ā OpenReview ā NeurIPS Homepage ā Chat
TL;DR#
Collaborative learning, while promising, faces challenges when participants are strategic and data quality varies. A naive approach to aggregation may lead to ‘unravelling’, where only the lowest quality data contributors remain, hindering model performance. This occurs because high-quality contributors withdraw to avoid being negatively affected by lower-quality data.
This paper introduces a novel method that tackles this issue. Using probabilistic verification, the researchers design a mechanism to make the grand coalition (all agents participating) a Nash equilibrium with high probability, thus breaking the unravelling effect. This is achieved without using external transfers, making the solution both effective and practical for real-world deployment. The method is demonstrated in a classification setting, highlighting its applicability to real-world scenarios.
Key Takeaways#
Why does it matter?#
This paper is crucial because it addresses a critical challenge in collaborative learning: the negative impact of data quality asymmetry. By offering solutions to the problem of unraveling, it enhances the reliability and stability of collaborative models, opening avenues for more robust and efficient decentralized learning systems.
Visual Insights#
Full paper#
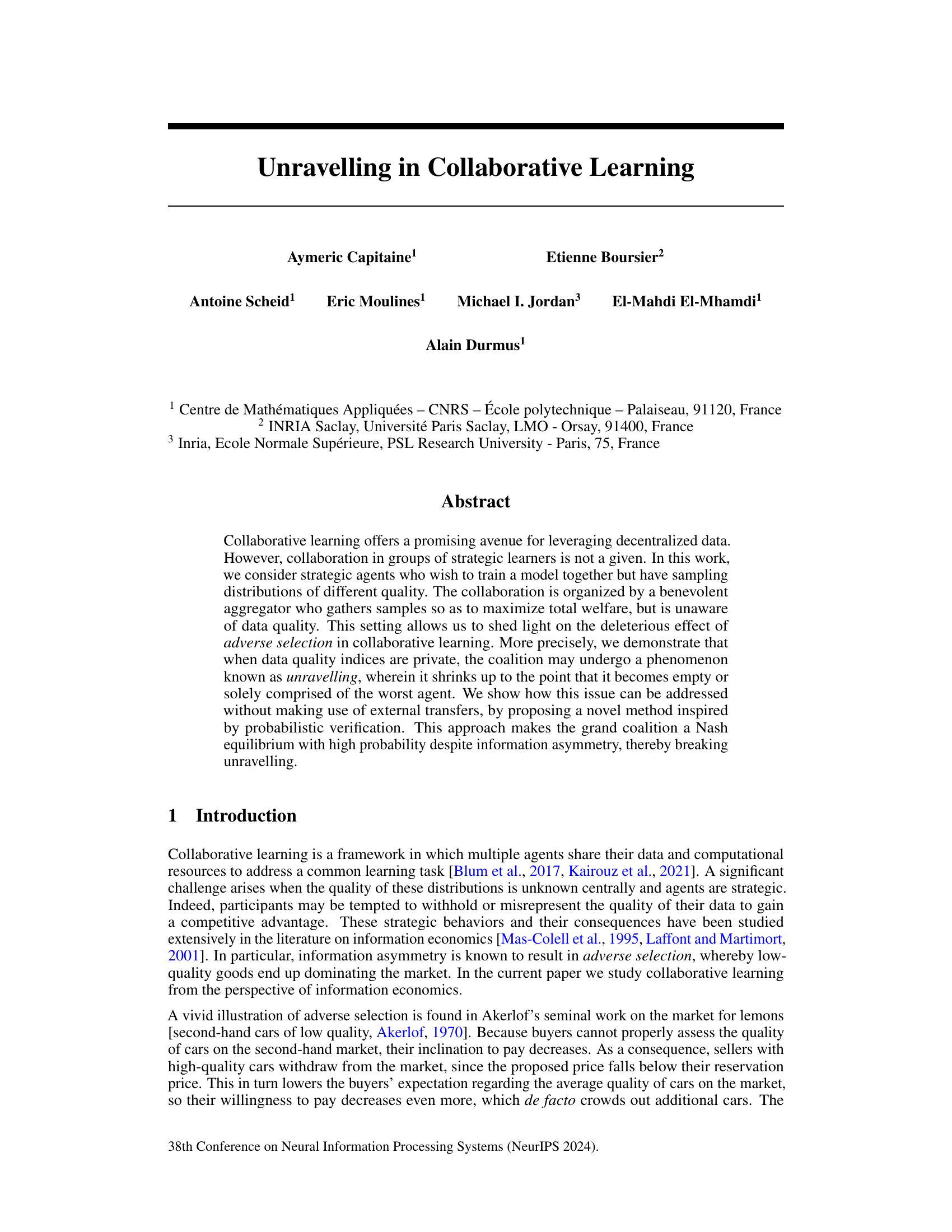
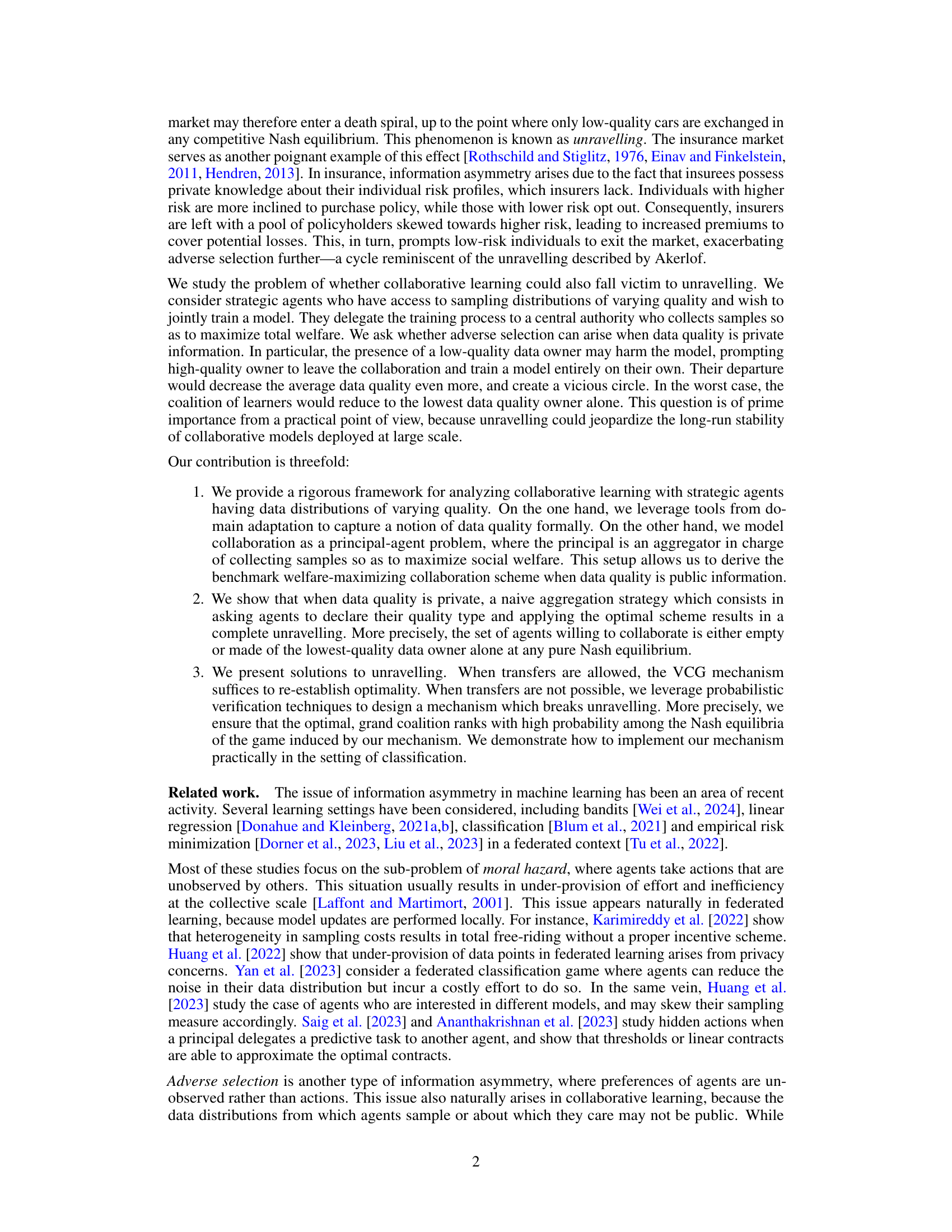
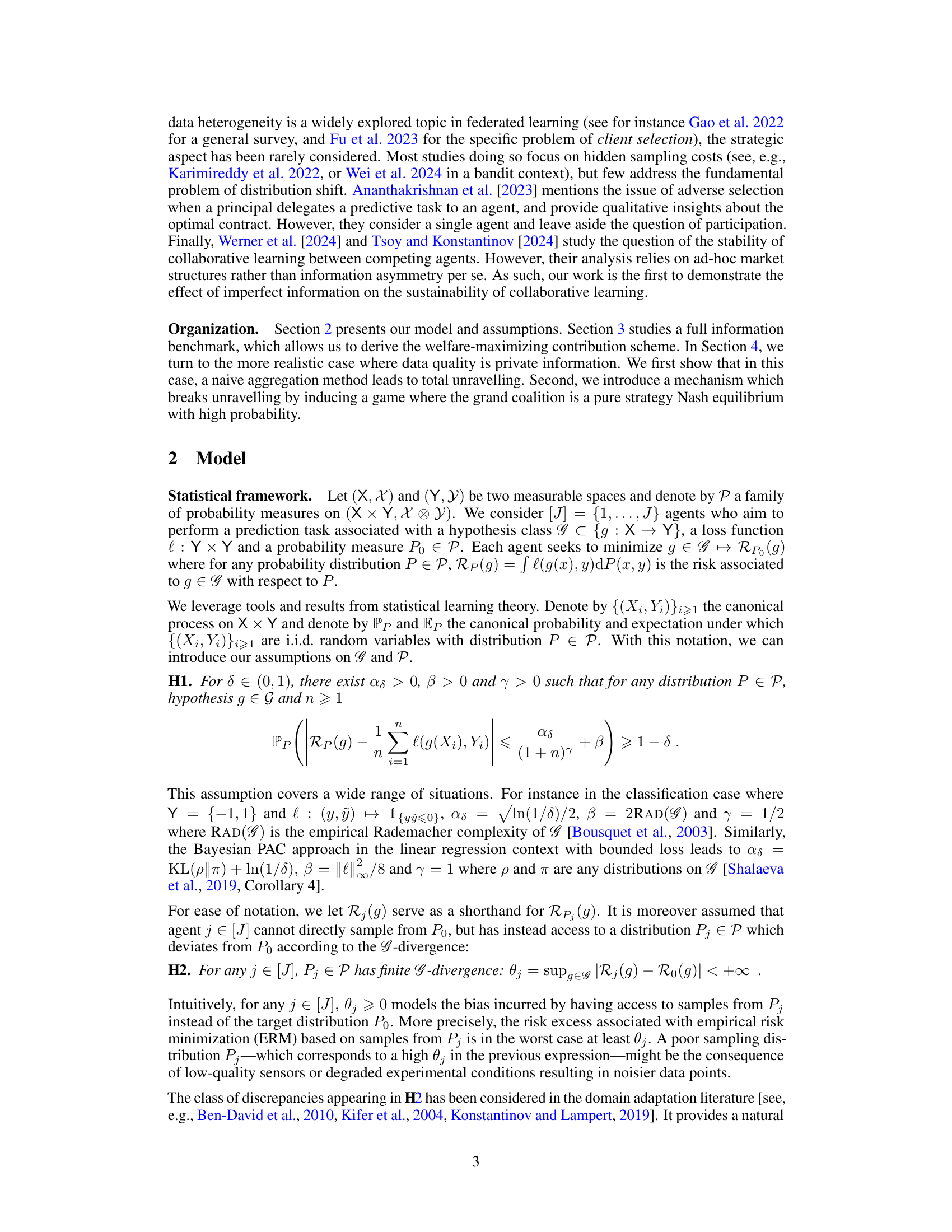
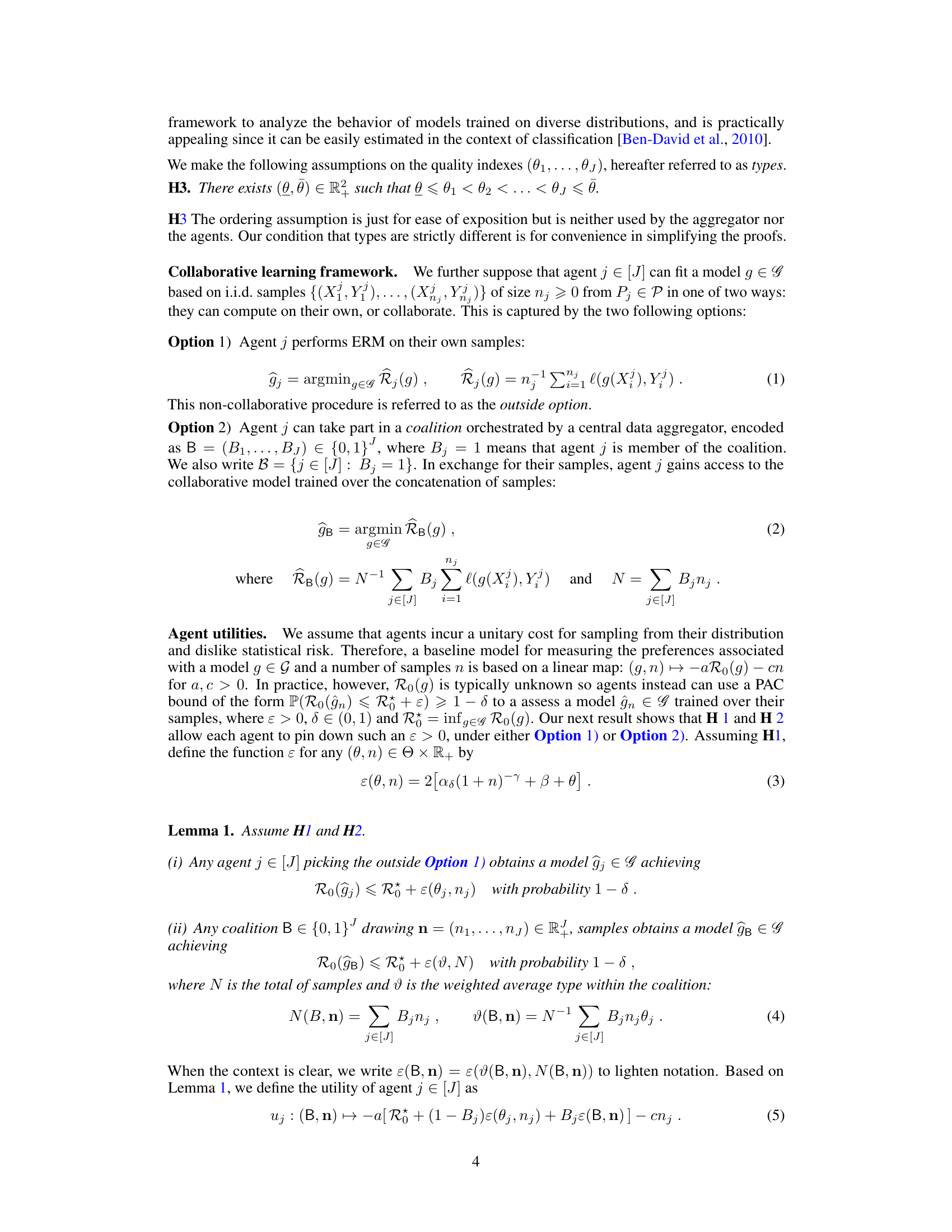
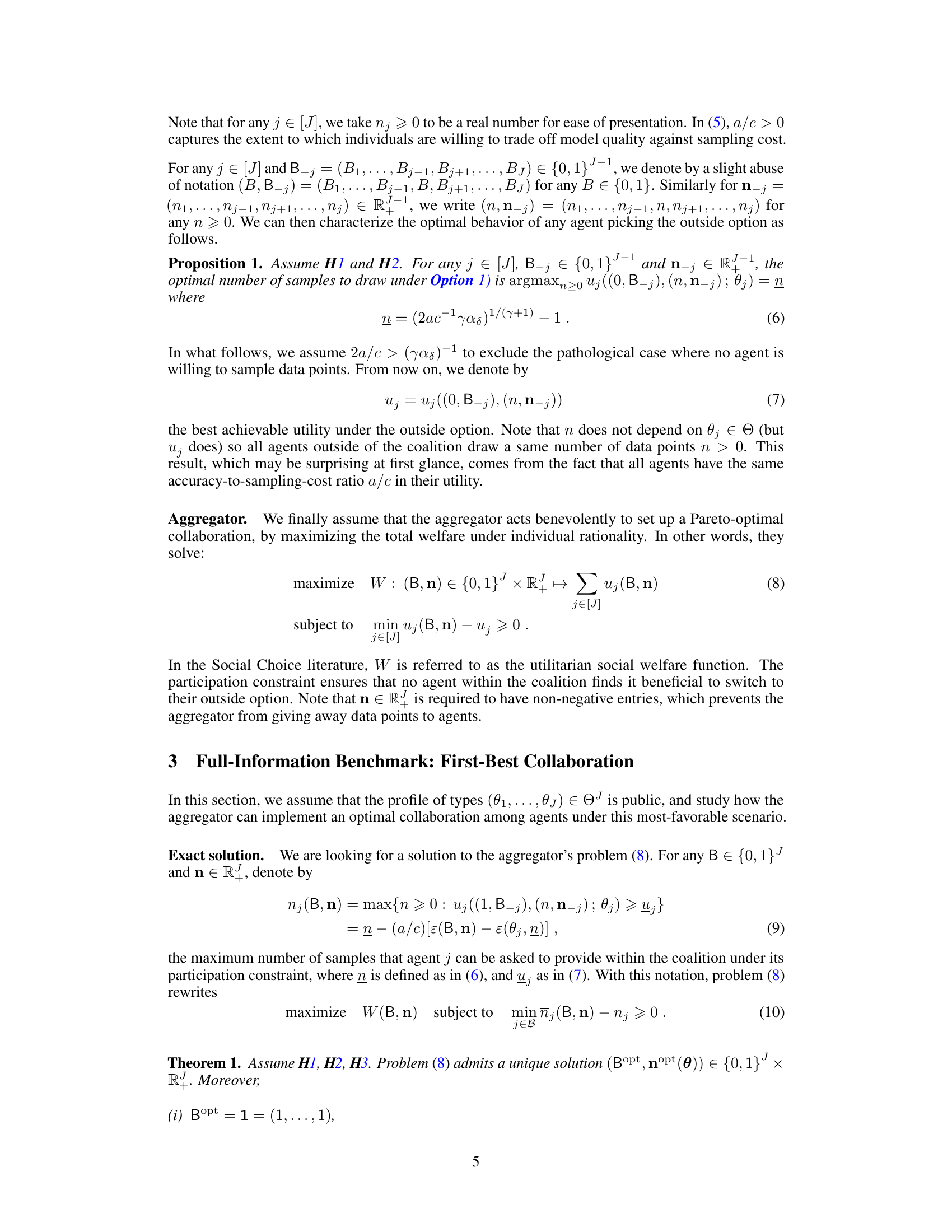
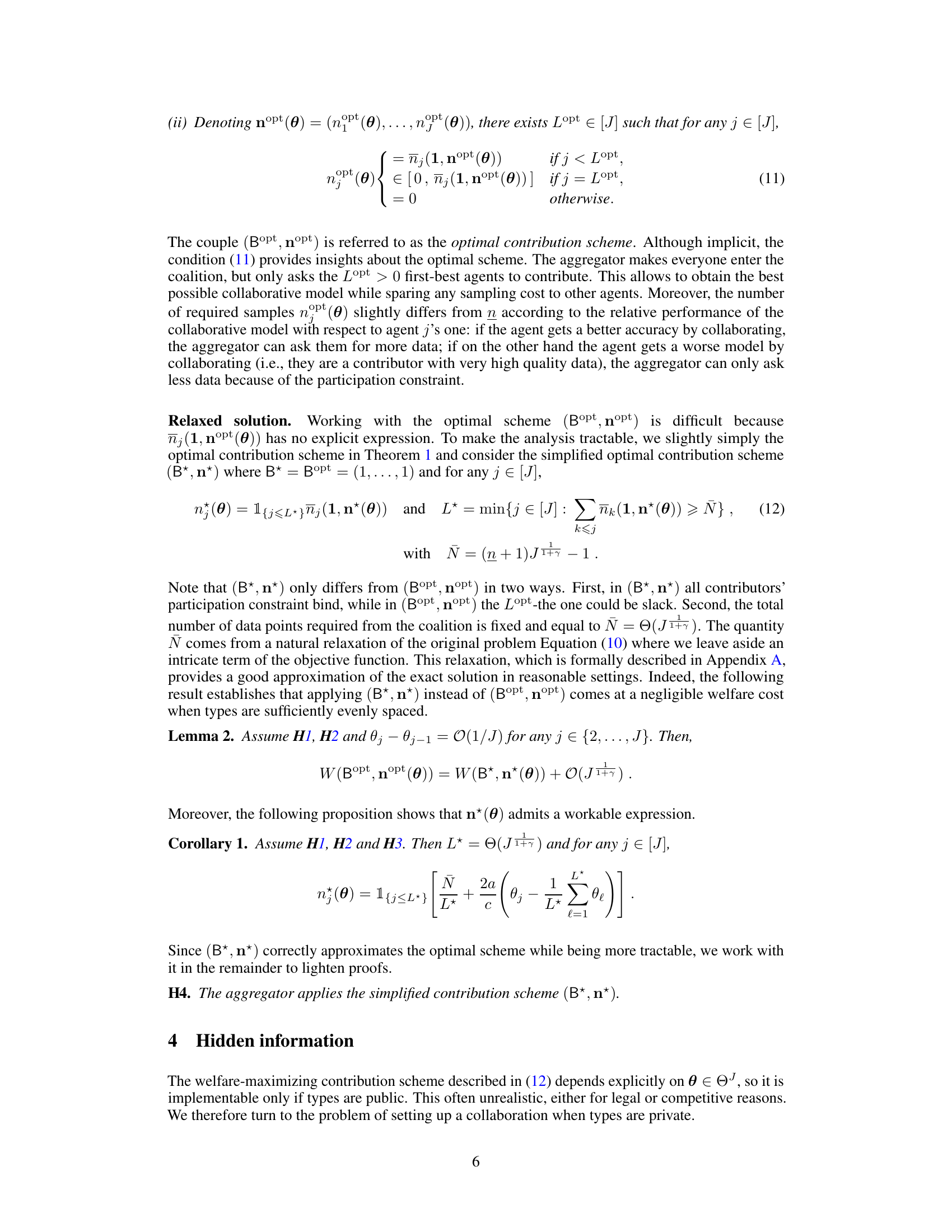
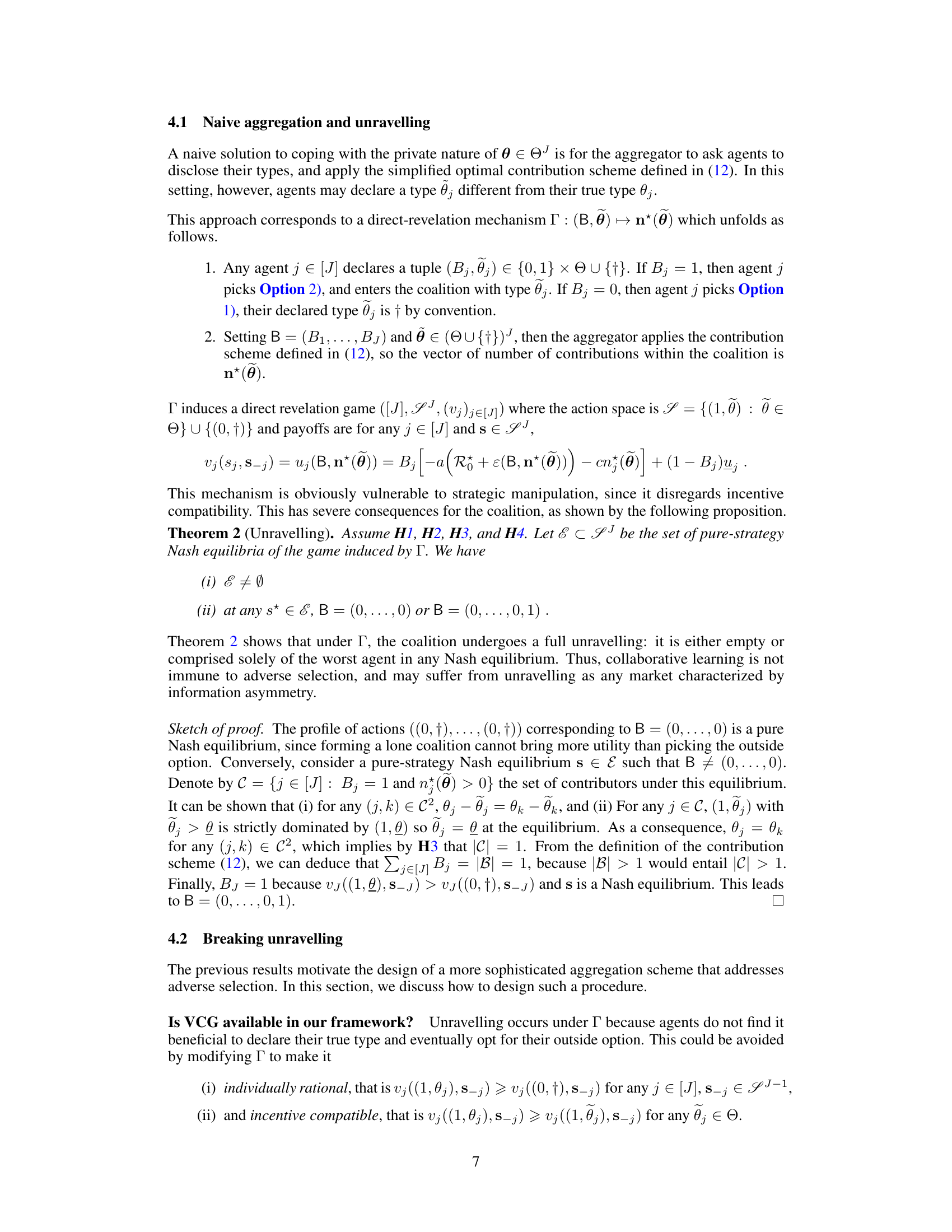
