TL;DR#
Key Takeaways#
Why does it matter?#
This paper is crucial for researchers in multi-agent reinforcement learning (MARL) and game theory. It addresses the critical issue of adaptive adversaries, which are commonly found in real-world scenarios but largely ignored in theoretical MARL research. By introducing the concept of consistent adversaries, and providing algorithms with provable guarantees, this work paves the way for more robust and efficient MARL algorithms in complex settings.
Visual Insights#
🔼 This table summarizes the results of the paper on learning against adaptive adversaries in Markov games. It shows the lower and upper bounds on policy regret achievable by a learner under different assumptions about the adversary’s behavior (memory, stationarity, consistency), and the size of the learner’s policy set. The rows represent different levels of adversary adaptivity, from unbounded memory to memory-bounded and consistent. The columns show the corresponding lower bounds (Ω) and upper bounds (Õ) on policy regret. The special case of a single-agent MDP is represented by m = 0 and stationary.
read the caption
Table 1: Summary of main results for learning against adaptive adversaries. Learner's policy set is all deterministic Markov policies. m = 0 + stationary corresponds to standard single-agent MDPs.
Full paper#
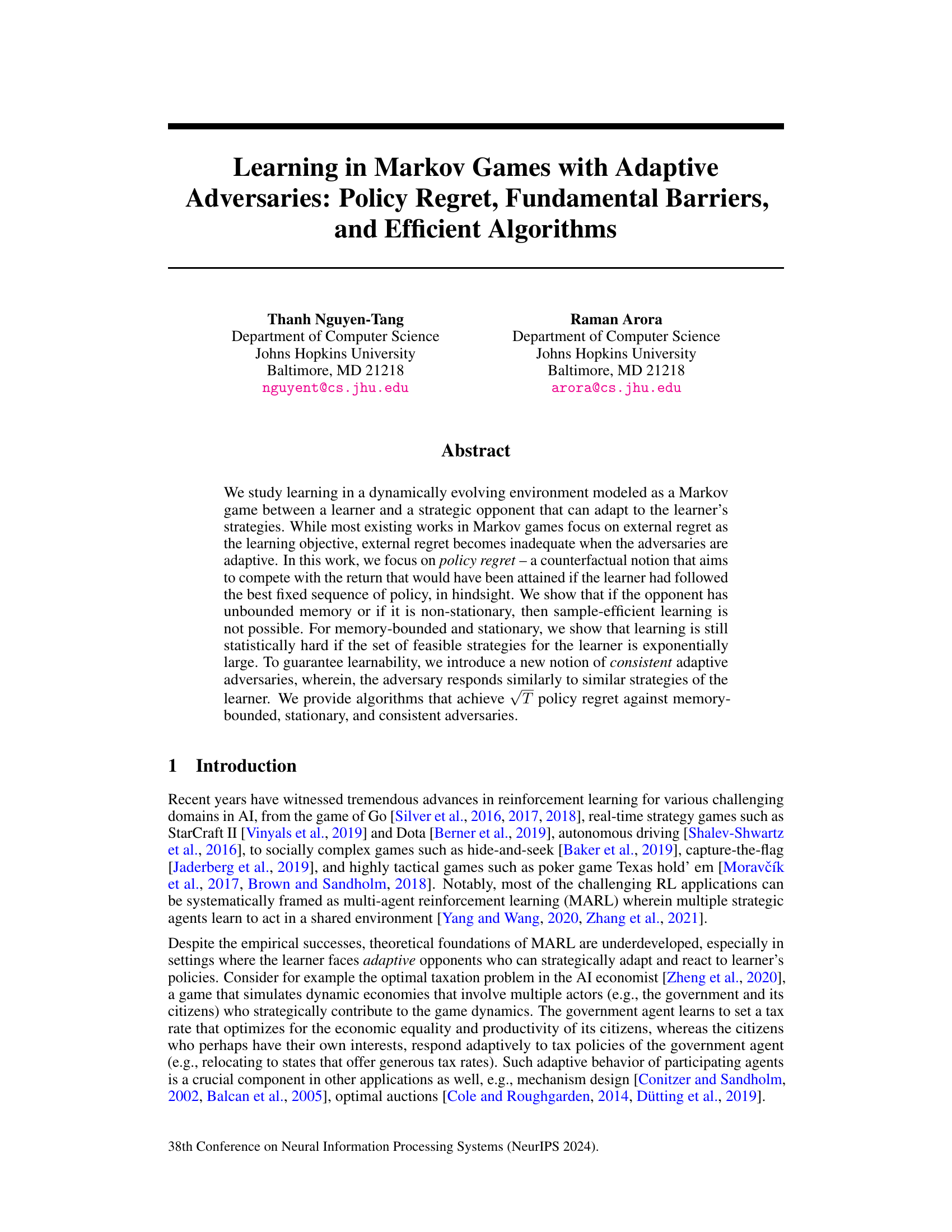
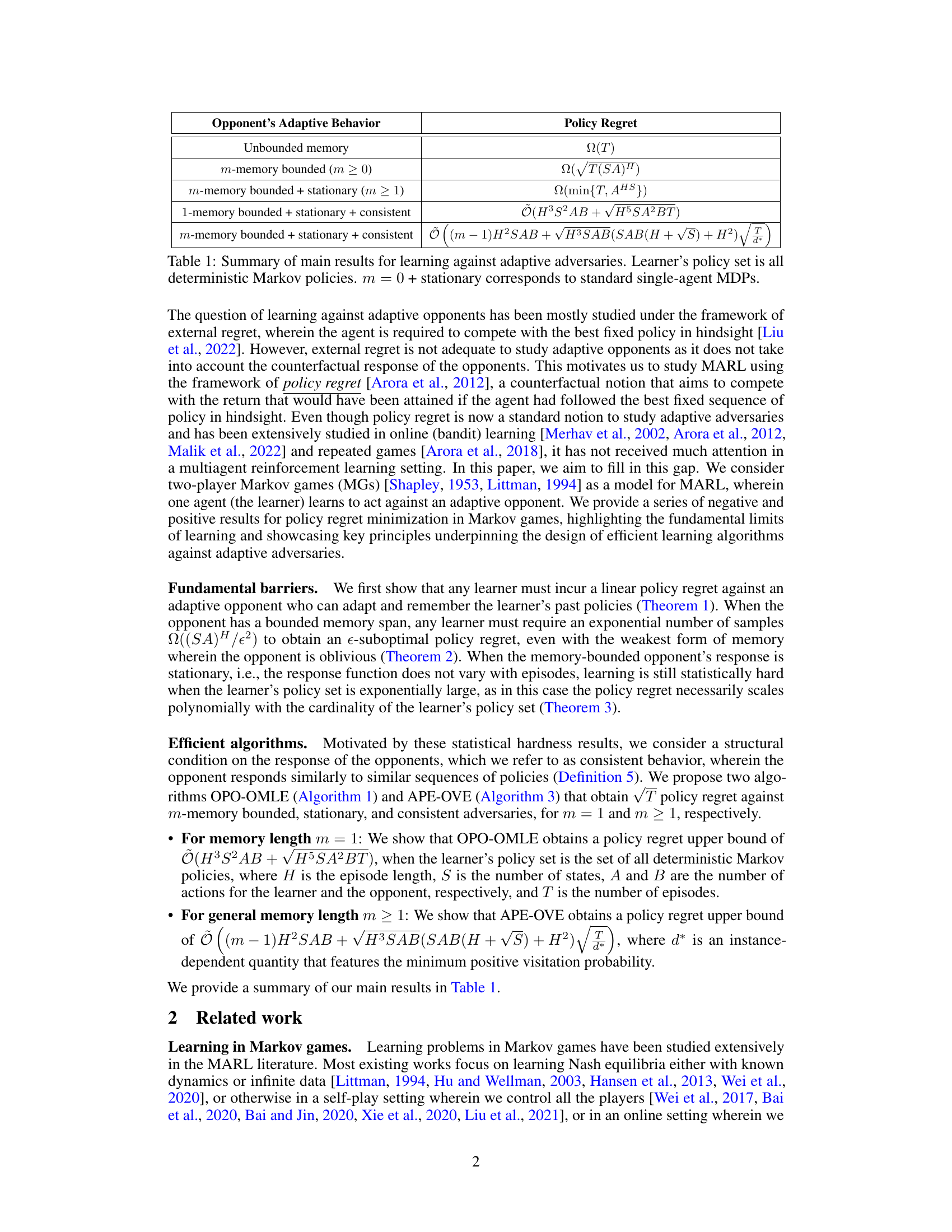
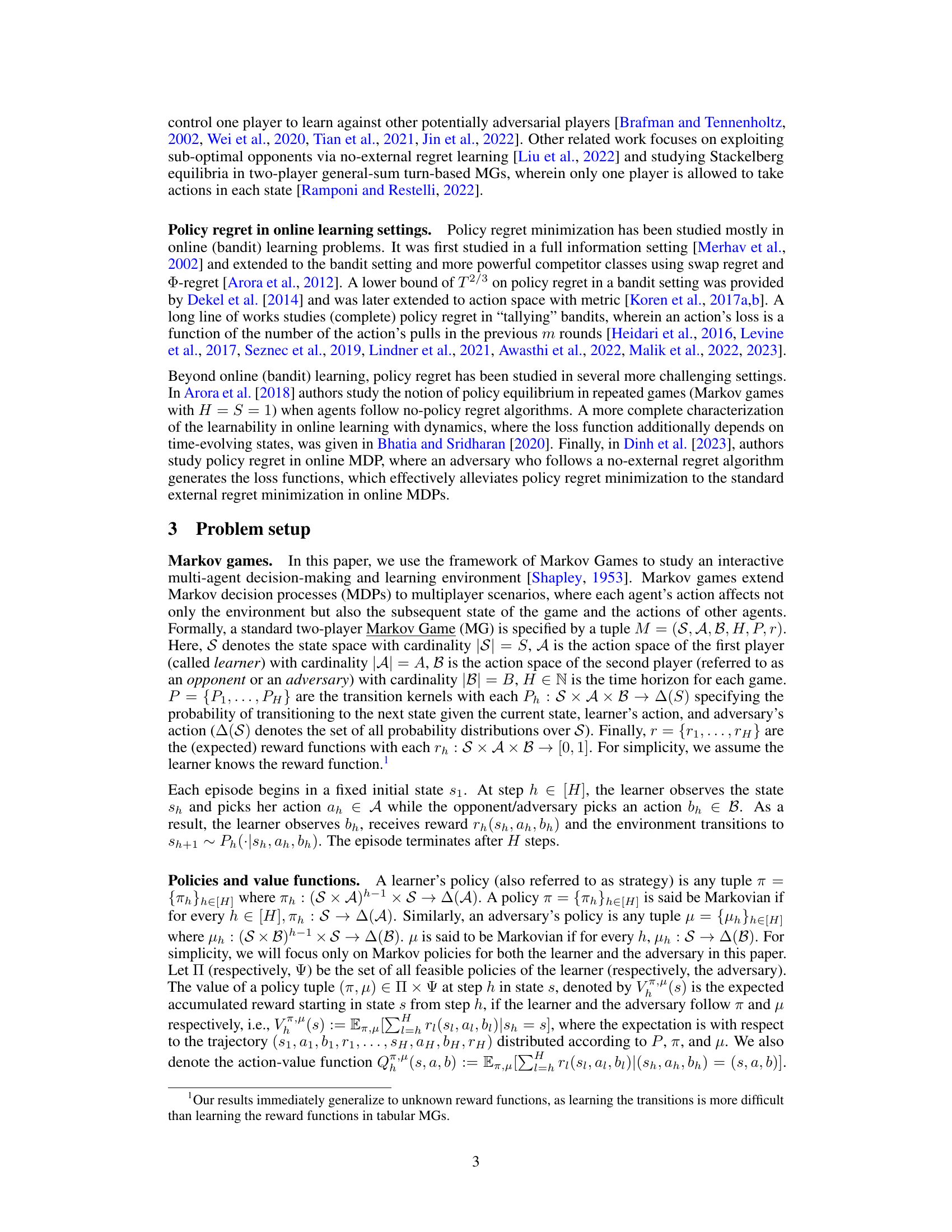
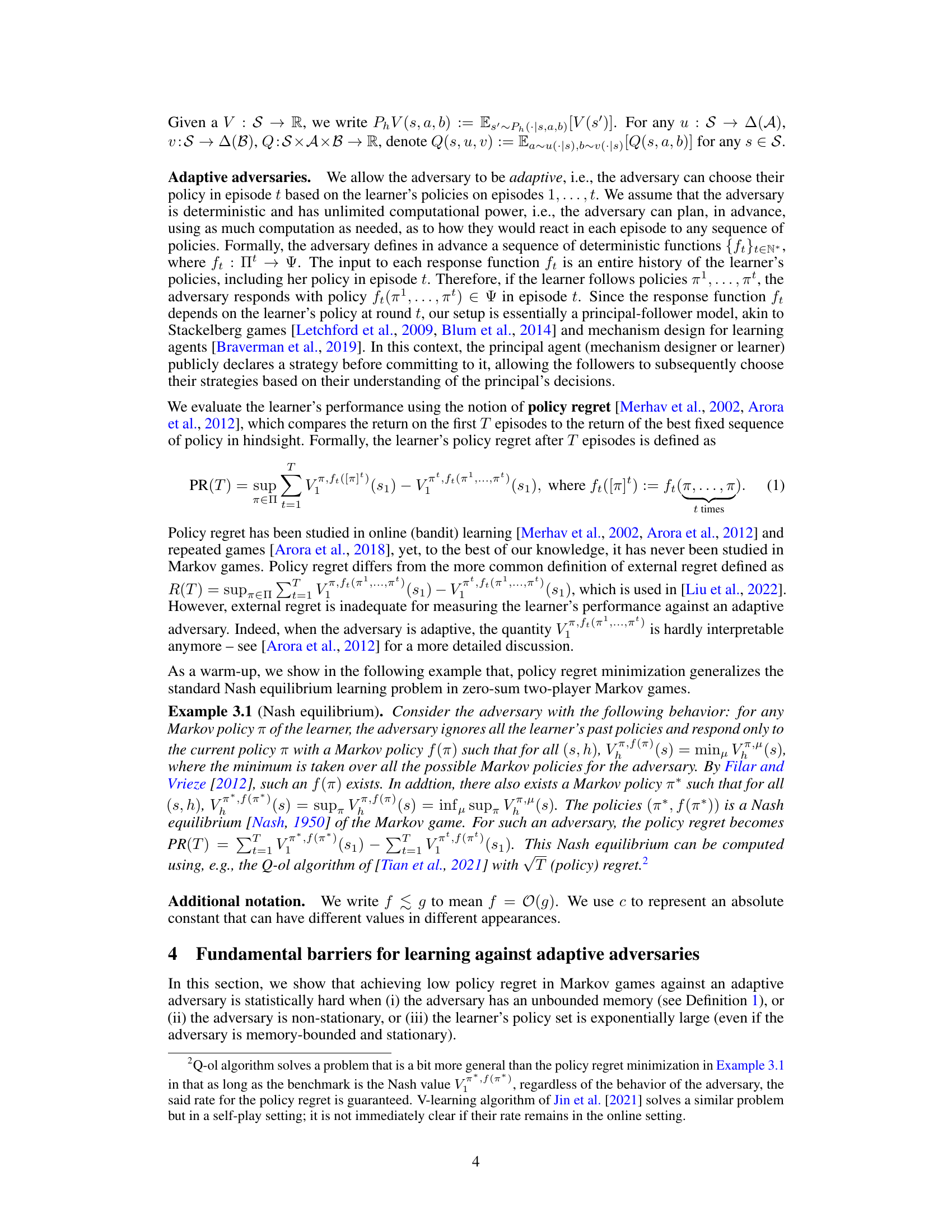
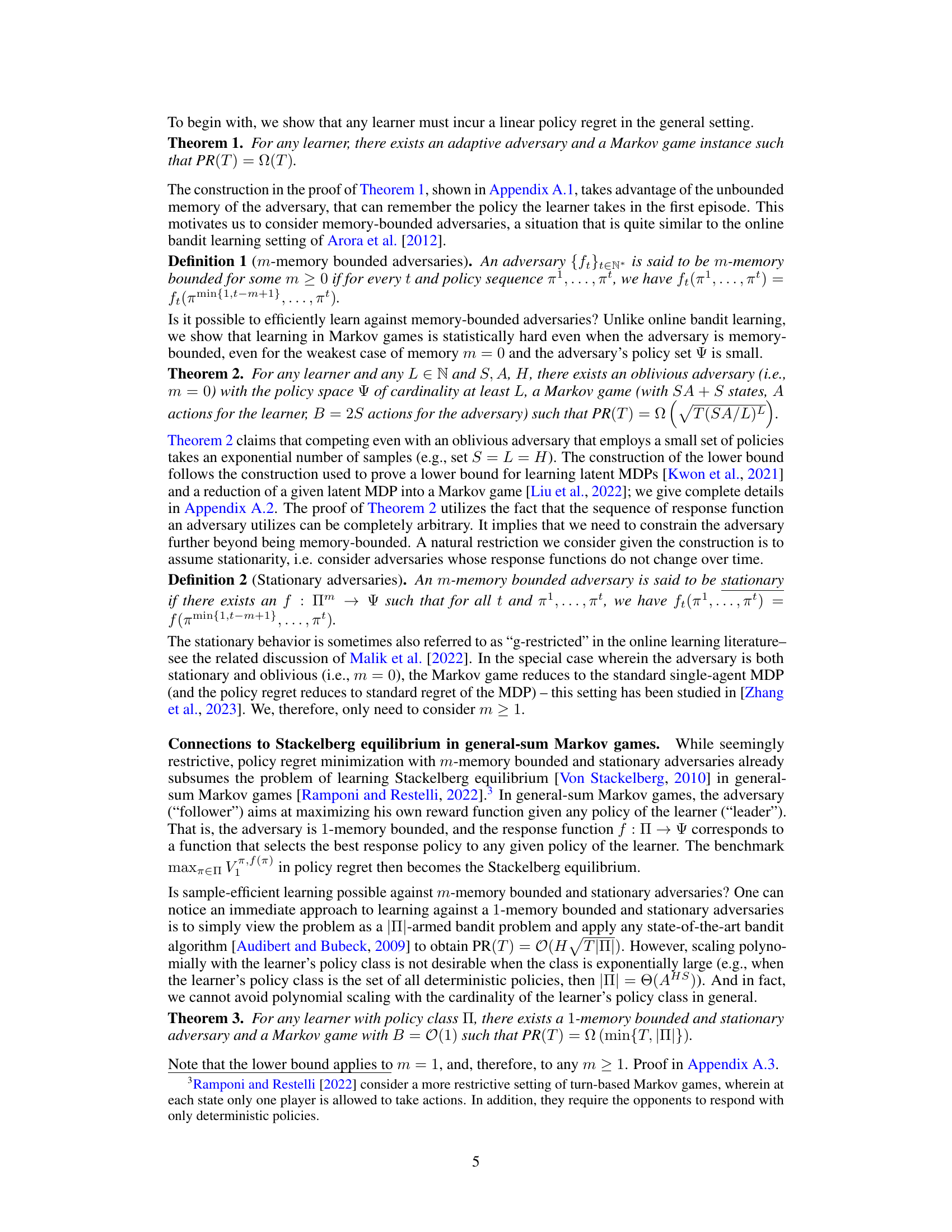
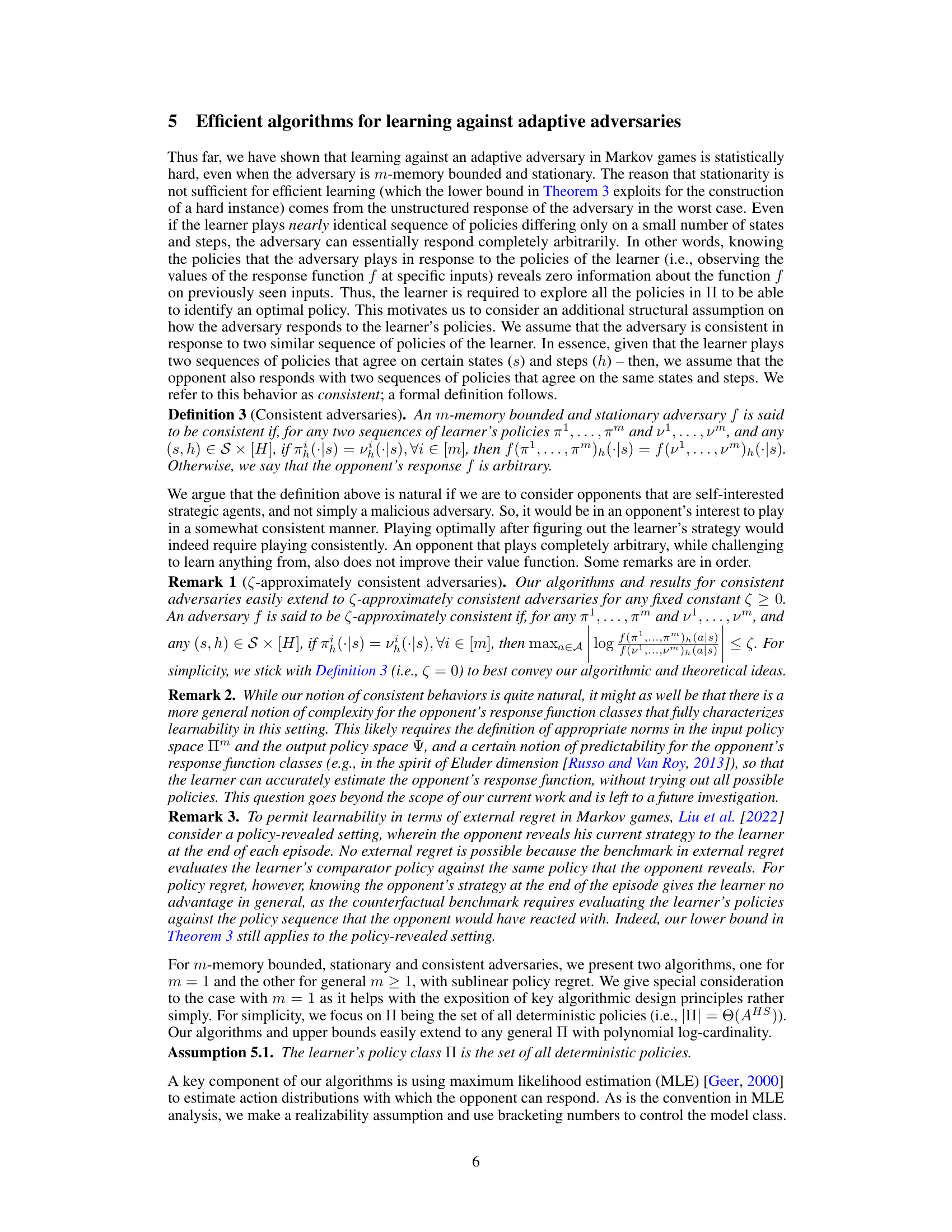
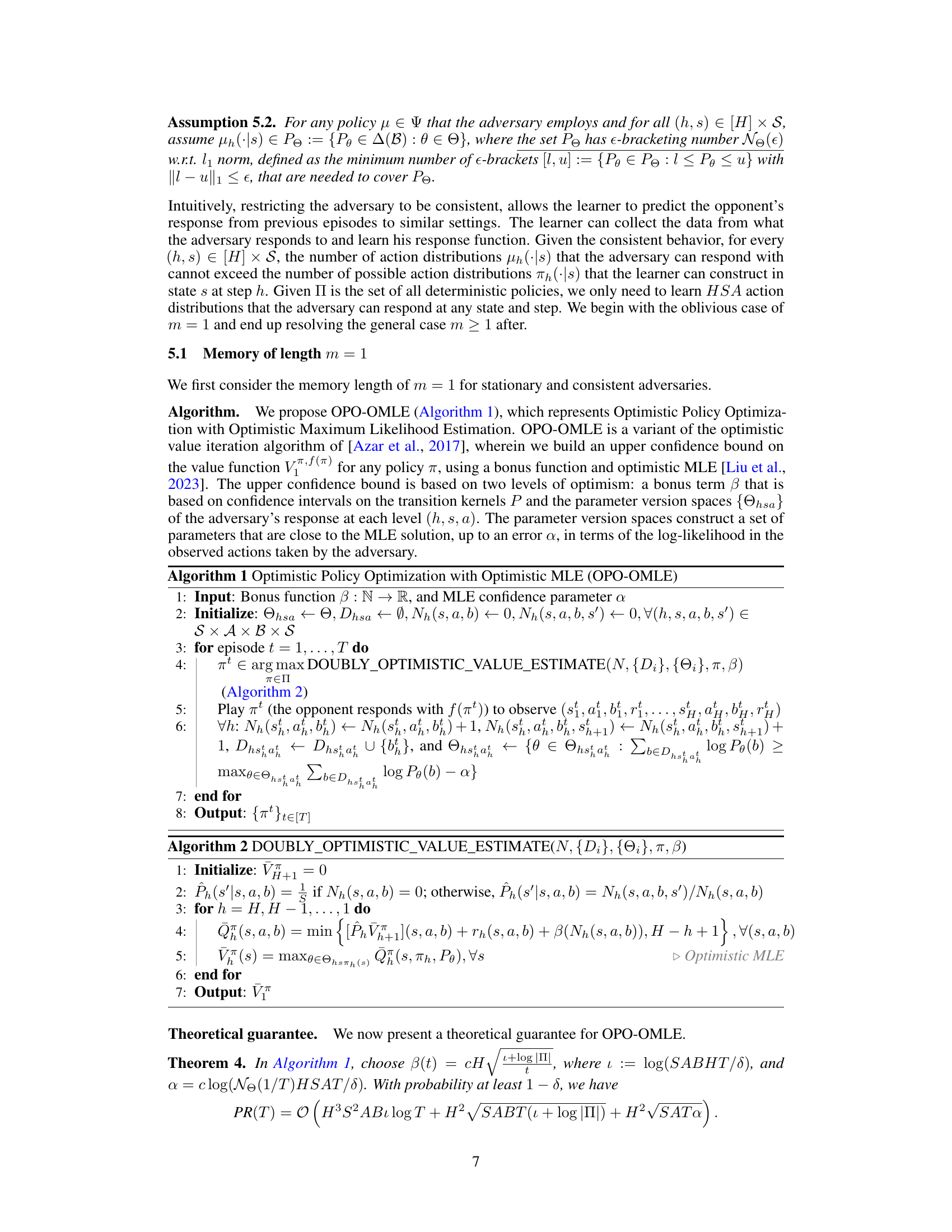
