TL;DR#
Transformer models have achieved significant success in various applications, but their theoretical capabilities, particularly when handling numerical data sequences (such as time series), remain not fully understood. Existing research primarily focused on string data, limiting our understanding of their expressive power in broader contexts. This paper aims to address this gap by exploring the capabilities of transformer models on numerical sequences and providing a more comprehensive theoretical analysis.
The study investigates the expressive power of ‘Unique Hard Attention Transformers’ (UHATs) over data sequences. The researchers prove that UHATs over data sequences are more powerful than those processing string data, going beyond regular languages. They connect UHATs to circuit complexity classes (TCâ° and ACâ°), revealing a higher computational capacity over numerical data. Furthermore, they introduce a new temporal logic to precisely characterize the languages recognized by these models on data sequences. This comprehensive analysis significantly expands our understanding of transformer capabilities and provides valuable insights for future model development and applications.
Key Takeaways#
Why does it matter?#
This paper is crucial for researchers in AI and formal language theory. It bridges the gap between theoretical understanding and practical applications of transformer models, particularly for non-string data like time series. By establishing connections to circuit complexity and introducing a new logical language, it opens exciting new avenues for research on transformer expressiveness and design. This work provides a strong foundation for future advancements in the design of more powerful and efficient transformer architectures.
Visual Insights#
Full paper#
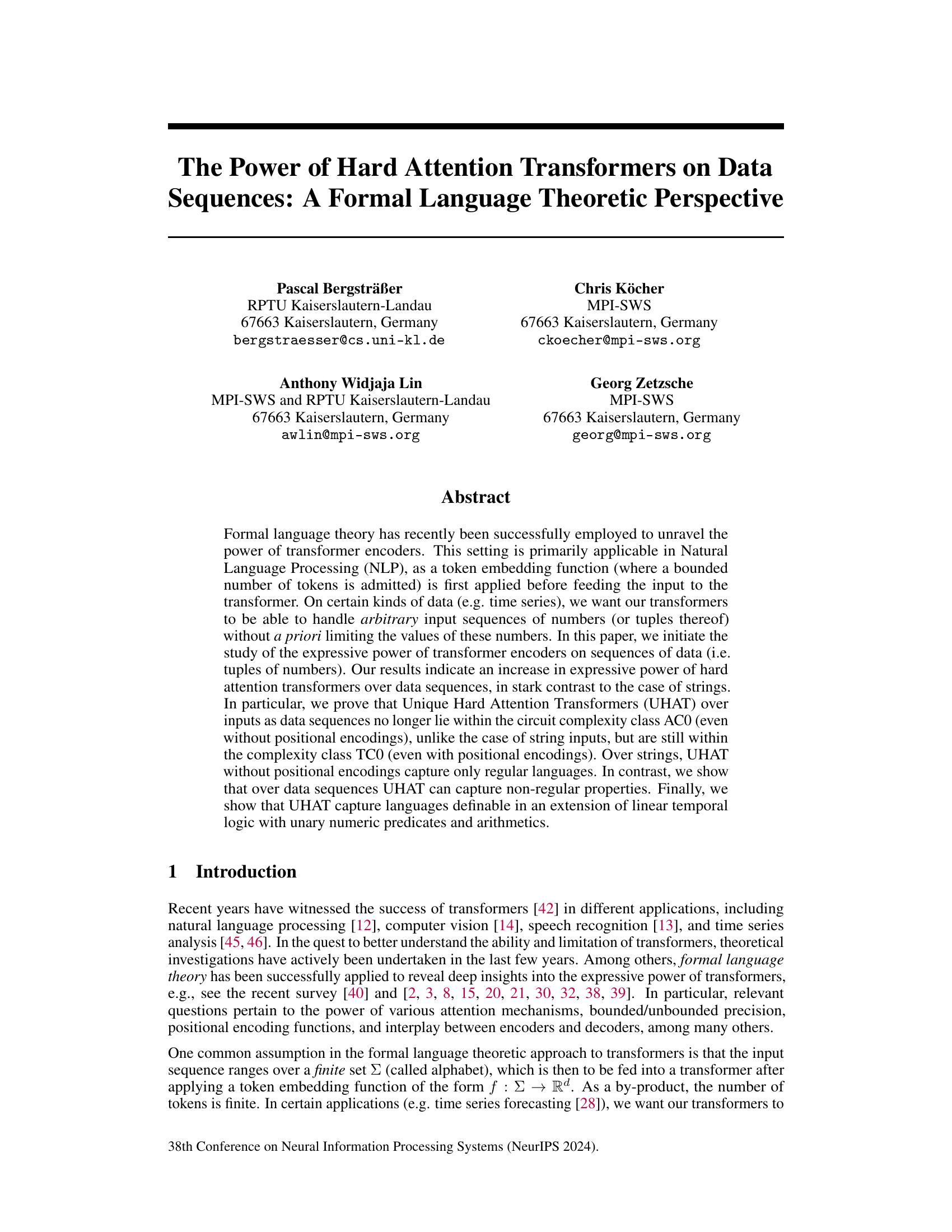
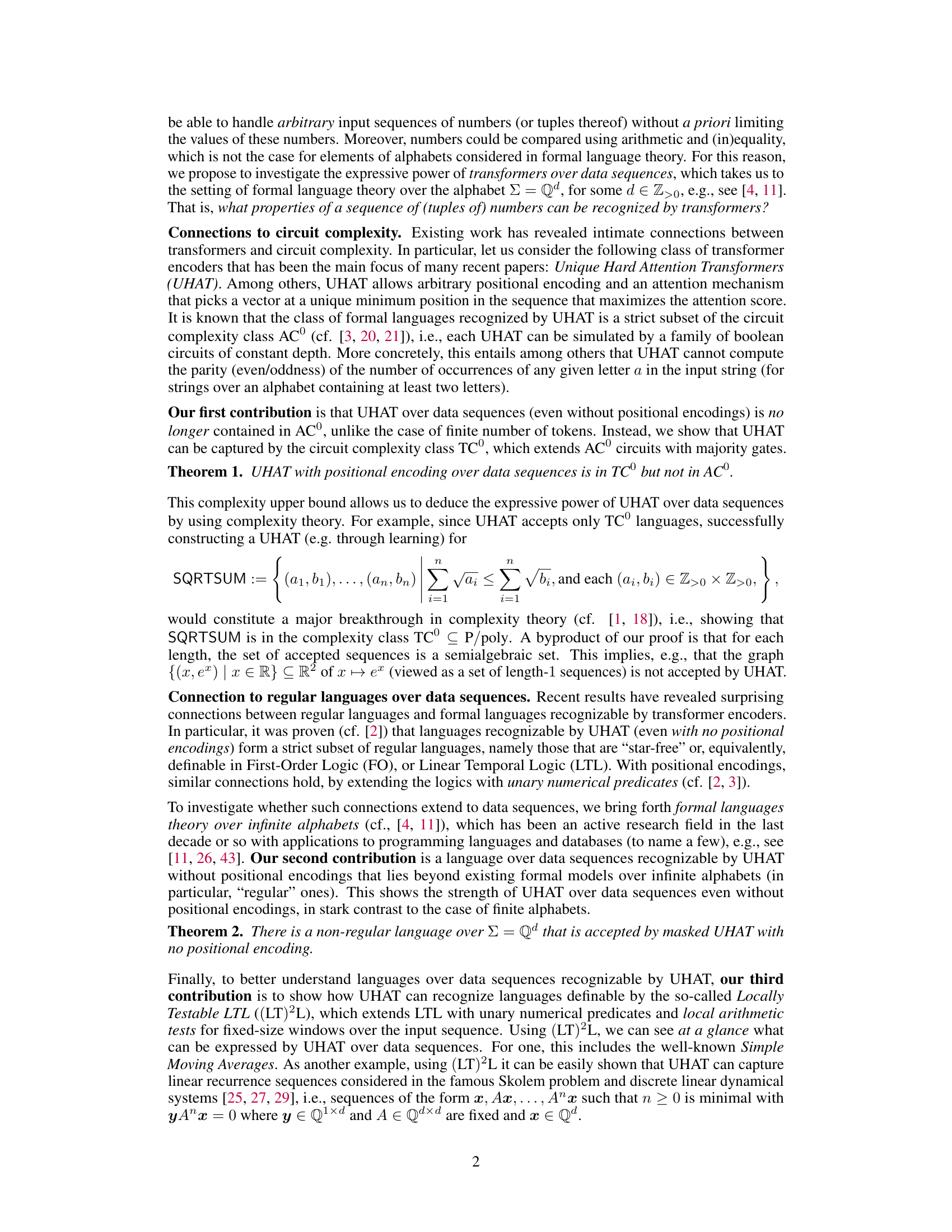
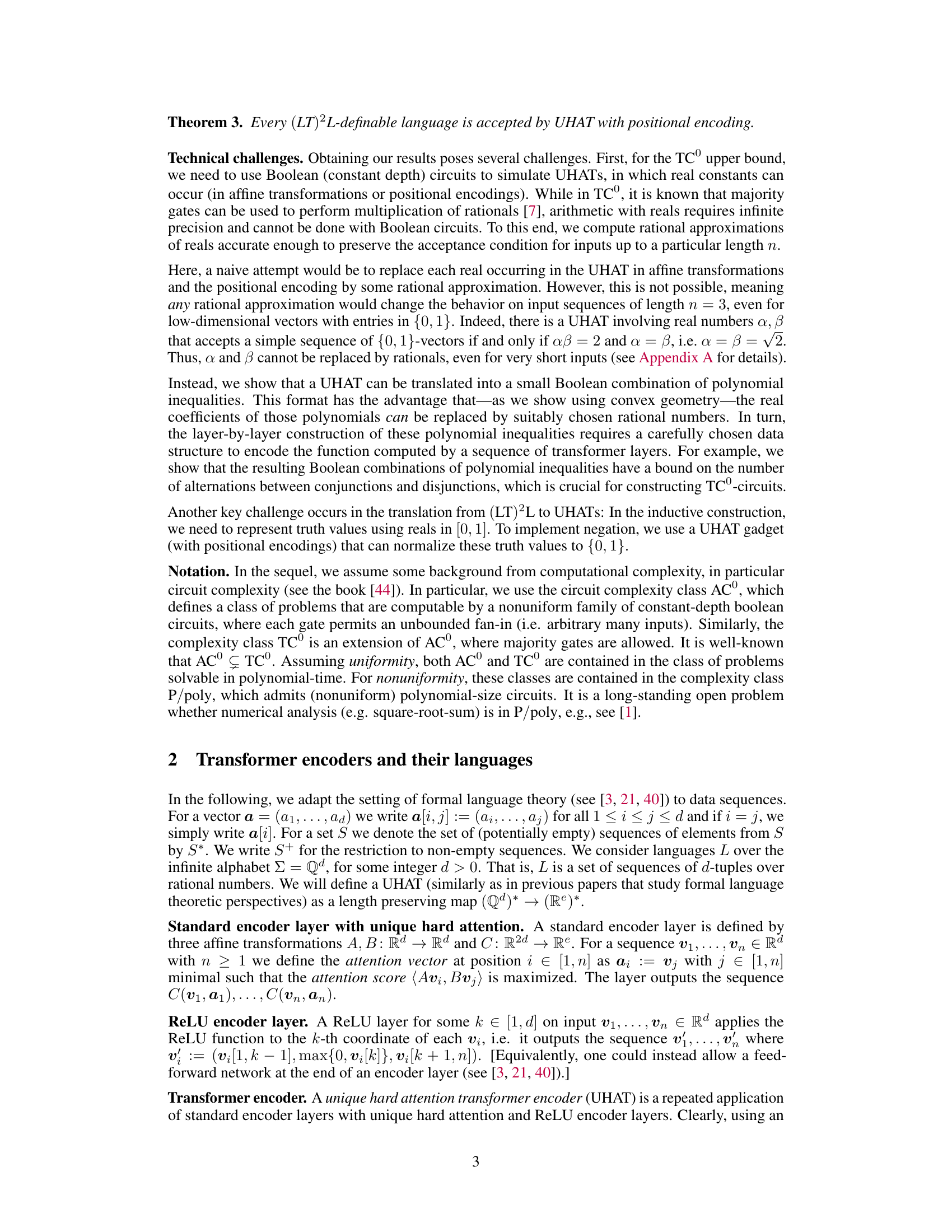
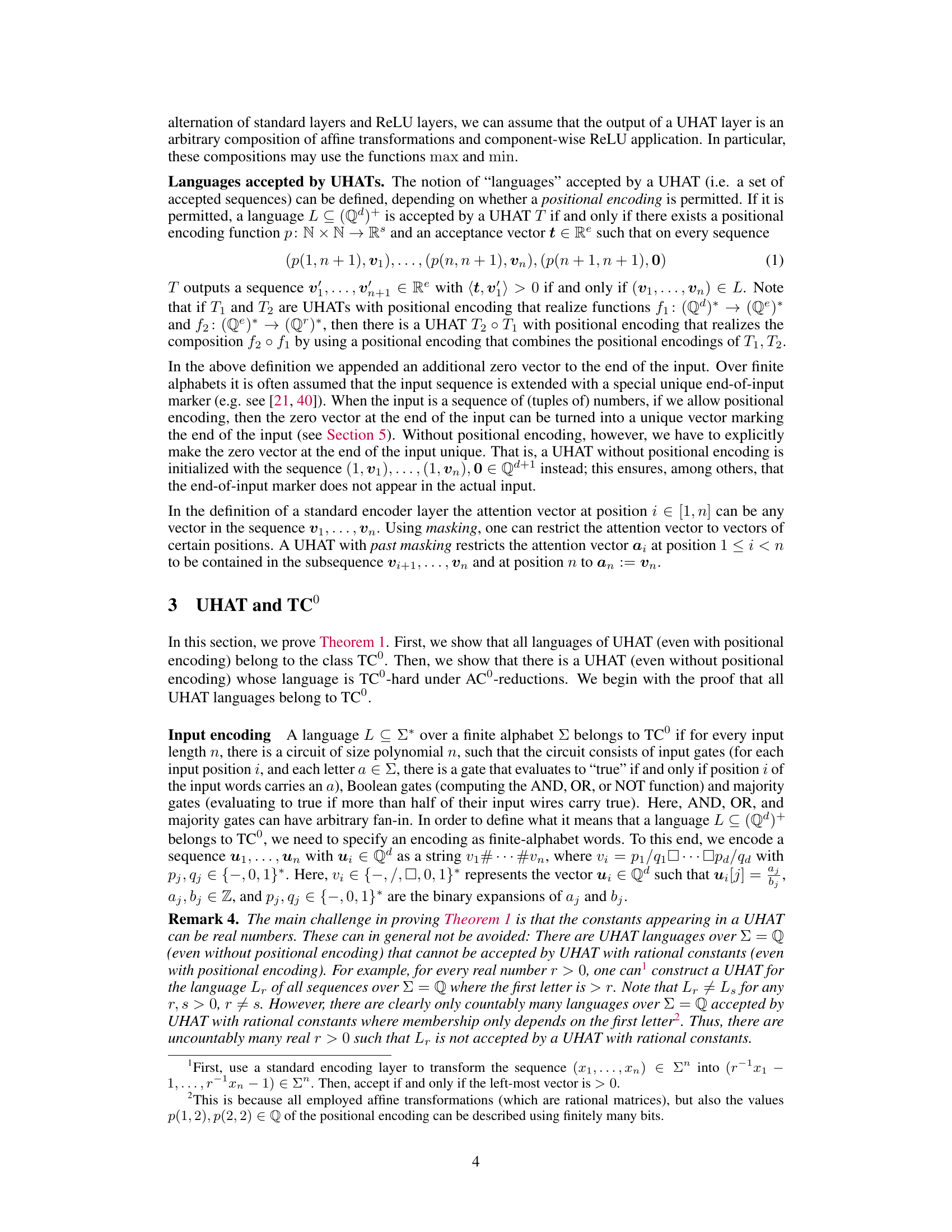
