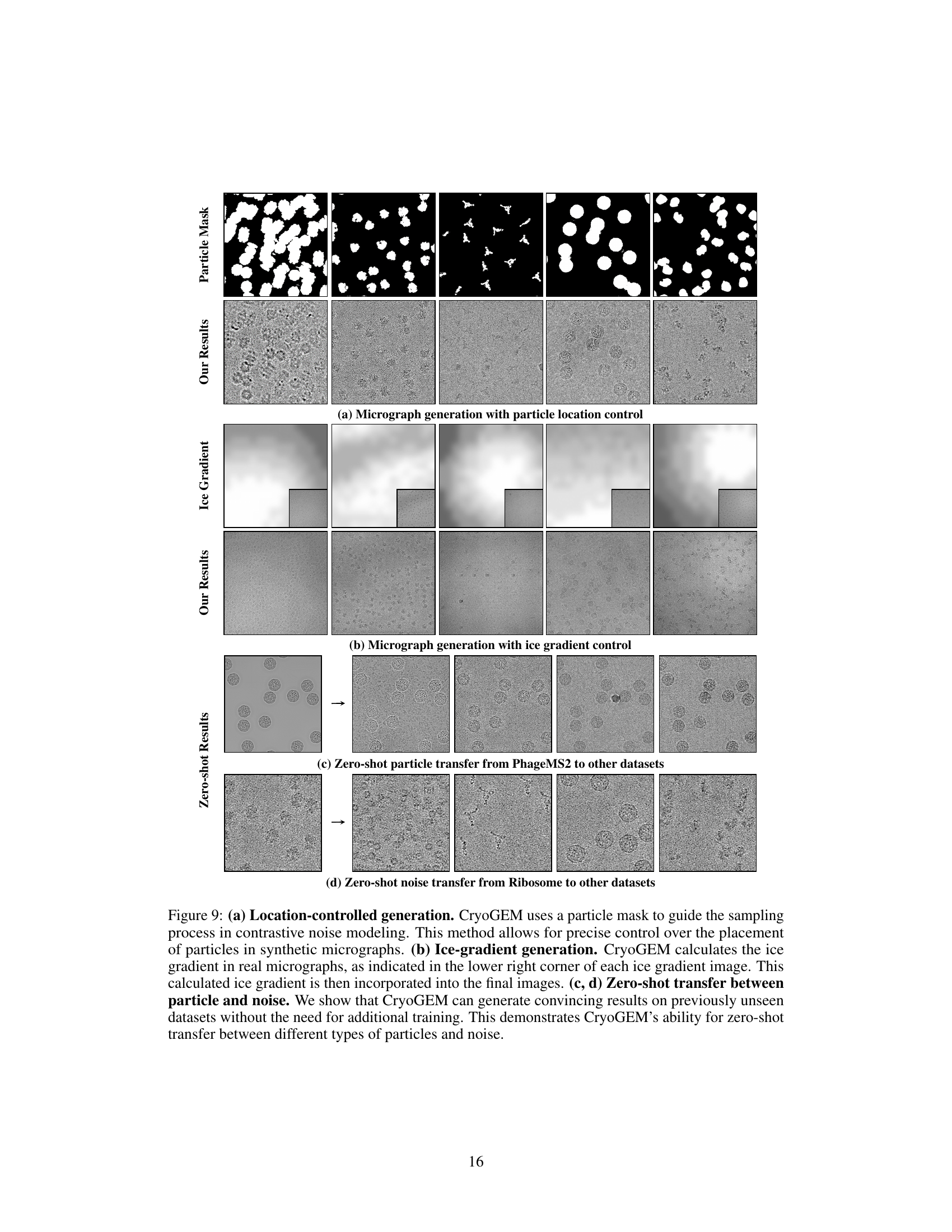
