↗ OpenReview ↗ NeurIPS Homepage ↗ Chat
TL;DR#
Constrained Markov Decision Processes (CMDPs) are crucial for incorporating safety and other critical objectives in reinforcement learning, but current methods struggle with function approximation and high-dimensional state spaces. Existing approaches often lack sample efficiency or are computationally expensive, particularly when dealing with infinite state spaces and the need to ensure strict constraint satisfaction. This limitation hinders the application of CMDPs in practical, real-world scenarios.
This paper introduces Confident-NPG-CMDP, a novel primal-dual algorithm that leverages a local-access model and function approximation to efficiently solve CMDPs. The algorithm’s key strength lies in its carefully designed off-policy evaluation procedure, which ensures efficient policy updates using historical data and enables it to achieve polynomial sample complexity. The authors demonstrate that Confident-NPG-CMDP provides strong theoretical guarantees, successfully handling model misspecifications while satisfying constraints and achieving near-optimal policies.
Key Takeaways#
Why does it matter?#
This paper is crucial because it’s the first to achieve polynomial sample complexity for solving constrained Markov decision processes (CMDPs) with function approximation, a significant challenge in reinforcement learning. This breakthrough opens new avenues for safe and efficient AI development in complex, real-world scenarios.
Visual Insights#
Full paper#
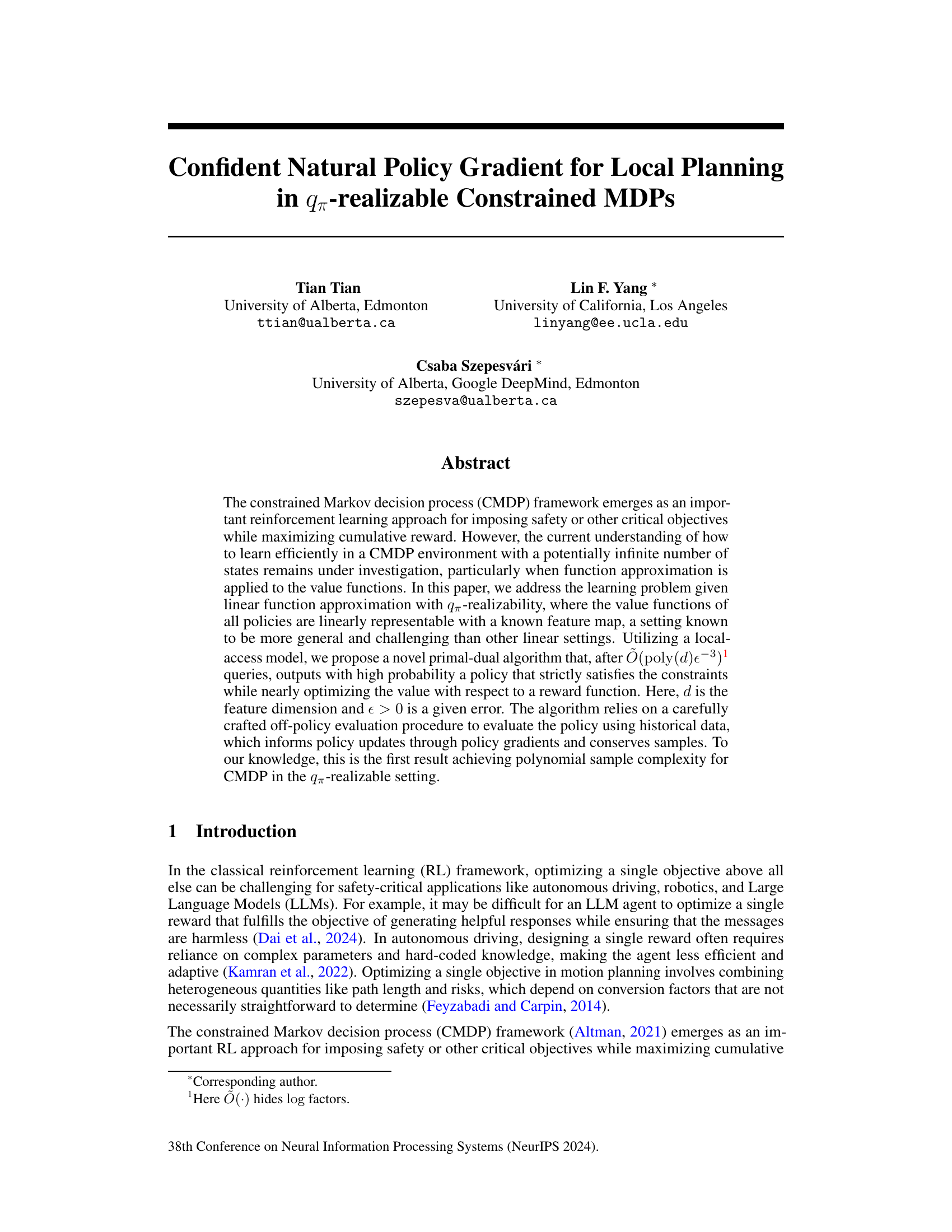
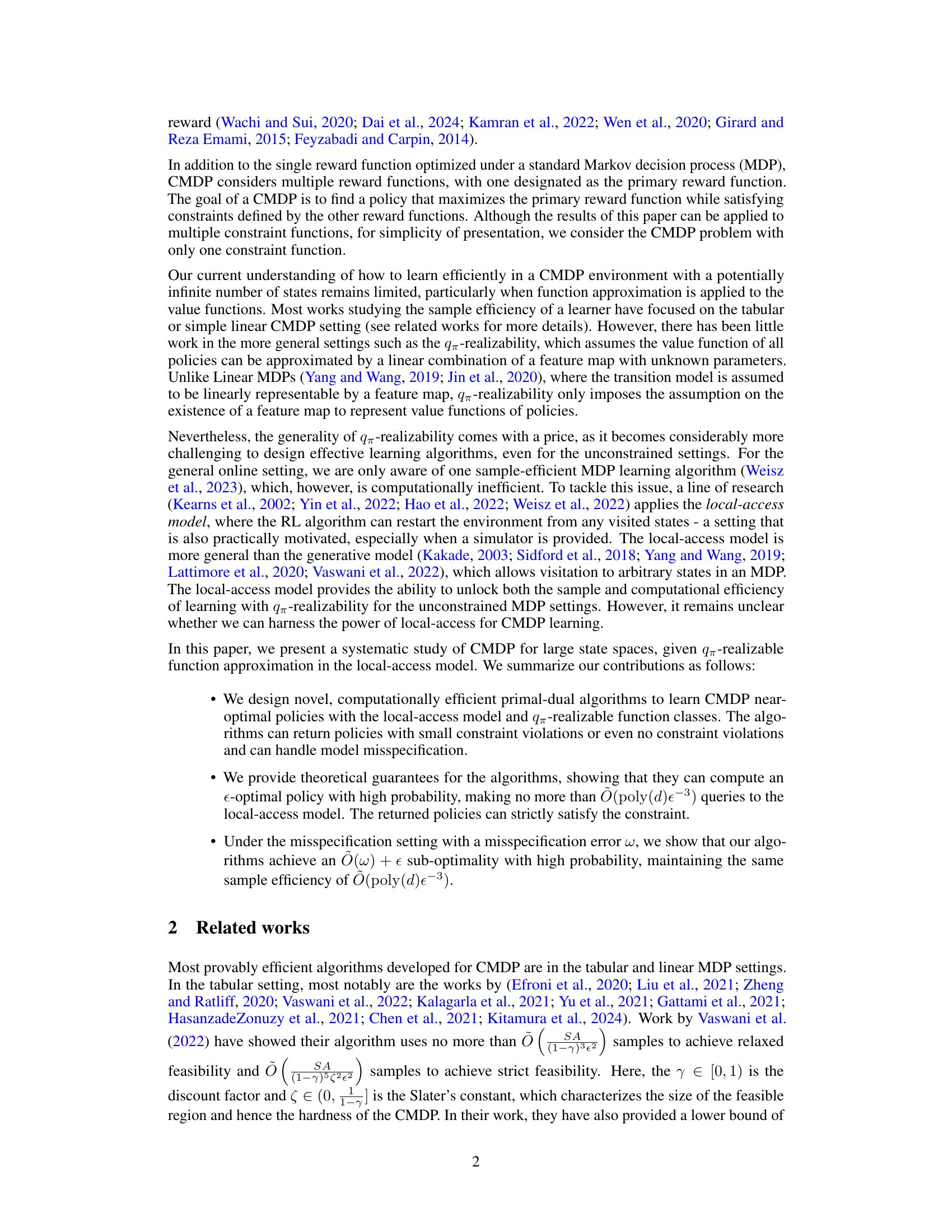
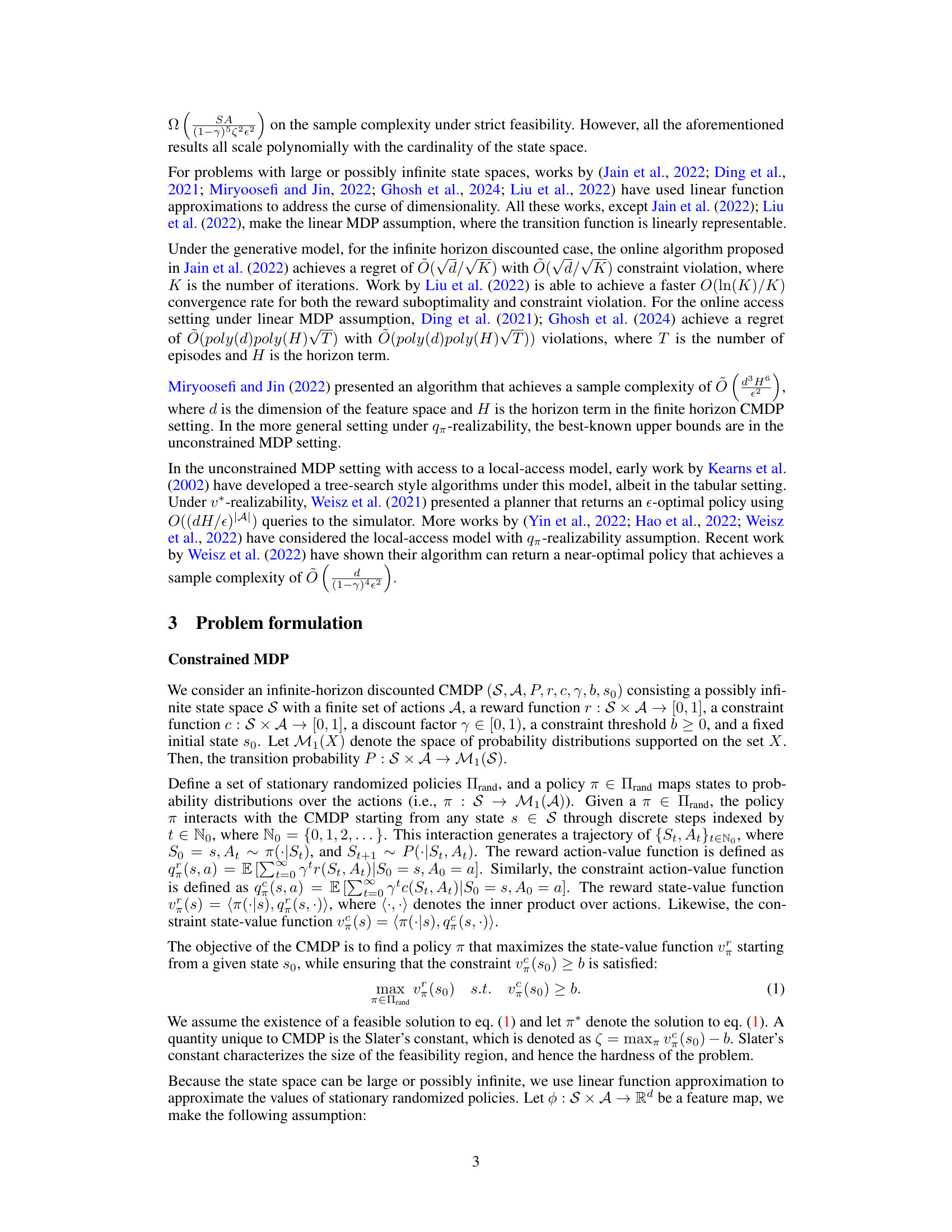
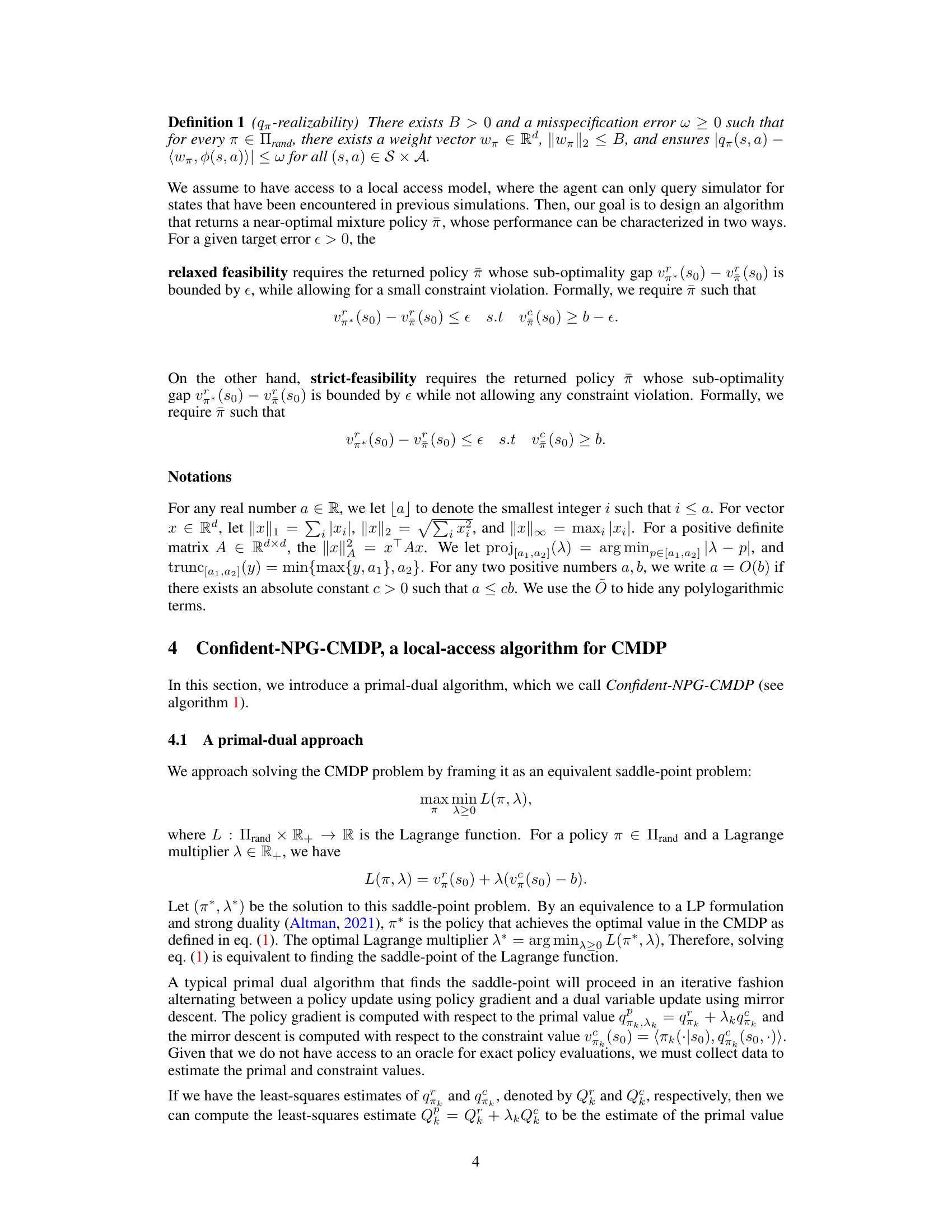
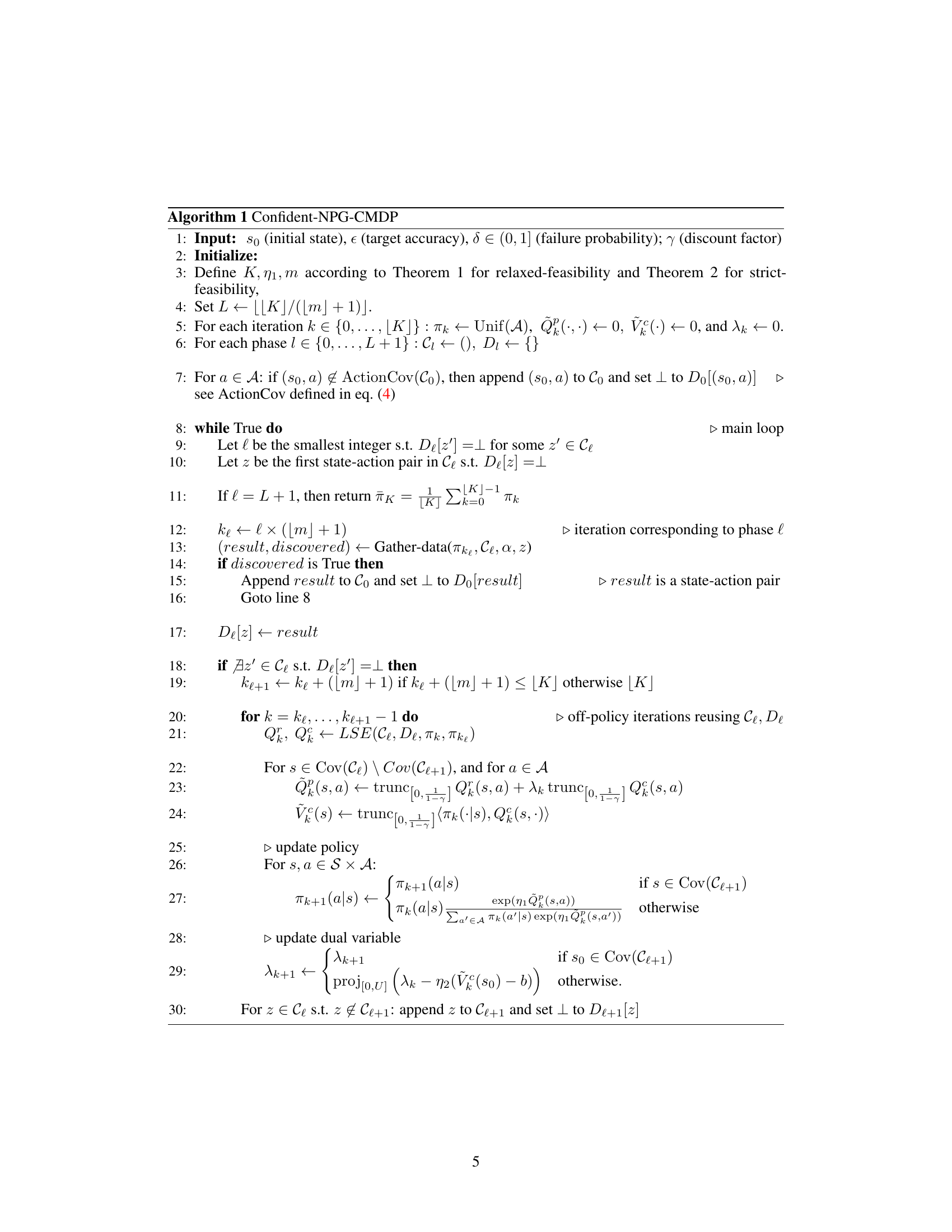
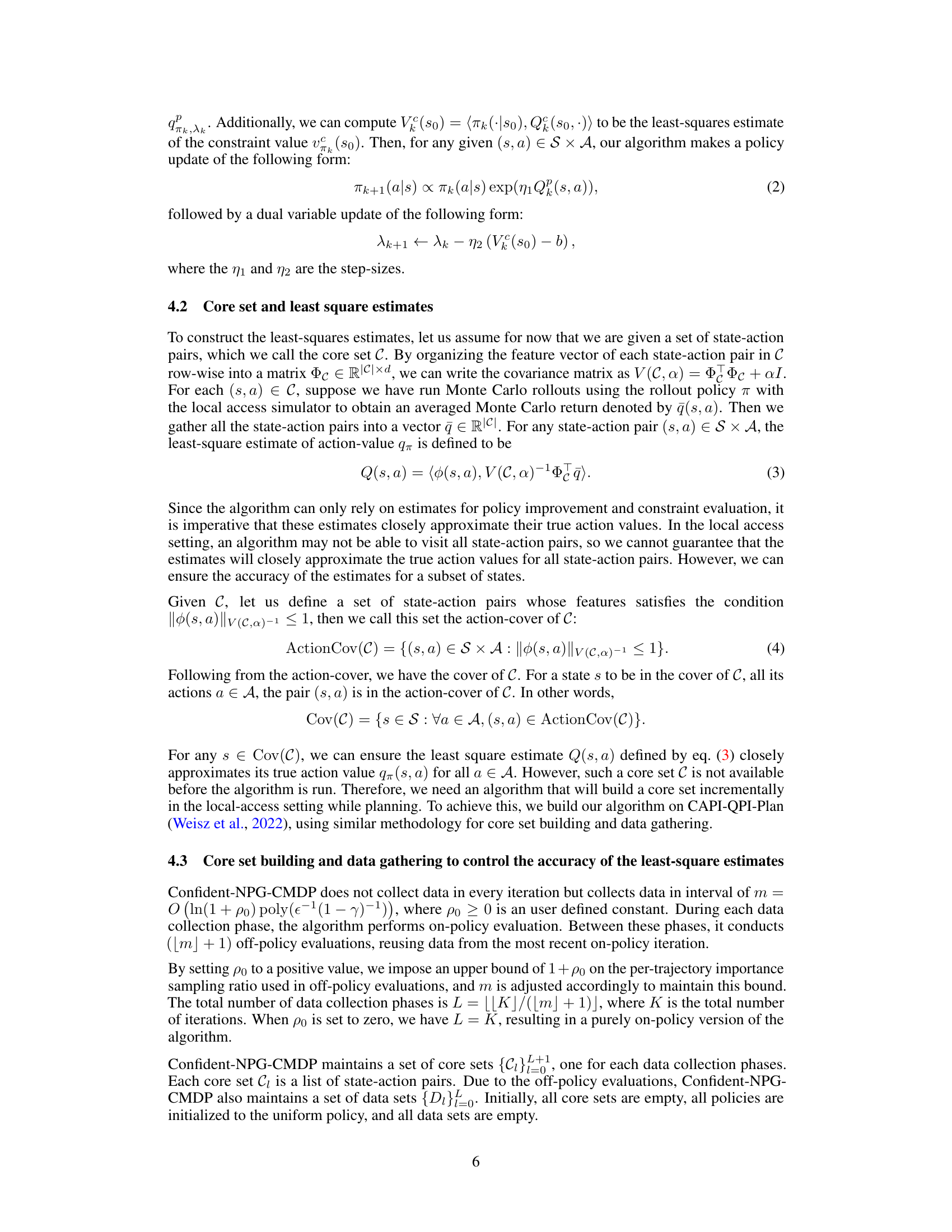