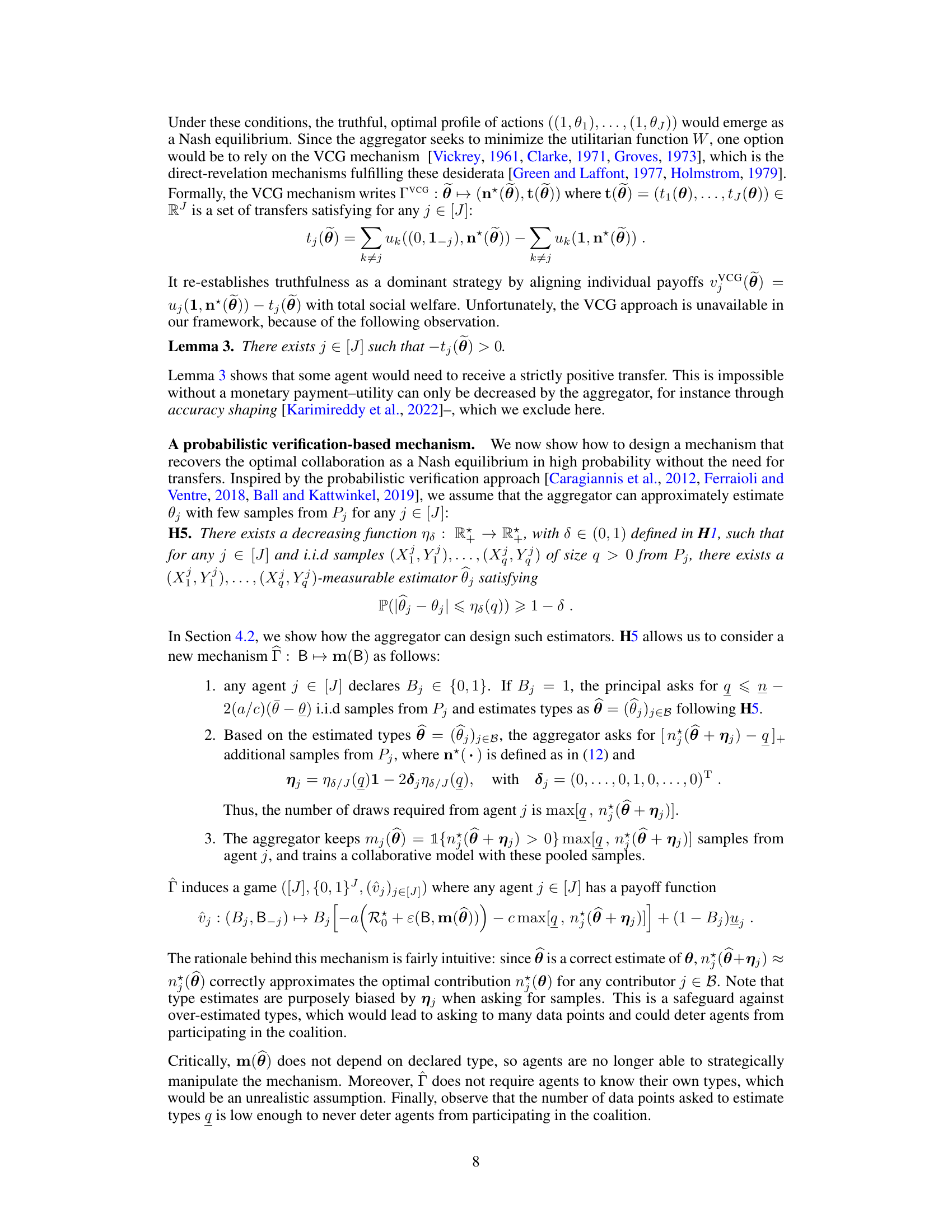
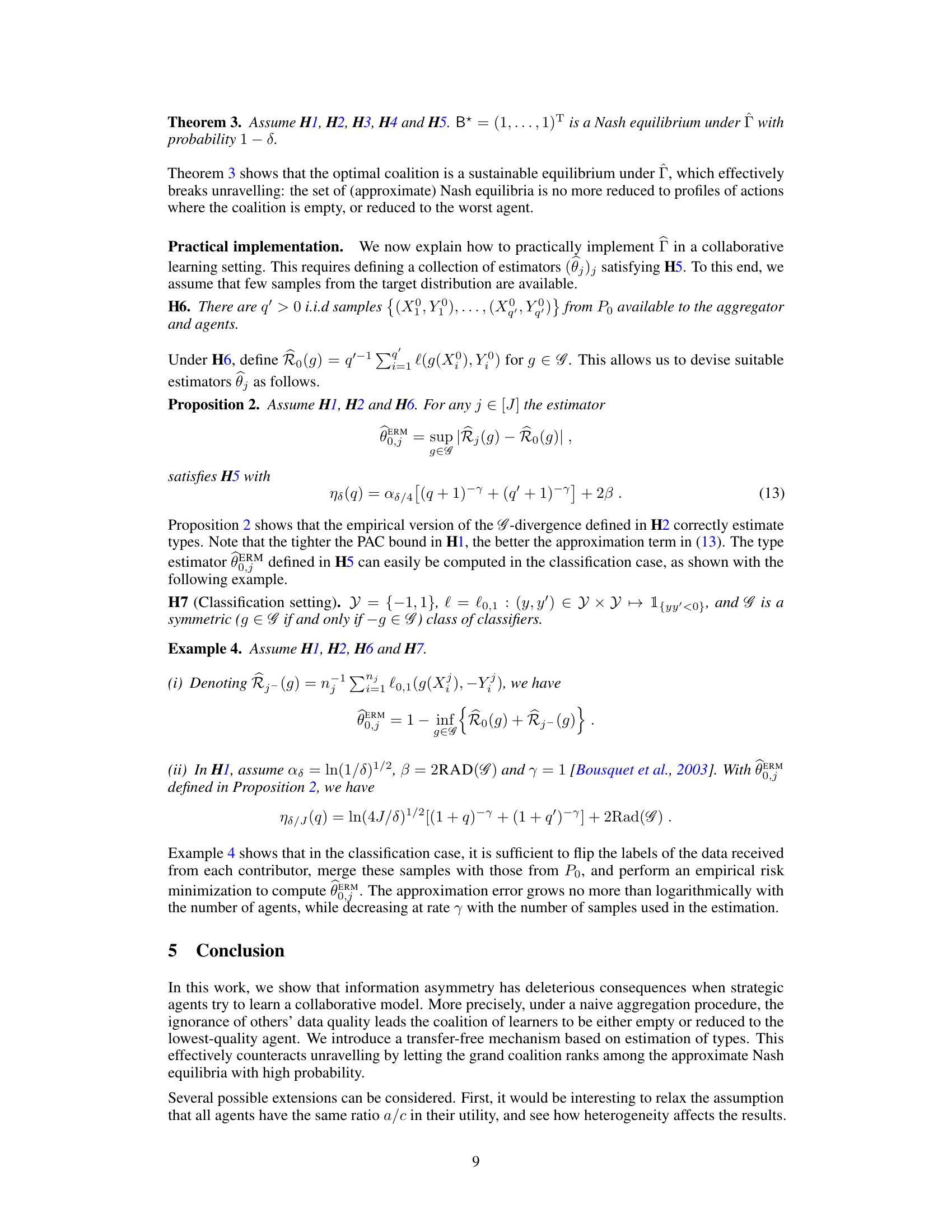
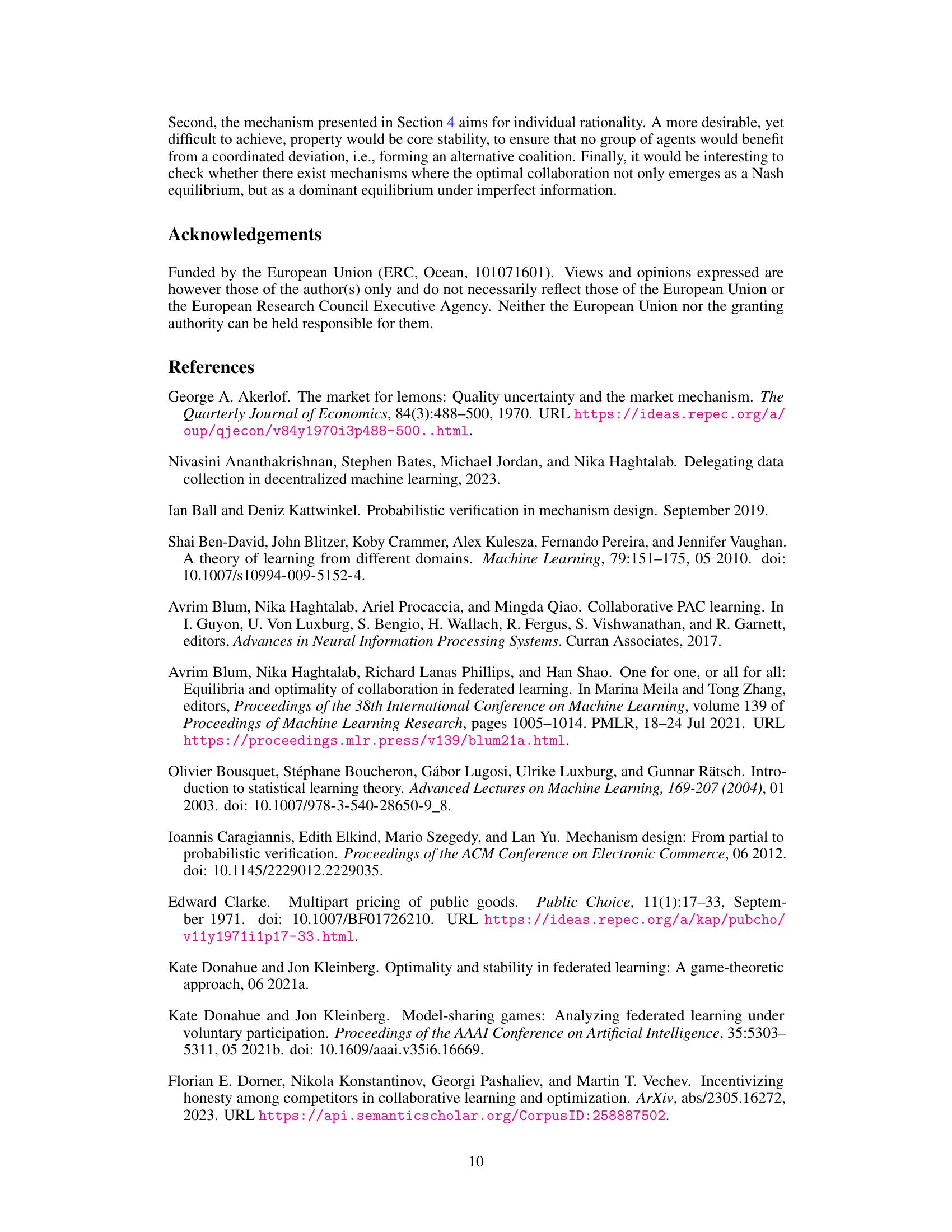
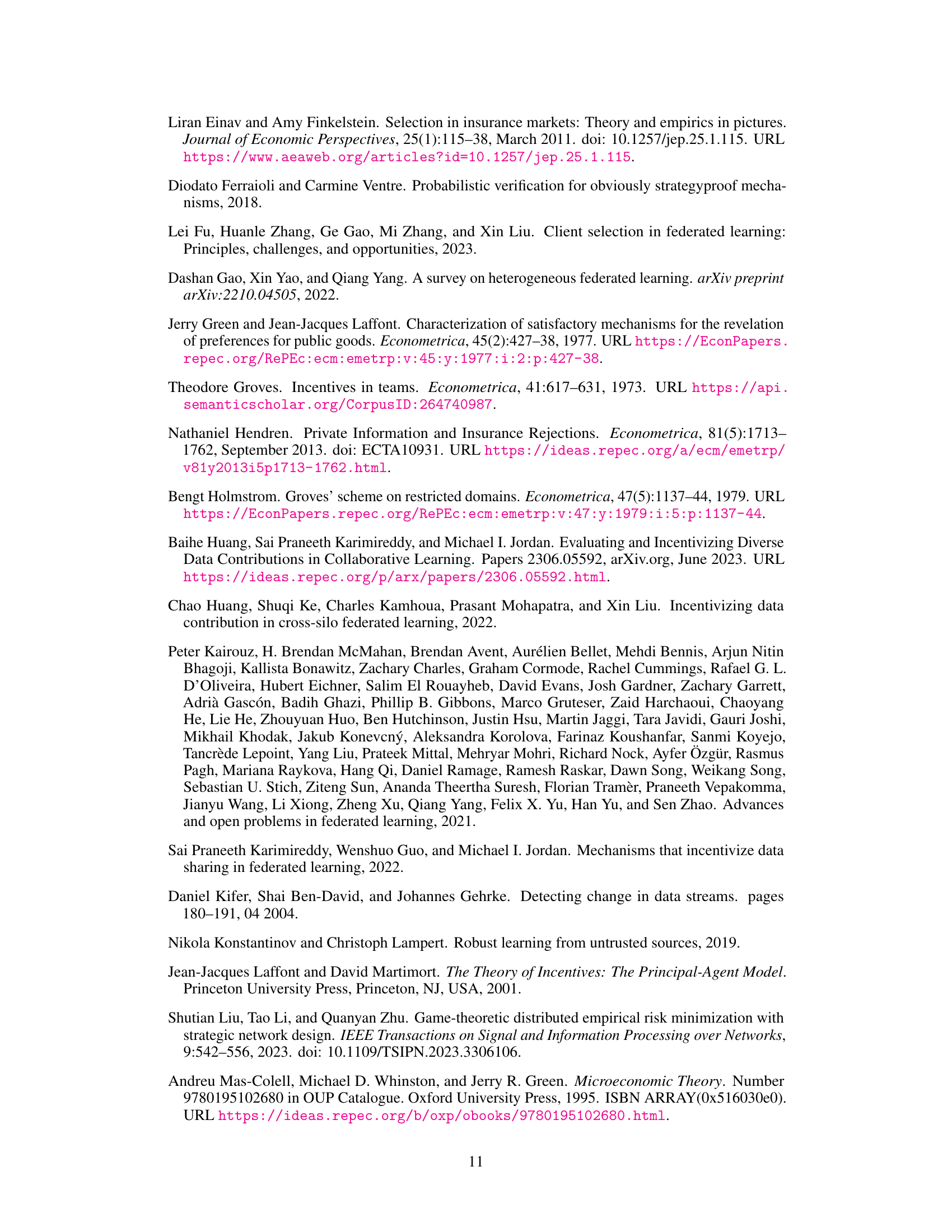
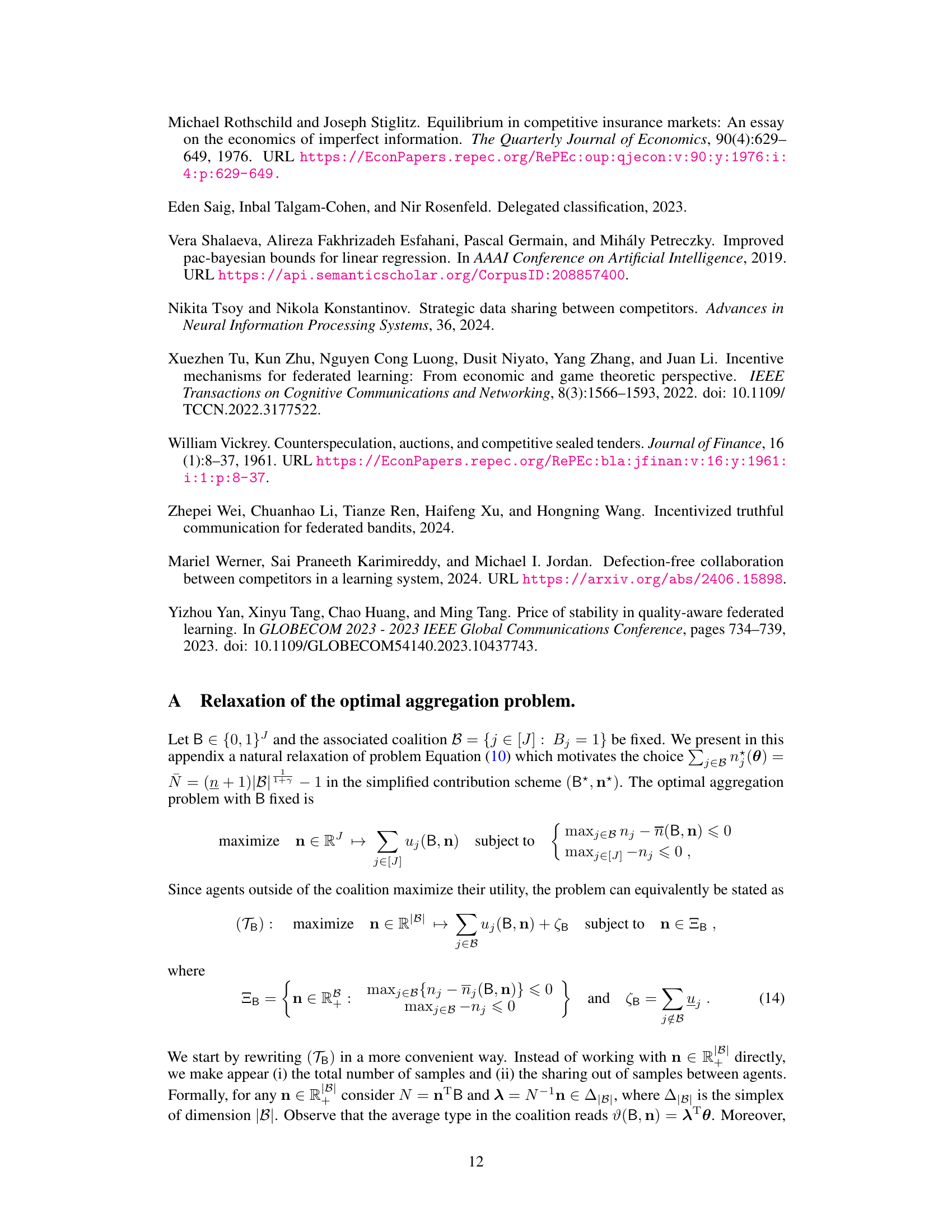
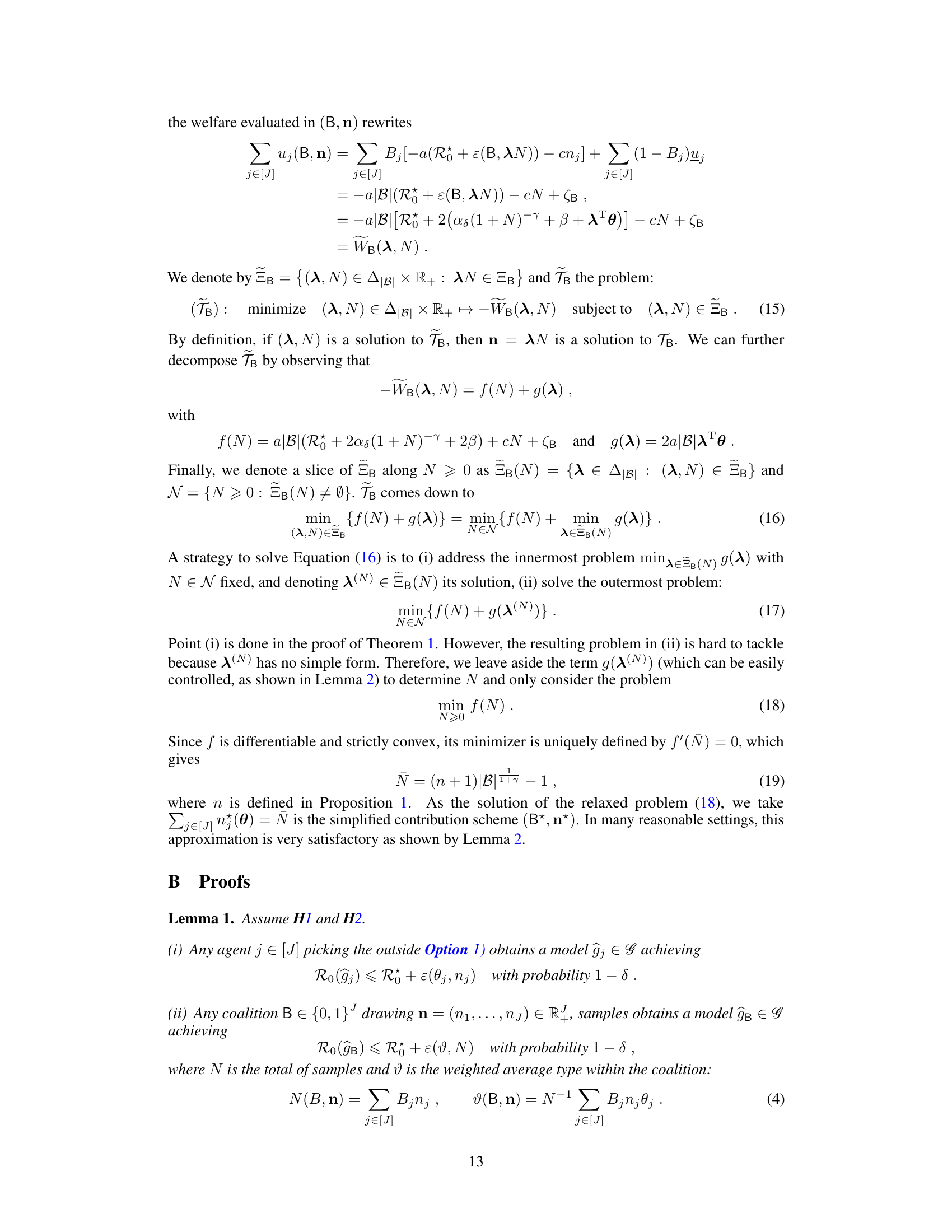
