TL;DR#
Contrastive learning, a self-supervised method, has shown remarkable success in learning robust and transferable representations. However, a comprehensive theoretical understanding comparing single- and multi-modal approaches has been lacking. This paper tackles this challenge by providing a theoretical analysis and experimental validation comparing the optimization and generalization performance of multi-modal and single-modal contrastive learning. The research highlights the importance of understanding feature learning dynamics to guide model design and training.
The study introduces a novel theoretical framework using a data generation model with signal and noise to analyze feature learning. By applying a trajectory-based optimization analysis and characterizing the generalization capabilities on downstream tasks, the authors show that the signal-to-noise ratio (SNR) is the critical factor determining generalization performance. Multi-modal learning excels due to the cooperation between modalities, leading to better feature learning and enhanced performance compared to single-modal methods. The empirical experiments on both synthetic and real datasets support their theoretical findings.
Key Takeaways#
Why does it matter?#
This paper is crucial for researchers in contrastive learning. It provides a unified theoretical framework comparing single- and multi-modal approaches, addressing a significant gap in the field. The findings on signal-to-noise ratio (SNR) and its impact on generalization are highly valuable, opening doors for improved model design and training strategies.
Visual Insights#
🔼 This figure visualizes the training dynamics and downstream task performance of both single-modal and multi-modal contrastive learning methods. It consists of four subplots: training loss, test accuracy, signal learning, and noise memorization. Each subplot displays the performance of both methods across 200 epochs. The results show that multi-modal contrastive learning generally