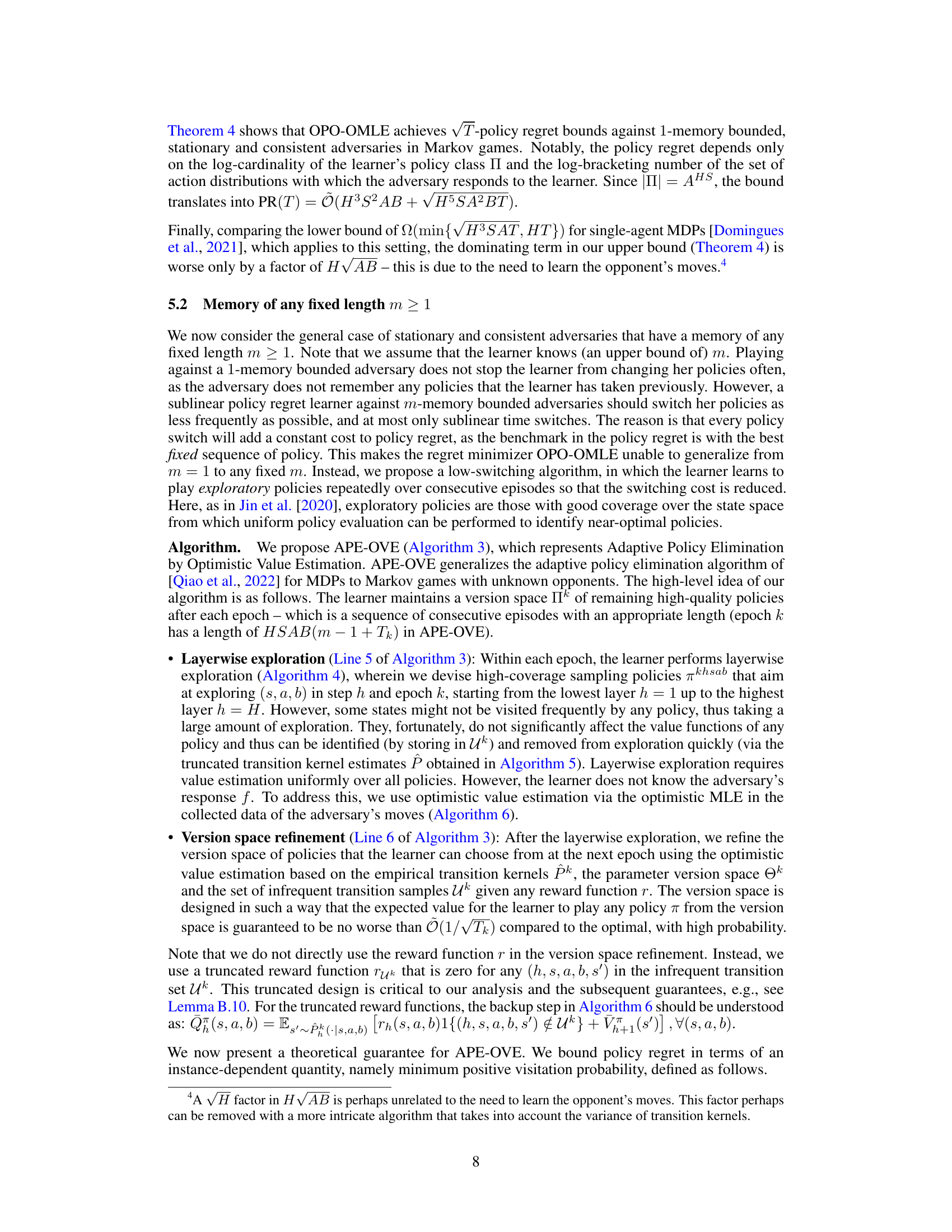
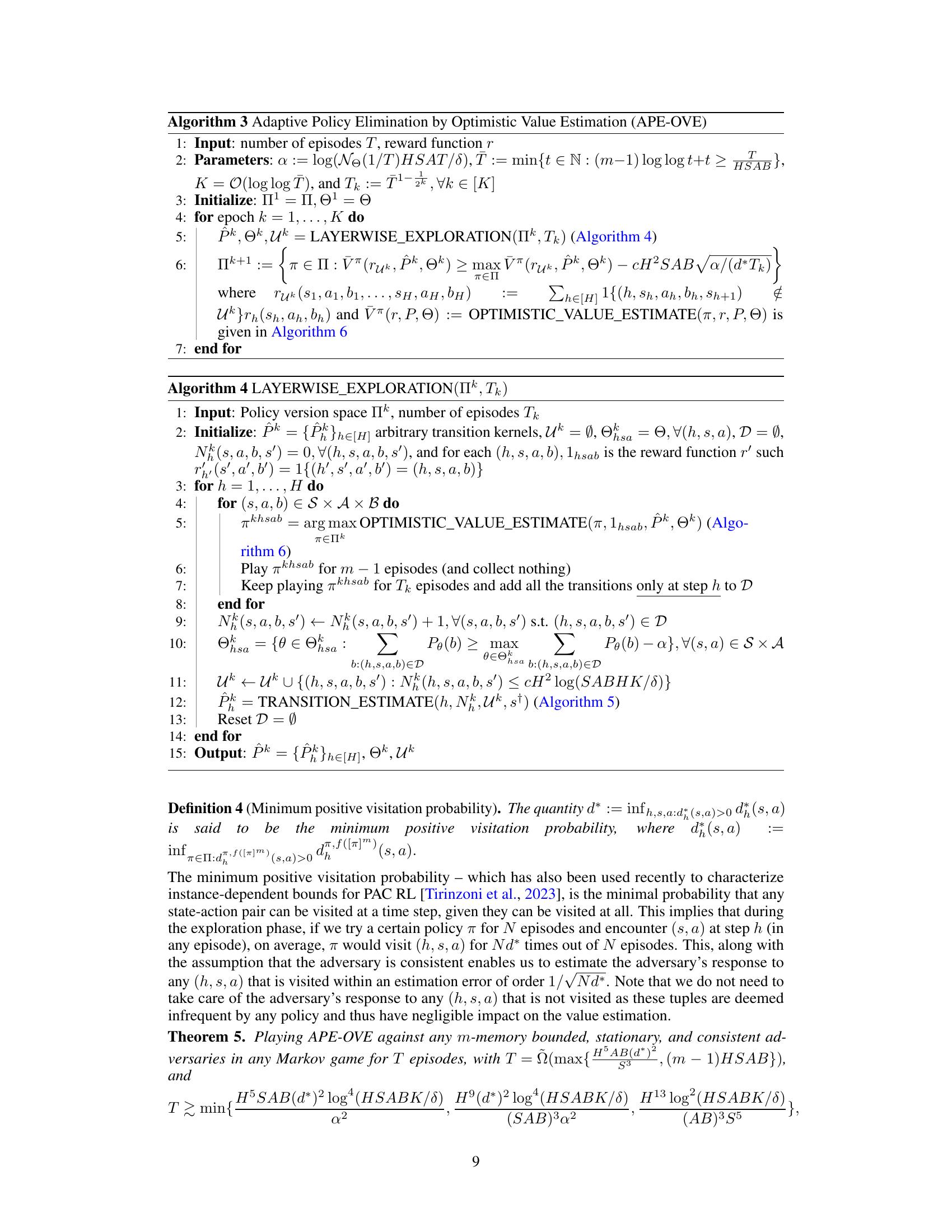
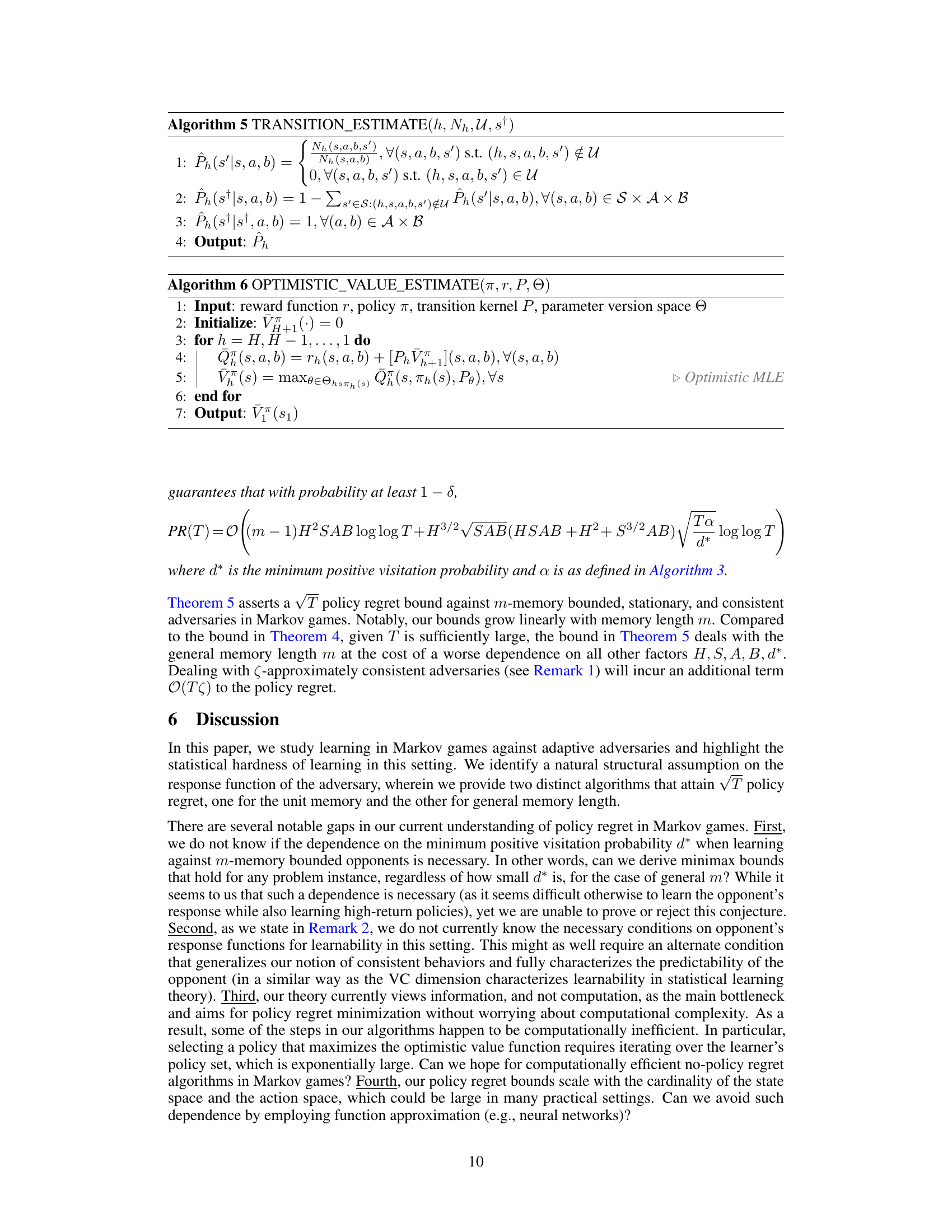
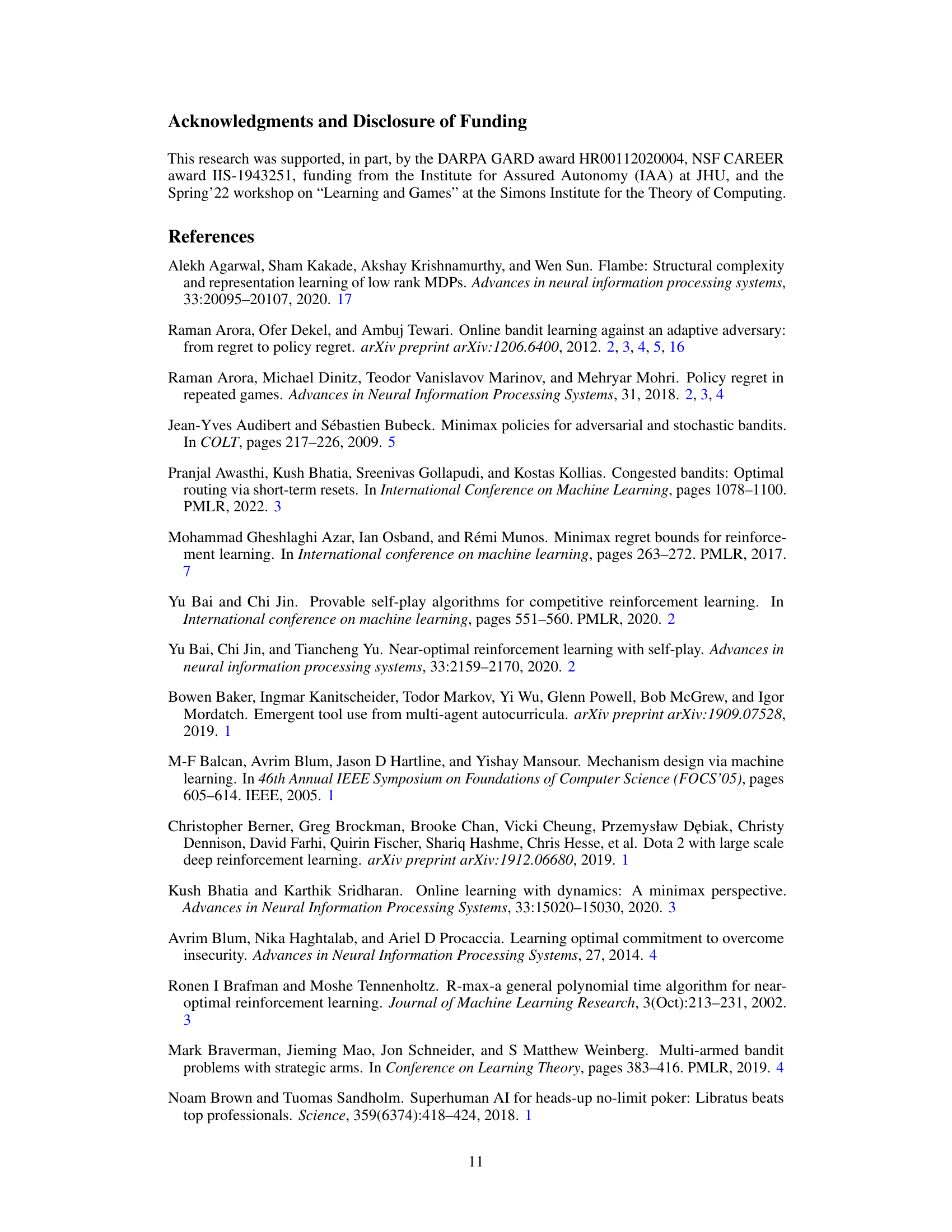
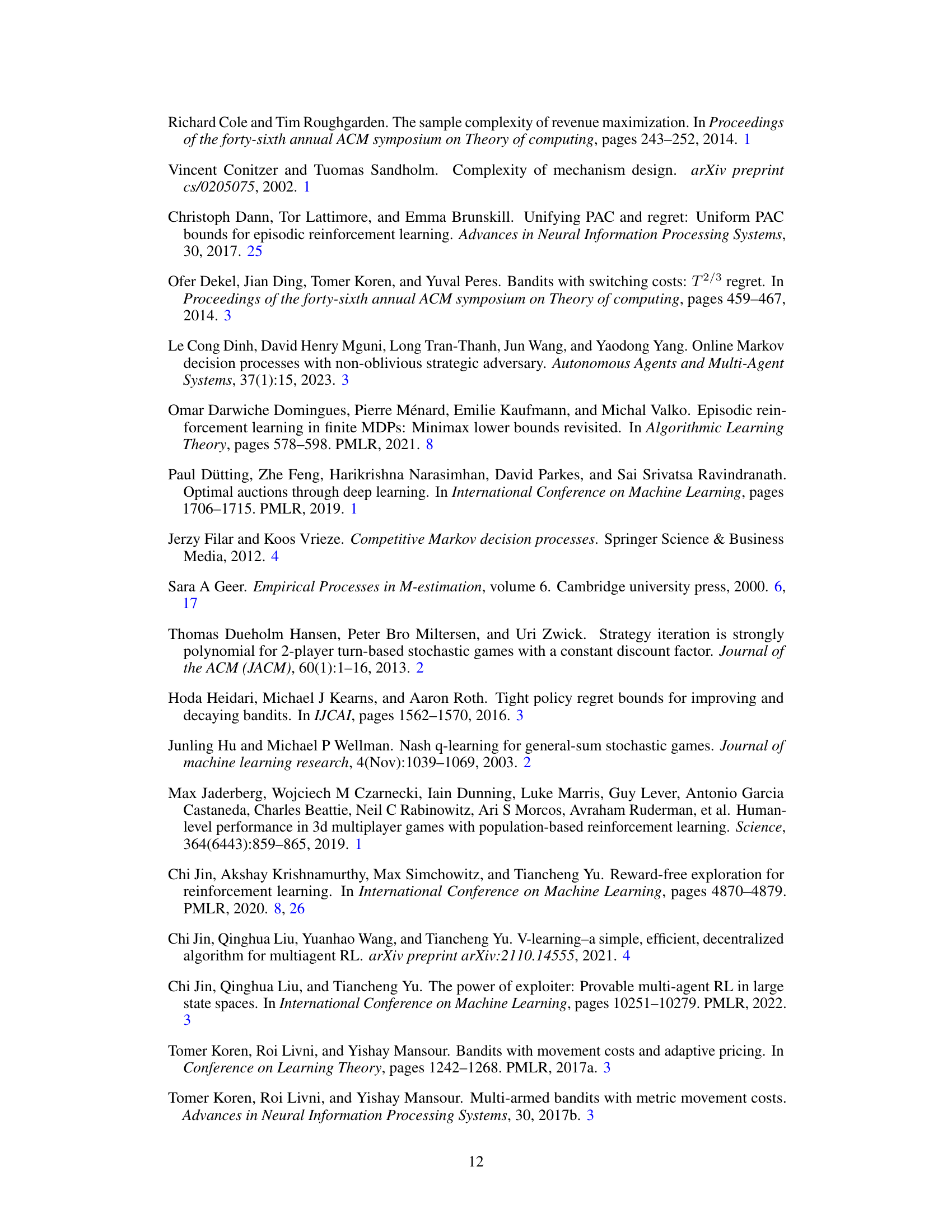
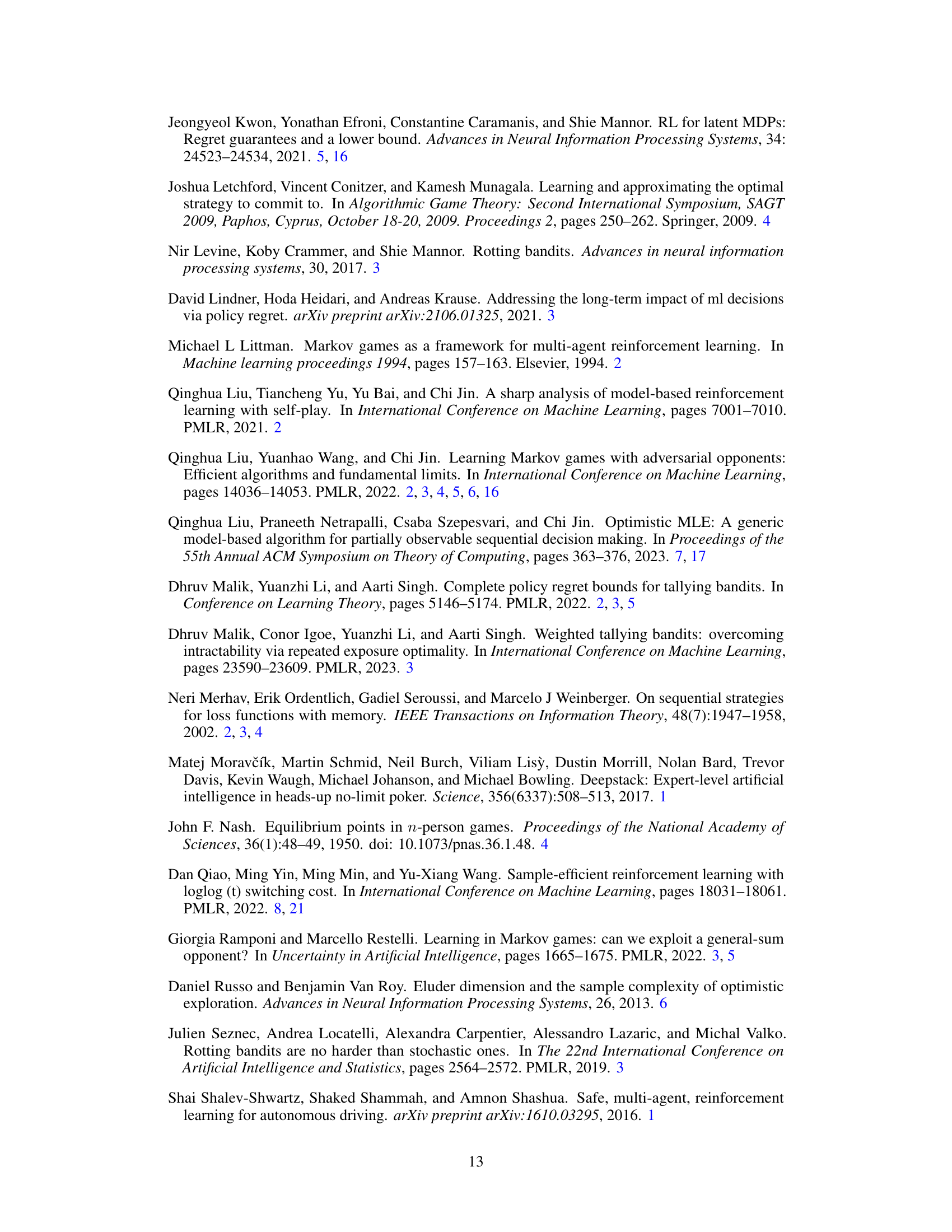