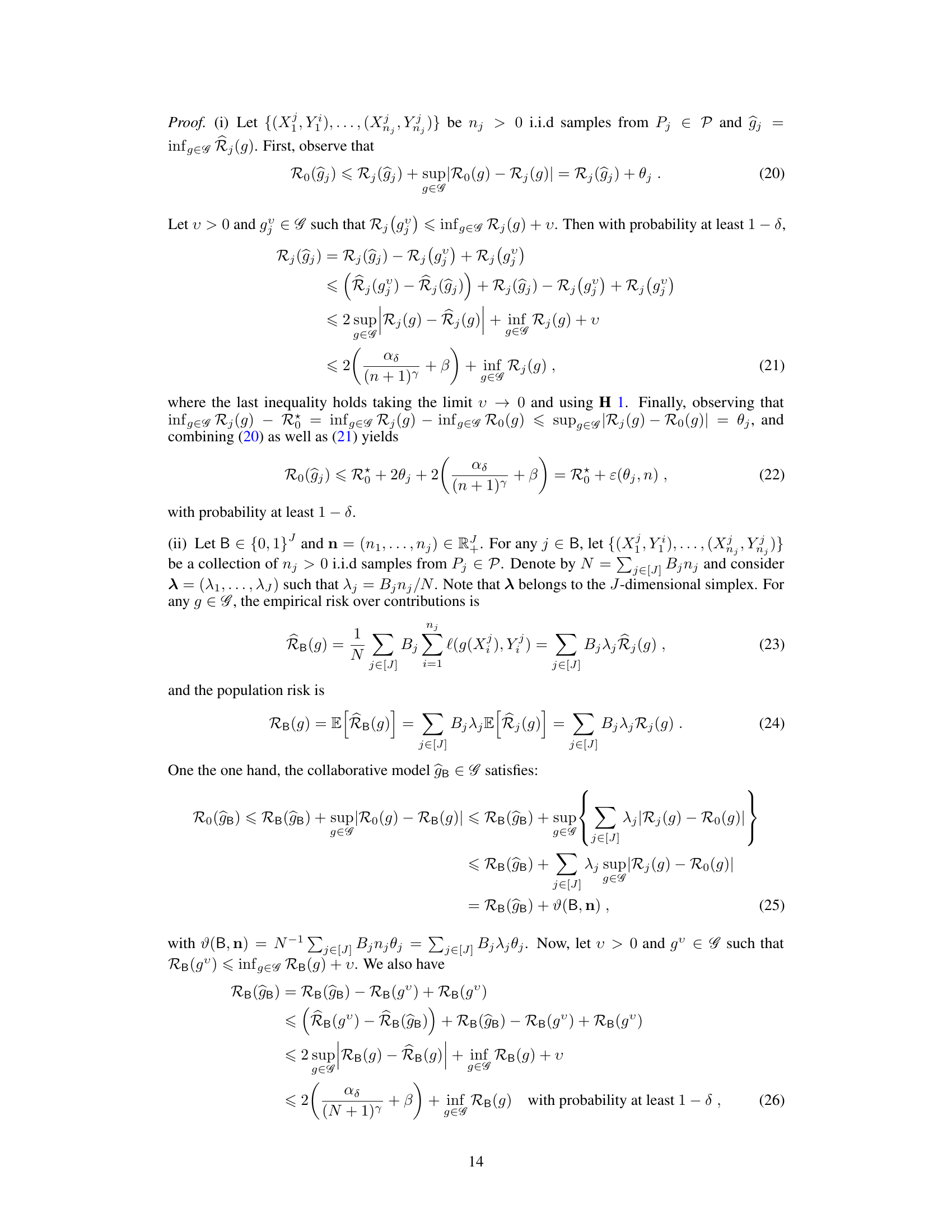
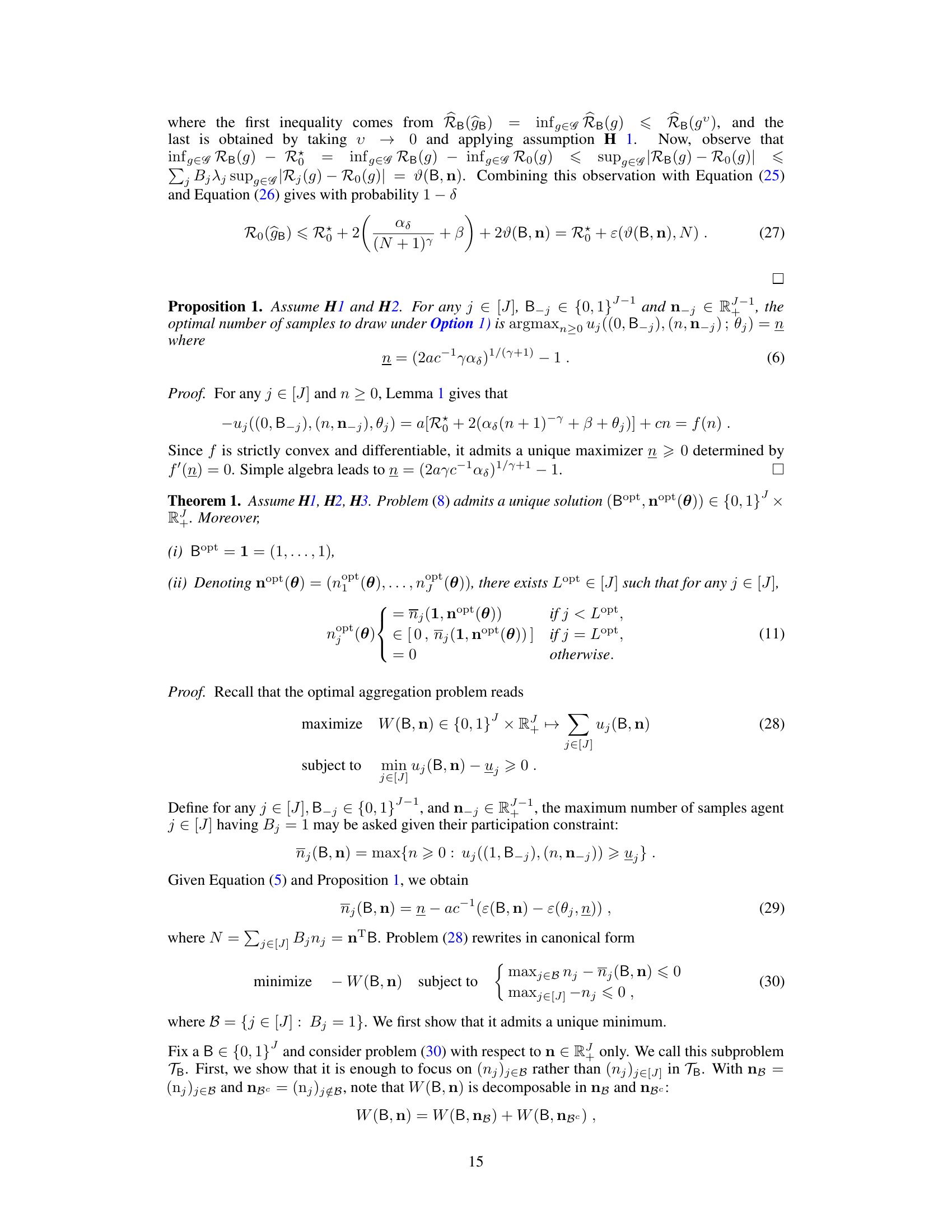
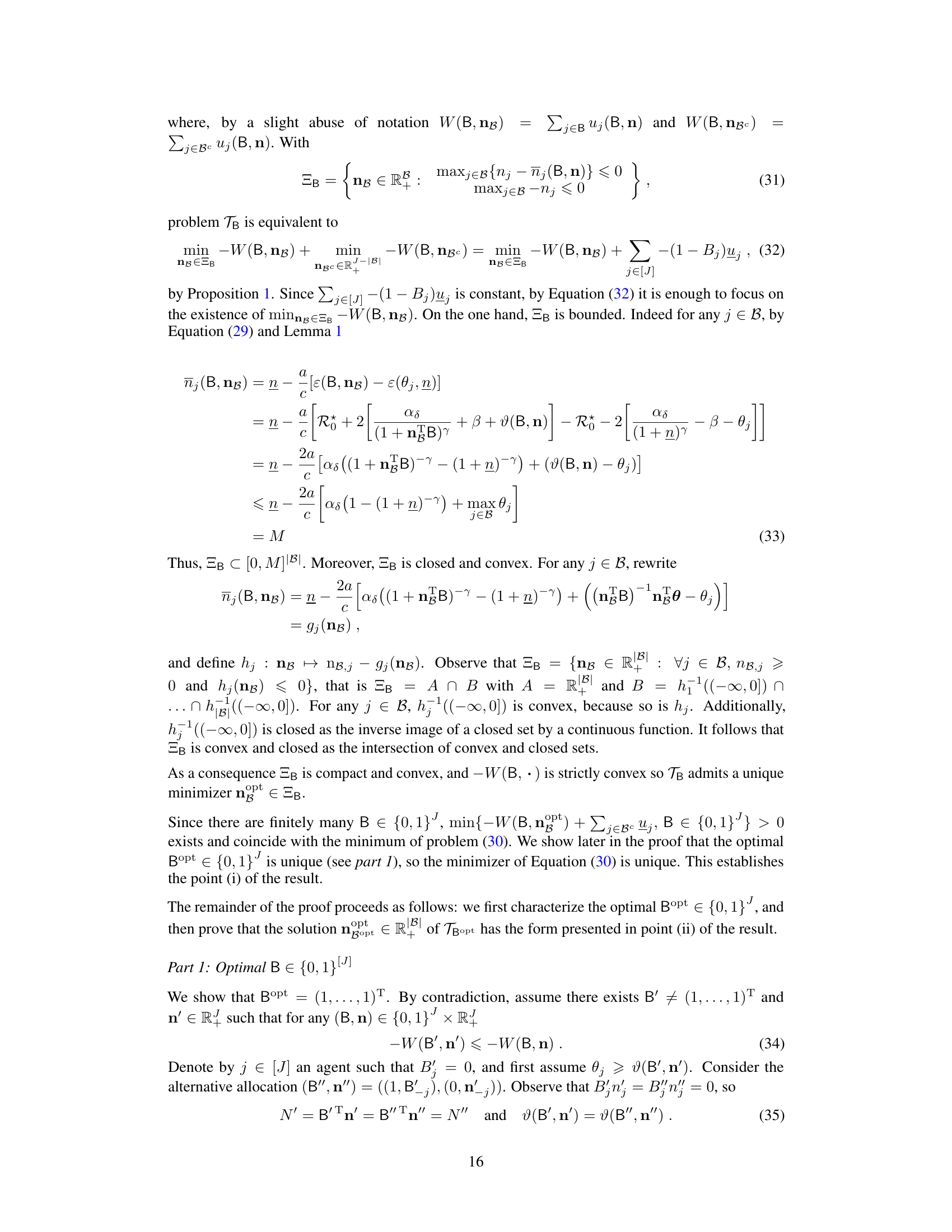
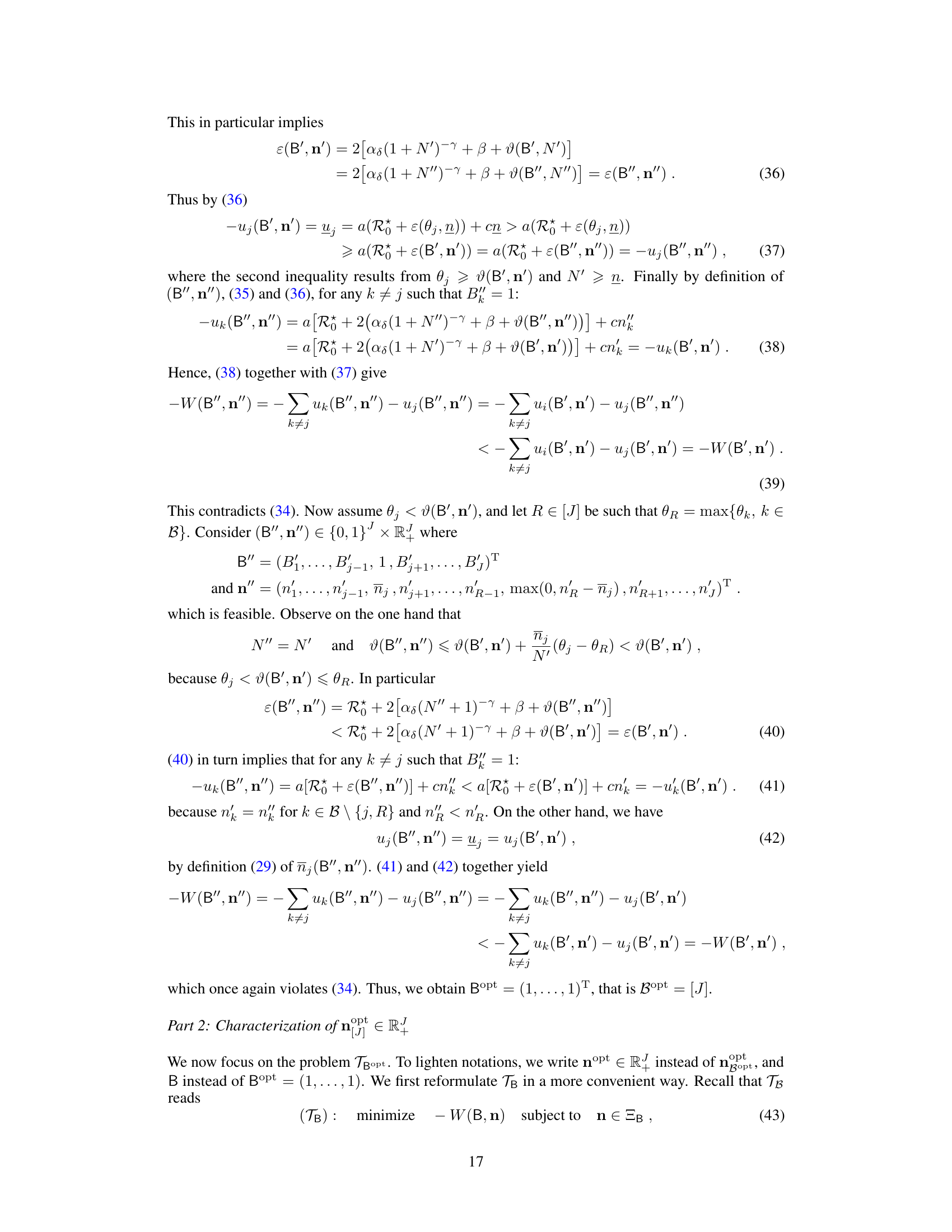
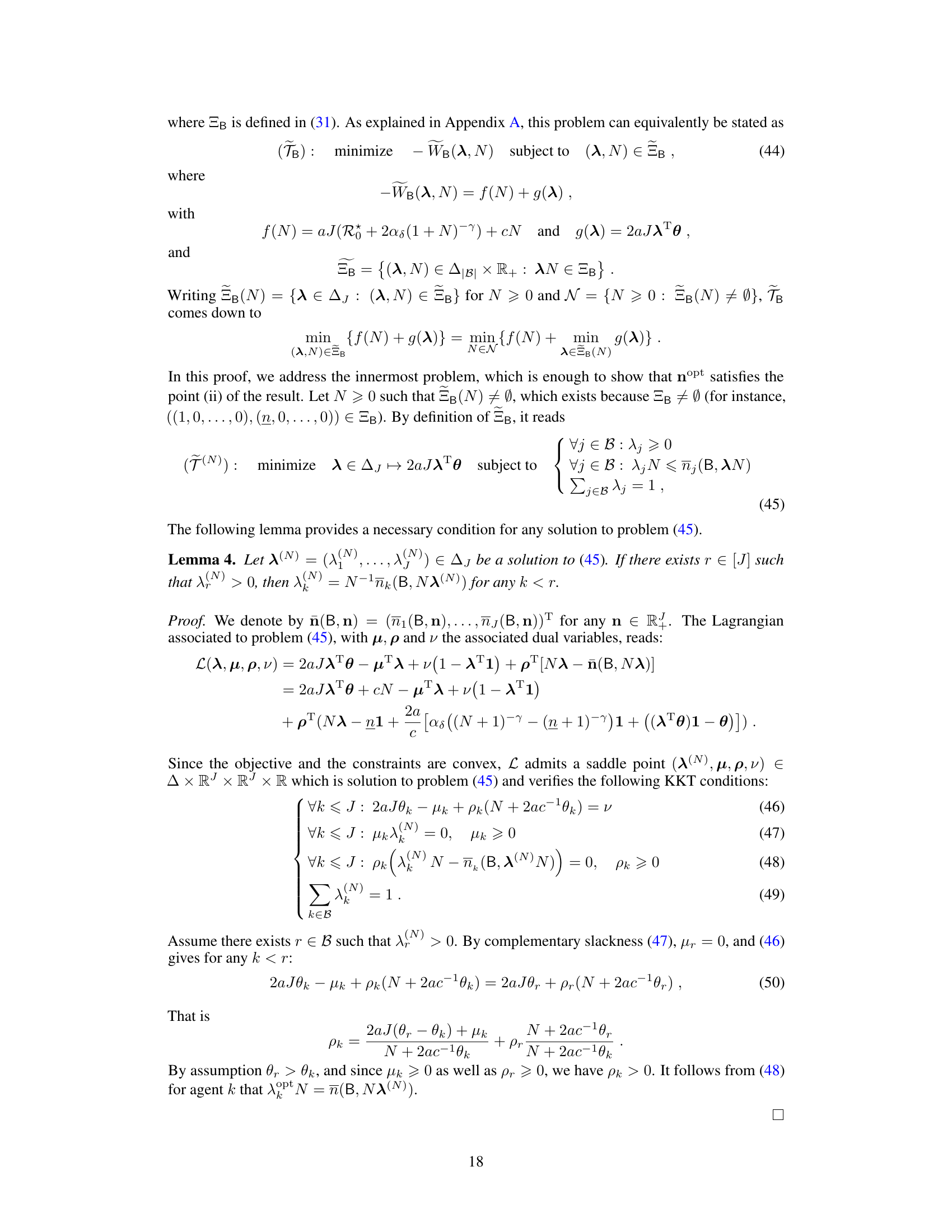
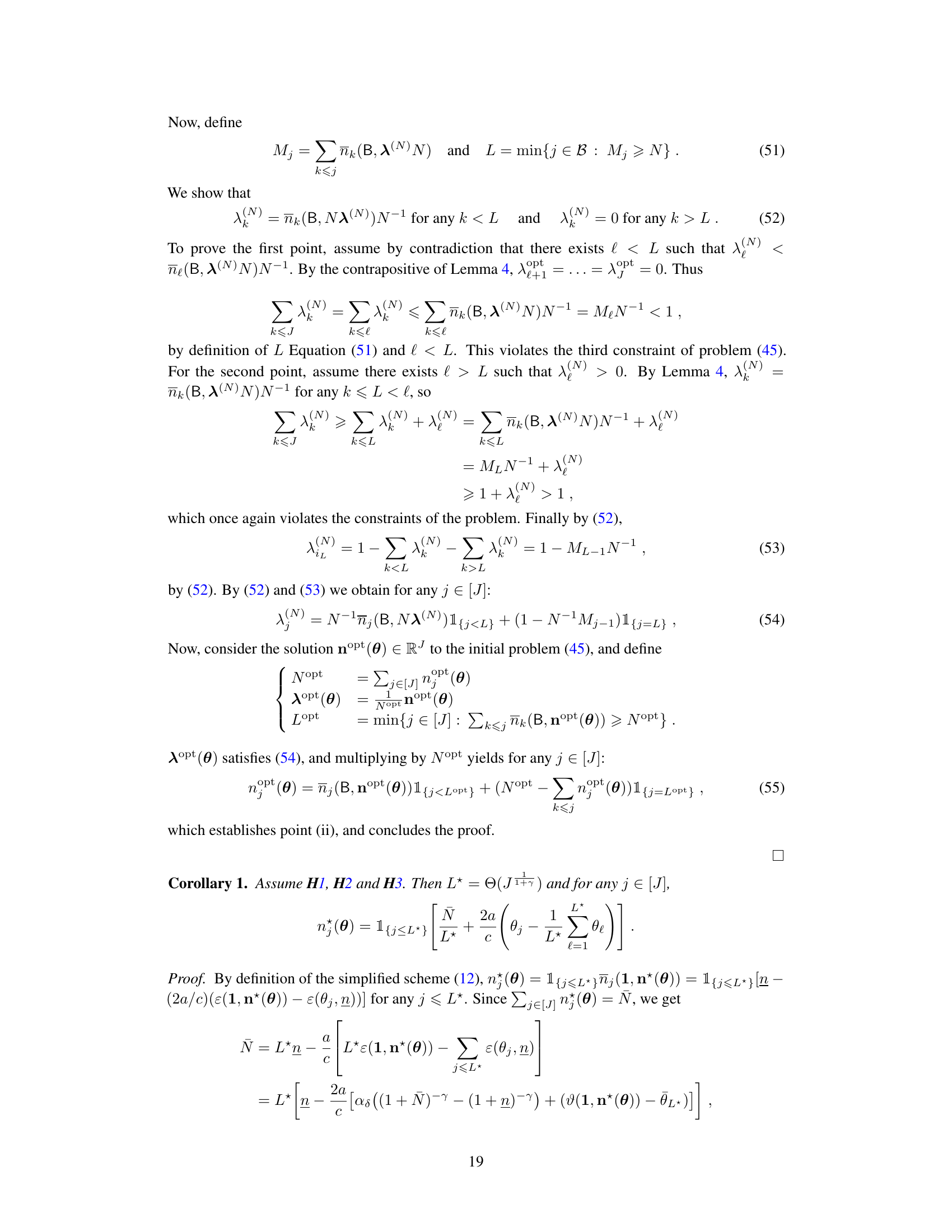
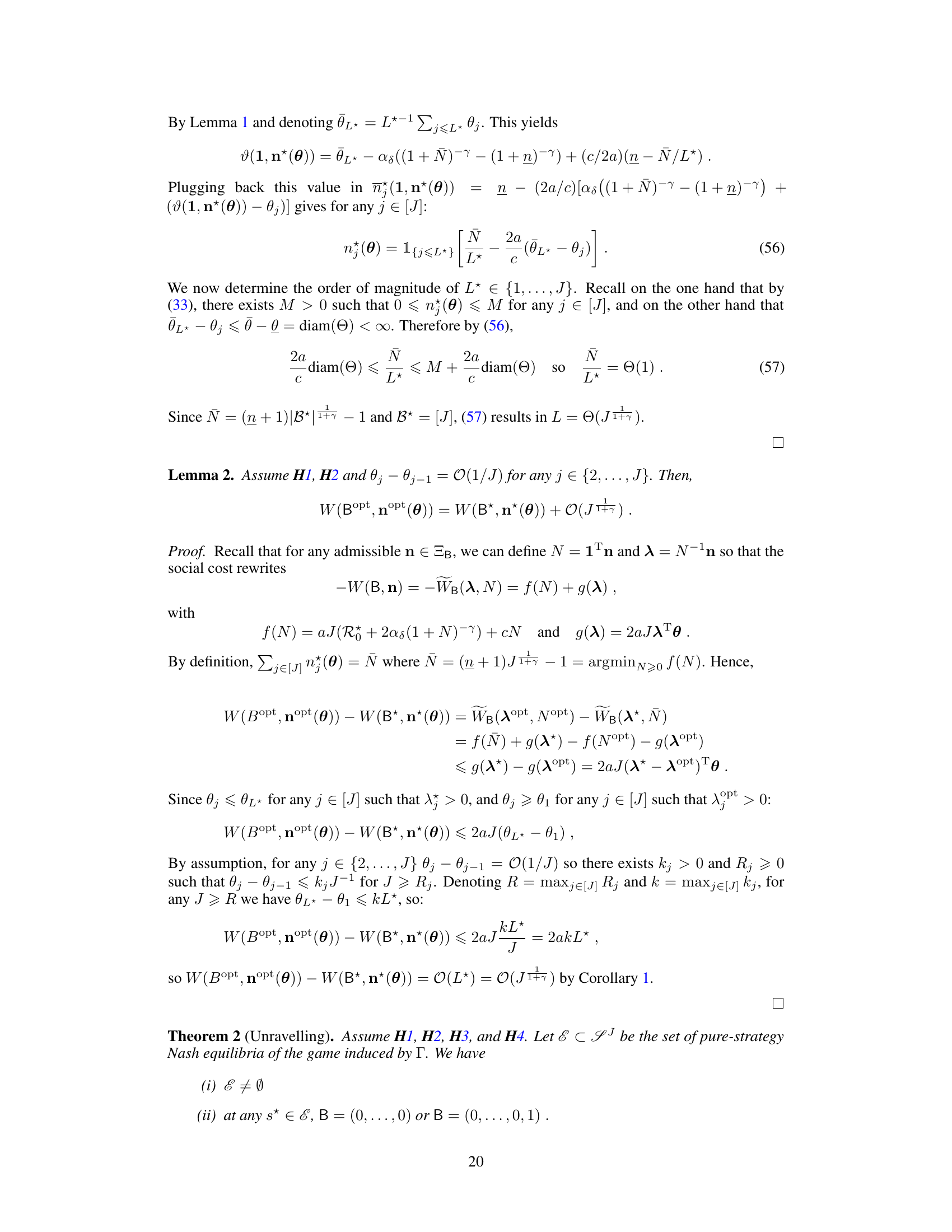