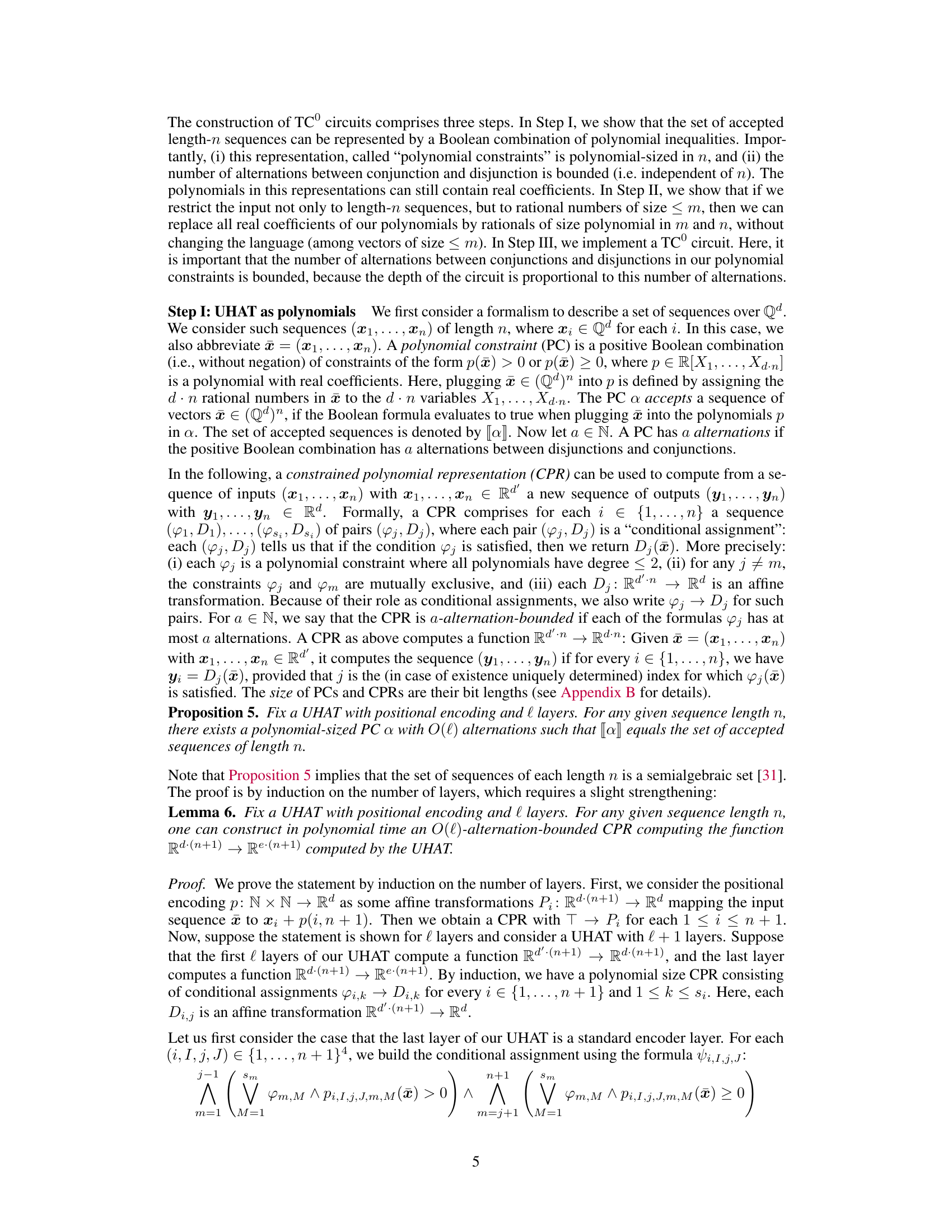
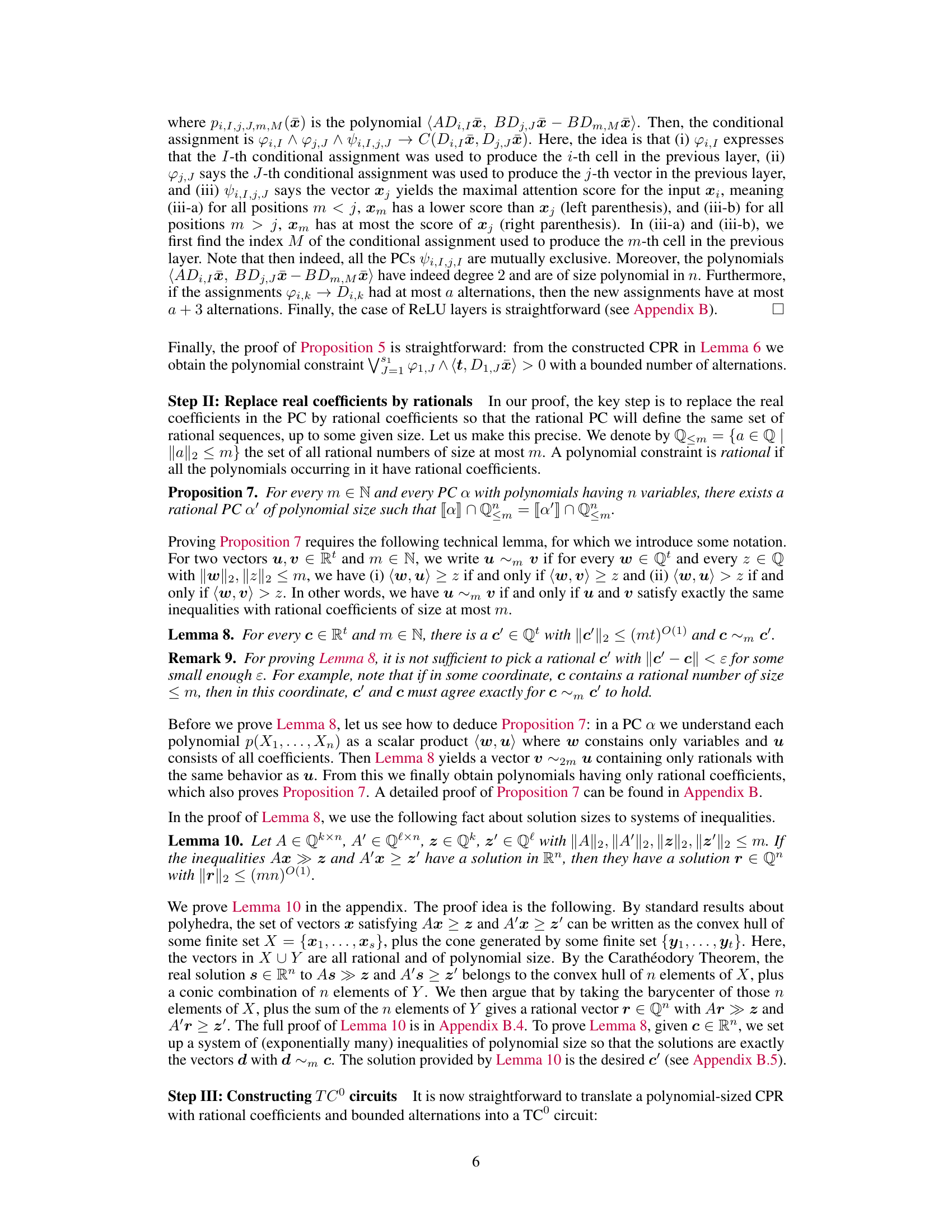
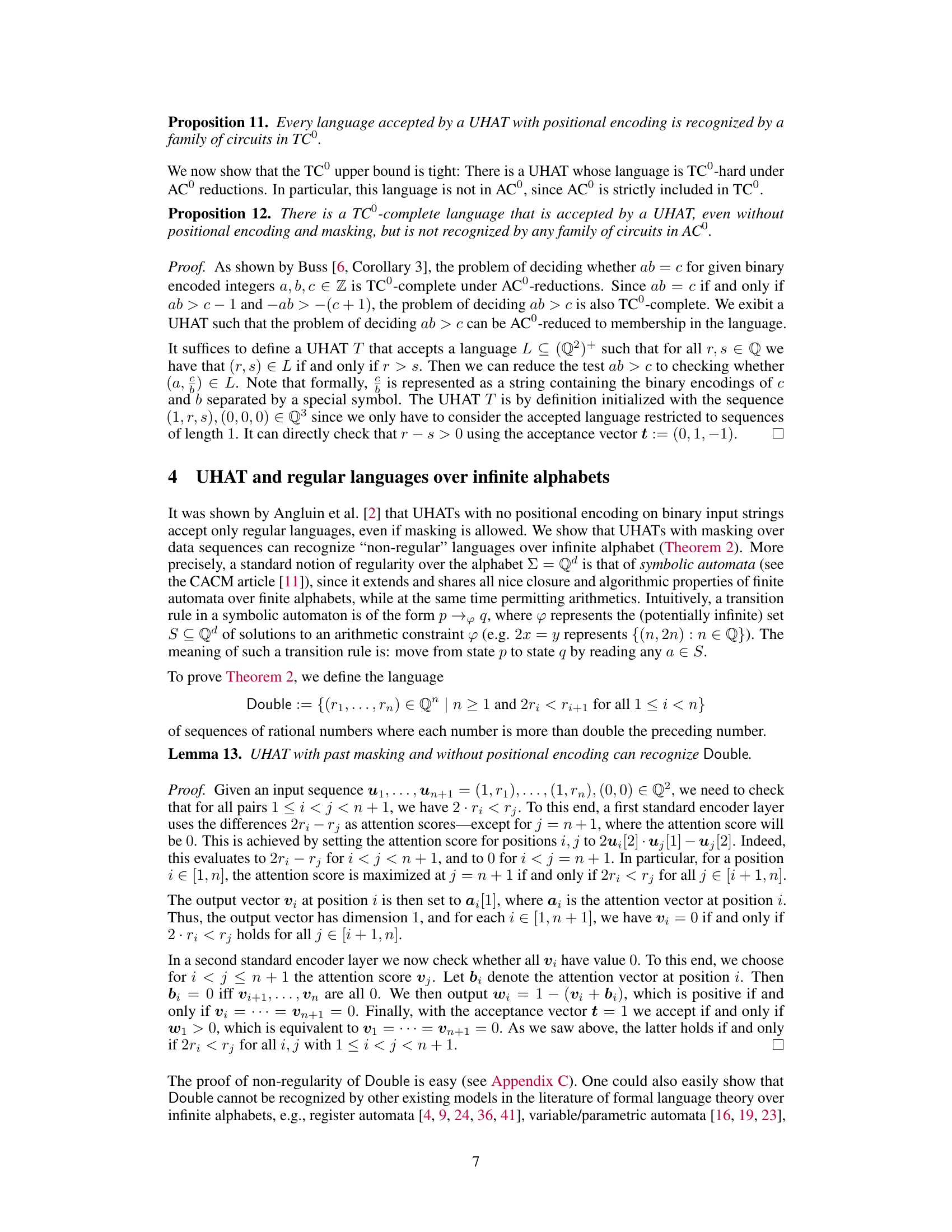
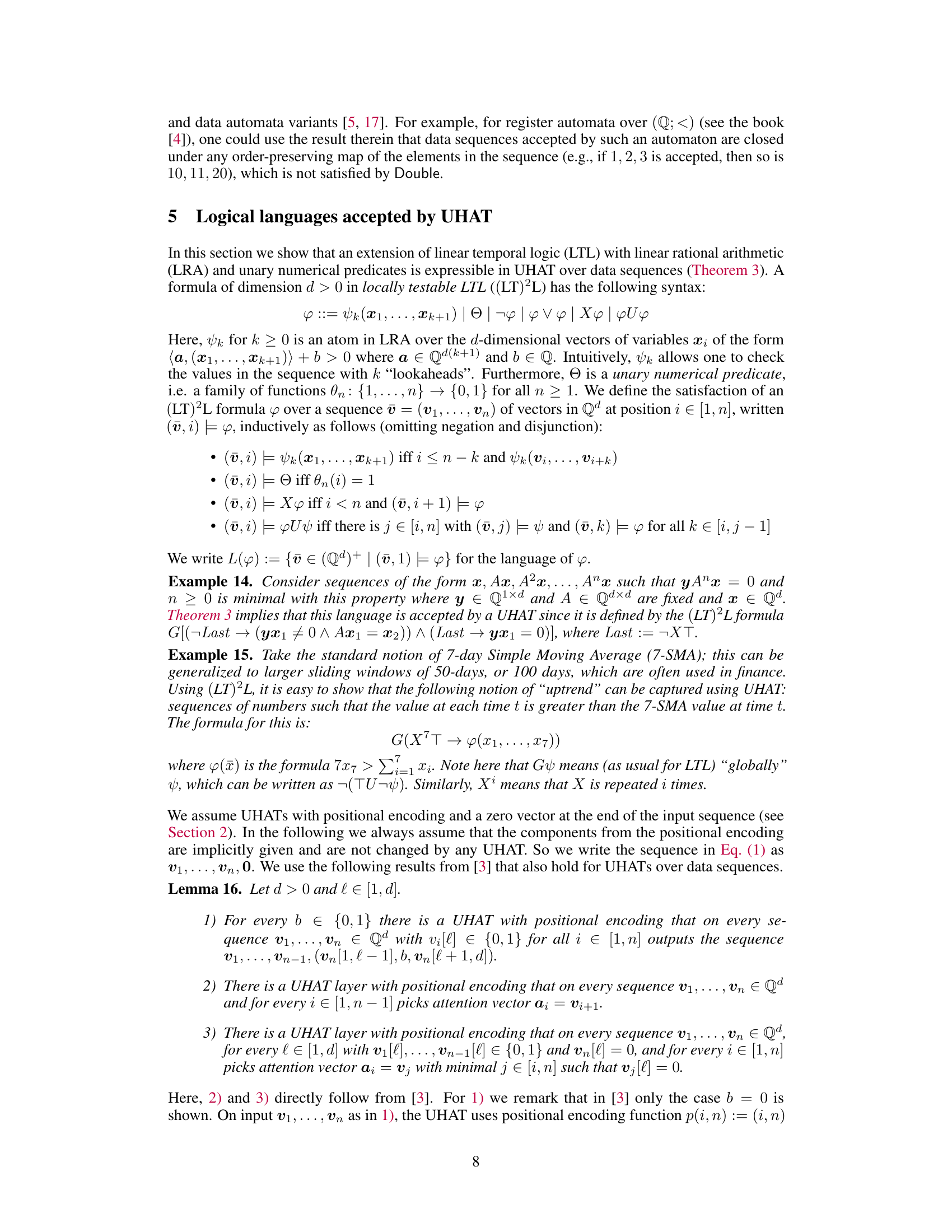
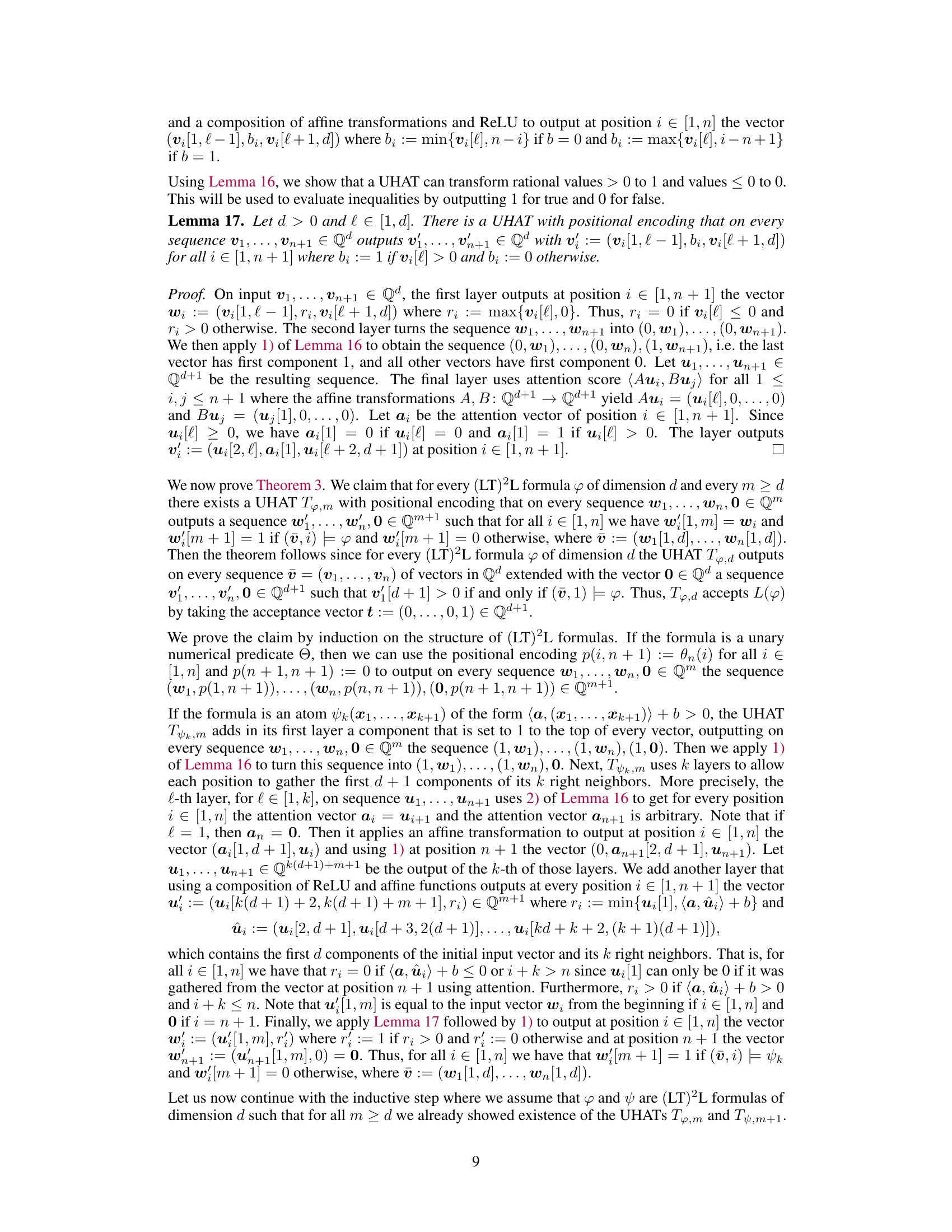
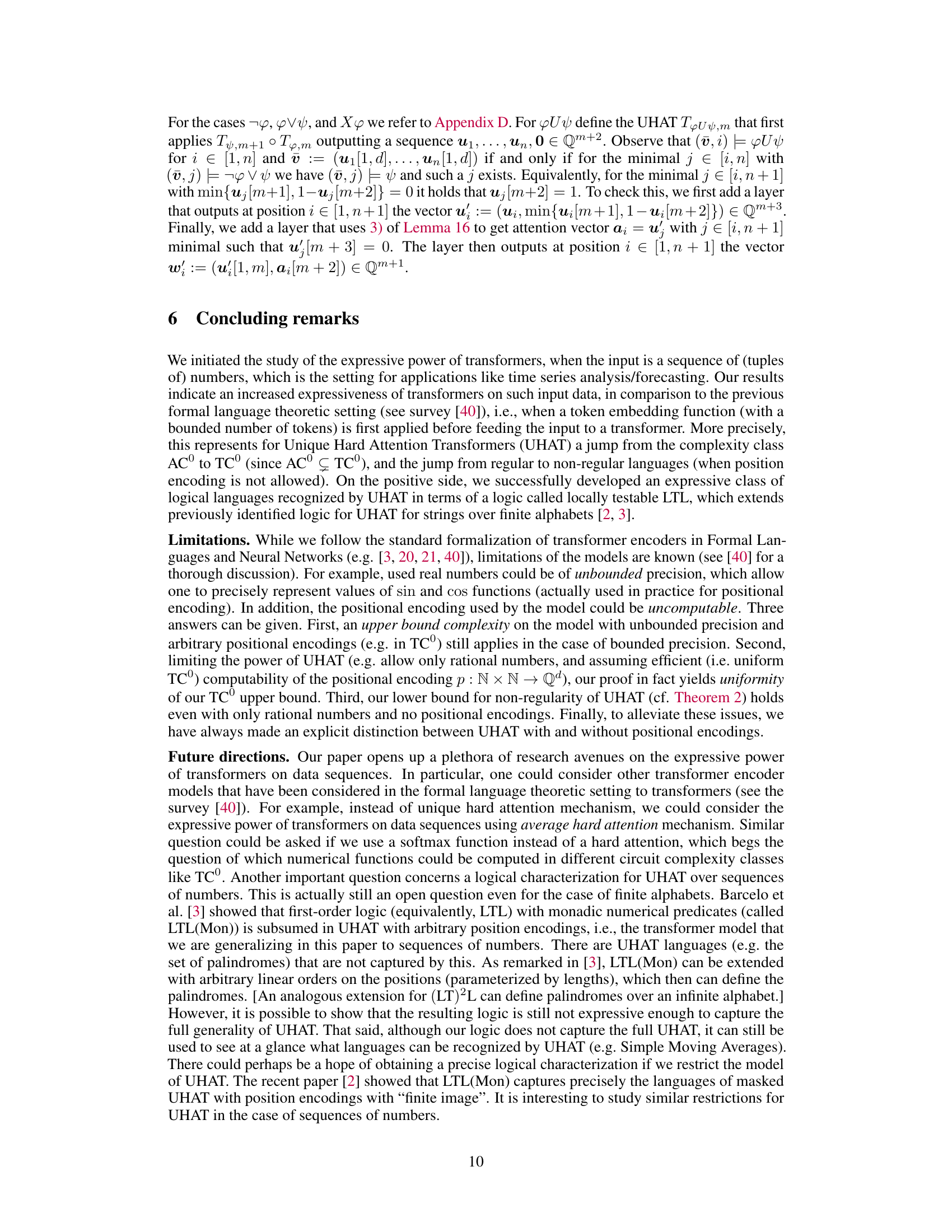
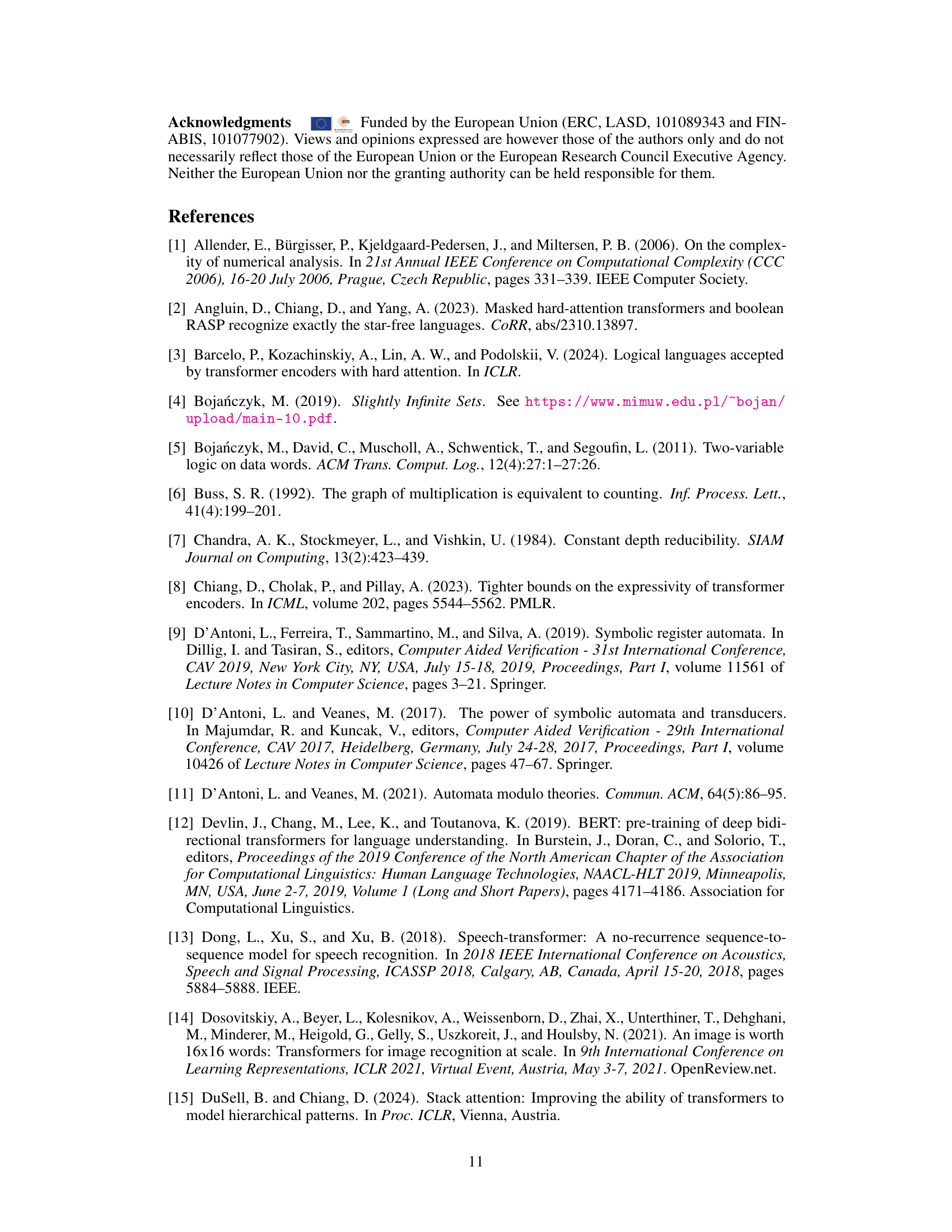
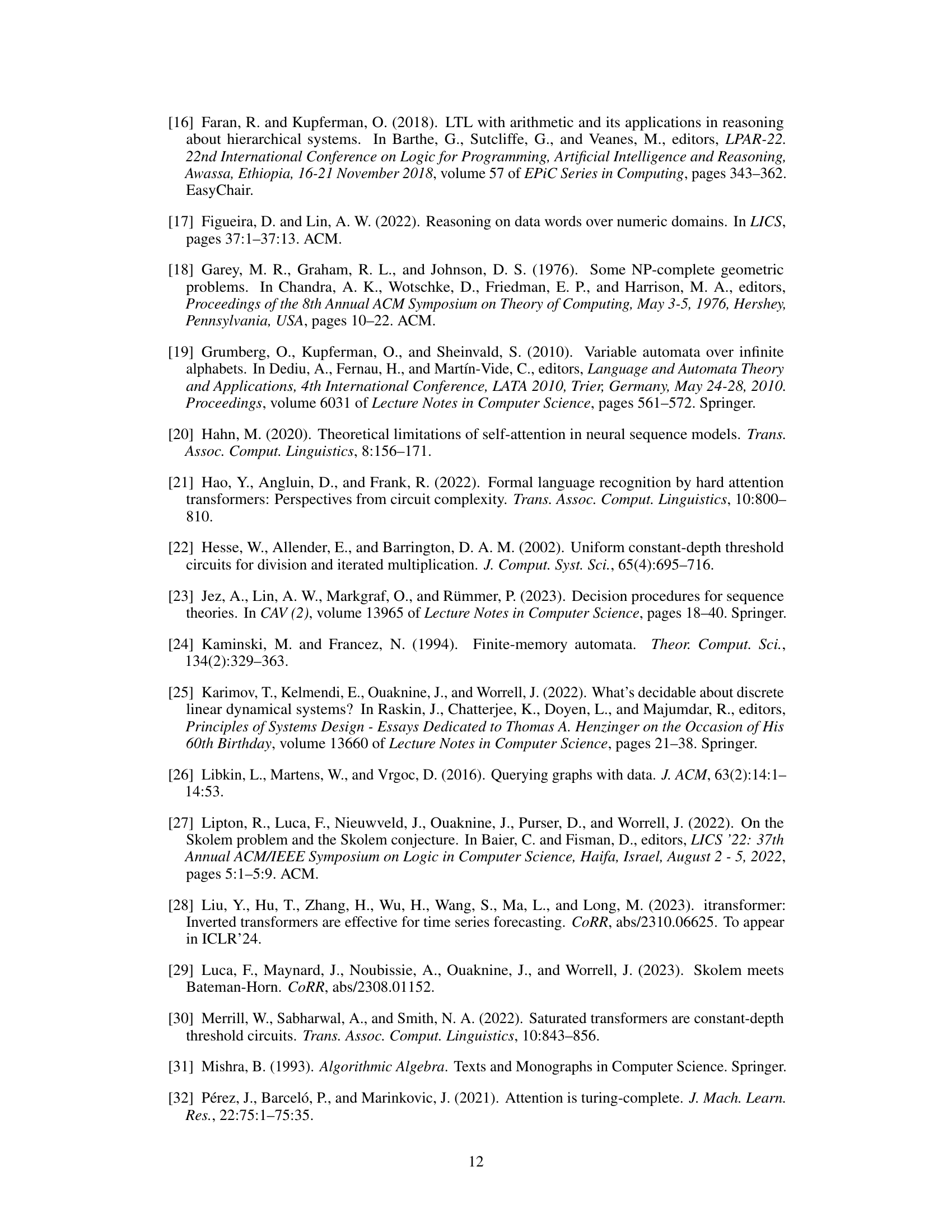