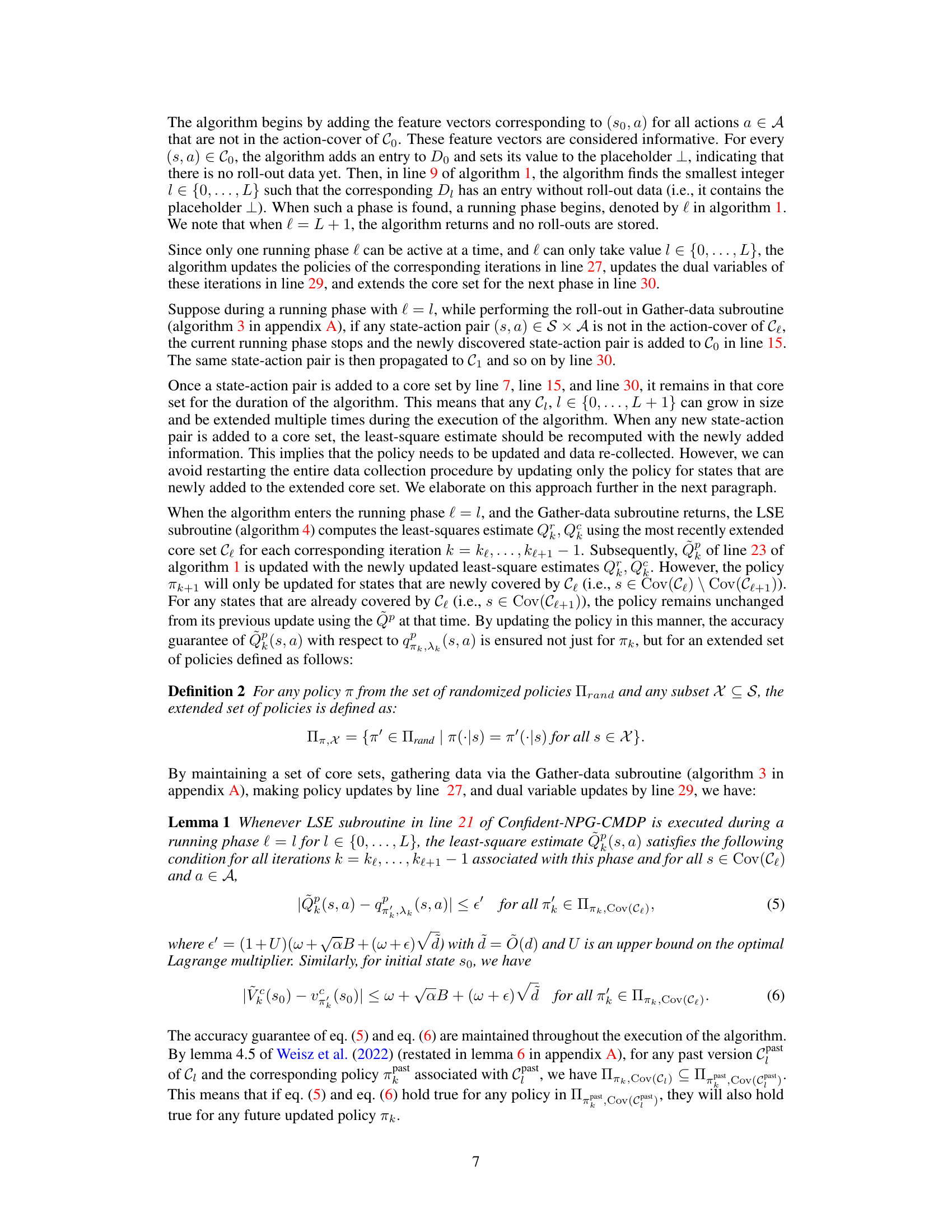
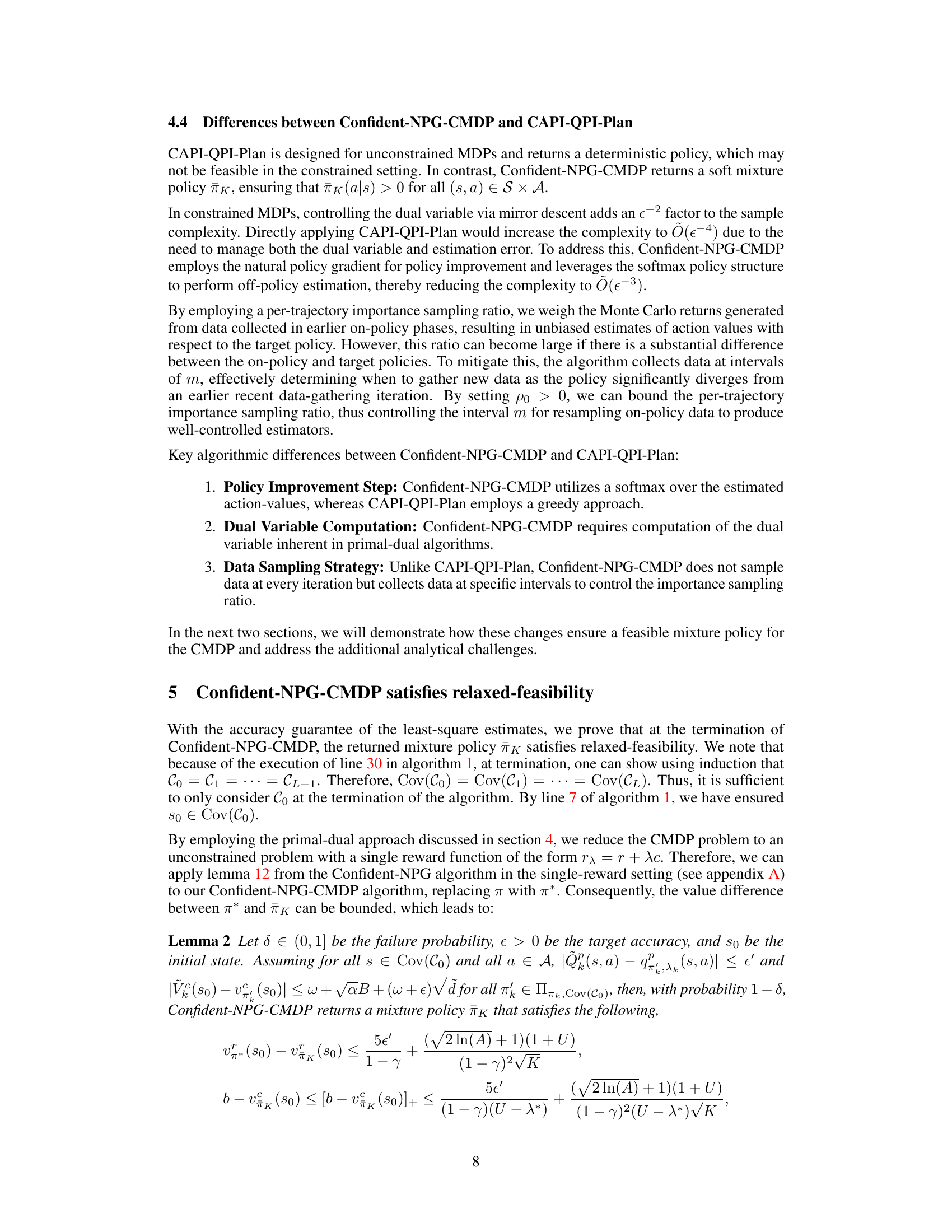
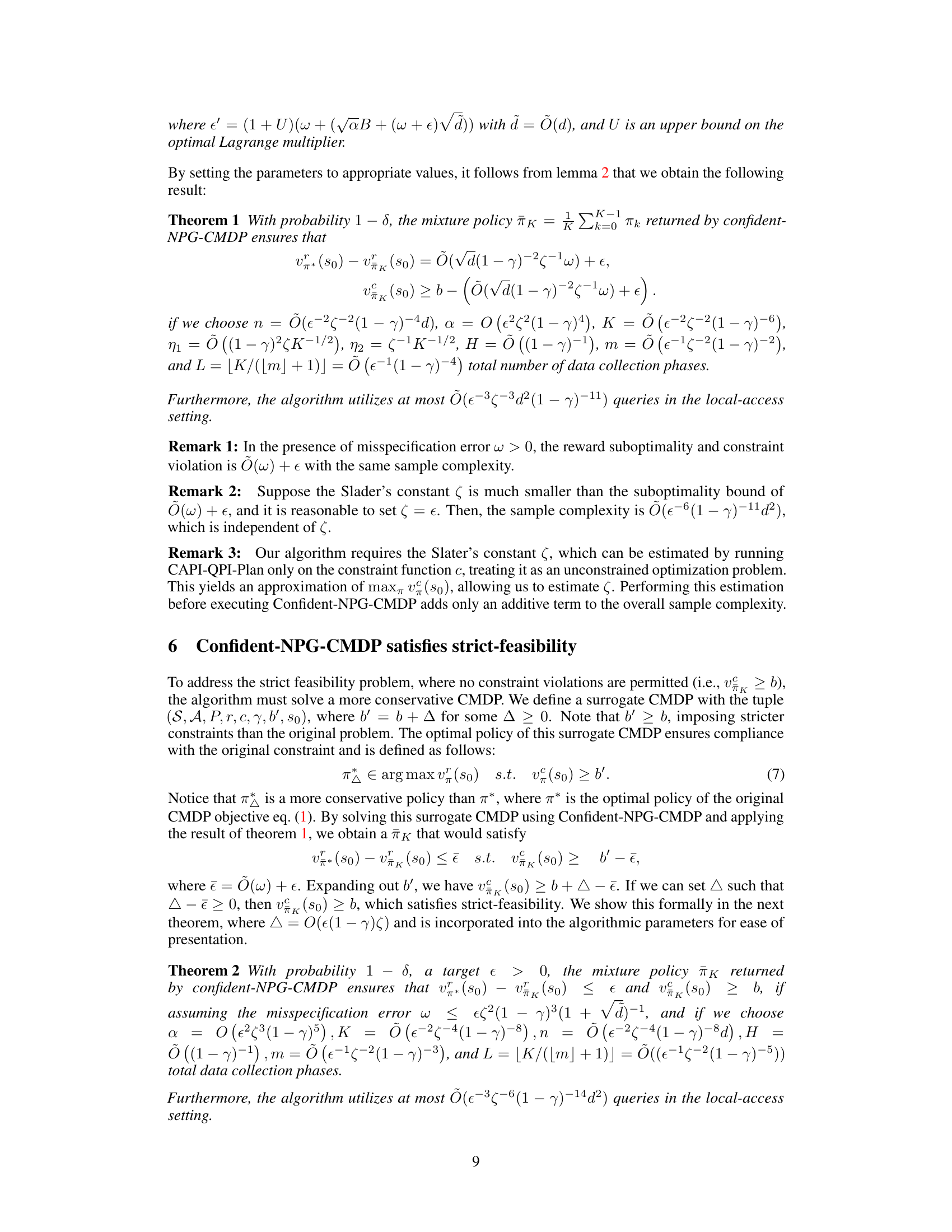
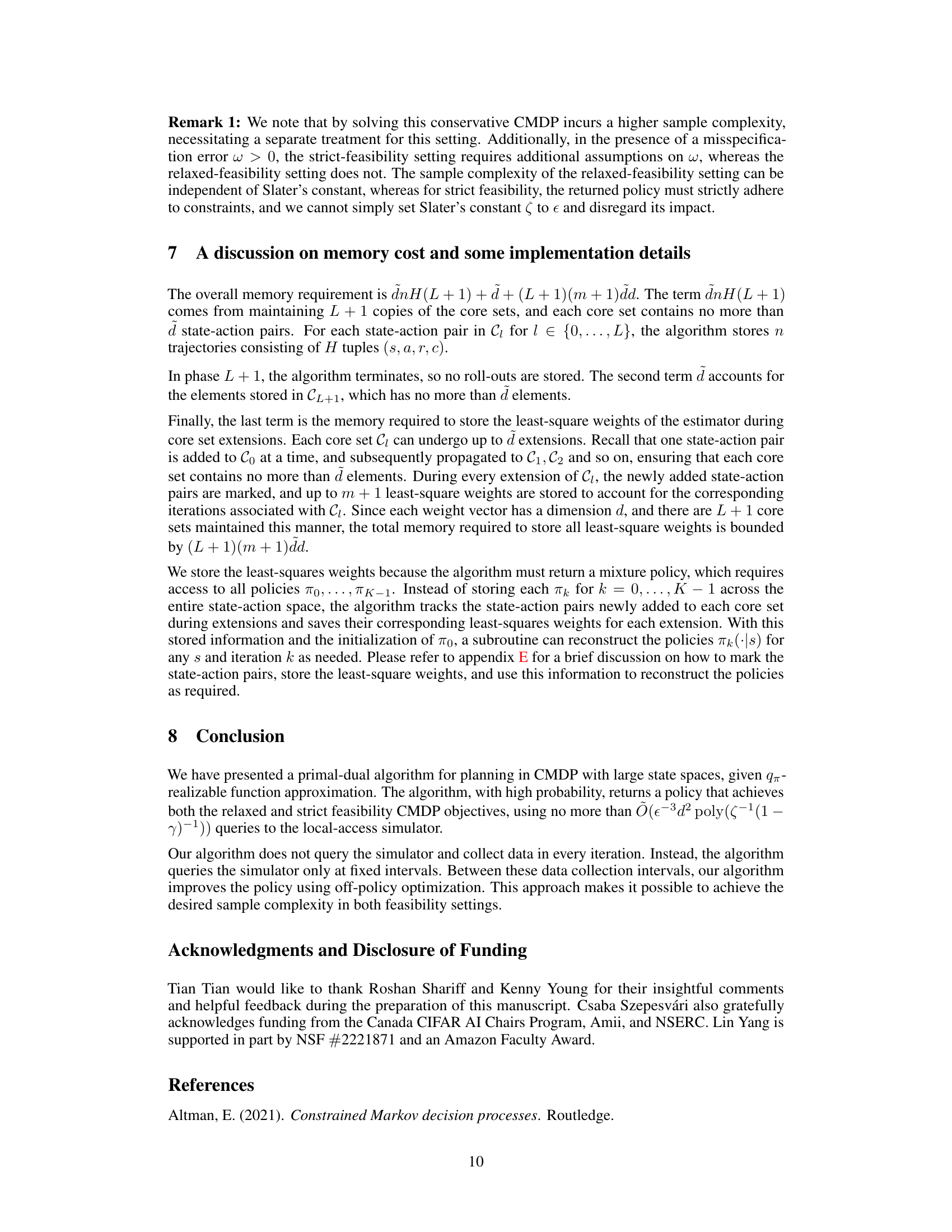
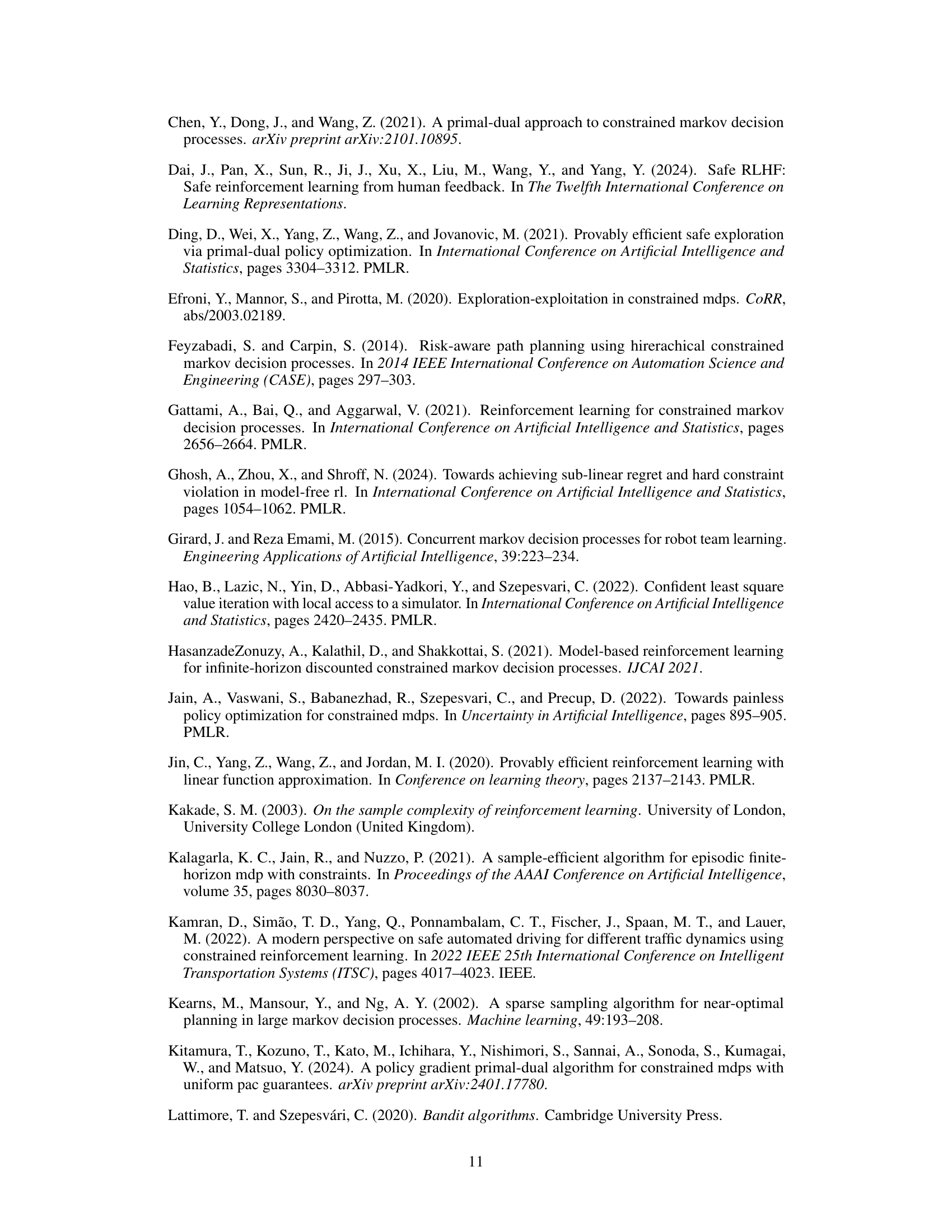
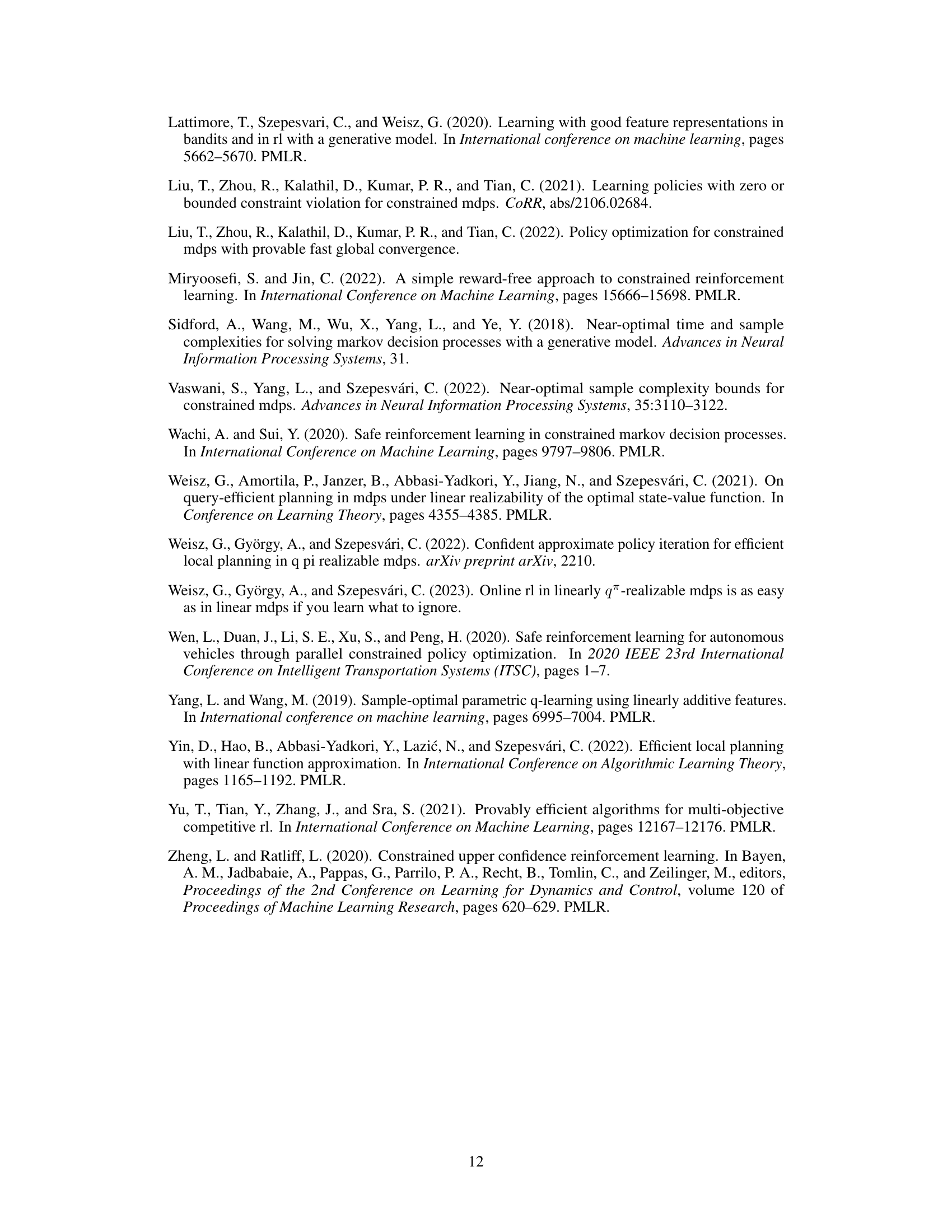
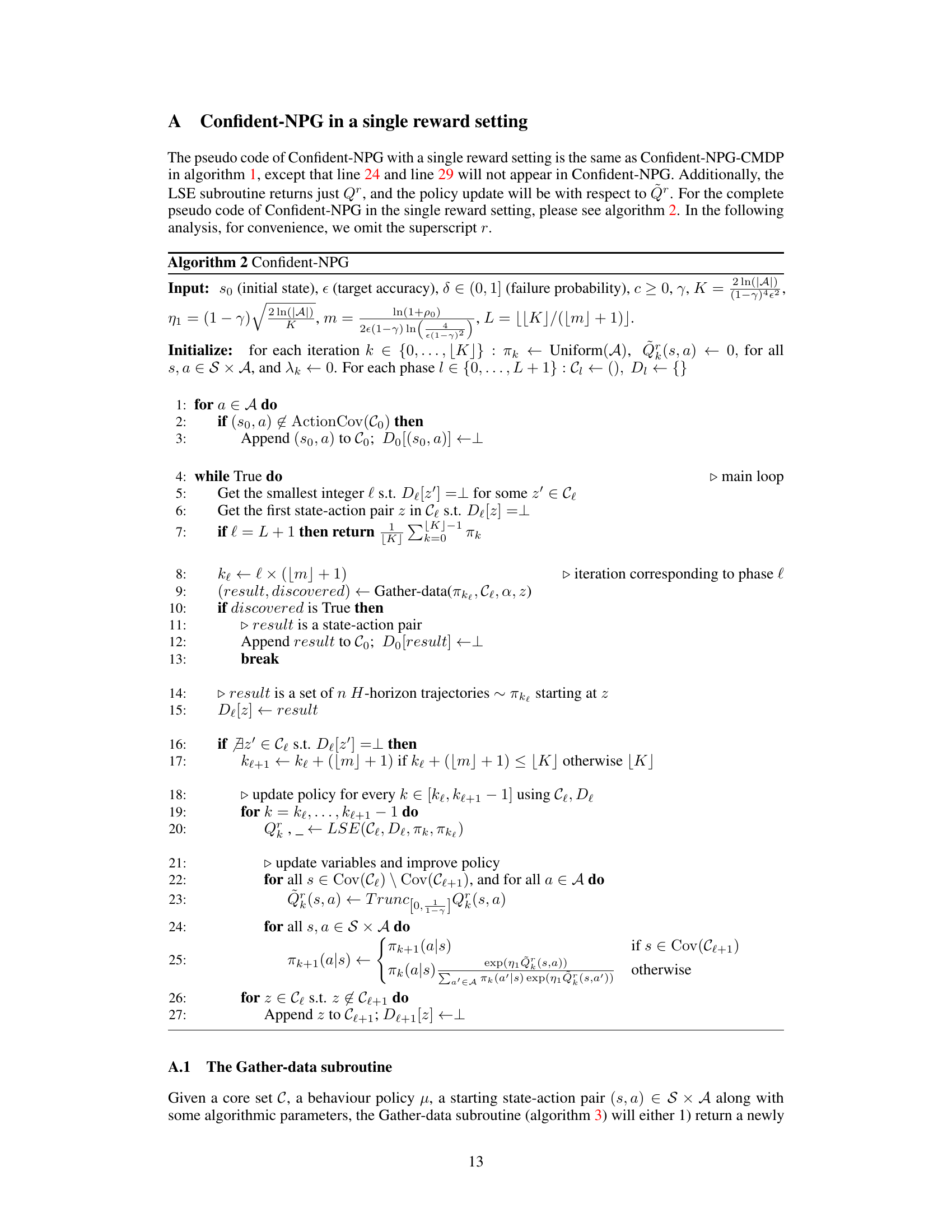