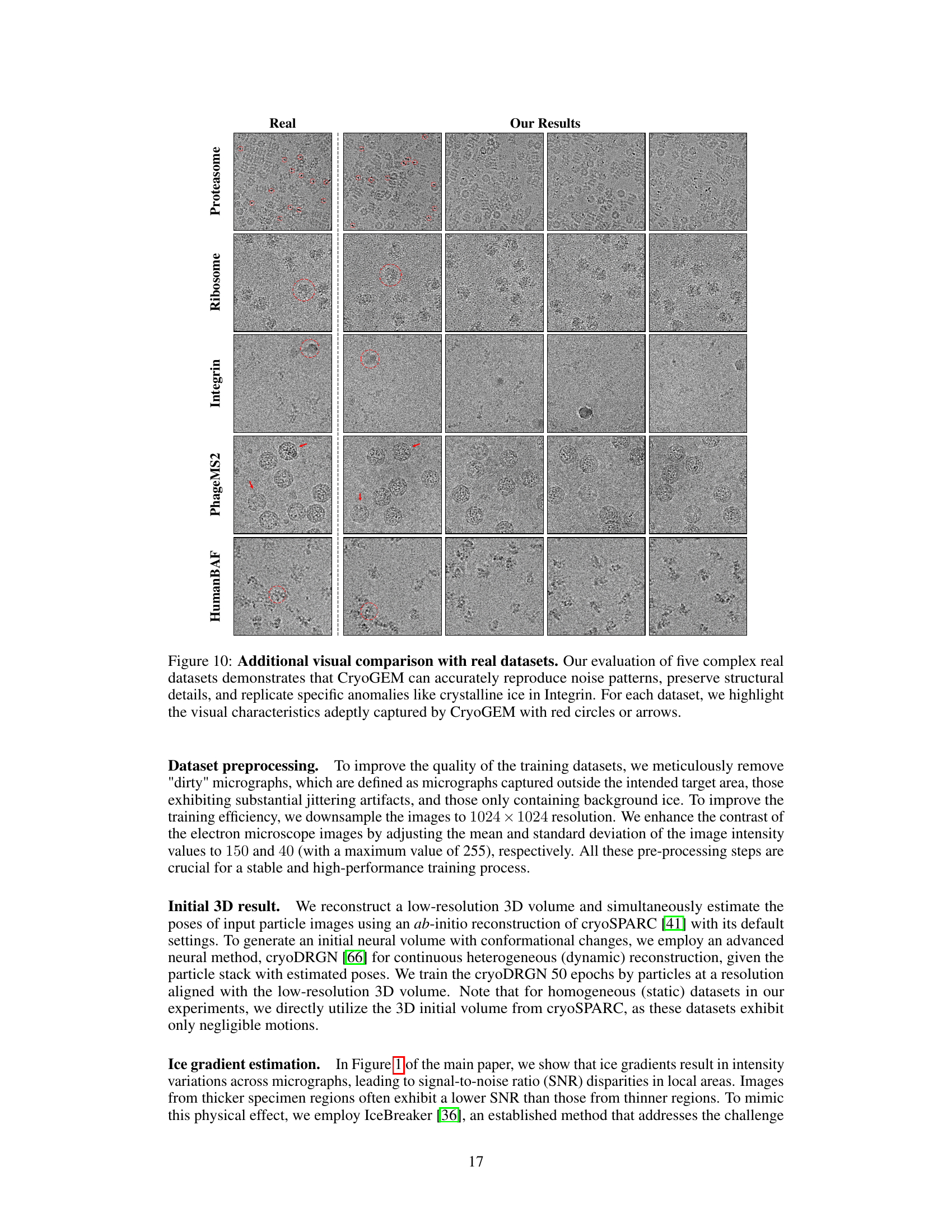