achieves lower training loss and higher test accuracy, indicating better generalization. The signal learning curve suggests that multi-modal learning focuses more on learning signal information, while single-modal learning might concentrate on memorizing noise.
read the caption
Figure 1: Training loss, test accuracy, signal learning and noise memorization of single-modal and multi-modal contrastive learning.
🔼 This table presents a comparison of the performance of single-modal and multi-modal contrastive learning models on a real-world dataset, ColoredMNIST. The ColoredMNIST dataset is a variation of the standard MNIST dataset that introduces spurious correlations between digit labels and colors. The results show that multi-modal contrastive learning significantly outperforms single-modal contrastive learning in terms of test accuracy under this distribution shift, achieving 82.13% compared to 12.68%. This highlights the advantage of multi-modal learning in handling data with spurious correlations, demonstrating better generalization to out-of-distribution data.
read the caption
Table 1: Performance comparison for single and multi-modal contrastive learning.
Full paper#
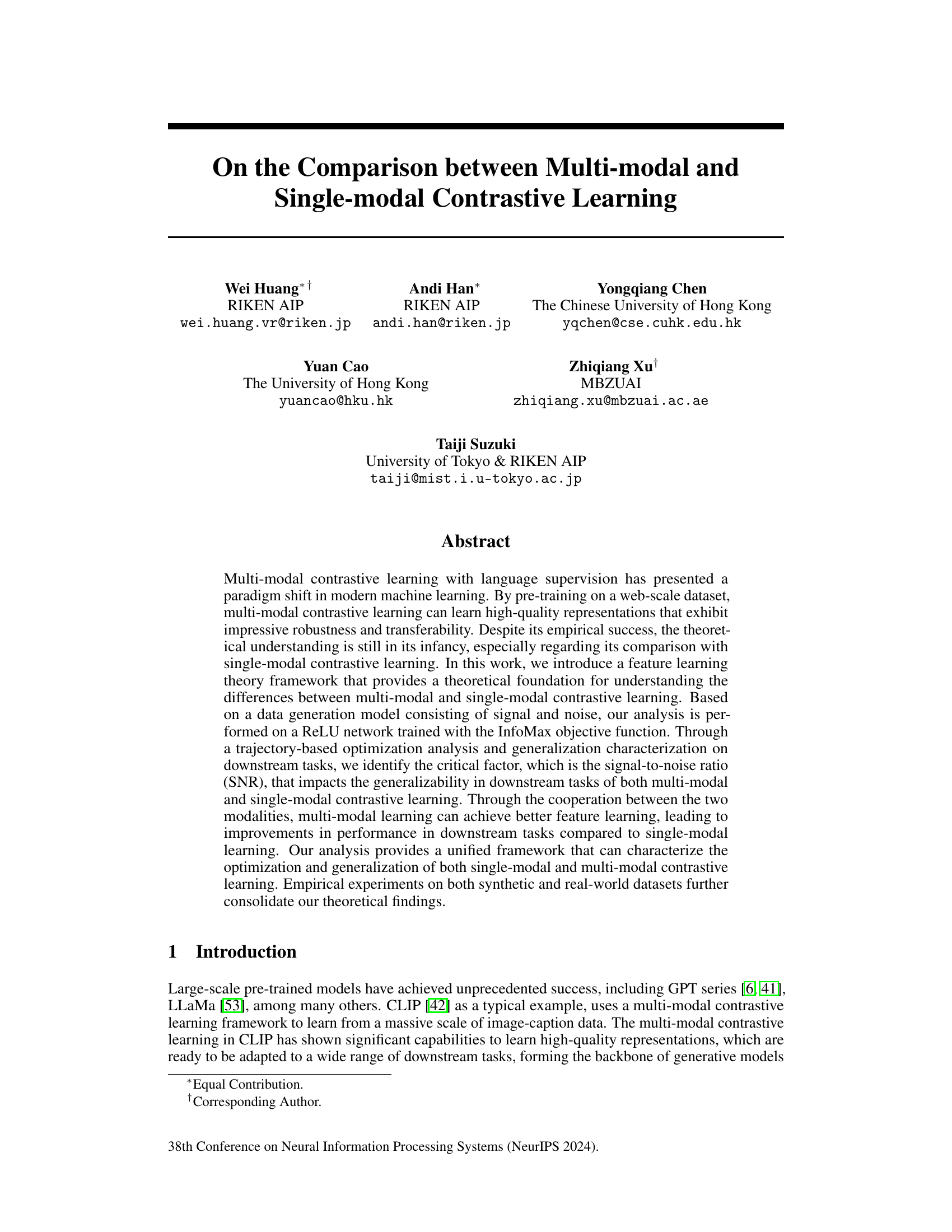
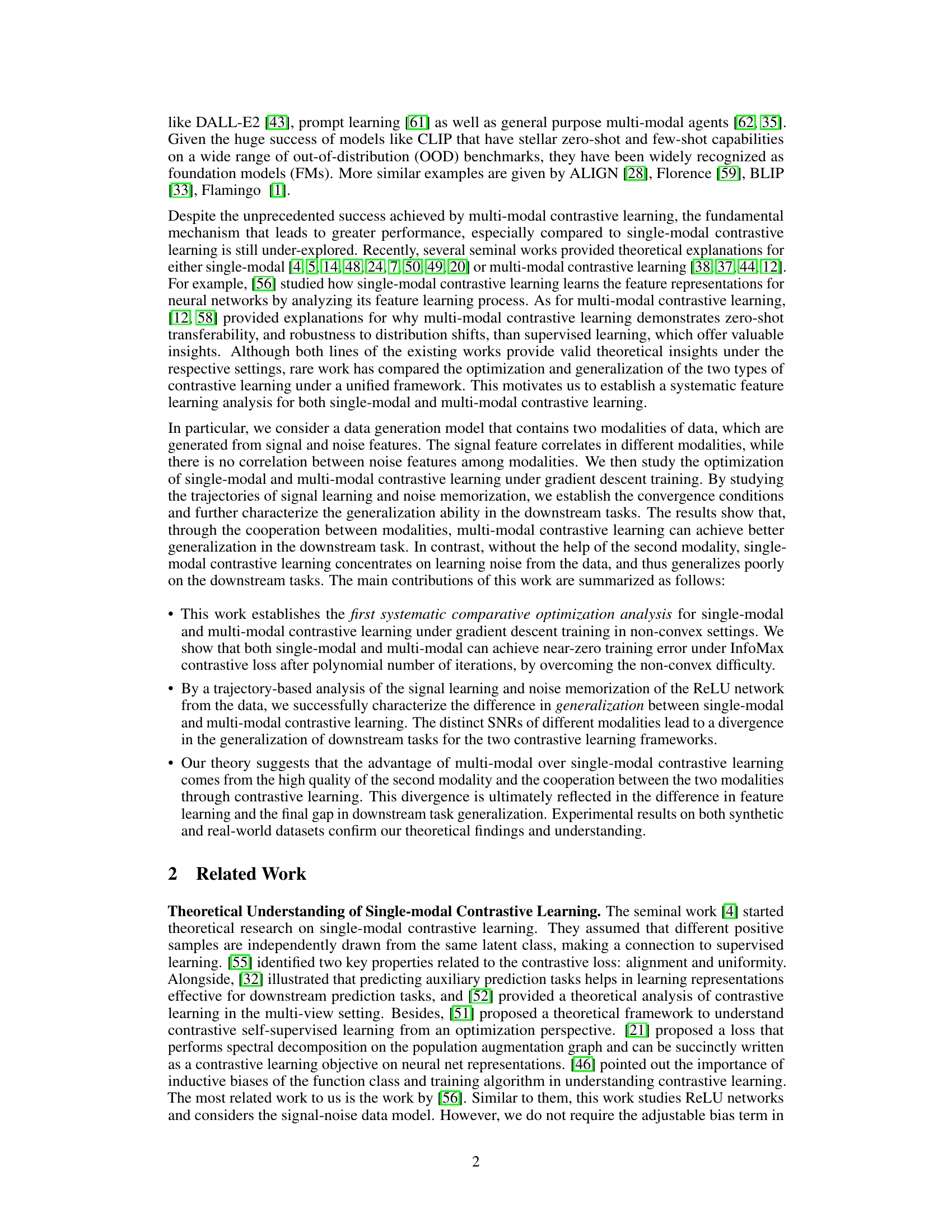
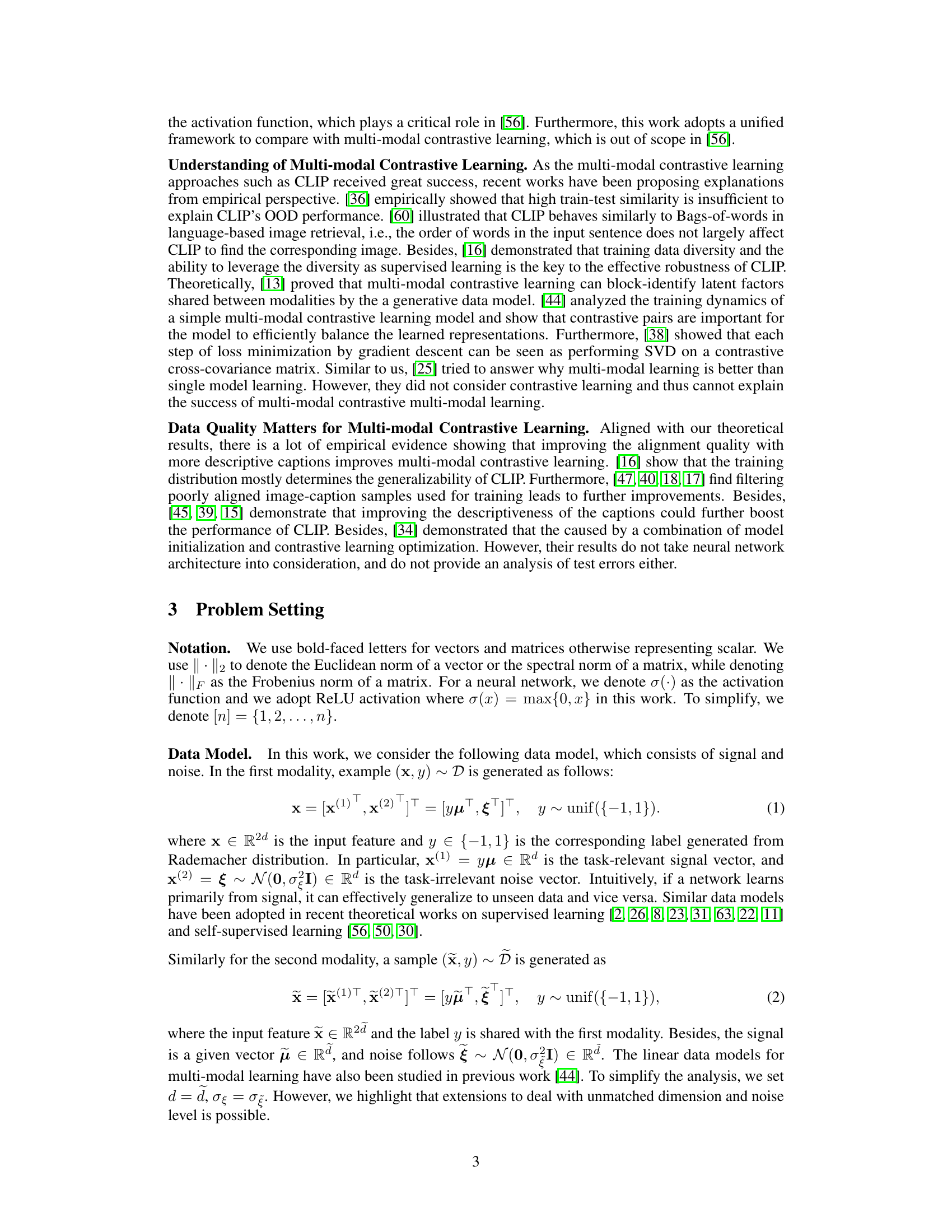
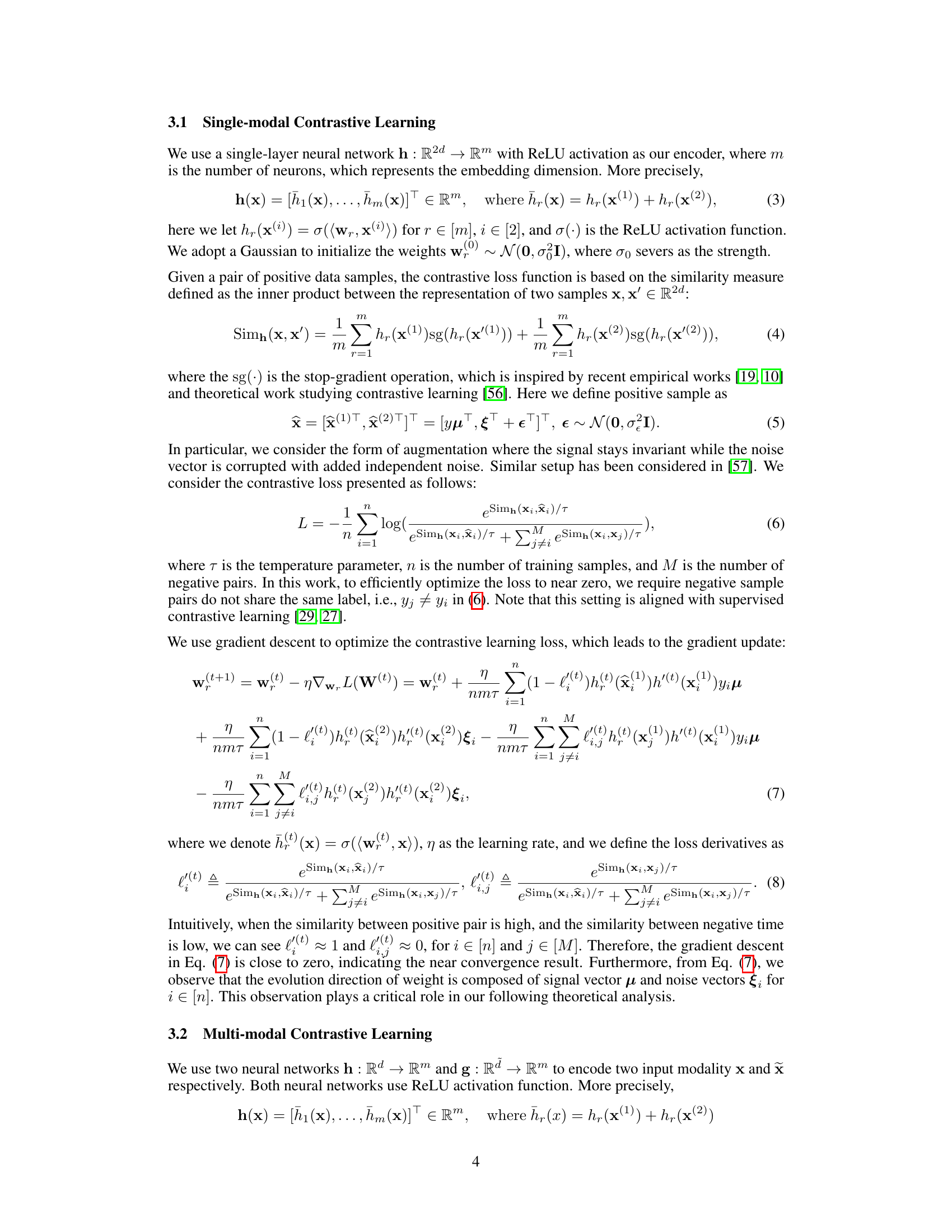
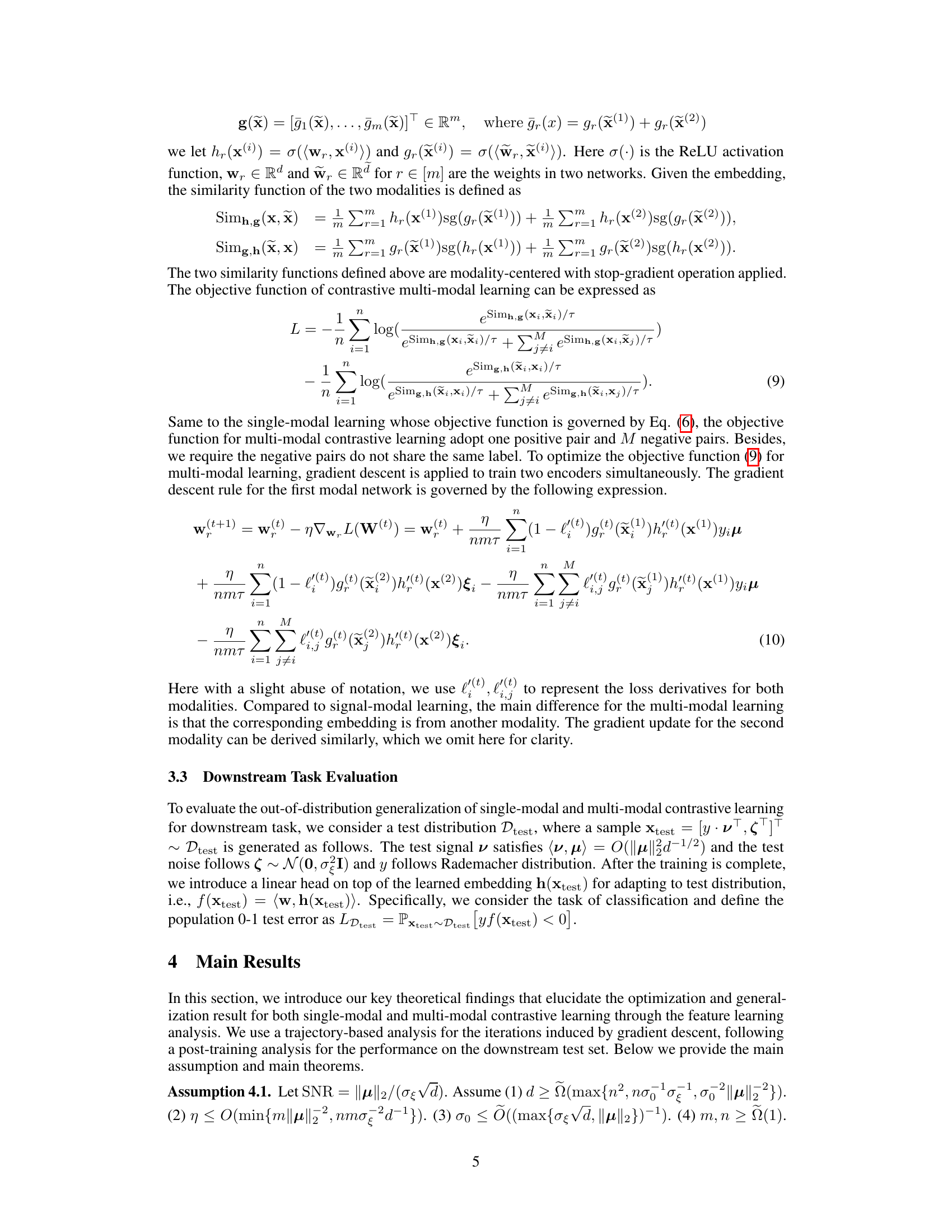
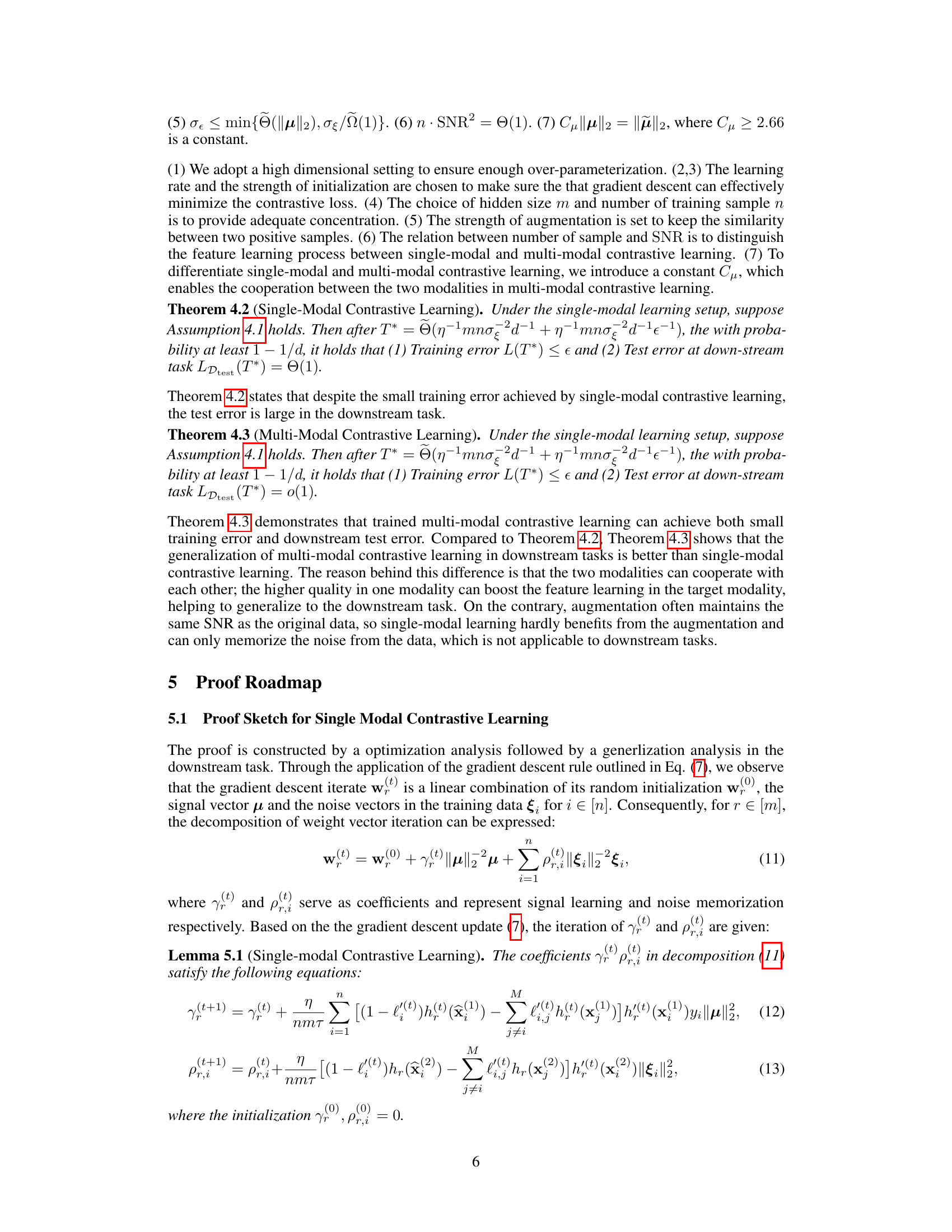
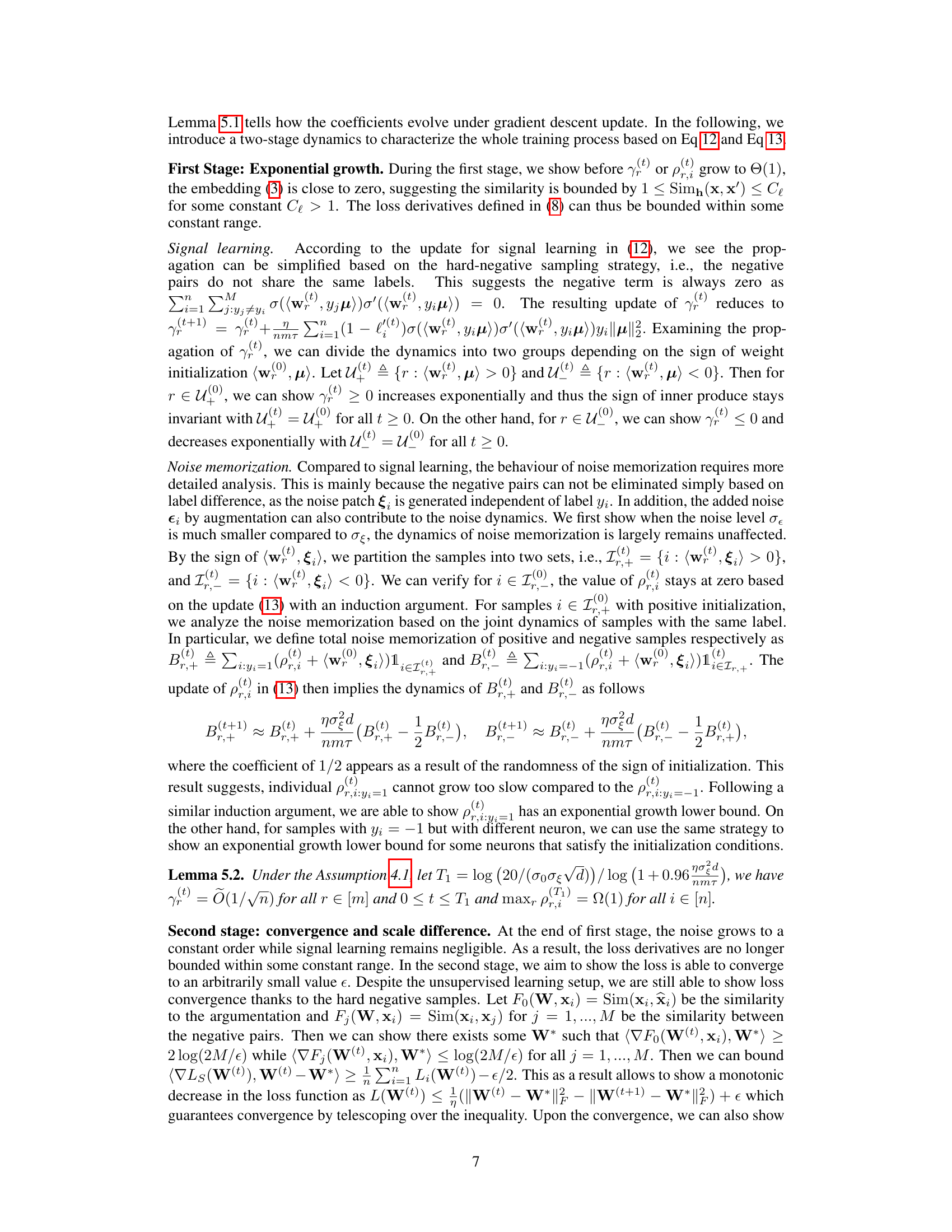
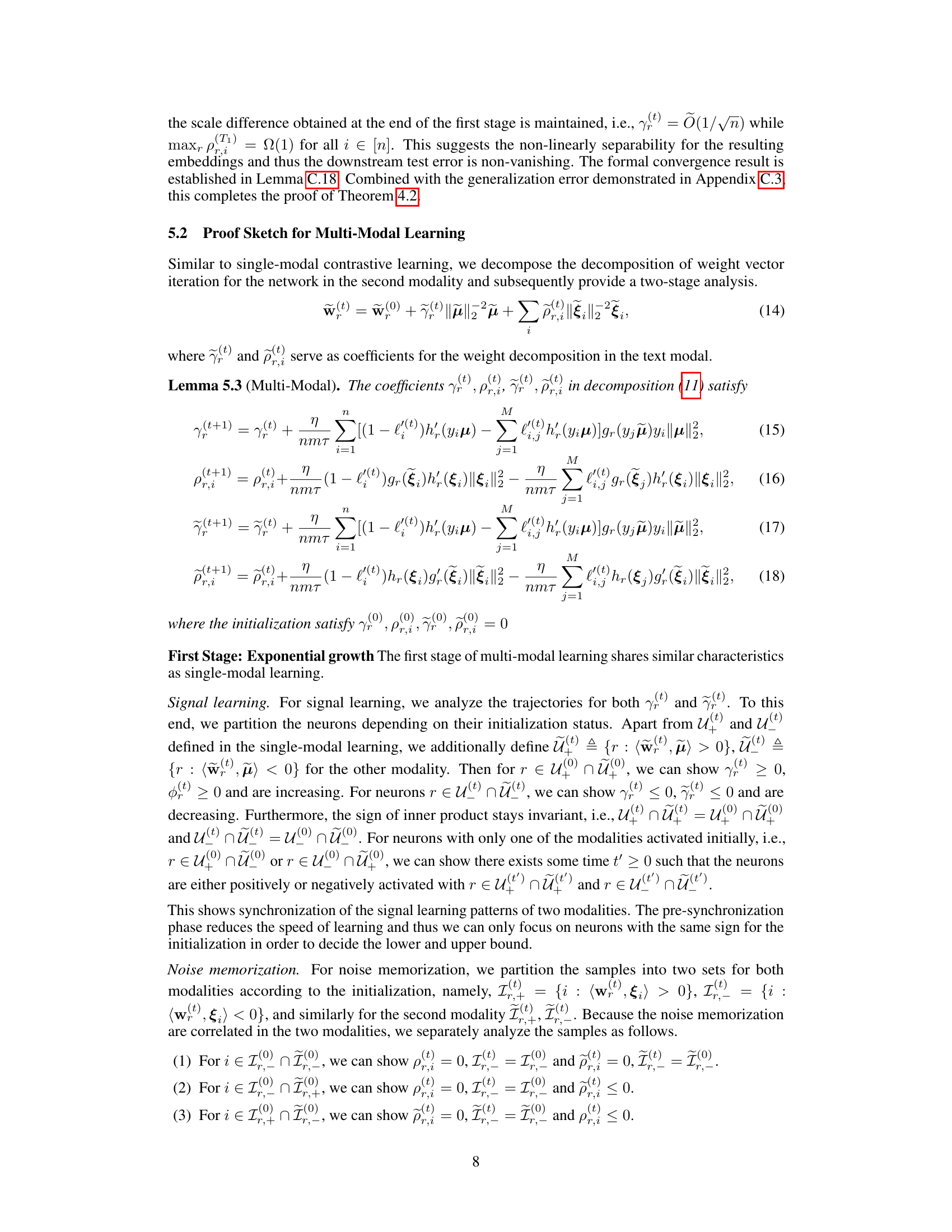