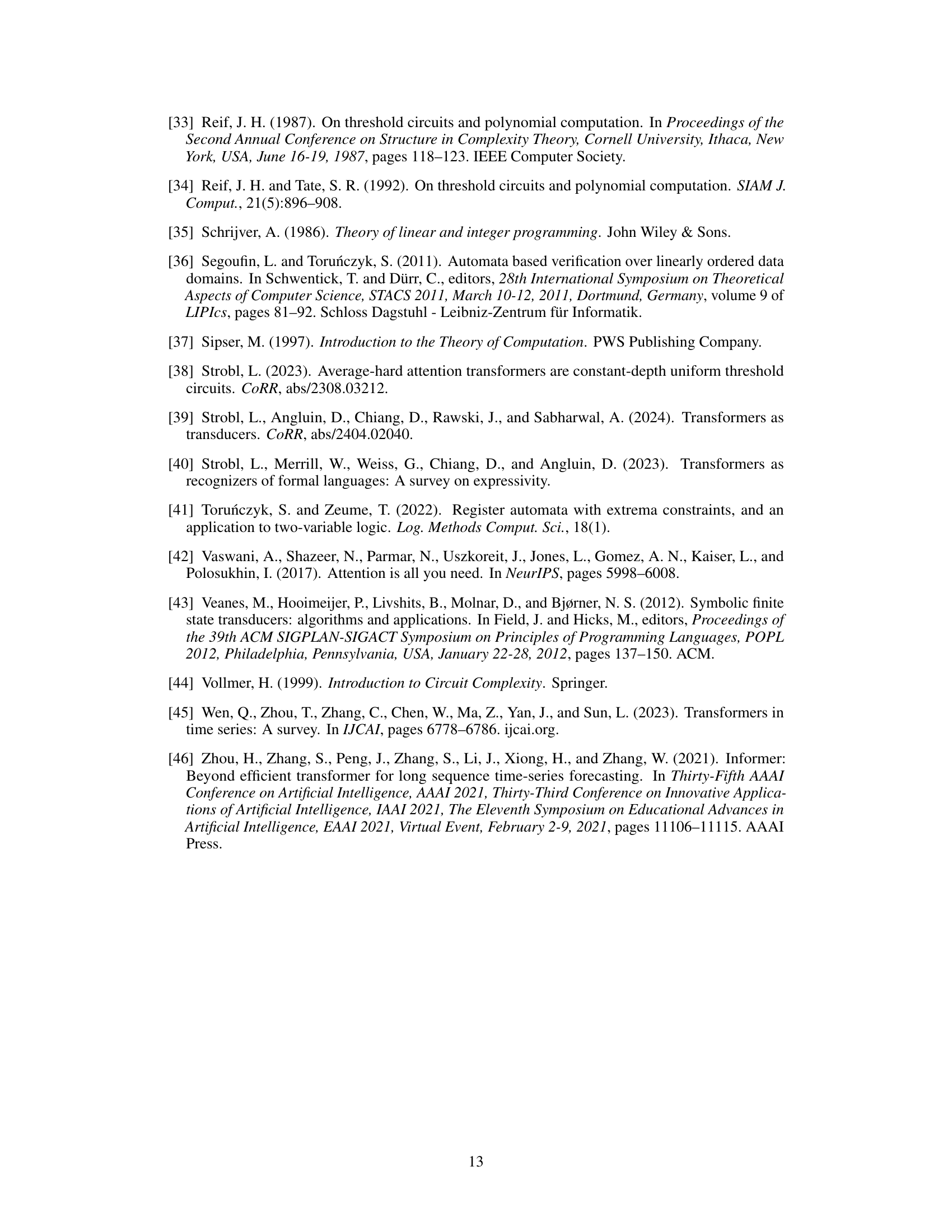
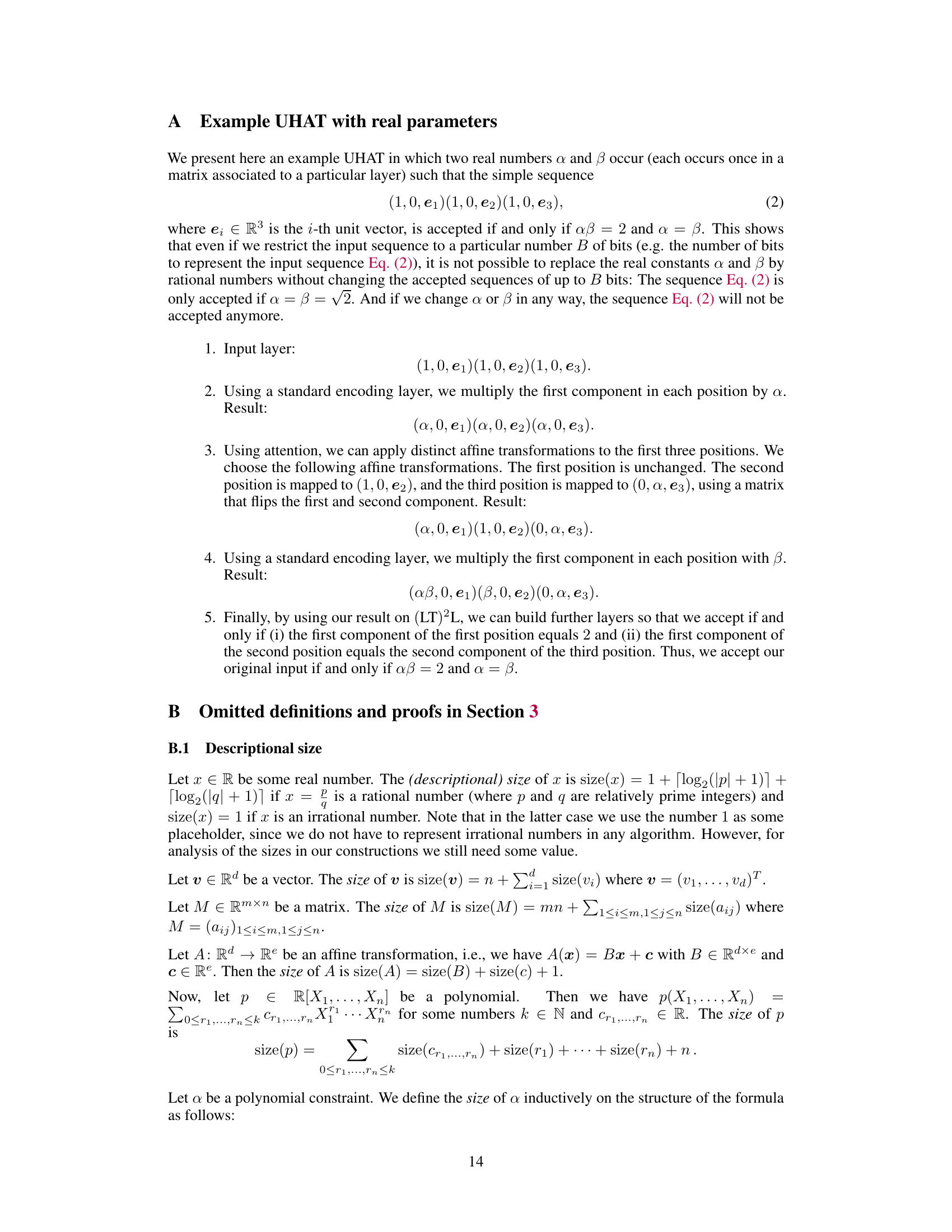
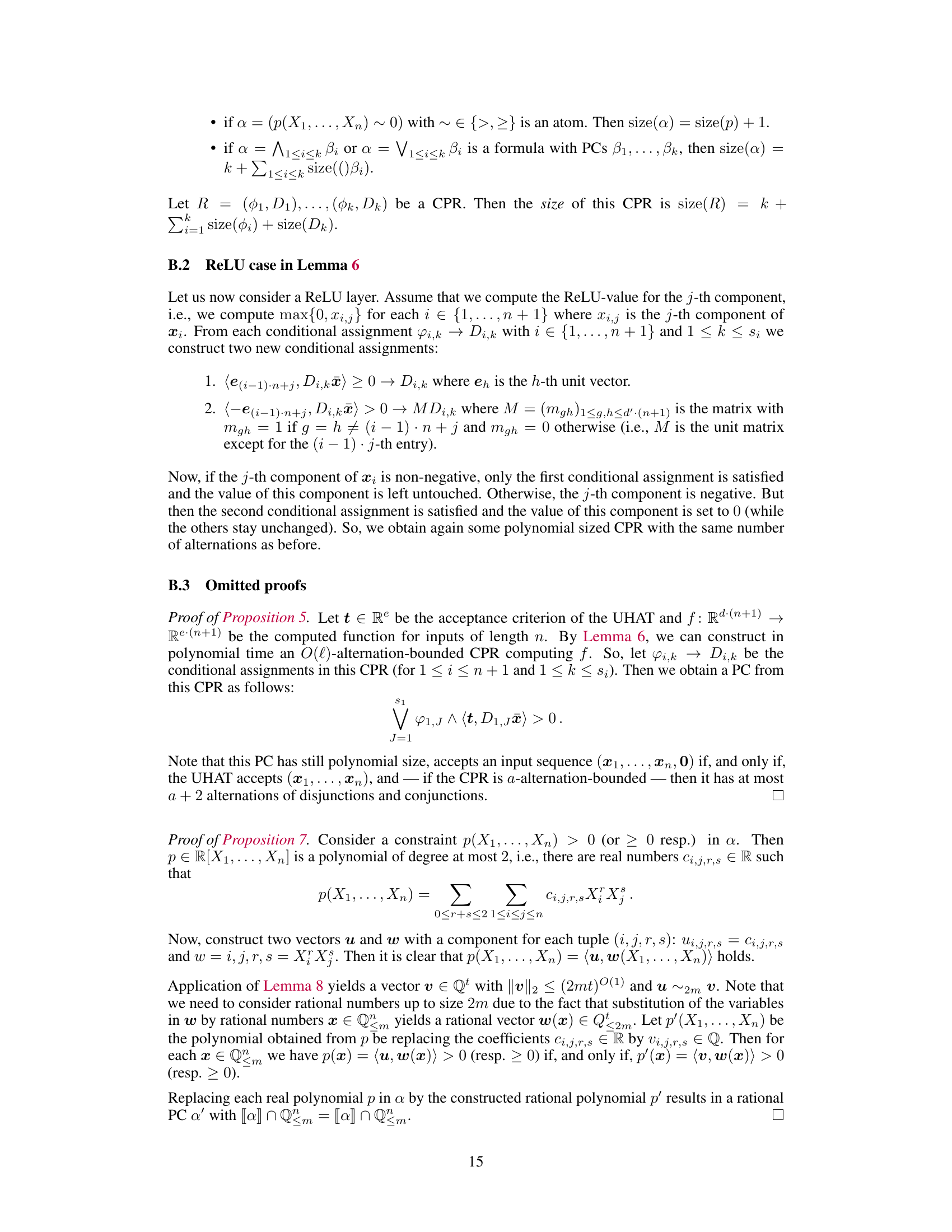
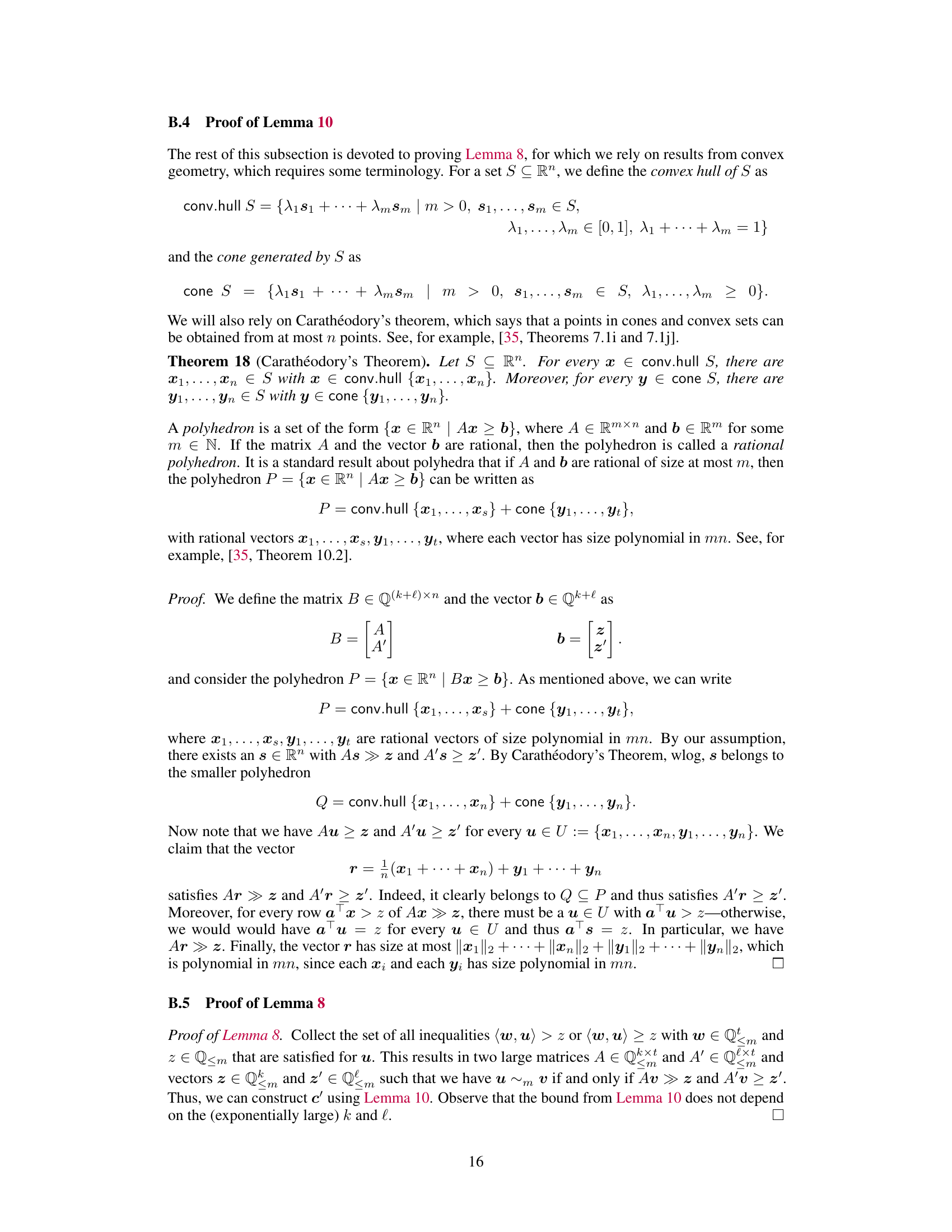
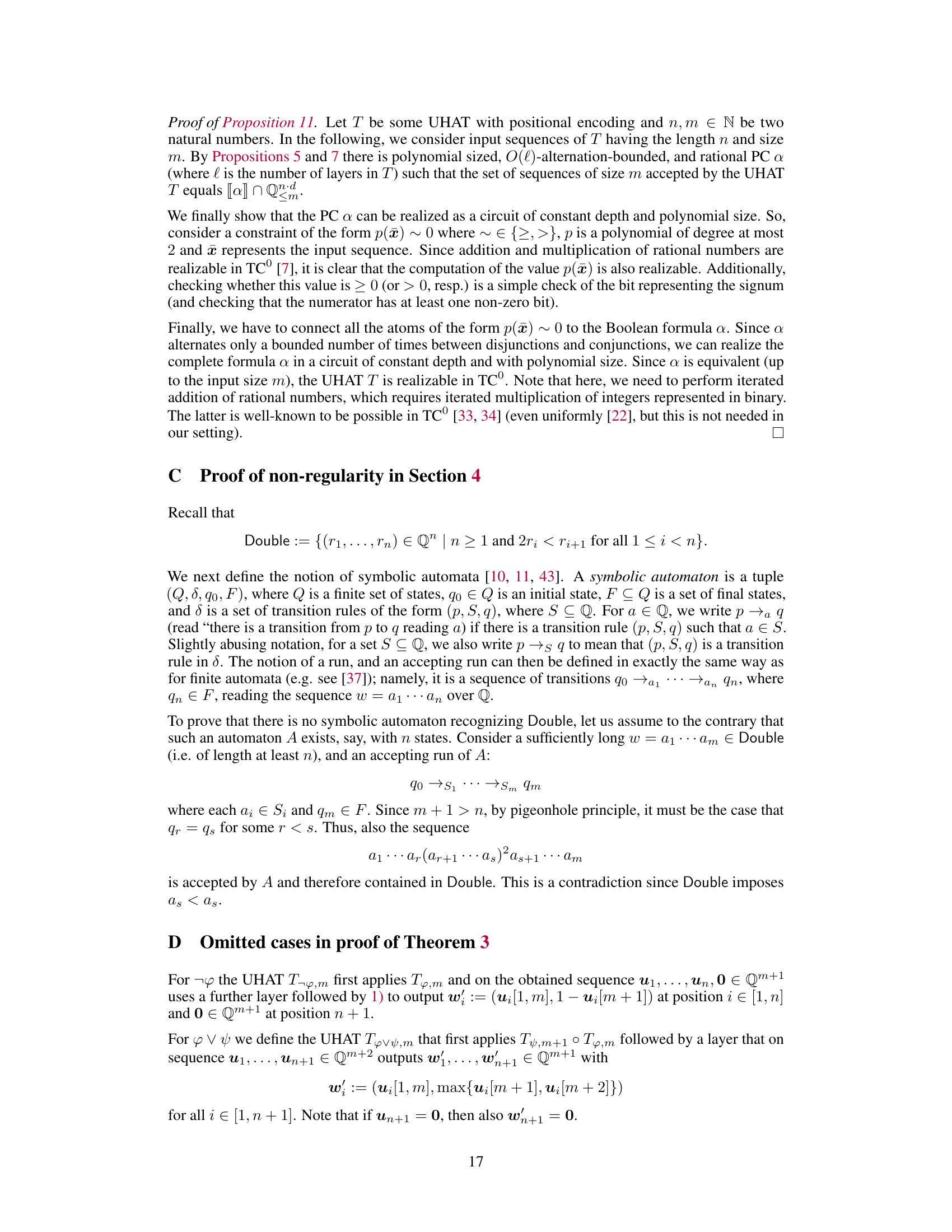
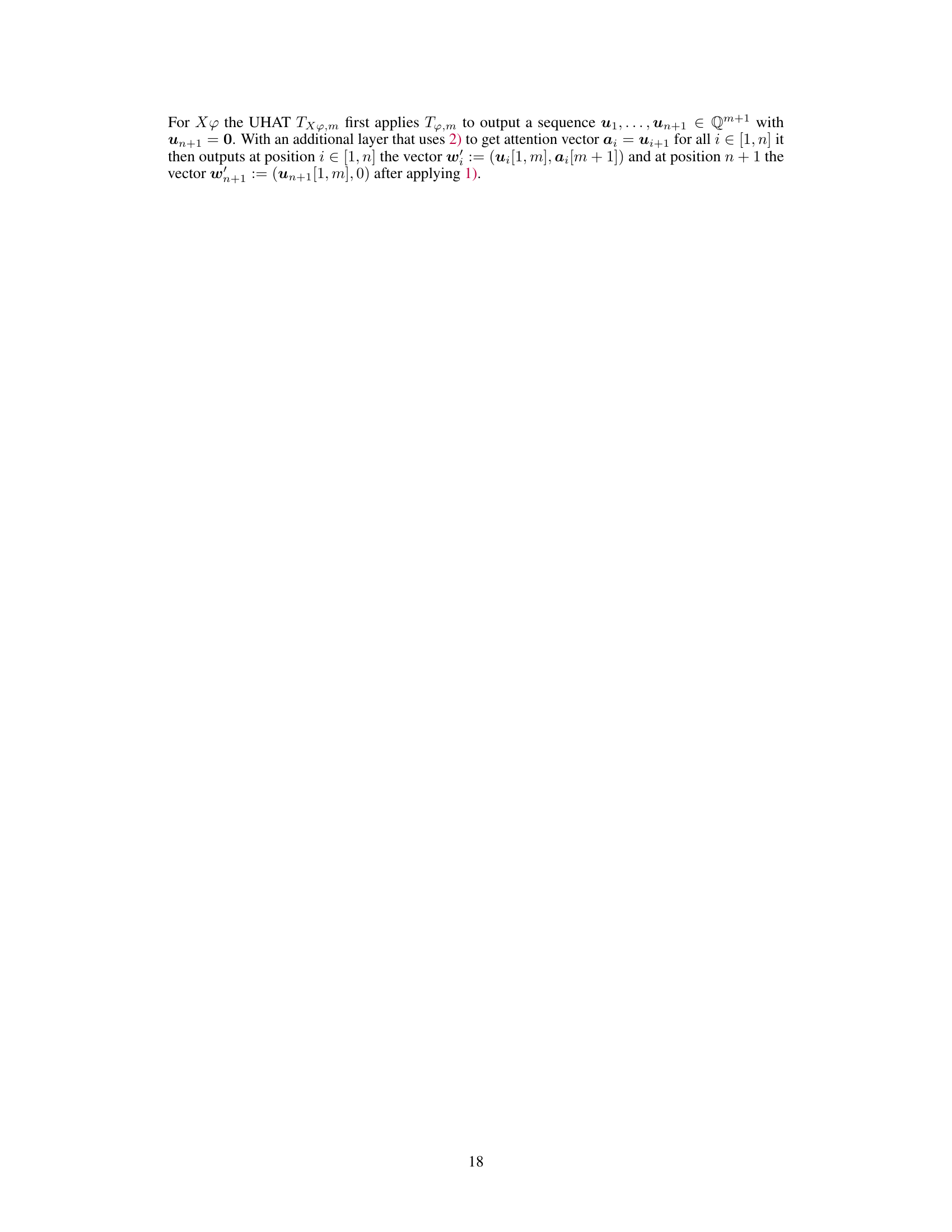
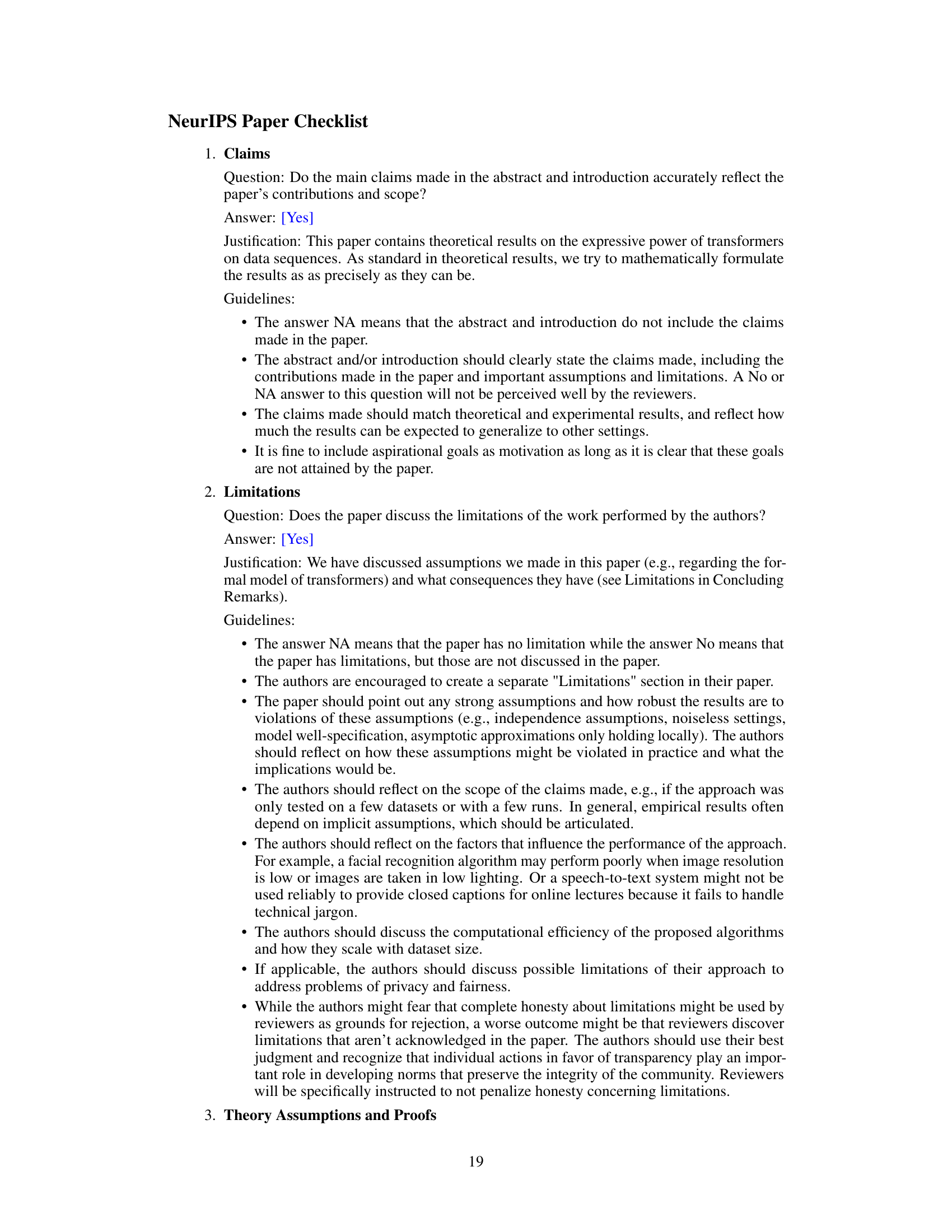
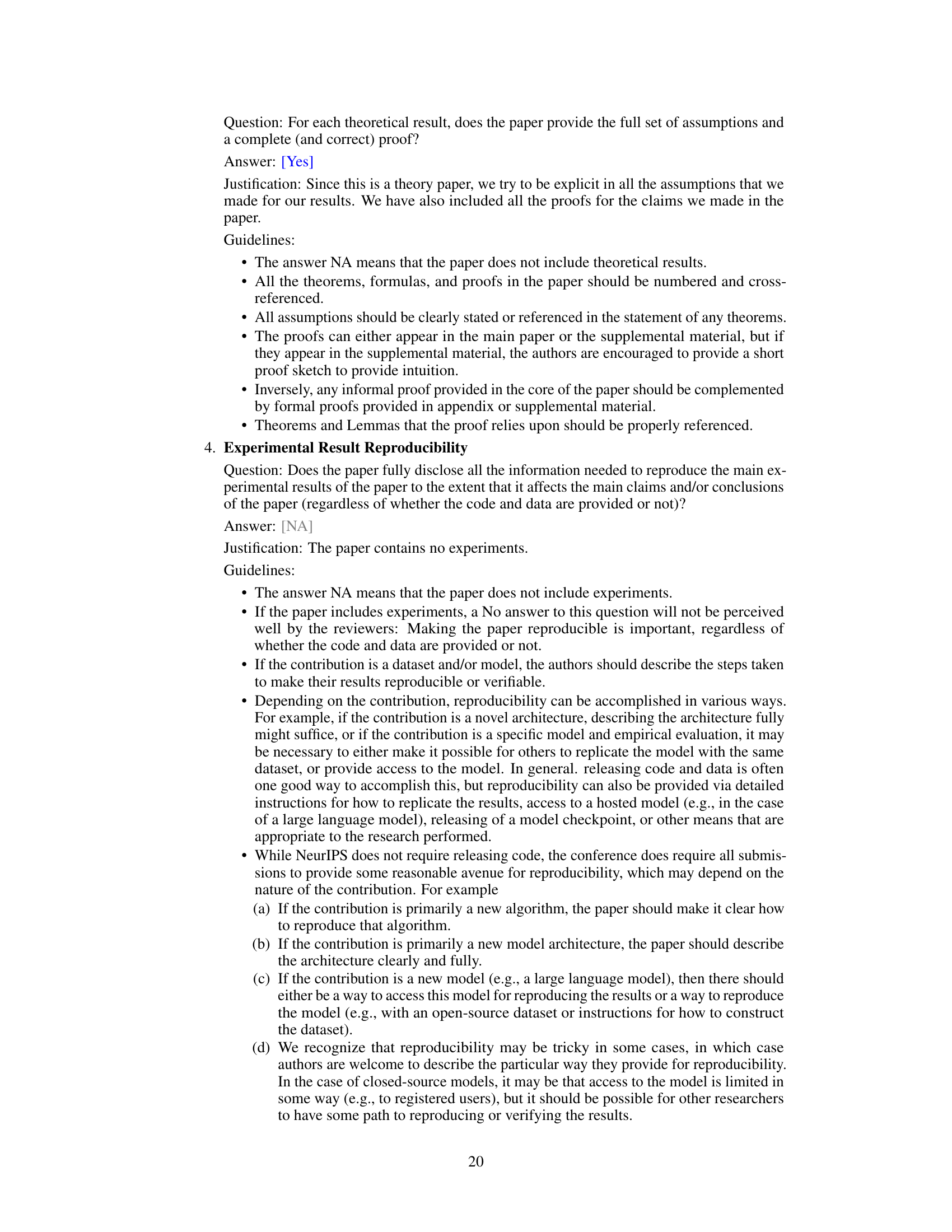