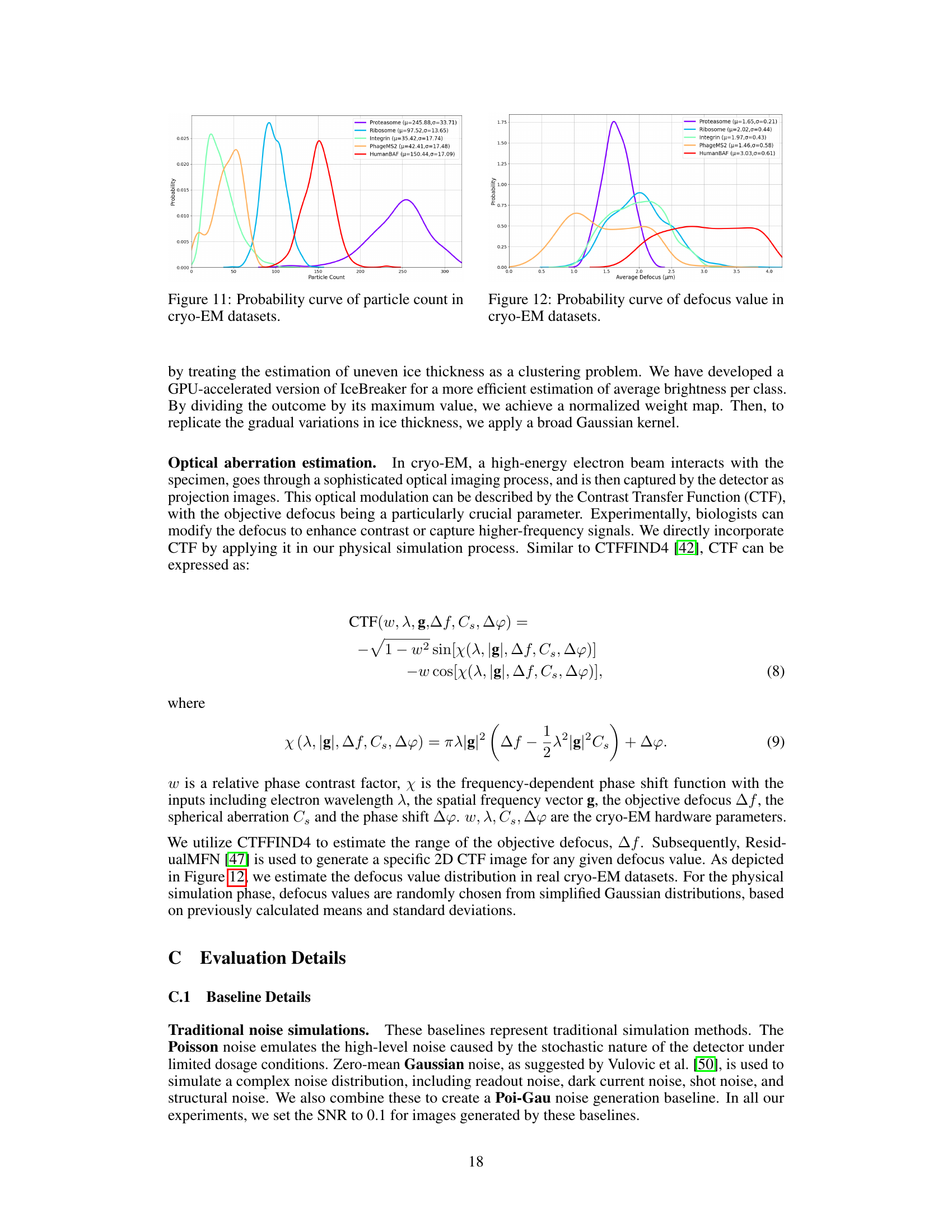
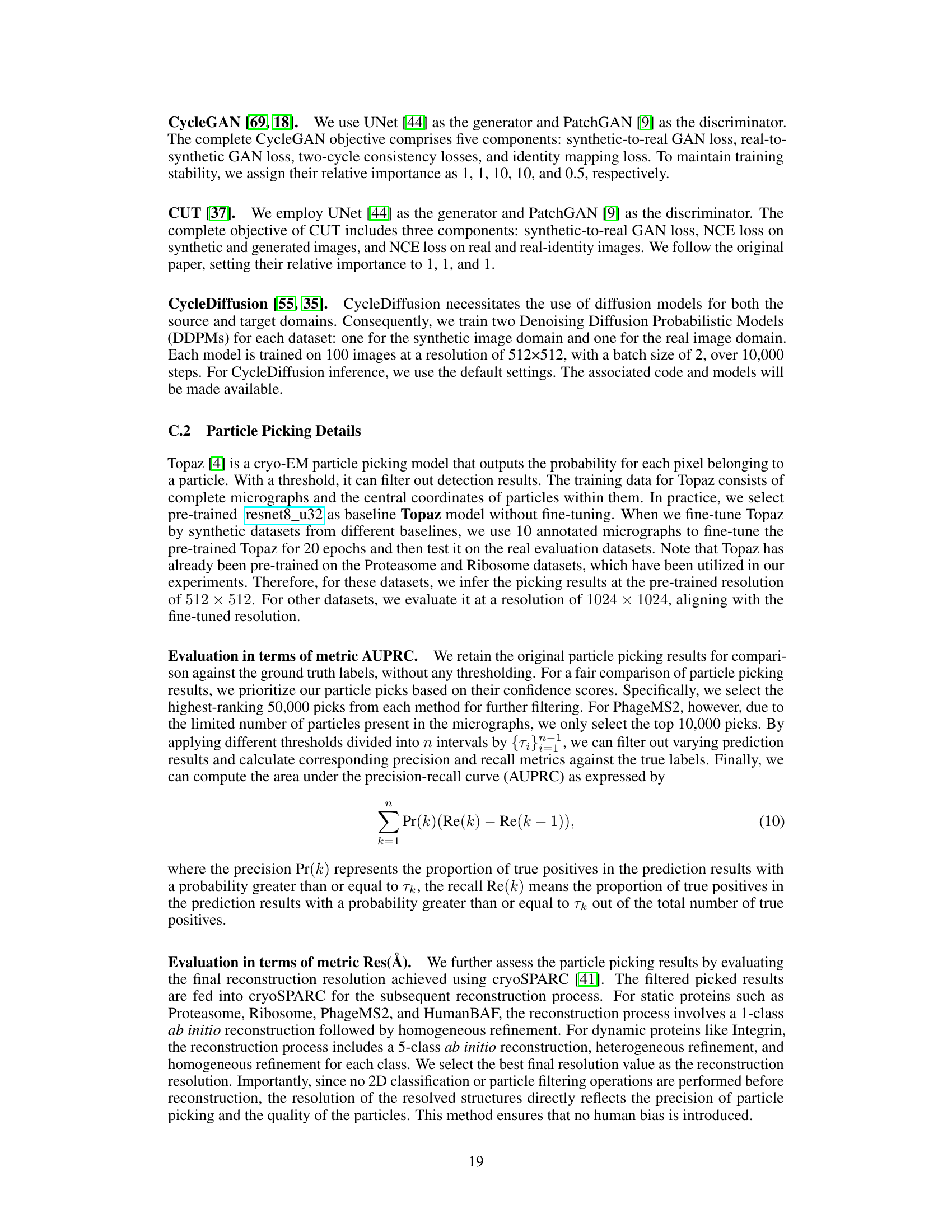