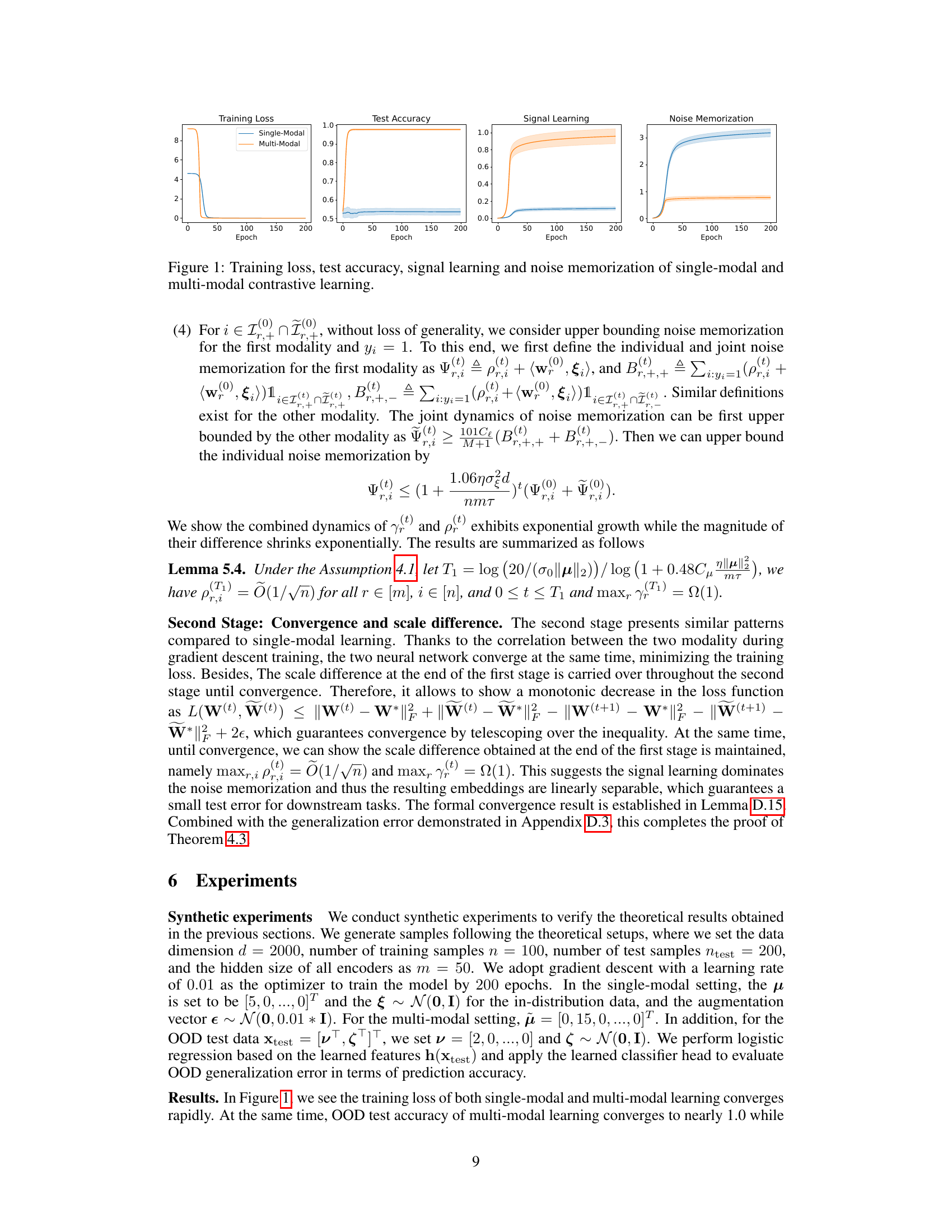
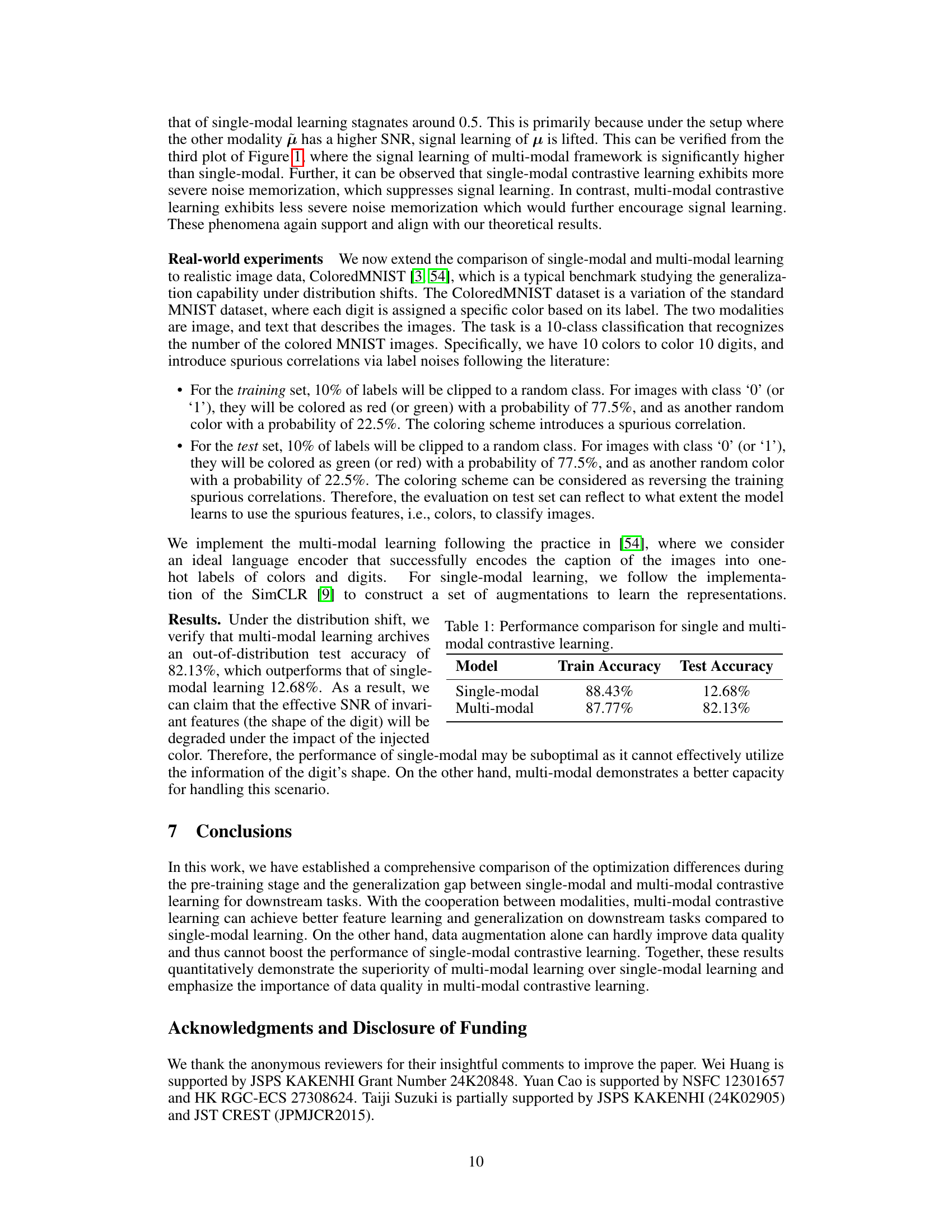
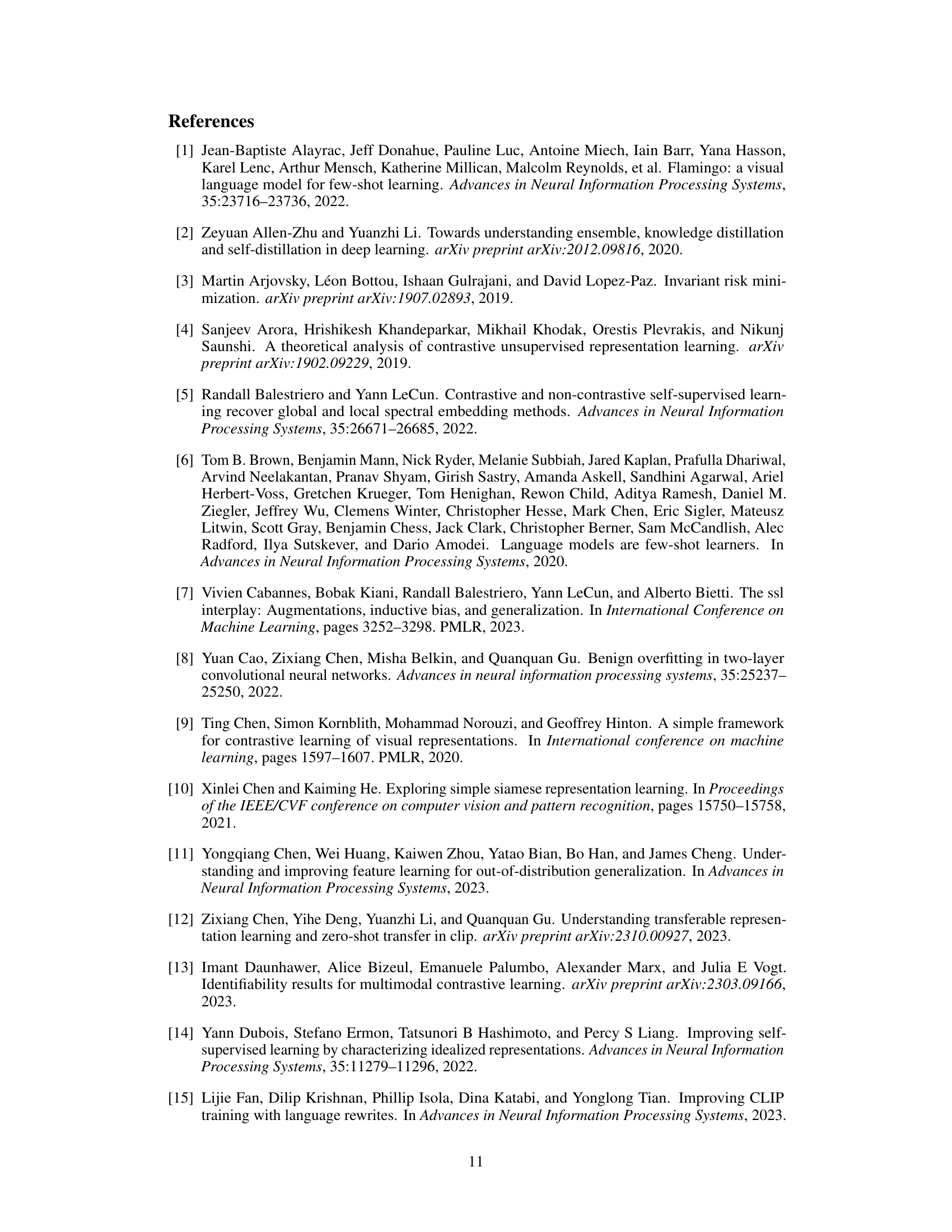
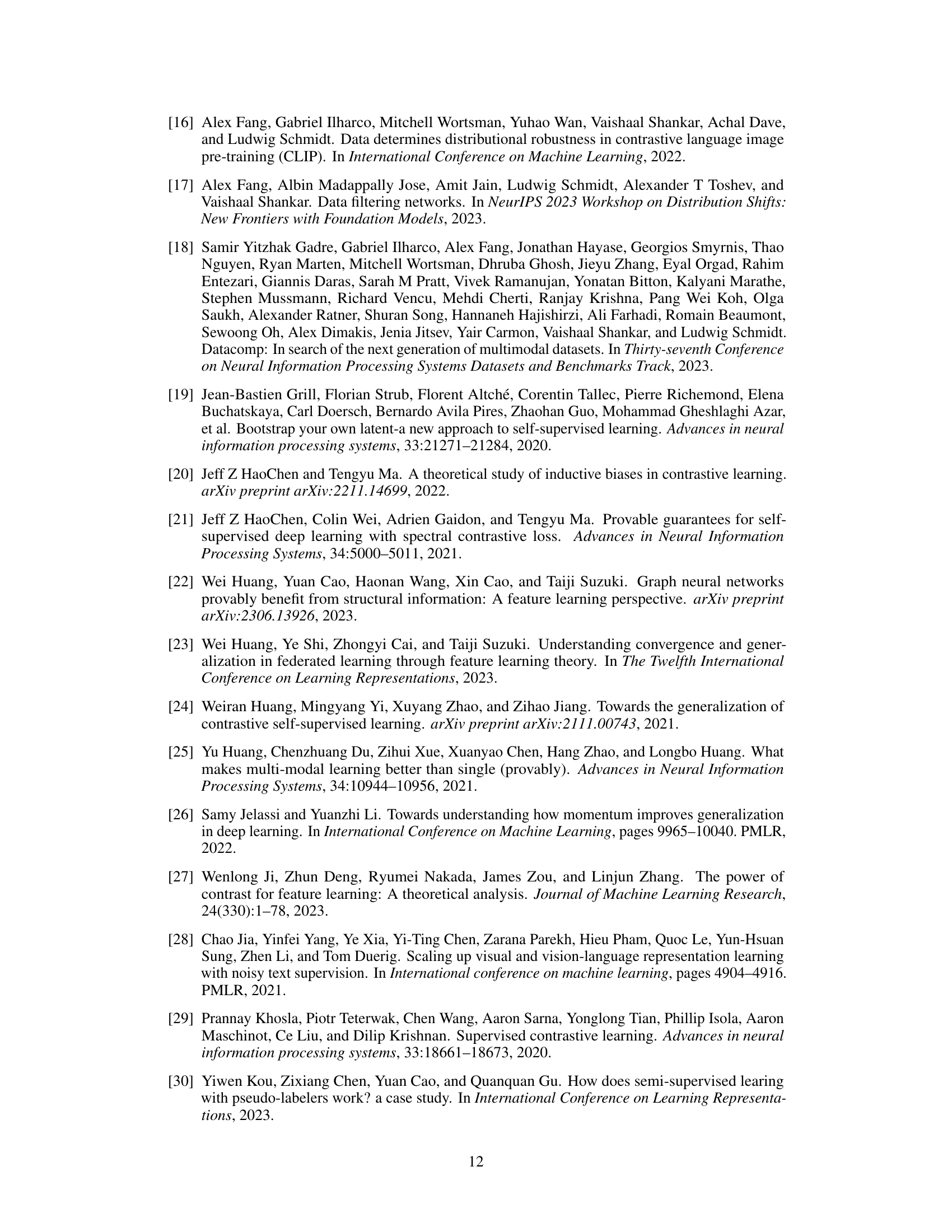
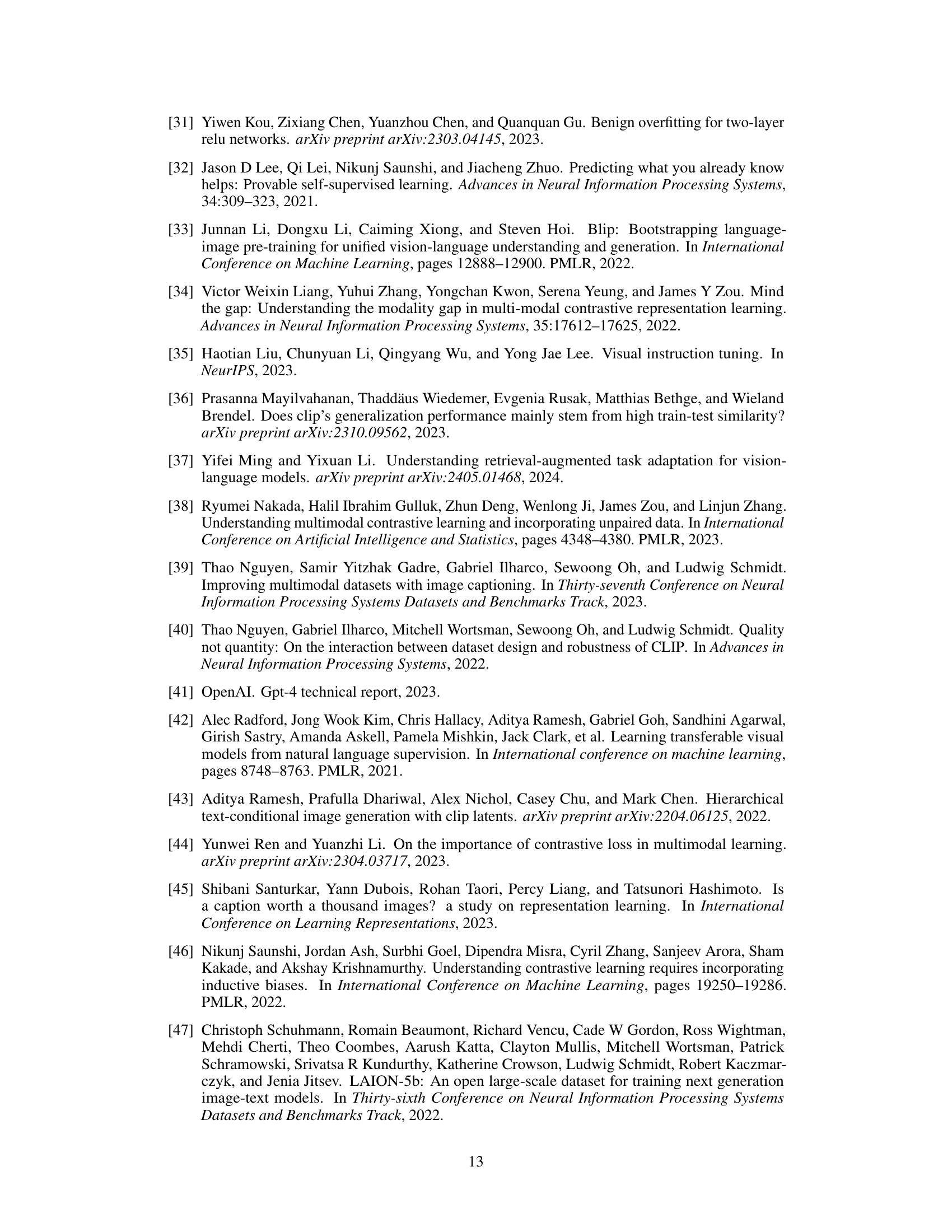
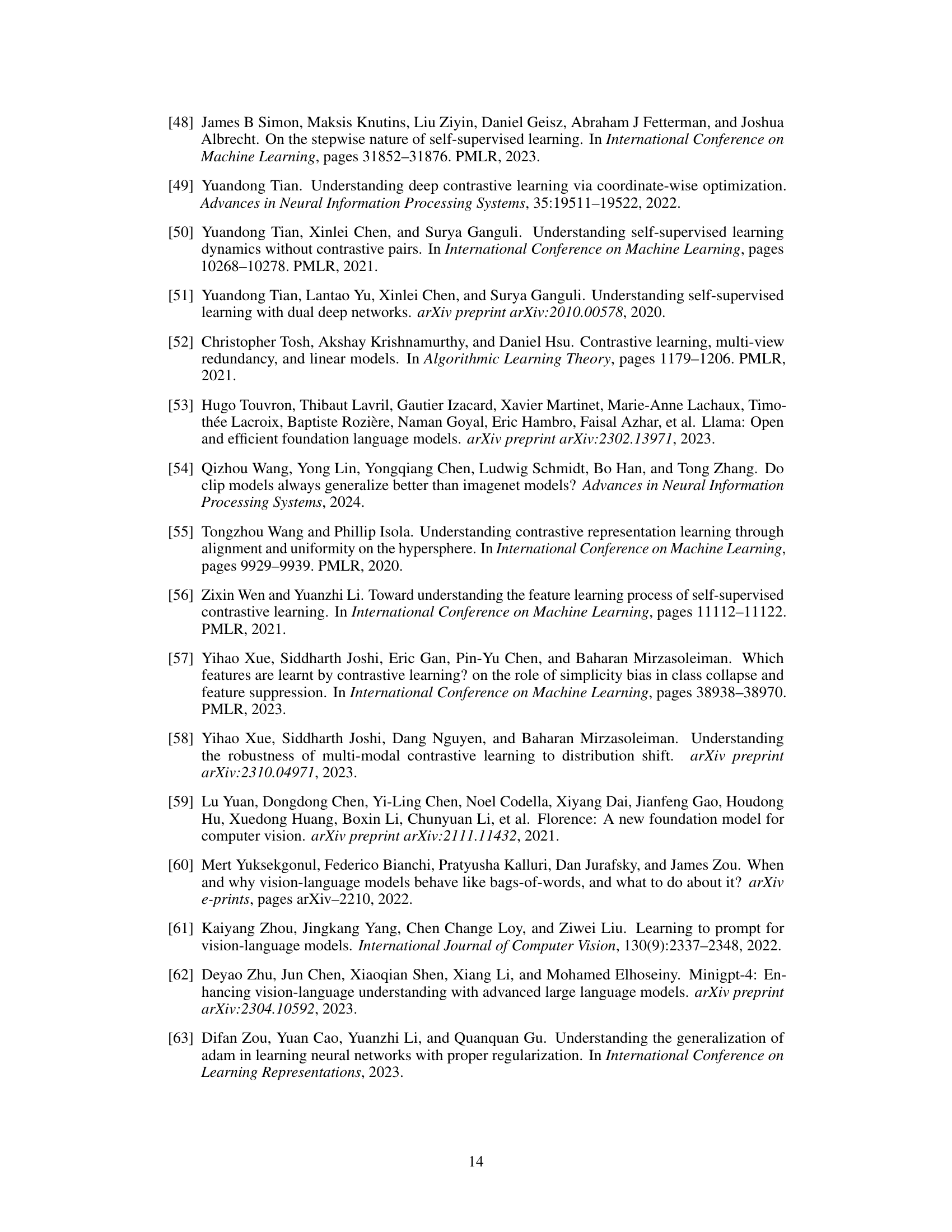
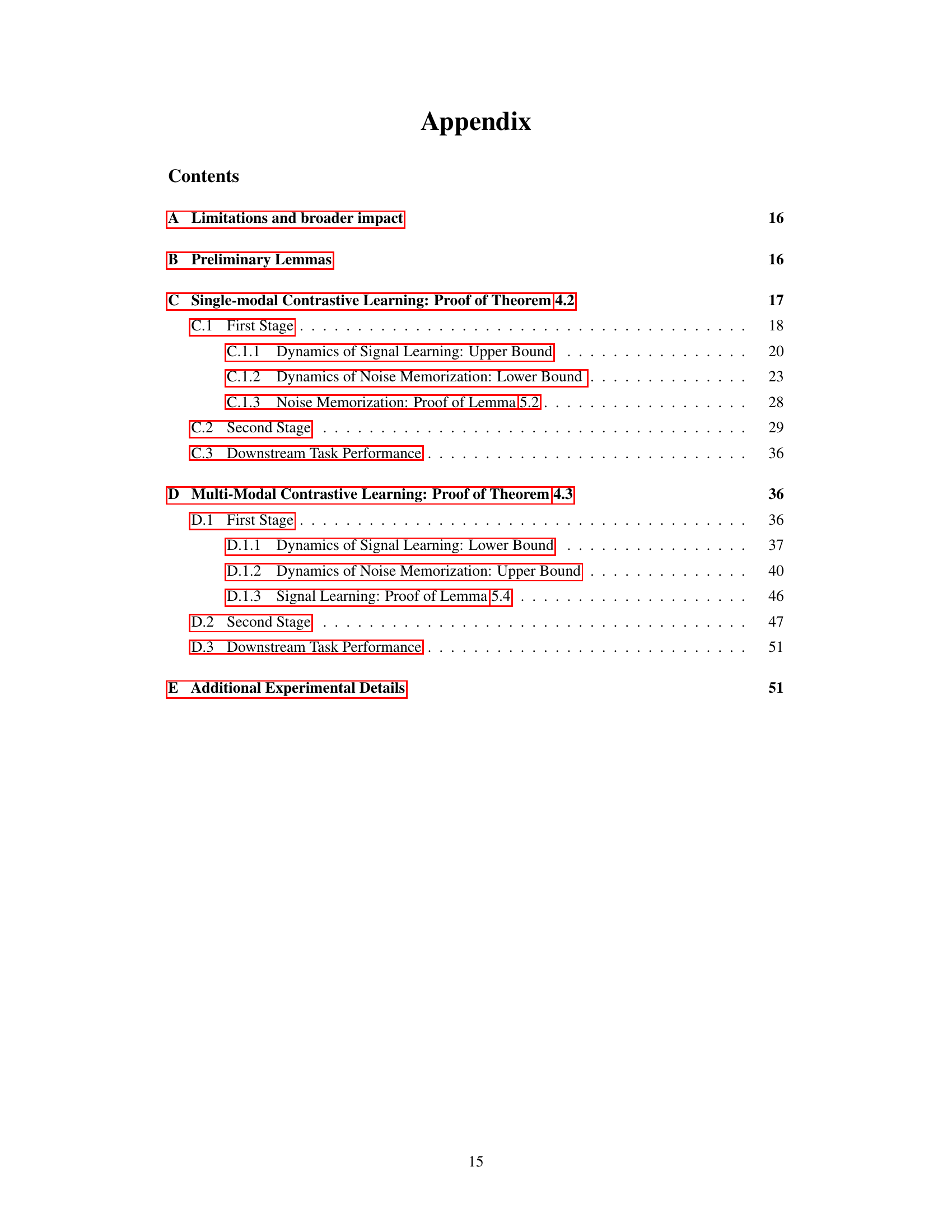
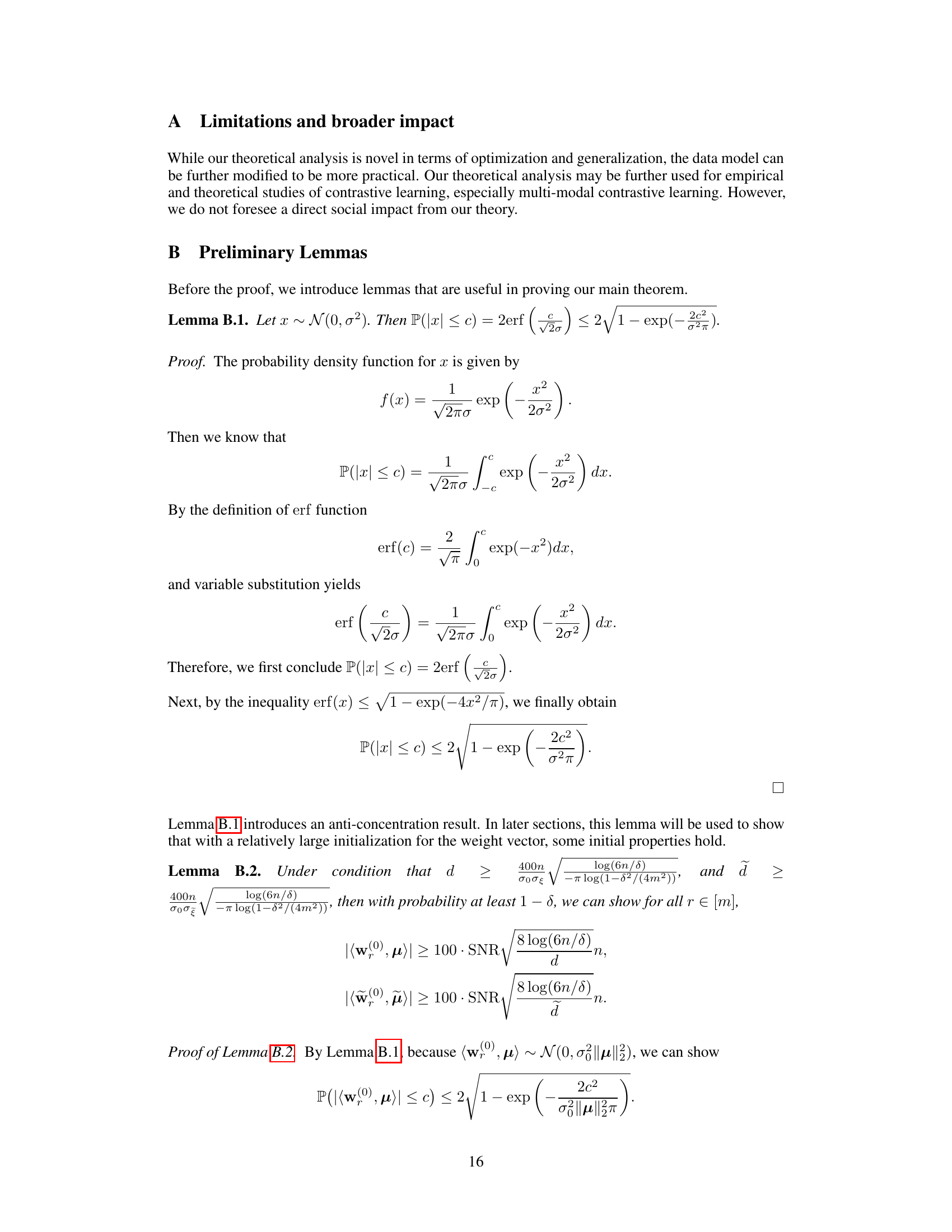
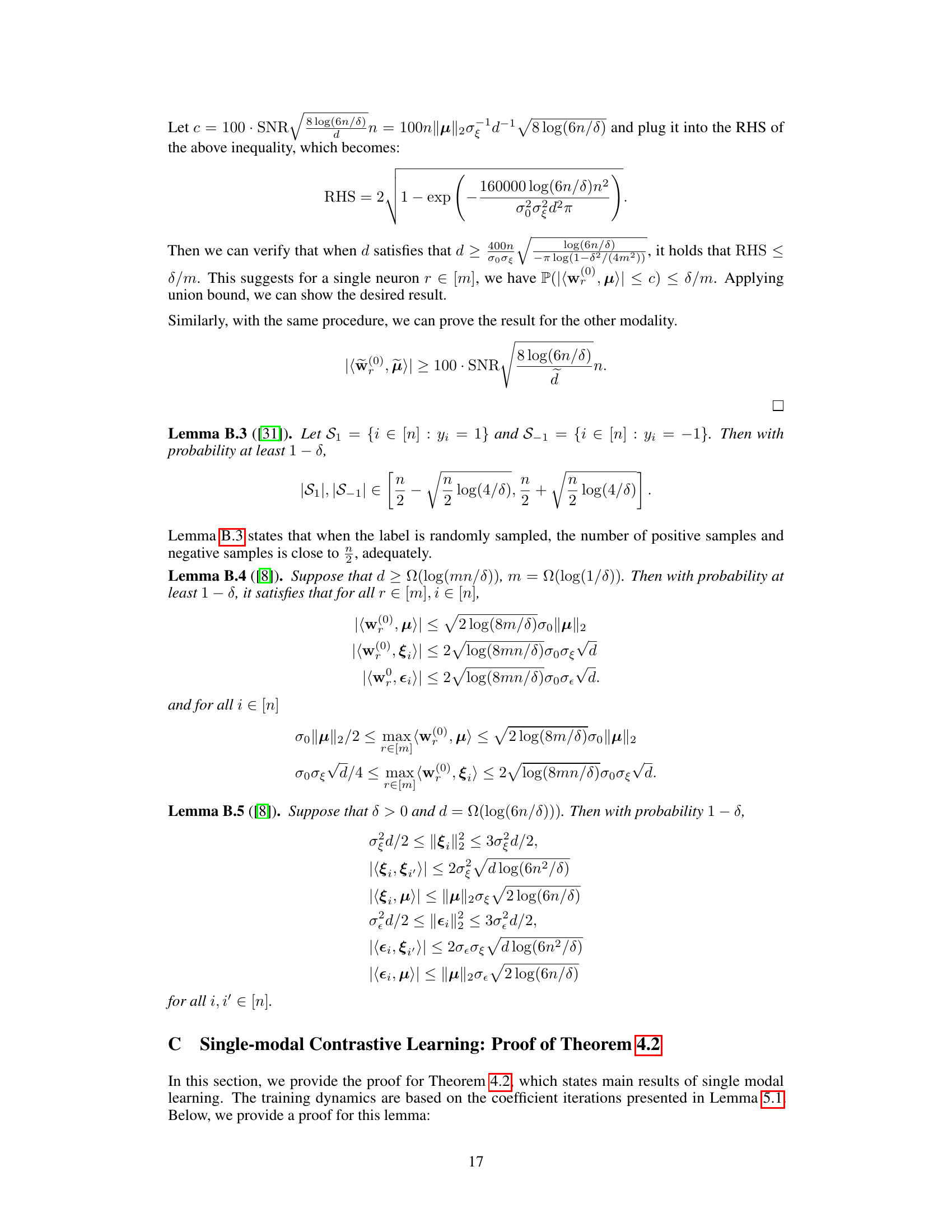
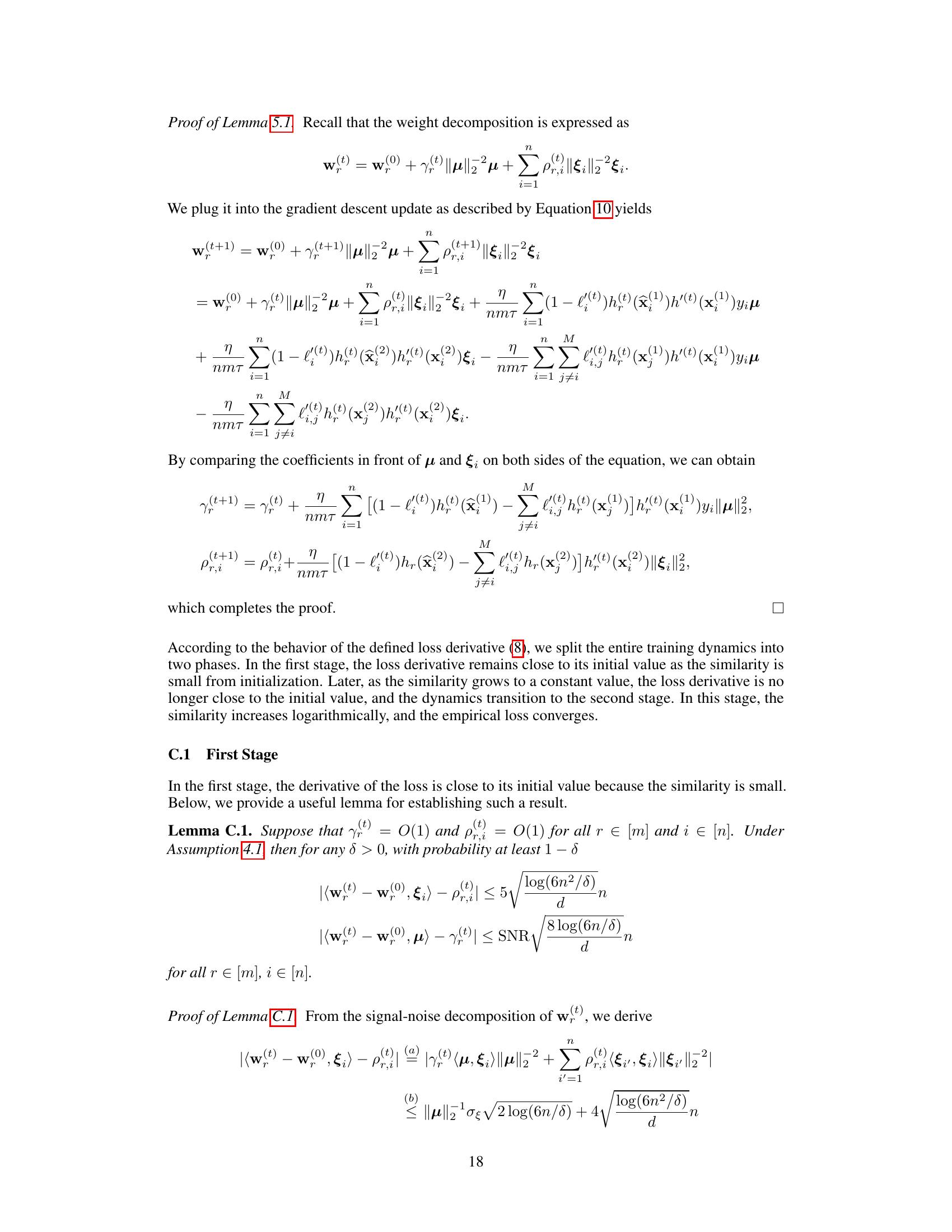
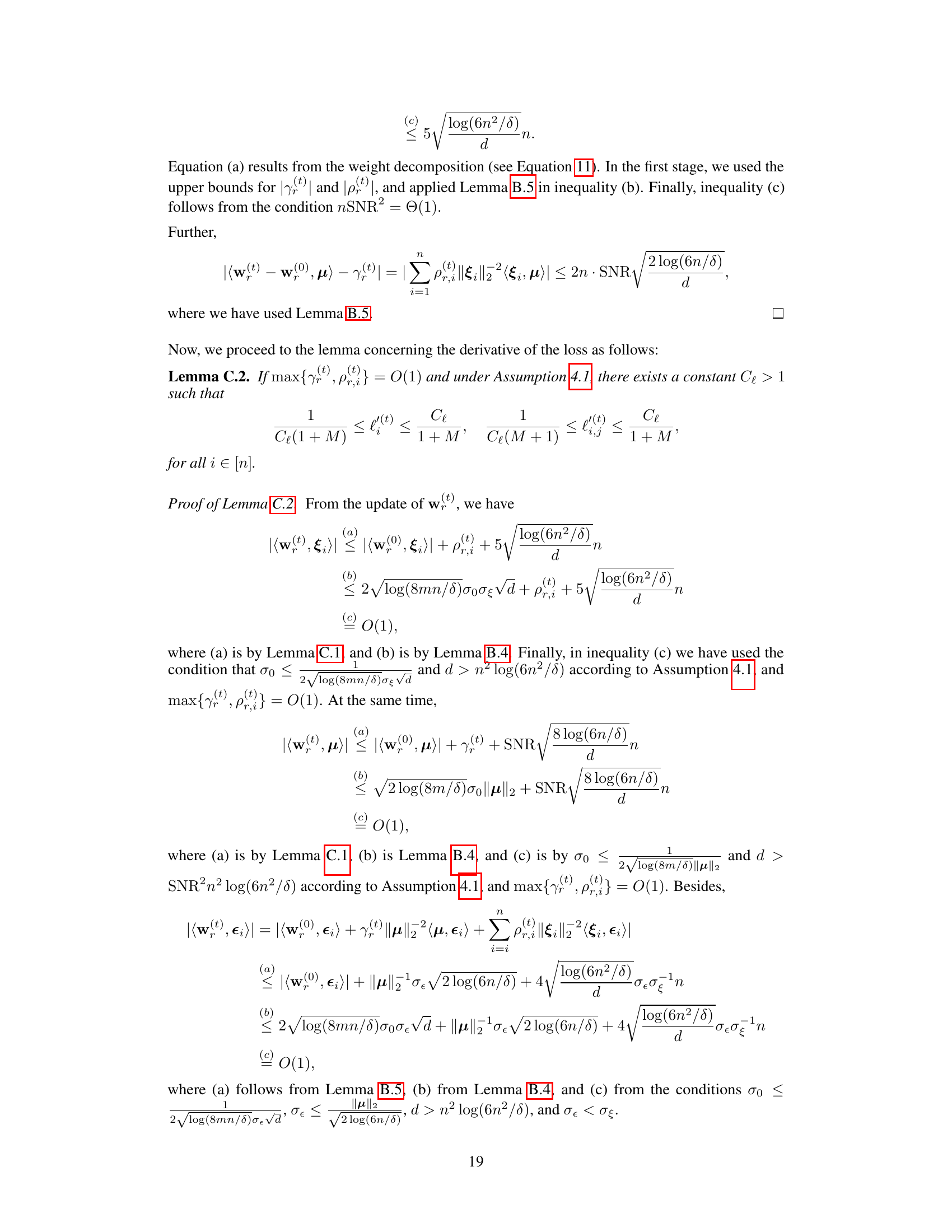
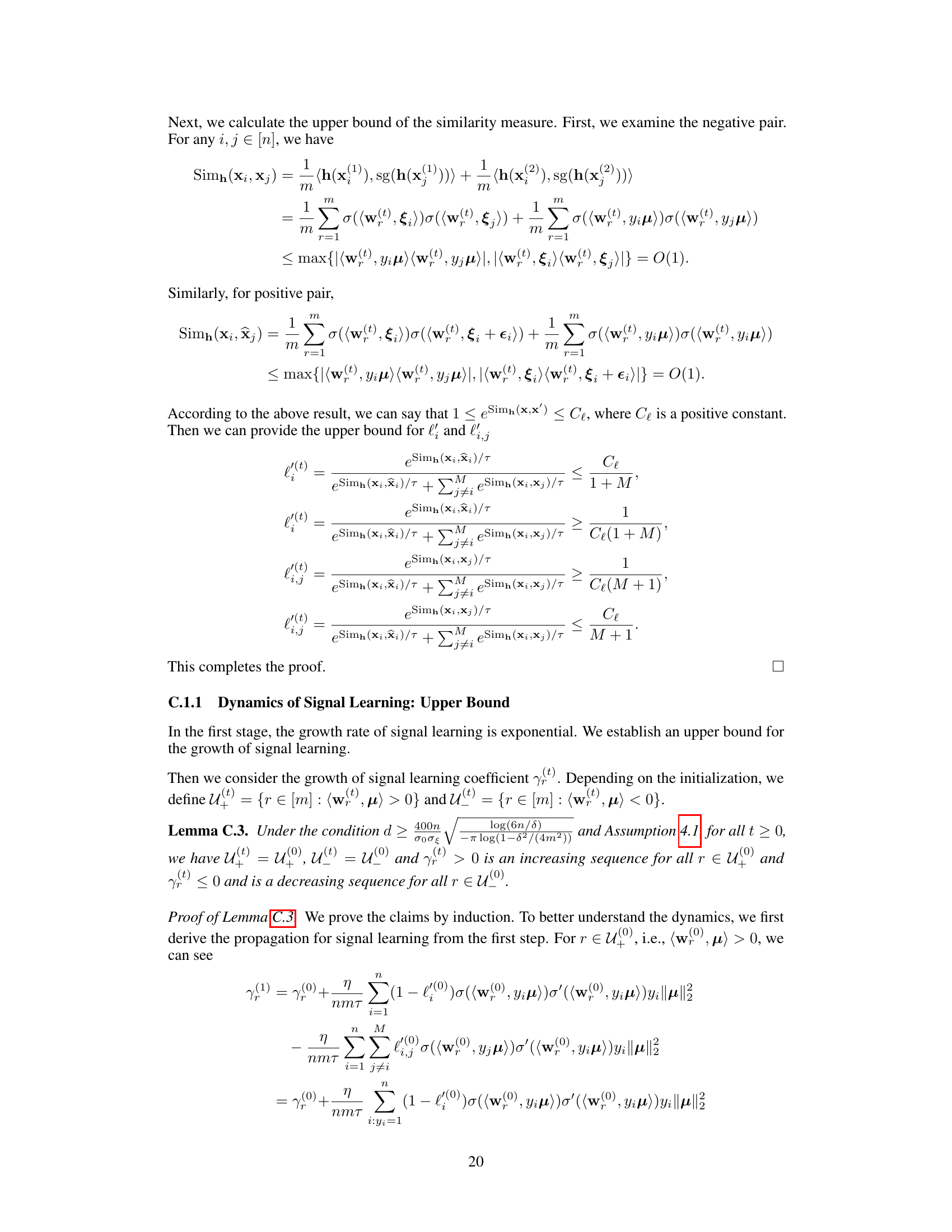