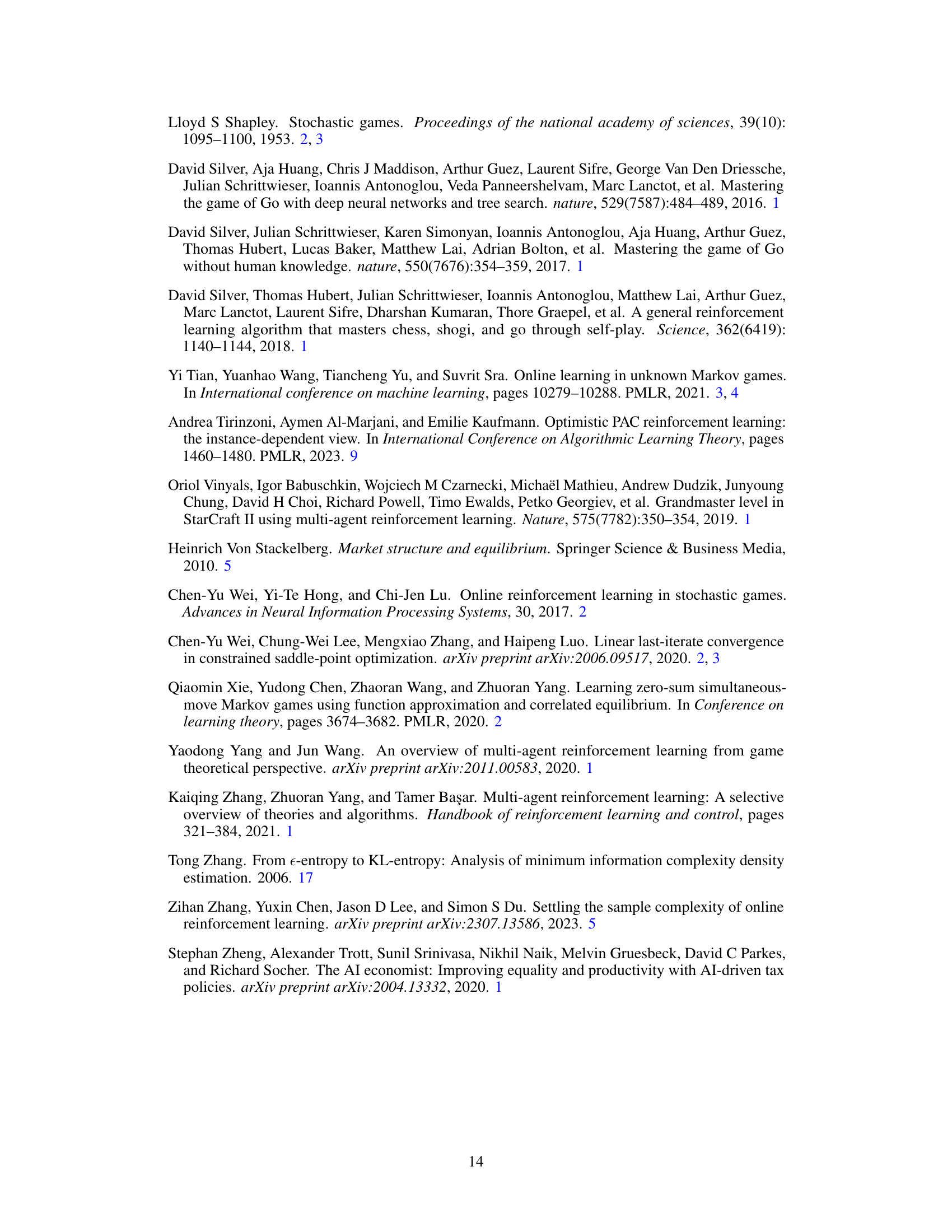
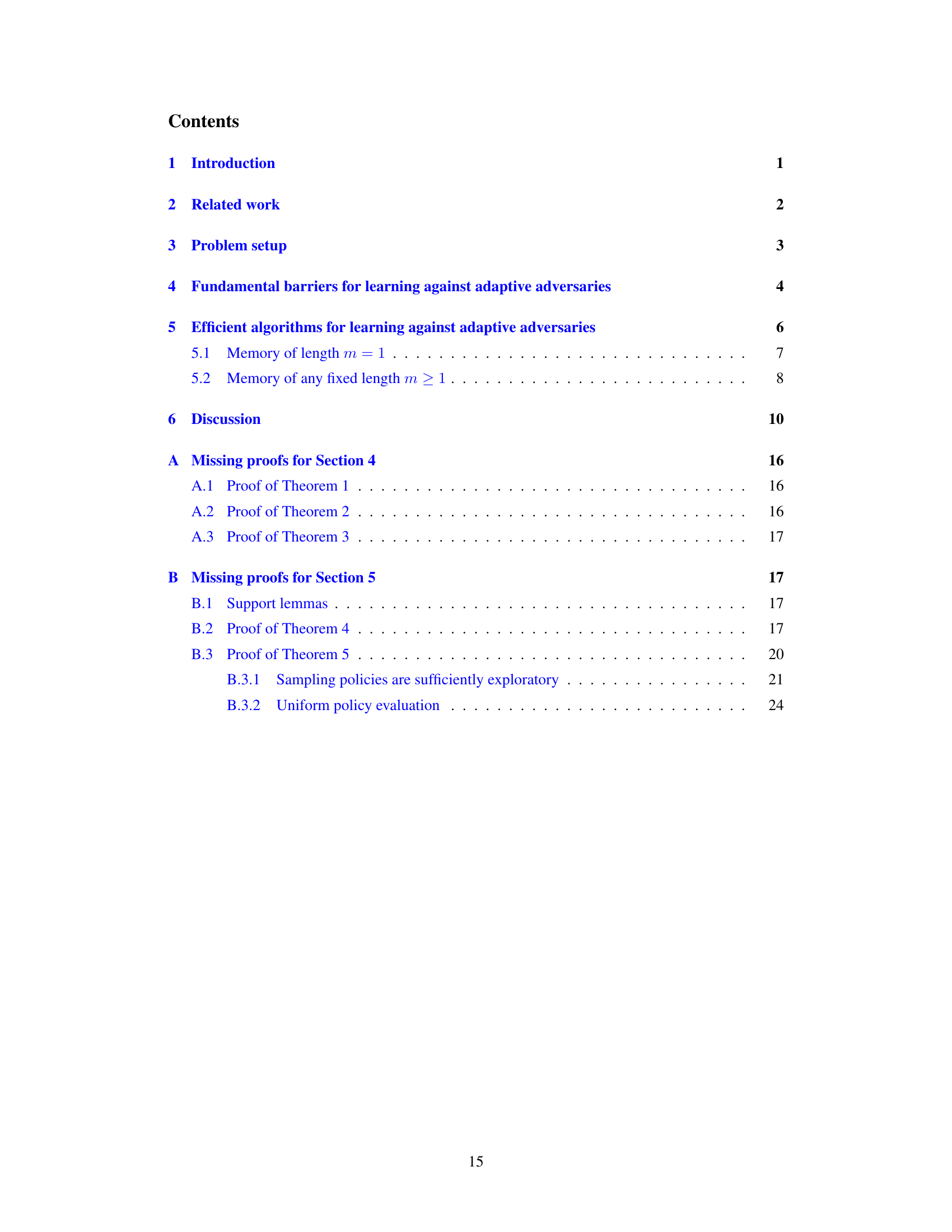
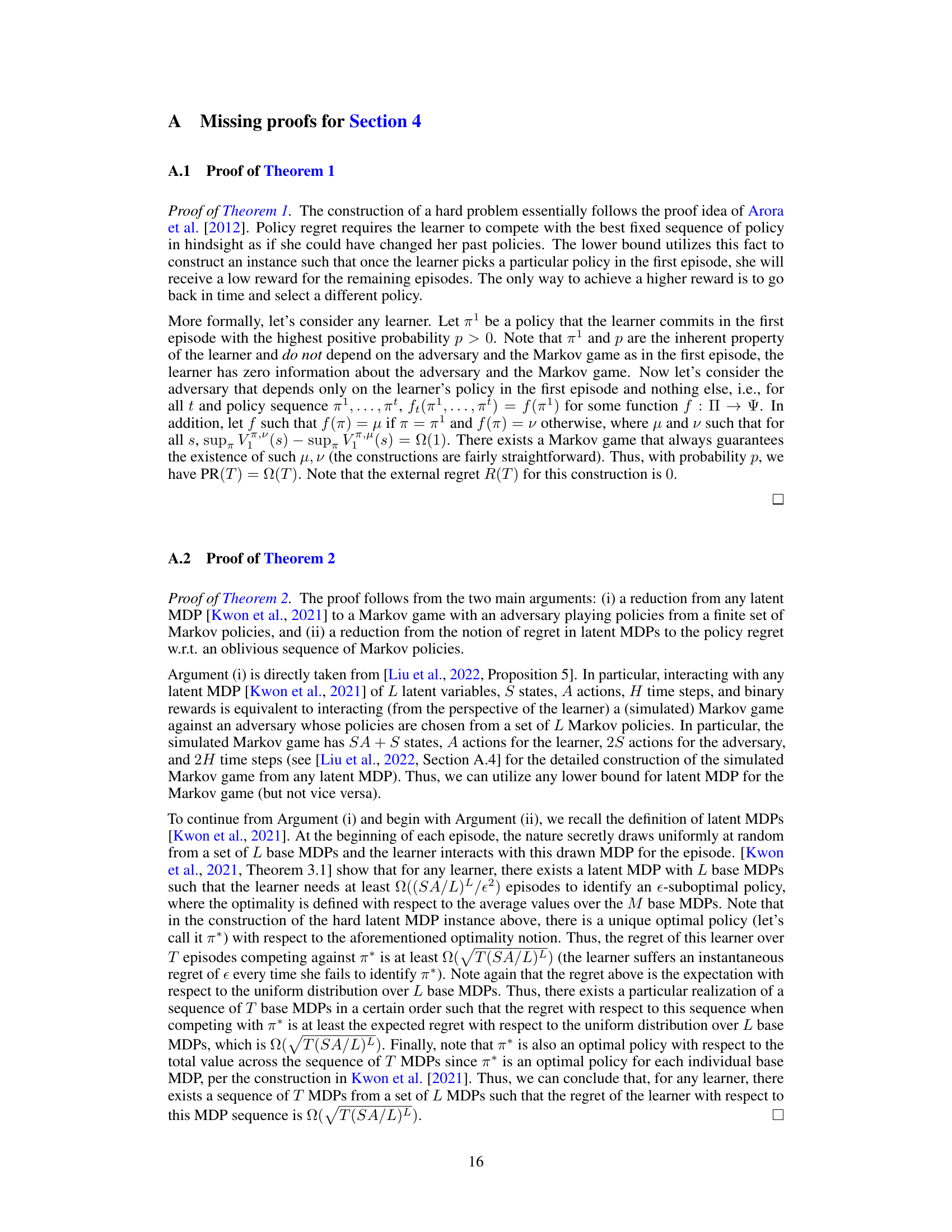
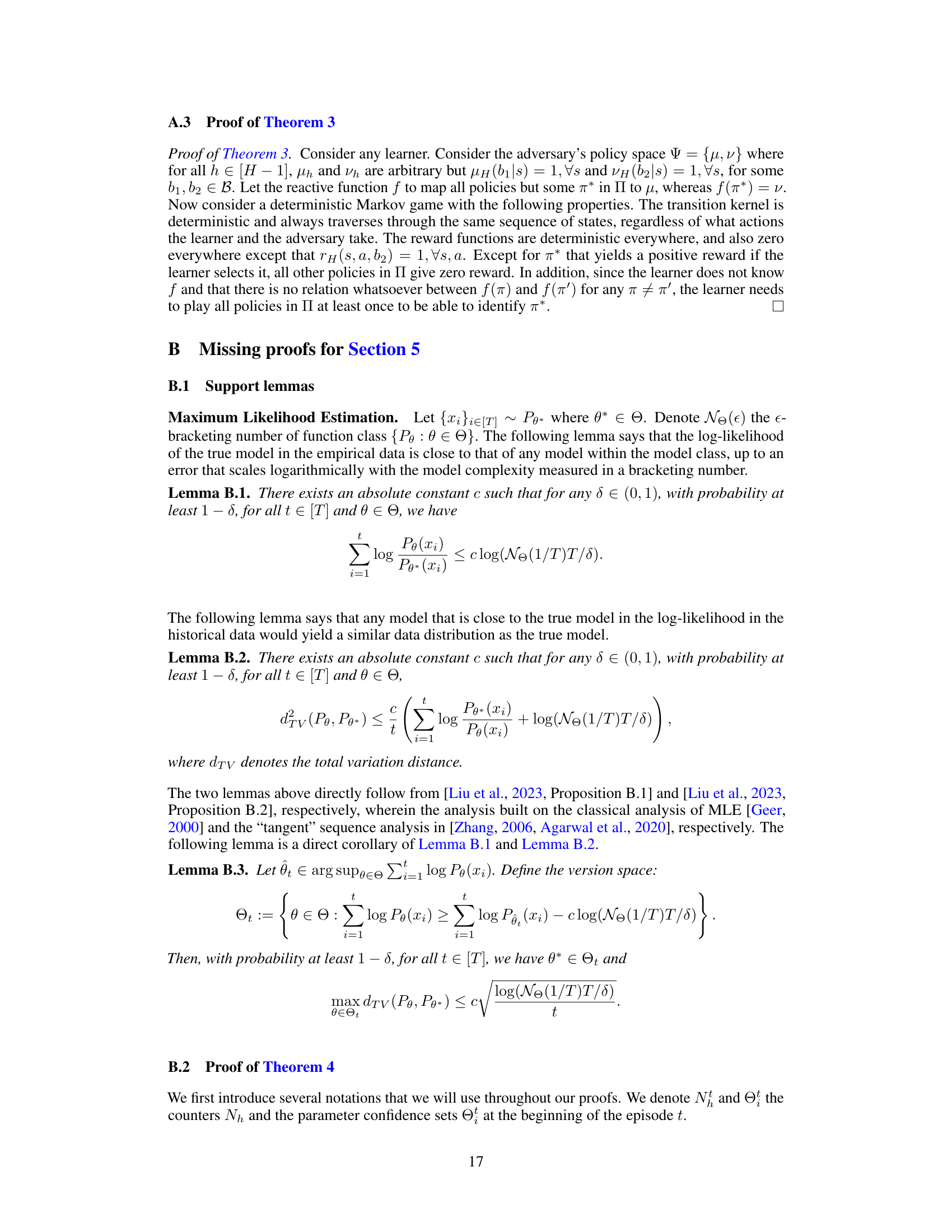
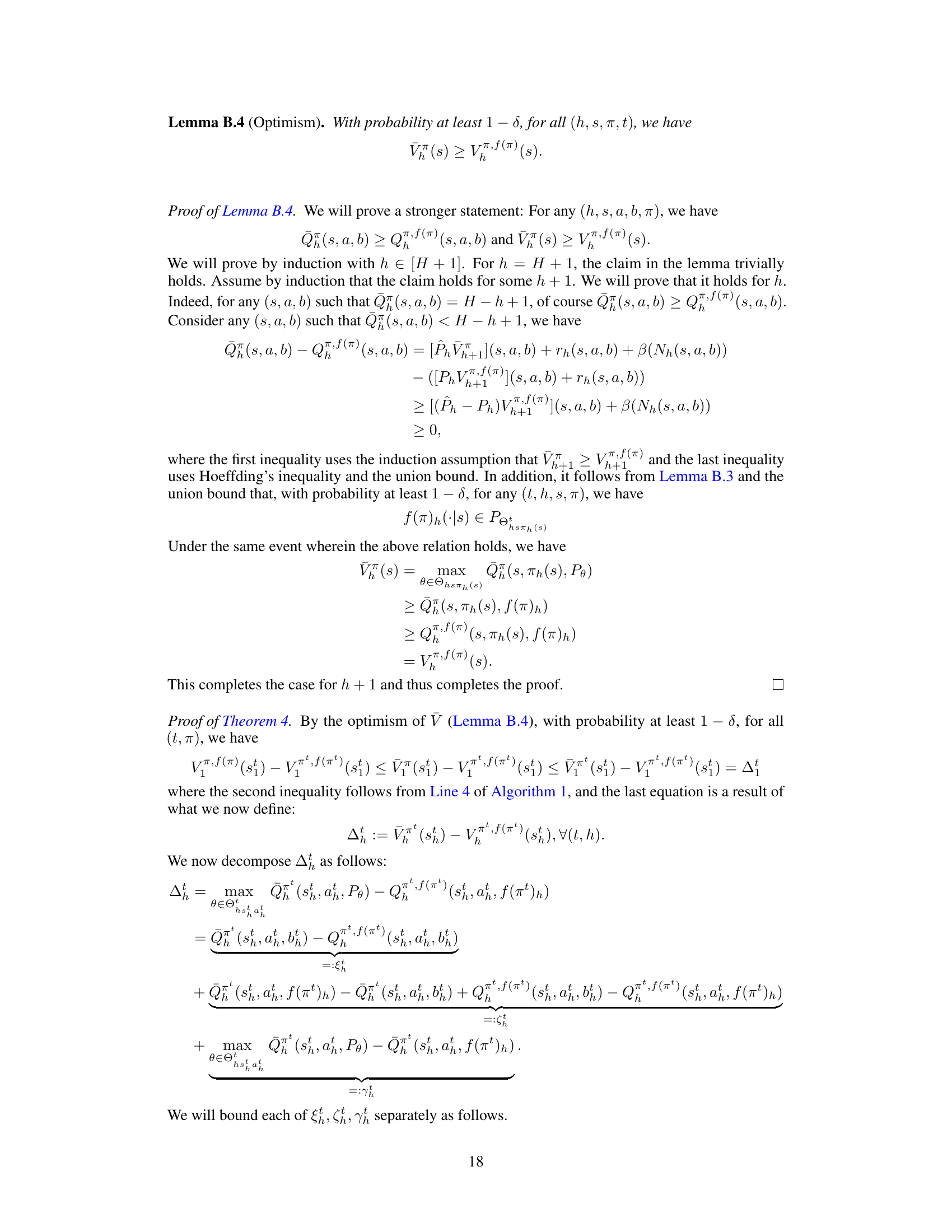
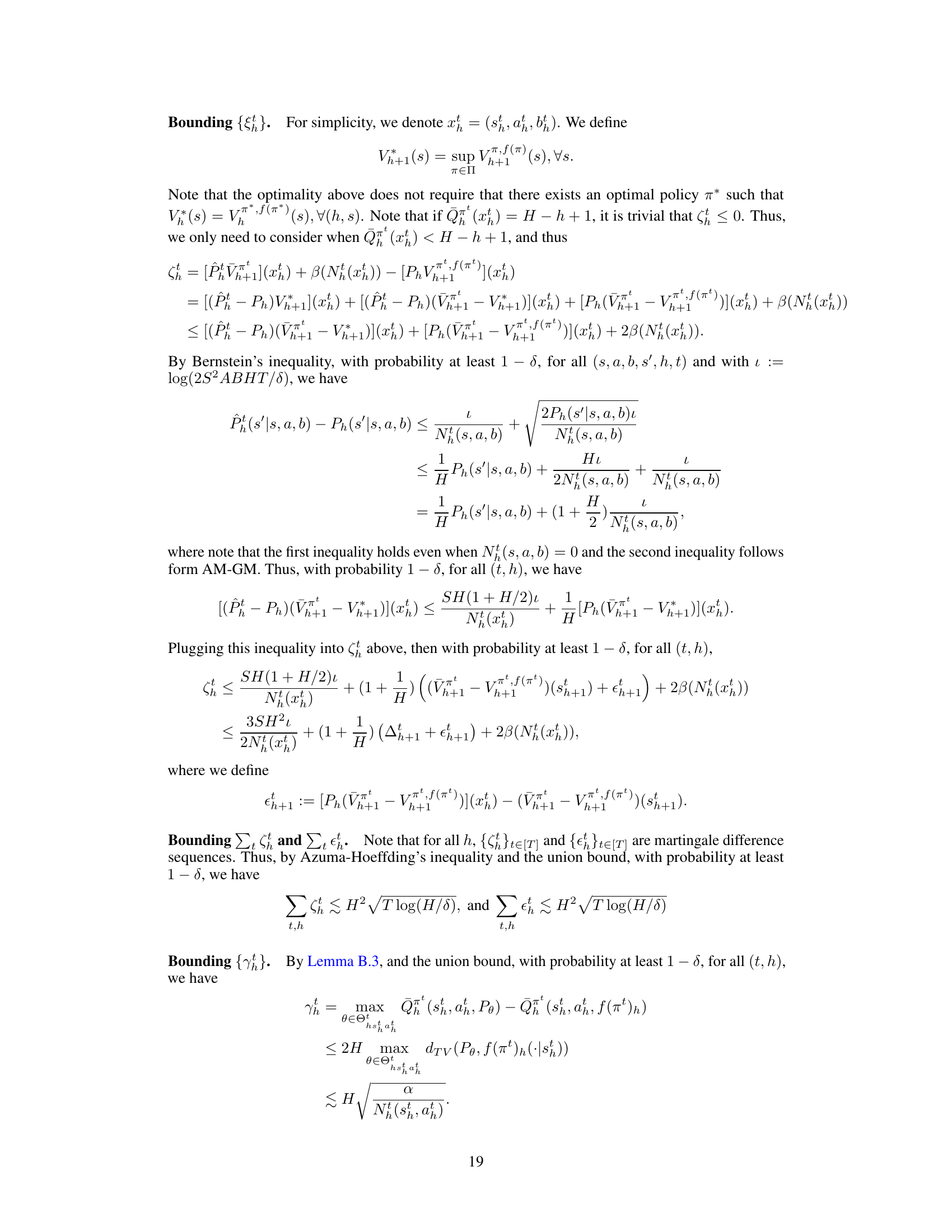
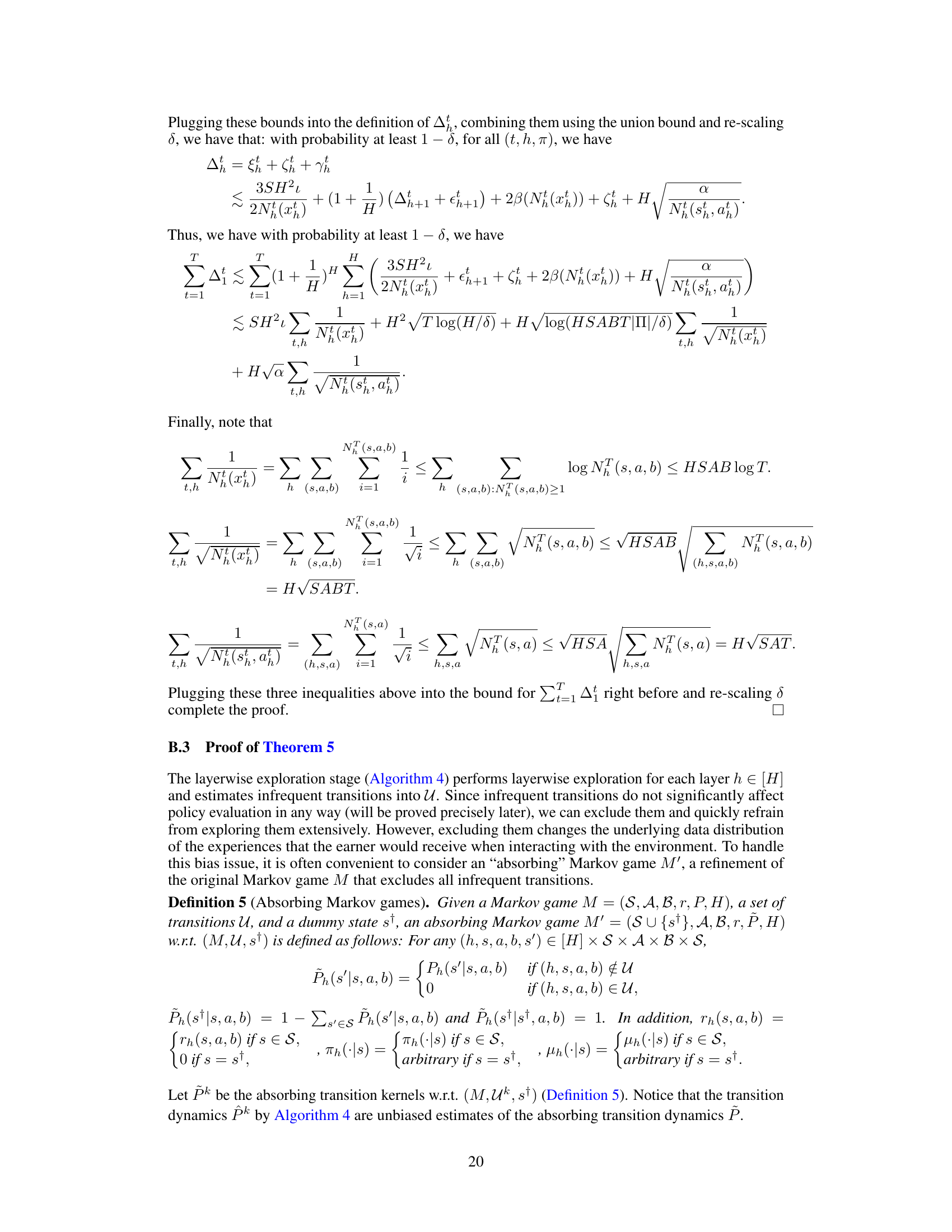