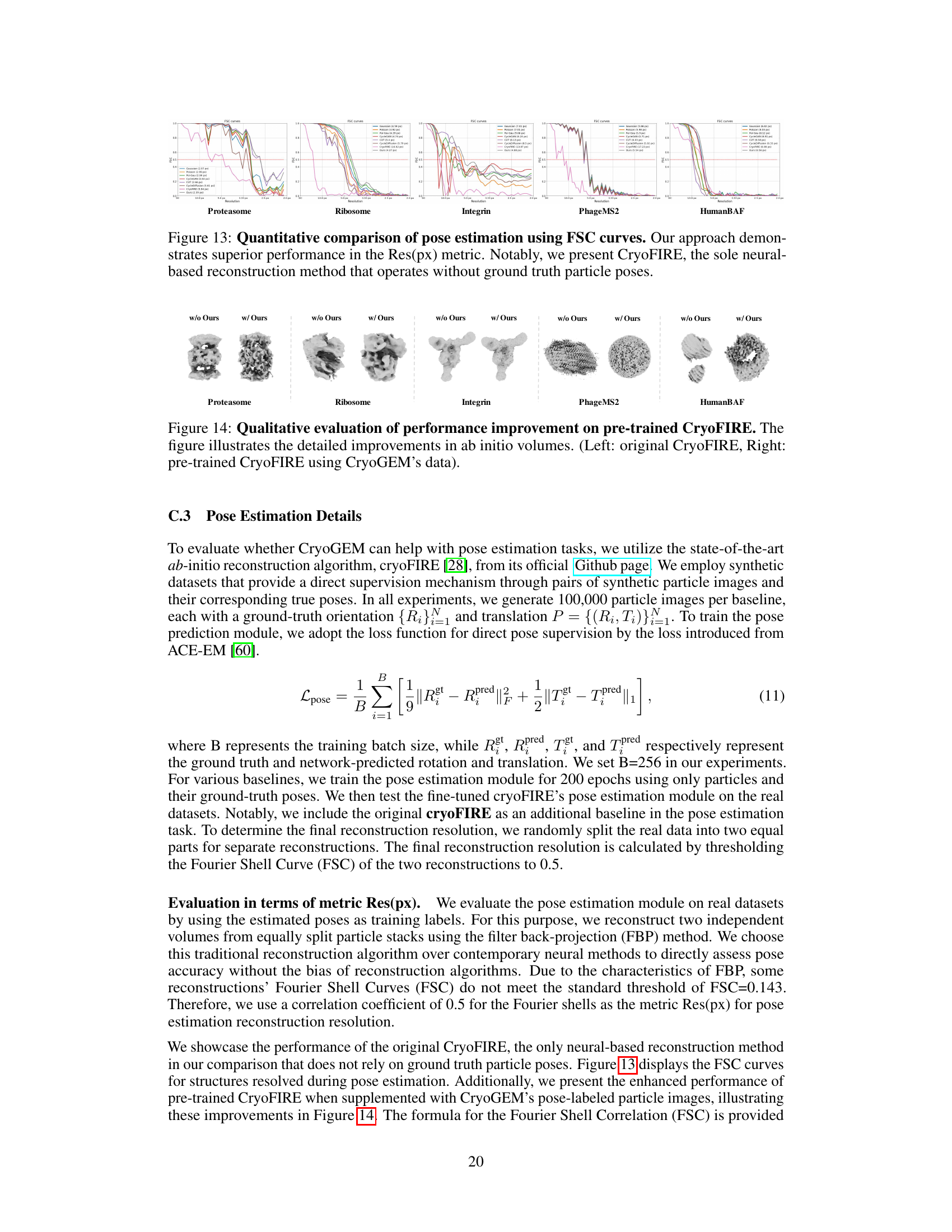