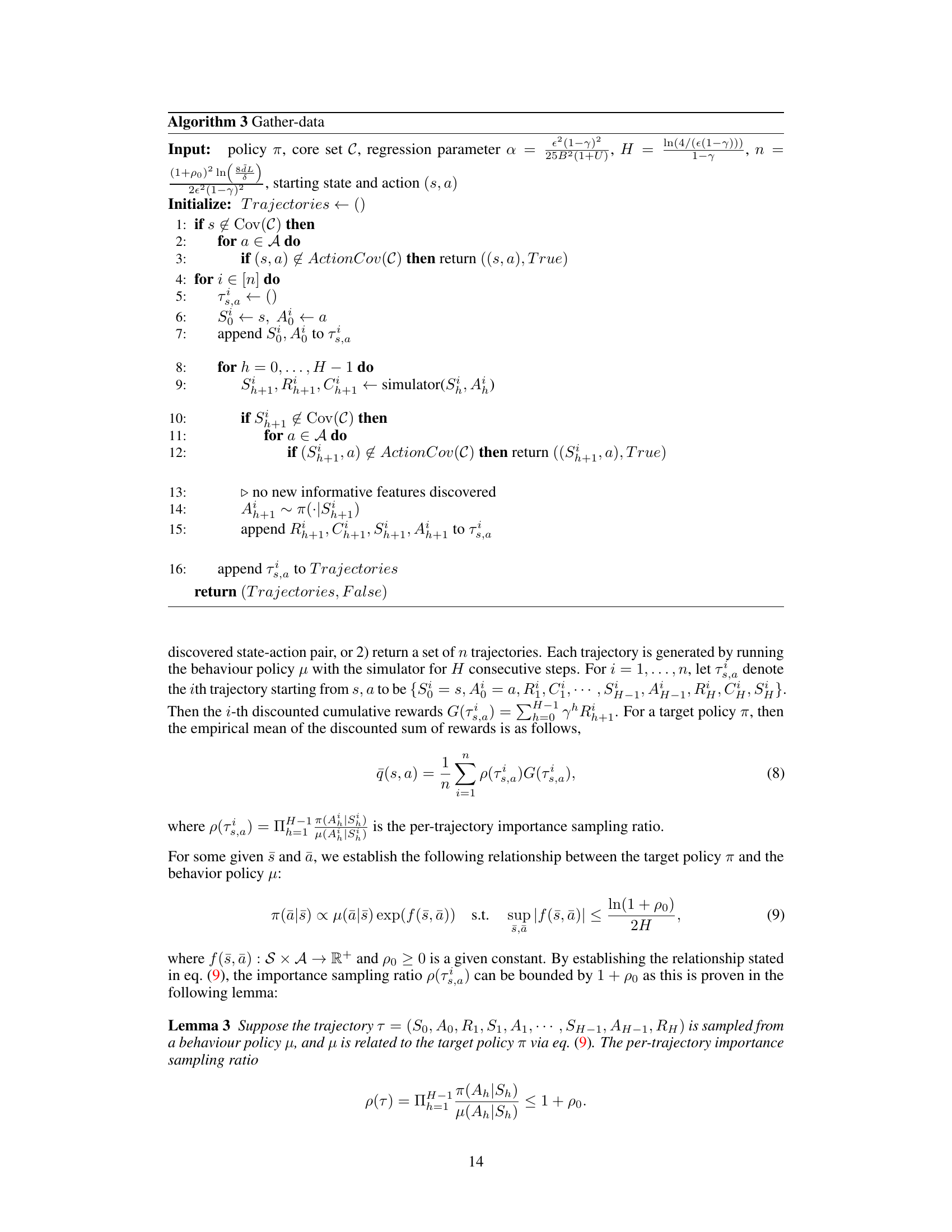
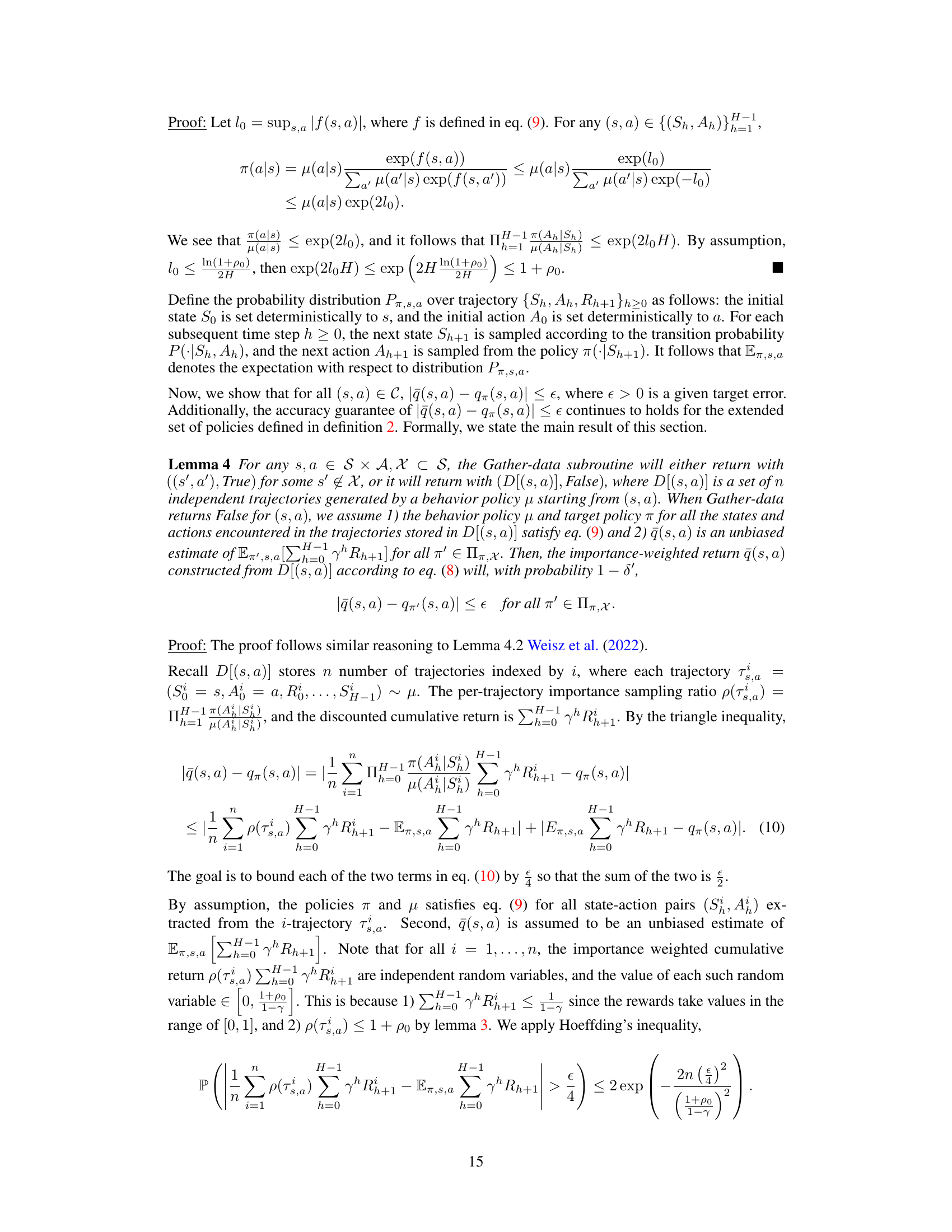
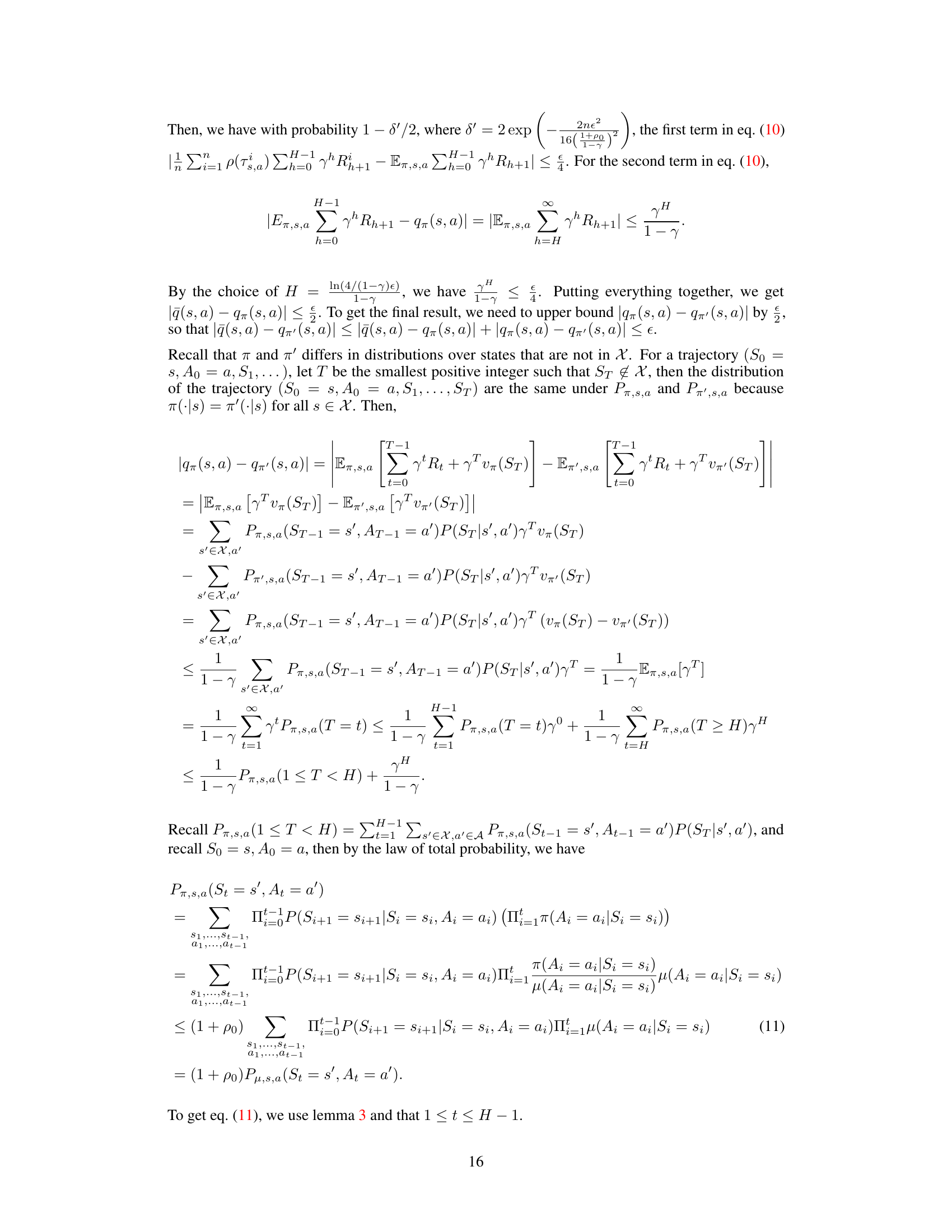
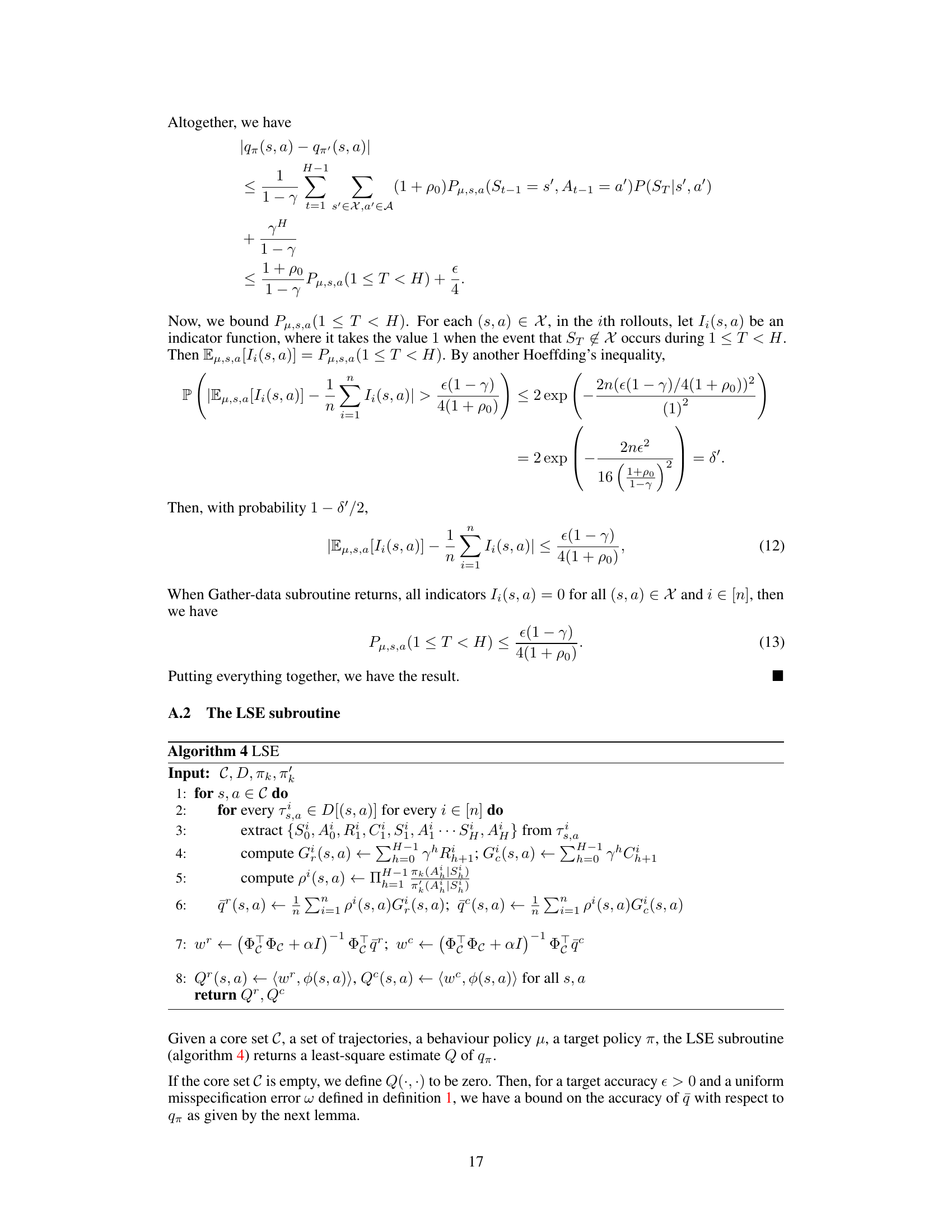
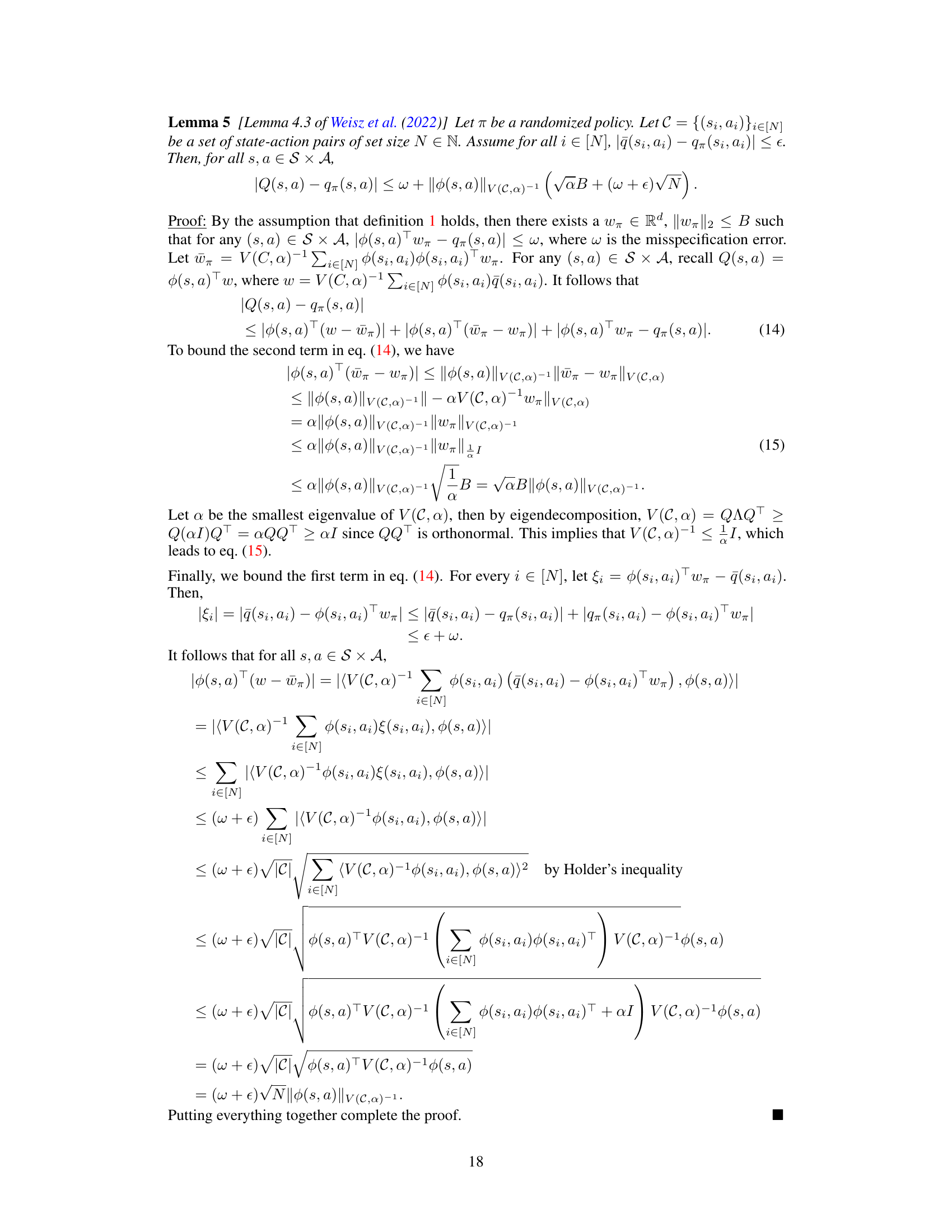
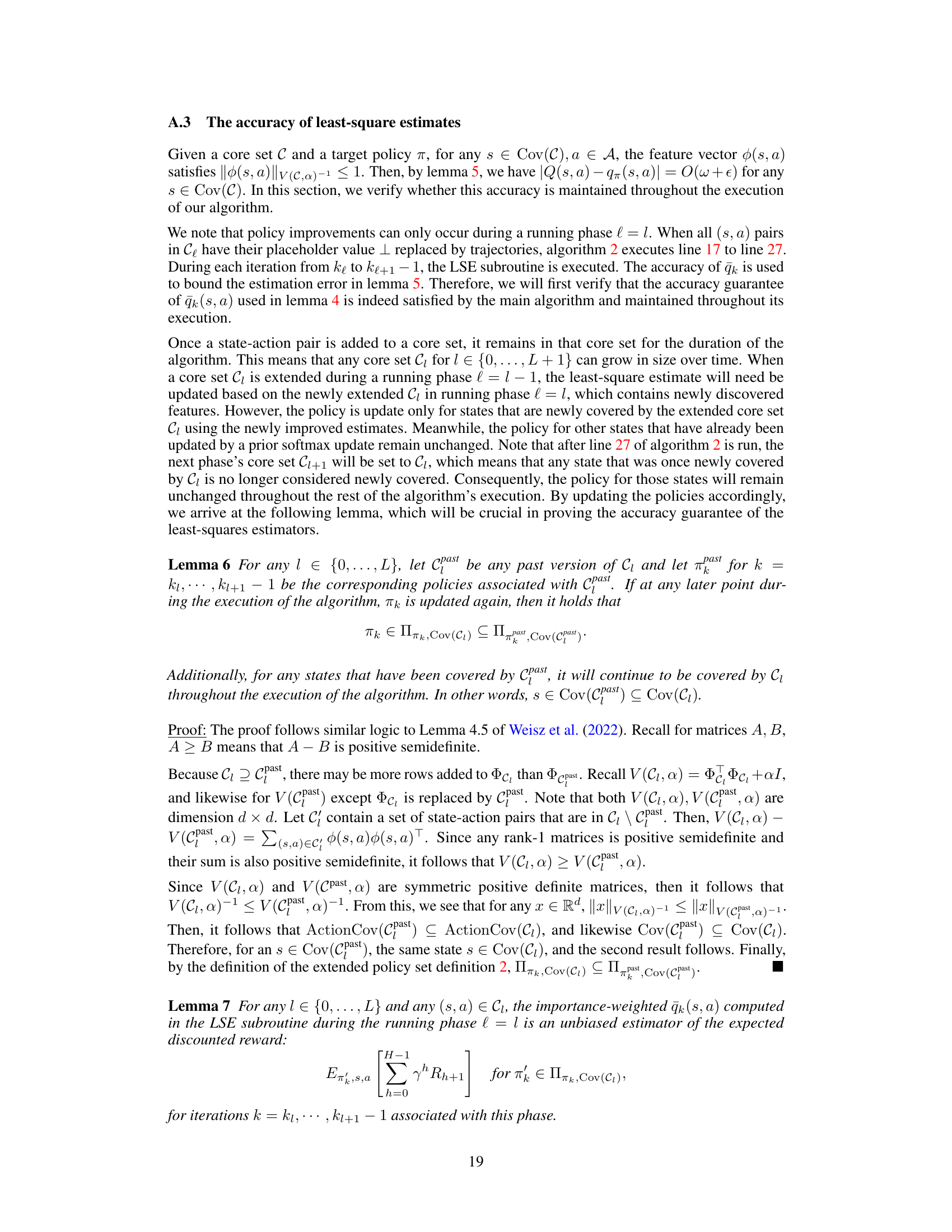
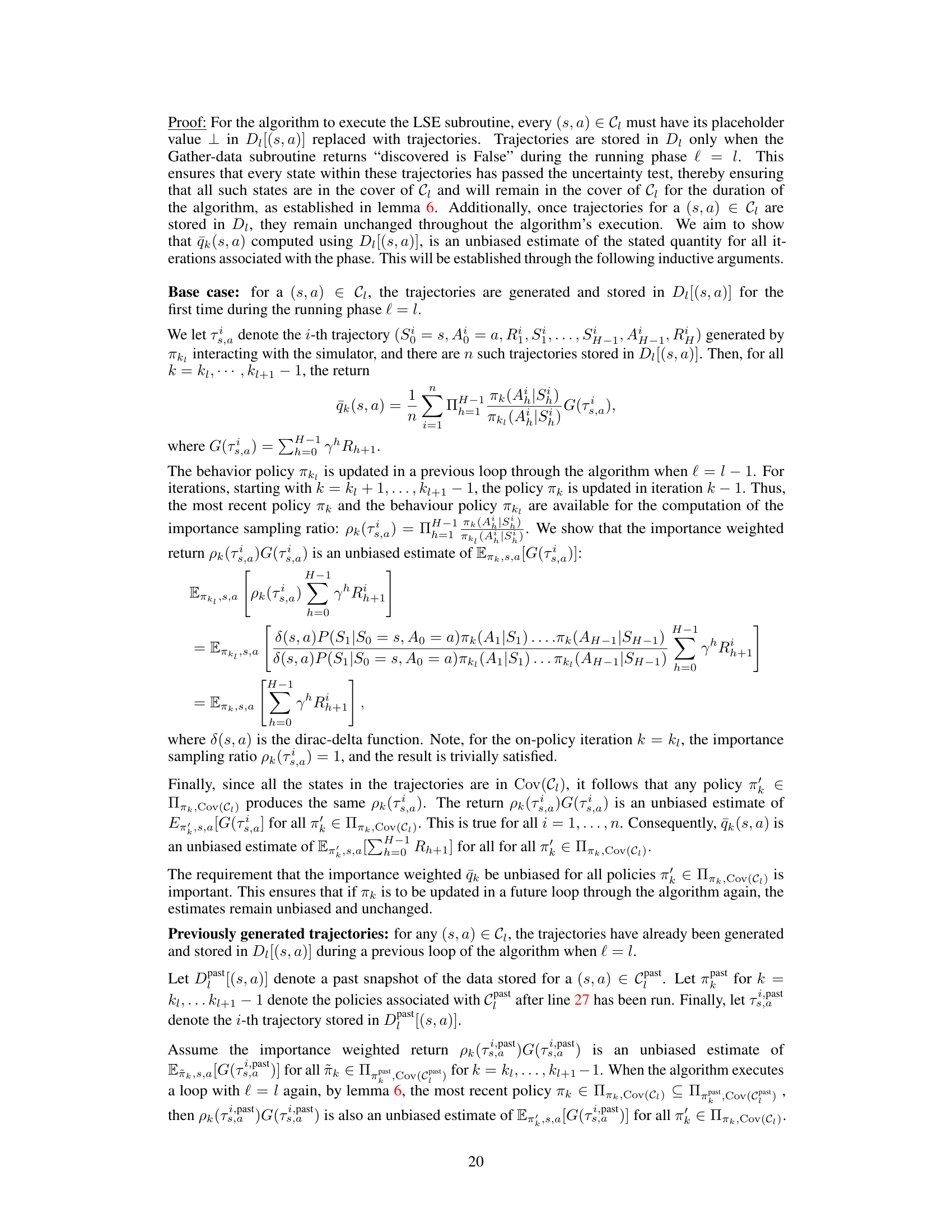